AI-Powered PDF Translation now with improved handling of scanned contents, handwriting, charts, diagrams, tables and drawings. Fast, Cheap, and Accurate! (Get started for free)
7 Essential AI Translation Skills New Graduates Need for Career Success in 2025
7 Essential AI Translation Skills New Graduates Need for Career Success in 2025 - Mastering OCR Translation Outputs Through Tesseract Engine Basics
In the evolving landscape of AI-powered translation, the ability to effectively process images and extract meaningful text is becoming increasingly vital. Tesseract, an open-source OCR engine, provides a powerful foundation for achieving this. Its ability to handle over a hundred languages, combined with its advanced deep learning capabilities (like LSTM), makes it a versatile tool for handling multilingual OCR tasks. Graduates can go beyond simply extracting text from images; Tesseract can be trained on customized data, adapting to the nuances of various document types and languages. By integrating this OCR with Python translation tools, users can quickly and efficiently translate the extracted text into other languages. As the need for swift and accurate multilingual translations increases, proficiency in leveraging Tesseract, possibly in conjunction with image processing, will prove indispensable for securing future opportunities in the field. Understanding how to refine Tesseract's settings, such as page segmentation and recognition modes, allows for tailoring the engine to specific needs. This core skillset, in conjunction with continuous learning in AI and machine learning, will be invaluable in establishing a competitive edge in the 2025 translation job market.
Tesseract, initially developed by HP and later maintained by Google, is a valuable open-source OCR engine that handles over 100 languages. This makes it a potentially strong tool when we're dealing with documents in various writing systems, especially in a translation context. While it excels at turning images into editable text, its accuracy depends heavily on the image quality. Poorly resolved images or distracting backgrounds are often major sources of error, potentially affecting the subsequent translation's correctness.
Recent iterations of Tesseract have incorporated advanced techniques like binarization and deskewing, improving the pre-processing of images before OCR. This cleaning and straightening process can significantly help the engine recognize text better by providing cleaner input data. A unique feature of Tesseract is its use of machine learning. Essentially, it learns from the documents it processes, gradually getting better at understanding different fonts and page layouts.
This potential for improvement through experience can be incredibly helpful in reducing errors and enhancing the overall translation quality over time. Using Tesseract to translate documents can streamline the process and save considerable time compared to doing it all manually. This is particularly true when dealing with lots of text, which is quite common in many translation tasks.
Integrating Tesseract into our translation processes not only impacts accuracy but can help speed things up. Translators can focus more on the actual meaning and nuances of the text rather than tediously extracting it first, leading to potentially better translations. Although Tesseract can handle complex layouts like tables and multiple columns, it can struggle with very structured documents. Here, some extra pre-processing and understanding document structure can improve results.
As a command-line tool, we can easily automate Tesseract within broader translation systems or workflows, allowing us to handle large numbers of documents in batch mode. This can greatly enhance efficiency in translation projects. However, Tesseract is often criticized for its performance with handwritten text. The reason is that handwriting styles are far more diverse than printed text, creating a major challenge for consistent accuracy.
Researchers are currently working on improving Tesseract by using more sophisticated neural network methods. They hope this will allow the engine to capture more contextual details and language nuances across different languages. In the translation space, this kind of improvement is crucial, and we can anticipate this area of research to continue growing in importance in the coming years.
7 Essential AI Translation Skills New Graduates Need for Career Success in 2025 - Understanding Neural Machine Translation Accuracy Limits
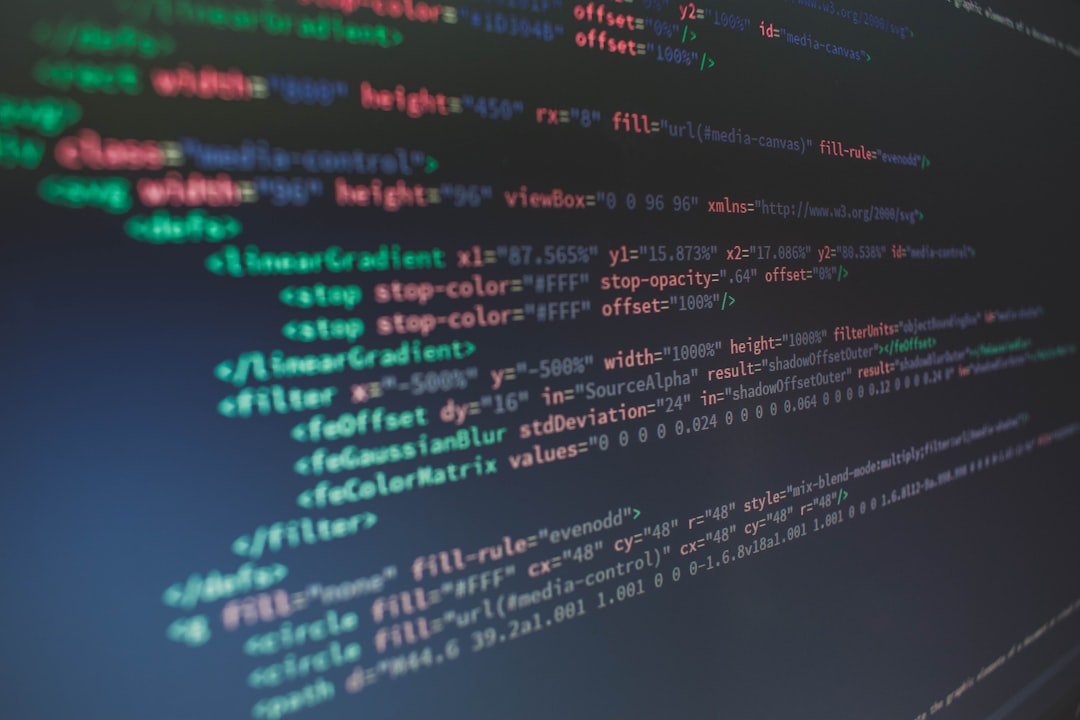
Understanding the limitations of Neural Machine Translation (NMT) is crucial for anyone entering the AI translation field in 2025. While NMT systems are impressive in their ability to translate quickly and cheaply for specific tasks, they can struggle with complex linguistic features. For example, translating sentences that rely on unique grammatical structures or cultural nuances in a particular language can be challenging for these systems. They are trained on huge datasets, but this doesn't always mean they get the meaning or context of a sentence perfectly right. Consequently, you might end up with translations that are not entirely grammatically accurate or don't fully convey the original meaning.
As AI continues to change how we translate, it's becoming increasingly important for new graduates to be aware of these challenges. This awareness is vital to help them make the most of AI-powered translation tools while also recognizing their limits. By understanding both the strengths and weaknesses of these tools, future translators will be better equipped to handle a wider variety of translation scenarios. This balanced approach allows graduates to integrate AI into their workflow efficiently and critically, making them more adaptable and successful in the evolving translation landscape.
While neural machine translation (NMT) has brought significant advancements in speed and cost-effectiveness, it's important to acknowledge that its accuracy isn't without limitations. These systems often struggle with sentences that have multiple meanings or rely on culturally specific idioms. This makes them less suitable for tasks that require a deep understanding of language nuances, like literary translation.
The accuracy of NMT can vary greatly depending on the languages involved. When translating between languages with vastly different grammatical structures, or those without shared linguistic roots, it's more likely to encounter systematic errors. This could be due to the model's difficulty in capturing complex relationships between words in different languages.
Another issue is that, unlike human translators, NMT systems don't have the ability to remember context throughout a longer piece of text. This can lead to situations where a translation might make sense in isolation but doesn't flow logically with the preceding sentences. This becomes particularly problematic when handling longer texts or documents.
Furthermore, specialized terminology or rare words can create major hurdles. If a NMT model doesn't encounter a particular word or phrase frequently enough during training, it might struggle to understand its meaning. This is a major concern when translating technical or highly specialized materials.
Real-time translation, although incredibly useful, often comes at the expense of accuracy. The need for speed can lead to simplifications and errors, especially when dealing with complex or lengthy sentences. Researchers are continuously working to find the best balance between speed and accuracy.
Dialects are another obstacle. These models are typically trained on standard forms of languages, making them less capable of handling regional variations or informal language. This can result in translations that feel artificial or don't accurately convey the intended tone.
It seems that even the most advanced NMT models still benefit greatly from human intervention. Studies have shown that adding a human editing step can significantly improve translation quality. This highlights the fact that there's still a long way to go before machine translation fully replaces human translators in many contexts.
The quality of training data heavily impacts NMT performance. If the datasets contain inaccurate translations or are poorly structured, the resulting model will inherit these flaws and suffer reduced accuracy.
It's also important to note that NMT lacks a true understanding of cultural contexts. This can lead to translations that fail to capture the subtleties and nuances of cultural references or expressions. This makes it challenging to convey the original message accurately, sometimes resulting in translations that feel mechanical or lack depth.
Finally, NMT's performance also depends heavily on the availability of resources for the languages involved. Languages with limited online presence or digital data resources tend to have models that perform significantly worse. This creates an interesting imbalance in the effectiveness of translation technology across different language communities.
In conclusion, while NMT has revolutionized translation in many ways, we need to be mindful of its limitations and acknowledge that human expertise still plays a crucial role in ensuring accuracy and cultural sensitivity in many translation scenarios. It will be fascinating to see how these technologies develop and adapt in the future.
7 Essential AI Translation Skills New Graduates Need for Career Success in 2025 - Fast Batch Processing Skills for Large Translation Projects
The increasing use of AI in translation has made the ability to quickly process large volumes of text a vital skill. New translators need to become proficient in using advanced translation software and systems that can handle many languages at once and automate parts of the translation process. While traditional language skills are still essential, aspiring translators must also develop strong technical skills, like a good understanding of how translation software works. This includes being comfortable with various file formats and managing large translation projects through sophisticated tools.
Furthermore, recognizing the limitations of AI-powered translation is crucial. These systems, while helpful, can sometimes miss important contextual clues or cultural nuances in the source text. Therefore, new graduates must learn to use these tools strategically, integrating their own linguistic expertise to ensure high-quality translations. This combination of human skills and technological proficiency is essential for future translators to meet the demands of fast-paced translation environments. As the need for quicker and more accurate translations across multiple languages increases, mastering batch processing techniques and using translation tools effectively will be key to finding success in the translation field in the coming years.
Handling large translation projects efficiently is increasingly important, and fast batch processing skills are becoming crucial. Tools like Transifex showcase the potential of AI-powered Translation Management Systems (TMS) to automate and streamline these processes. We're seeing a significant reduction in translation time, which allows businesses to communicate faster across languages. However, while tools like Transifex offer impressive potential, the ability to manage large batches of translations effectively requires a strong foundation in software, data management, and efficient workflow design.
A key aspect of this is parallel processing. Splitting large translation jobs across multiple computers significantly speeds things up and lets us handle enormous document volumes. But this speed comes with a tradeoff – we risk a higher probability of errors slipping through. Humans tend to overlook small inconsistencies when dealing with large datasets, making it important to have rigorous quality checks in place. The nature of the languages being translated also matters. Languages with complex grammar or highly specialized vocabularies often require more pre-processing, affecting the overall batch processing time.
OCR, while valuable, can be a bottleneck in these processes. Its accuracy strongly depends on the input image quality, meaning even small imperfections can create significant translation errors, especially when dealing with huge document sets. Automating translation workflows can be very beneficial, but we have to be mindful of potential risks. If the system misidentifies or misinterprets documents, it can cascade errors through multiple translations before we notice.
It's crucial to train AI systems with data tailored to the specific needs of each translation project. Custom training data can significantly improve the quality of translation in specialized areas like law or medicine where accurate terminology is essential. Comparing the performance of different tools using benchmarks is a necessary step in choosing the most appropriate tools for a particular task. Speed and accuracy will vary, and these differences can indicate the value of exploring different technologies or integrating tools in innovative ways.
Working with multilingual documents in batch mode introduces a new set of challenges. Each language often needs different processing approaches, and if not managed correctly, it can increase complexity and potentially lead to problems. Cultural nuances are another area where fast batch processing can struggle. Systems may miss subtle meanings embedded in the text, highlighting the need for human reviewers, particularly for sensitive materials.
As AI continues to revolutionize translation, mastering the art of fast, accurate batch processing will become increasingly vital for anyone hoping to succeed in the translation industry. The field is evolving rapidly, and new graduates need to stay updated on the latest tools and technologies to remain competitive in the dynamic world of AI translation.
7 Essential AI Translation Skills New Graduates Need for Career Success in 2025 - Post Editing Machine Translation Without Quality Loss
In the rapidly evolving landscape of AI-powered translation, post-editing machine translation (MTPE) has become a vital skill for new graduates aiming for career success in 2025. This technique combines the speed of automated translation with the precision of human review to achieve a balance of efficiency and accuracy.
Essentially, MTPE involves humans refining machine-generated translations to ensure clarity, accuracy, and cultural appropriateness. There are different approaches to this refinement, such as light post-editing, which focuses on minor adjustments for quick turnaround, and full post-editing, which delves deeper into improving overall quality. New graduates need to understand the nuances of these methods to effectively choose the most appropriate approach for various projects, demonstrating their adaptability.
The rising prominence of machine translation has simultaneously increased the demand for skilled human editors who can bridge the inherent quality gap often found in AI-produced translations. The ability to critically evaluate machine output and refine it to a level that meets the specific demands of a client becomes a highly valued skillset. Thus, post-editing knowledge becomes central to any quality assurance process within translation workflows, assuring the success of projects where speed and accuracy are critical.
Essentially, mastering MTPE in 2025 will allow new graduates to navigate the ever-changing translation landscape, leveraging both cutting-edge AI tools and their own linguistic expertise. By understanding these post-editing techniques and their role within the translation process, new professionals can better ensure the quality of translations, thus positioning themselves for a successful career in this field.
In the world of AI-driven translation, achieving high-quality outputs often involves a blend of automated translation and human refinement. This process, known as Machine Translation Post-Editing (MTPE), is becoming increasingly important as the demand for quick and accurate translations across languages grows.
Machine translation engines like Google Translate or DeepL provide initial drafts, but these often require human intervention to achieve the desired quality level. The ISO 18587:2017 standard outlines the quality expectations for MTPE, guiding professionals in their work. Post-editors scrutinize the machine-generated translations, checking for clarity and correcting errors to make the text understandable and accurate.
There are different approaches to post-editing. Light Post-Editing (LPE) is focused on efficiency, aiming for swift revisions with minimal changes to ensure readability. Conversely, Full Post-Editing (FPE) prioritizes quality, striving for a translation that's nearly indistinguishable from a human-produced version. Post-editors need a solid foundation in translation, often supplemented with specific training to meet the standards set by ISO 18587.
Choosing the right post-editing approach is crucial, and it hinges on several factors: the time available for the project, the desired quality level, and budget considerations. These aspects influence the overall strategy employed in a translation project. As the global need for language services continues to rise, human post-editors play an essential role in guaranteeing the quality of machine-generated translations.
MTPE effectively bridges the gap between speed and quality in translation projects. It helps to increase the scalability of translation, allowing companies to tackle a wider range of translation tasks. Therefore, comprehending the MTPE workflow and related best practices is crucial for new graduates looking to carve a successful career in this field. It's an area that combines the efficiency of AI with the nuanced understanding that only a human translator can provide, demonstrating a synergistic approach that will continue to grow in importance as the translation landscape evolves.
However, it's worth noting that MTPE can be influenced by various factors. The languages involved in the translation can significantly impact the level of editing required, with languages having greater structural or grammatical differences often demanding more intensive post-editing. Additionally, specialized or technical topics may also require a greater focus on human refinement due to the nuances and subtleties that machine translation engines can sometimes miss. The availability of training data for a specific language pair can also play a role in the overall quality of the machine translation output and, consequently, the amount of post-editing needed to achieve the desired level of quality.
Despite the various challenges, MTPE offers a promising avenue for leveraging AI tools while maintaining high-quality translation outputs. As new technologies continue to emerge, we can expect to see further refinement and adaptation of MTPE workflows, making it a core skill for those seeking to thrive in the evolving world of translation.
7 Essential AI Translation Skills New Graduates Need for Career Success in 2025 - Cross Platform Translation Memory Management
Cross-platform Translation Memory (TM) management is becoming increasingly important in AI-powered translation. As translation increasingly leverages automation, understanding how TM systems work across various platforms is vital for maintaining consistency and efficiency in translation projects. TMs improve translation by allowing previously translated segments to be reused, classifying them based on how similar they are to new text (e.g., "exact match" or "fuzzy match"). This speeds up the process and ensures consistency. Furthermore, knowing how to integrate TM with localization software is crucial to preventing the needless retranslation of content, saving time and money. Gaining experience with these technologies can help new graduates become more productive and competitive in the translation job market by enabling them to efficiently manage complex multilingual projects using AI-driven tools. While the speed and convenience of AI translation are attractive, the potential for errors and inconsistencies underscores the need for skilled human oversight and TM management to achieve high quality results.
In the dynamic field of AI-powered translation, the ability to manage translation memory (TM) across different platforms is becoming increasingly vital. It's no longer enough to just understand how to use a specific translation tool – today's translators need to be comfortable navigating the complexities of interconnected systems.
One aspect that's particularly important for new graduates to consider is how different translation software and platforms can seamlessly interact. Many modern TM systems are designed to integrate with a variety of tools, ensuring a consistent translation experience across projects that may involve various platforms and software. This integration is especially valuable for teams collaborating on large translation projects, helping them streamline workflows and prevent inconsistencies across multiple tools.
Furthermore, advanced TM systems are increasingly incorporating shared memory pools. This means that different translators working on related projects can contribute to and access a unified pool of previously translated segments. This centralized knowledge base enhances consistency across various projects, ensuring terminology remains uniform and contributing to better quality control, especially for large organizations working with a multitude of clients or products.
Also noteworthy is how TM systems leverage historical data. The more a TM system is utilized, the better it becomes at suggesting translations. These systems learn from past translations and adapt to individual translators' styles and preferences, leading to a higher quality of suggestions over time. This adaptive capability, often powered by machine learning algorithms, significantly reduces the time translators spend evaluating options, which ultimately leads to faster turnaround times for translation projects.
Interestingly, TM systems can contribute significantly to cost efficiency in the translation industry. By effectively reusing previously translated segments, companies can dramatically reduce the manual effort needed for translating similar text across multiple documents or projects. For businesses with high volumes of repetitive content, this can lead to considerable savings, with estimates suggesting reductions of up to 75% in some cases. However, it's important to note that relying on historical data comes with the risk of propagating any errors present in previous translations. Thus, regular audits and updates of the TM system are essential to guarantee ongoing high translation quality.
Another area where graduates should develop an awareness is how some TM systems integrate with OCR technology. This integration can be a game-changer when dealing with source materials that exist only in physical form, such as scanned documents or historical texts. OCR enables the automated extraction of text from images, making those materials accessible for translation within the TM framework.
Moreover, cloud-based TM solutions are becoming more common, providing a flexible, collaborative workspace for geographically dispersed translation teams. Working in the cloud allows for efficient collaboration and ensures consistent outputs even with diverse team compositions.
Understanding these various aspects of cross-platform TM management equips new graduates with valuable skills for navigating increasingly complex and collaborative translation projects. It highlights the importance of understanding the interplay between TM, AI, and a variety of other translation technologies to enhance productivity and produce high-quality results in a cost-effective manner. In essence, managing translation memory effectively across different platforms will become a crucial skillset for success in the future of AI translation.
7 Essential AI Translation Skills New Graduates Need for Career Success in 2025 - Working With Translation APIs and Automation Tools
Working With Translation APIs and Automation Tools
In today's business environment, speed and affordability are paramount when it comes to translation. This has led to a growing demand for translators who are skilled in using translation APIs and automation tools. Essentially, these APIs act as bridges between different translation programs and services, allowing for seamless integration within various workflows. By understanding how to utilize these connections, new graduates can build more efficient translation processes, enhance productivity, and manage increasingly intricate projects with greater ease.
Furthermore, automation tools are becoming increasingly essential for managing large volumes of translation projects. They allow for batch processing of translations, ultimately resulting in significant time and cost savings. While these tools offer remarkable efficiency, it's important for aspiring translators to develop a discerning eye, recognizing the potential limitations they might introduce, especially concerning culturally specific aspects and contextual subtleties within the translated text.
The ever-evolving landscape of AI translation necessitates that new graduates develop a nuanced understanding of how to leverage these powerful tools responsibly. It's not merely about harnessing automation for the sake of speed; it's about striking a balance between speed and maintaining the quality and accuracy of translated materials. In the years ahead, those who can master the art of integrating these tools with a human touch—preserving cultural sensitivity and ensuring linguistic accuracy—will be best positioned for career success.
Working with translation APIs and automation tools is opening up new possibilities in the field, but it's important to understand their strengths and weaknesses. One of the most compelling aspects is the ability to significantly cut translation costs. Some services offer rates as low as a penny per word, making large-scale projects far more accessible. This cost-effectiveness is particularly attractive for companies looking to expand their reach globally.
The synergy between OCR and translation is also quite interesting. OCR, a technology that converts images of text into digital formats, can streamline the translation process, especially when dealing with documents that only exist in print form. Instead of manually retyping text, OCR makes it readily available for translation, accelerating workflows considerably.
However, this convenience comes with a trade-off: speed versus quality. Automated translation tools are incredibly fast, churning out translations in seconds, but this speed can sometimes be at the expense of accuracy. When faced with intricate language structures or culturally specific expressions, AI-powered translation can struggle. It's a constant reminder that while AI is helpful, human expertise is still needed to ensure the translation captures the true meaning and intent of the source text.
One area that often gets overlooked is the potential for context loss in AI translation. While the output may be grammatically correct, it might not make sense within the overall context of a larger text or document. AI, in many ways, struggles to understand the deeper connections between words and sentences, which can be crucial to faithfully conveying the original meaning.
Translation Memory (TM) systems offer an efficient approach to reduce redundancy and maintain consistency. They utilize fuzzy matching, which means they can suggest translations even if the input text varies slightly from previous instances. This is very helpful for speeding up the translation process, but it's crucial to carefully monitor the TM, as it can unintentionally propagate errors from past translations if not regularly reviewed.
The ability to translate multiple languages at once is becoming increasingly common with APIs. This is beneficial for companies operating globally, as it allows them to address translation needs for a wide variety of markets simultaneously.
Many APIs also offer the capability to be trained on specific terminology within an industry. This customization enables the translation system to better adapt to specialized language in areas like law or technology. Essentially, with sufficient training data, the API learns to provide more relevant and accurate translations for a particular niche.
Quality control is also improving thanks to the implementation of automated quality checks within certain translation tools. This can detect potential errors and inconsistencies before human post-editing begins. This proactive approach reduces the manual workload for translators by flagging common mistakes early on.
However, challenges remain. Real-time translation tools, although incredibly convenient, can struggle with complex linguistic structures. The emphasis on speed can hinder their ability to fully grasp the nuanced meaning, making human review important in sensitive contexts.
Some of the latest developments show the potential for translation APIs to accept more than just text. Multi-modal input, including audio and video, is gaining traction. This broadens the range of materials that can be translated and opens the door for comprehensive translation workflows across diverse media forms.
It's clear that translation APIs and automated tools are fundamentally changing the translation landscape. While they provide incredible speed and cost-effectiveness, it's critical for anyone working in the field to be aware of the potential limitations and challenges. Striking the right balance between AI and human expertise will be vital for ensuring that translations remain both accurate and culturally sensitive in the future.
7 Essential AI Translation Skills New Graduates Need for Career Success in 2025 - Real Time Speech to Text Translation Implementation
Real-time speech-to-text translation is a significant development in the translation world, reflecting the growing need for quick and easy communication in our globally connected world. These systems combine speech recognition and machine translation to offer instant, fluid conversations across languages. This can make communication smoother in many settings, from business negotiations to casual conversations. While tools like Google Translate support many languages and can translate speech in real-time, they often stumble with the complexity of certain sentences or culturally specific language.
It's vital that new graduates in the translation field learn not just how to use these real-time translation tools but also how to critically evaluate their output. This involves ensuring the translation accurately represents the original meaning and is culturally appropriate. As the field of AI translation continues to change, understanding these technologies will become increasingly essential for success in this field. New graduates will need to adapt to the limitations of these real-time tools and develop their own skills to improve the quality of translations when needed.
Real-time speech-to-text translation is a fascinating area, offering the potential for truly seamless communication across language barriers. These systems can translate spoken words at remarkable speeds, close to 200 words per minute, which makes them potentially useful for urgent situations like live events or emergency responses where time is critical. However, even the most advanced systems still carry error rates in the 5-10% range, particularly when dealing with background noise or different accents. This reinforces the necessity for human oversight, especially for translations that require absolute precision, like in medical or legal contexts.
One significant limitation is the influence of training data on these systems. Models trained mostly on certain dialects or demographic groups can struggle to understand more diverse language variations, which is a significant hurdle in catering to a truly global audience. It's a bit like teaching a language model just one regional accent - it'll understand that well but could have trouble recognizing or translating other speech patterns. Another issue crops up with complex languages. Languages like Turkish or Finnish, with their complex structures and ability to create lots of different word forms from one root, can trip up translation systems. These languages challenge models that heavily rely on pre-defined vocabulary.
There are some very interesting developments happening on the fringe of speech recognition. For example, merging real-time speech translation with augmented reality (AR) could potentially change how we experience the world. We could see translations overlaid directly onto our view of the real world—imagine visiting a foreign country and having the signs and menus instantly translated as you look at them. While exciting, the accuracy and reliability of this kind of technology are crucial areas that need further development.
Although real-time translation is a powerful tool for immediate communication, when it comes to accuracy, machine translations that are then edited by humans often surpass their real-time counterparts. It's often the case that those post-edited translations become clearer and more contextually rich, particularly for technically focused material where precision is essential. On the flip side, developing sophisticated neural machine translation systems requires a lot of computing power. These systems can demand dedicated hardware like GPUs, especially when processing speech in real-time. This can be a significant factor for smaller translation businesses who might not have the resources to manage that kind of infrastructure.
Cultural nuances are a recurring challenge for these systems. They can struggle to understand subtle cultural meanings or informal expressions in language, which can lead to misunderstandings and misinterpretations. Humans are much better at handling these situations, highlighting the continued importance of human translators in situations where communication is high stakes.
It's not just spoken language that's being targeted for real-time translation; OCR-based systems can do the same with written material. This offers opportunities for on-the-fly translations from images or video feeds, which is especially useful in settings like international conferences where visual information needs translation rapidly. A consistent problem for many of these systems is the variability among speakers. Differences in speed, pitch, and accents can significantly impact how well the system performs. Researchers are working to develop algorithms that are more adaptable and can deal with diverse speaker profiles, which is crucial for broader use across languages and populations.
Real-time translation is certainly advancing quickly, but the interplay between speed and accuracy remains a challenge. Furthermore, the importance of human translators in ensuring context, cultural sensitivity, and overall quality in translation projects continues to be highlighted in these advances. As the technology continues to improve and become more adaptable, it will be interesting to see how it impacts communication across languages.
AI-Powered PDF Translation now with improved handling of scanned contents, handwriting, charts, diagrams, tables and drawings. Fast, Cheap, and Accurate! (Get started for free)
More Posts from aitranslations.io: