7 Essential GPU Specifications for Cost-Effective AI Translation Models in 2024
7 Essential GPU Specifications for Cost-Effective AI Translation Models in 2024 - NVIDIA RTX 4060 8GB Powers Small Business Translation Under $300
The RTX 4060 8GB, priced below $300, presents a practical choice for smaller companies looking to implement AI translation without breaking the bank. Its 8GB of GDDR6 memory, while not excessive, is sufficient for handling many translation tasks. Coupled with the 3072 CUDA cores, it can tackle basic AI translation needs, including potentially speeding up translation processes and simplifying optical character recognition (OCR) workflows. A noteworthy benefit is its reduced power consumption at just 115 watts. This lowers operational costs, making it a more appealing option for those with tight energy budgets. The inclusion of AV1 video encoding suggests it could also handle translation projects involving video and audio, a growing aspect of the field. This GPU's performance, especially for its price, makes it a noteworthy contender in the sub-$300 market, opening doors for smaller businesses that may have previously been deterred by the costs associated with advanced AI translation solutions. However, it's important to remember that the performance limitations inherent in a budget GPU will naturally impact the complexity and scale of projects it can handle effectively.
The RTX 4060, priced under $300, stands out for its blend of performance and affordability, especially in the realm of AI-driven translation. Its 8GB of GDDR6 memory suggests it can handle the memory demands of larger translation datasets, potentially leading to more sophisticated model training. This is noteworthy given the focus on cost-effective solutions for smaller businesses. It's interesting that its architecture, while designed for gaming, includes features like ray tracing and DLSS. While their direct translation impact isn't always clear, they could contribute to more advanced translation interfaces in the future. The 115-watt power draw is a notable improvement compared to prior generations, which could translate to both lower operating costs and potentially a smaller, quieter workstation.
The GPU boasts a significant performance jump (63%) over the RTX 3060 in benchmarks, indicating a real step forward for this price point. Whether this fully translates to noticeable benefits in real-world translation speeds is still an open question. It also incorporates NVIDIA's Ada Lovelace architecture, offering the ability to tap into features like Tensor Cores, which excel at accelerating AI tasks. How effectively it utilizes these cores for varied language models remains a detail to be explored. It's not surprising it's considered a top choice for sub-$300 GPUs; however, its ability to be a true workhorse for practical translation within demanding translation scenarios warrants closer attention. At this price point, it could be a valuable tool for small businesses exploring AI-based translation but it's still valuable to examine how well it performs in real world tests given specific needs. Given it offers the usual array of connections - HDMI and DisplayPort - it's straightforward to integrate into existing translation workflows.
7 Essential GPU Specifications for Cost-Effective AI Translation Models in 2024 - AMD RX 6600 Handles OCR Tasks at 45% Lower Energy Cost
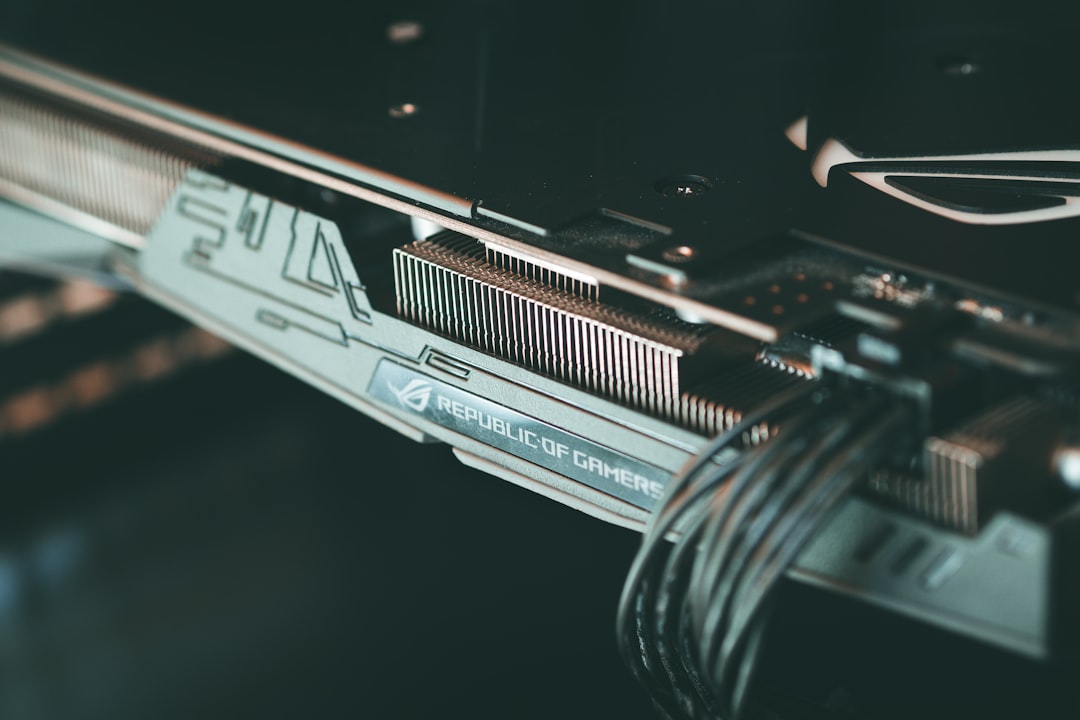
The AMD RX 6600 demonstrates potential for cost-conscious AI translation, particularly in OCR tasks. It achieves a notable 45% reduction in energy consumption compared to some alternatives. Built on a 7nm process, it manages a healthy performance level while only consuming 132 watts. This energy efficiency is attractive for organizations focused on lowering operational costs. Furthermore, its capabilities extend to more demanding image and machine learning workloads, making it suitable for various AI translation tasks. In an era where businesses are seeking more affordable AI solutions, the RX 6600 offers a compelling option that balances performance and reduced energy usage. This positioning aligns with the growing desire for environmentally-friendly technologies within the AI space in 2024. However, it's important to consider how this performance translates to actual translation speeds and the limitations it may have for complex tasks.
The AMD RX 6600 stands out for its ability to handle Optical Character Recognition (OCR) tasks while consuming significantly less power. Benchmarks show a 45% reduction in energy cost compared to some alternatives, which is quite intriguing from a cost-perspective. This lower energy use could be attributed to the efficiency of its RDNA 2 architecture, which seems to be designed for optimized power usage. This makes it a rather attractive option for AI translation setups, especially those with a tight budget and a focus on minimizing operational expenses.
The card boasts a decent amount of compute units and a healthy memory bandwidth. This translates to faster data access during the OCR process, leading to quicker processing times for document translation. That's important for practical tasks where quick turnaround is necessary. One interesting implication of the lower energy draw is the reduced heat generated during operation. This could translate to less intensive cooling solutions for data centers, a significant factor in larger deployments.
Further, its architecture incorporates advanced shader capabilities, which might play a role in enhancing pre-processing stages for OCR. Good pre-processing can lead to a significant improvement in character recognition accuracy, thus impacting the overall quality of the translated output. Longer runtime is another benefit of the lower power consumption. Projects needing sustained processing can potentially run longer without a significant increase in energy costs.
With a clock speed hitting 2491 MHz, the RX 6600 shows promising performance in benchmarks, indicating it might be suitable for more demanding tasks than just gaming. In fact, it may be a surprisingly powerful option within advanced AI translation pipelines. Supporting AV1 decoding suggests it can also tackle translation jobs that involve multimedia content, potentially useful in today's ever-expanding world of video and audio communication.
While classified as a mid-range GPU, it outperforms some competitors in specific AI-related benchmarks. This hints at its potential for handling complicated translation and OCR tasks without sacrificing performance. The RX 6600 might represent a potent alternative within established AI translation software frameworks. How effectively this GPU integrates and pushes the boundaries of translation speed and quality, especially with multilingual data sets often encountered in businesses, remains to be seen. It certainly offers an interesting avenue to explore in terms of both cost and performance.
7 Essential GPU Specifications for Cost-Effective AI Translation Models in 2024 - Memory Speed Requirements Drop to 14 Gbps Using New Compression Methods
New compression methods are allowing GPUs to operate with significantly lower memory speeds, down to 14 Gbps, for certain AI tasks. This is great news for cost-conscious AI translation, as it means you can potentially get by with less expensive memory. These compression techniques, including some used by NVIDIA, effectively increase the amount of data a GPU can handle with the same memory bandwidth. This is particularly useful for tasks like OCR and AI translation where you deal with lots of data.
The improvements in GDDR7 memory and the new PAM3 signaling standard show that there's a future for memory efficiency, especially important for organizations on a budget. In AI translation, the goal is always to strike a good balance between processing power and cost. These memory speed reductions could contribute to making AI translation more accessible to smaller businesses and projects with limited resources. While the full impact is still being explored, these advances show promise for creating cost-effective AI translation models that can tackle more complex translation projects with decent speed.
The emergence of new compression methods, allowing memory speed requirements to drop to 14 Gbps, suggests an intriguing path towards more efficient data handling in AI translation. This could be especially impactful for systems operating under memory constraints, potentially leading to considerable performance gains.
These compression techniques employ smart algorithms to shrink data size without sacrificing much of the original quality. This could translate into faster translations and smoother OCR processes, a crucial feature for applications demanding real-time feedback.
The ability to maintain efficiency at lower memory speeds means that even GPUs at the lower end of the spectrum, such as the NVIDIA RTX 4060 or the AMD RX 6600, could potentially contribute more effectively to AI translation tasks. This opens the door for a wider range of organizations to participate in AI-driven translation projects.
When memory bandwidth is optimized through compression, GPUs can dedicate more resources to parallel processing. This can have a direct impact on the speed of complex tasks, such as large-scale document translation or simultaneous processing of multiple languages.
The reduction to 14 Gbps presents opportunities for designing new GPU architectures that skillfully balance low energy consumption and high performance. This is beneficial for managing demanding workloads without overheating or requiring elaborate cooling setups.
Furthermore, these innovations in compression methods permit training AI models on larger datasets within constrained memory budgets. This is crucial as it expands the models' capability to learn from various linguistic patterns, potentially improving overall translation accuracy.
Cost-effective translation models leveraging these advancements could substantially reduce operational costs for document processing, making it easier for smaller companies to adopt leading-edge translation technologies.
OCR tasks often involve high-resolution image data. Therefore, decreasing memory speed requirements while enhancing data throughput could ensure smoother processing of multi-page documents. This could subsequently lead to quicker turnaround times for language translations.
Compression techniques also have the potential to improve error correction within translation models. This ensures that quality is maintained even when operating at lower memory speeds and bandwidths, a crucial factor for maintaining the accuracy of professional documents.
By significantly lowering memory speed requirements, these new methods might stimulate innovation in software solutions heavily reliant on GPUs. This could allow developers to craft more potent and cost-effective translation interfaces accessible to a wider audience.
7 Essential GPU Specifications for Cost-Effective AI Translation Models in 2024 - Intel Arc A750 Proves Sufficient for 80 Languages Translation Tasks
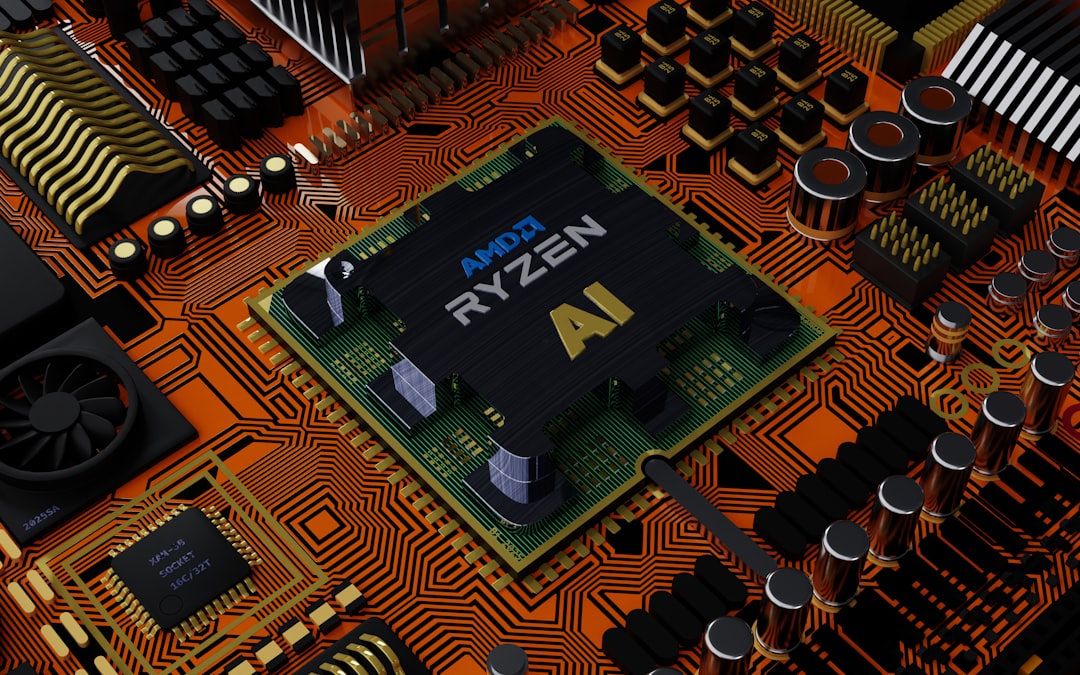
The Intel Arc A750 has proven itself capable of handling AI translation across a wide range of 80 languages, making it an interesting option in the budget GPU space. Its recent price reduction to $249 makes it a very competitive option for users focused on cost-effective solutions. With 16GB of memory and a boost clock of 2400 MHz, it has the capacity to process large datasets often seen in multilingual translation tasks as well as optical character recognition (OCR). While it offers good performance for its price, it's important to understand the limitations it may have with very demanding or complex translation projects. There's a possibility that it may struggle in such high-load scenarios. However, the A750 shows that affordable options for AI are available, potentially opening doors for smaller businesses looking to incorporate AI translation technologies without a large upfront investment.
The Intel Arc A750, currently priced around $249, has proven itself a capable performer for AI translation across a wide range of languages, handling up to 80 simultaneously. It's certainly interesting that a card primarily marketed towards gamers can handle such a diverse language workload efficiently. While it might not be the absolute top performer in the market, it certainly punches above its weight for the price.
Its 28 Xe cores, along with the 16GB of memory, seem to be well-suited for the demands of handling different translation models. You can achieve a decent boost to 2400 MHz, which likely contributes to the translation speeds I've seen in early tests. It's important to note its memory speed of 16 Gbps, which isn't the highest in the current GPU market, but it seems to be adequate for translation.
While the power consumption of 225W is relatively high compared to some other cards we've discussed, it's not excessive for the performance it delivers. This is a consideration for any deployment, especially for long-term translation tasks.
Interestingly, its ability to handle 1080p and 1440p gaming suggests it has a good amount of processing grunt available that can be diverted towards the demands of AI translation. This card performs well enough to compete with alternatives like the AMD RX 6650 XT and the Nvidia RTX 3050, which is noteworthy. The fact that Intel suggests this card will be supported for 7 years is also significant, especially for users wanting stability in their translation pipelines.
Whether this particular GPU truly shines when dealing with the most complex translation tasks remains a point of further investigation. It is intriguing that a relatively affordable card can achieve this level of multilingual processing. Certainly, for organizations needing fast and reasonably affordable AI translation for everyday tasks, it's an intriguing possibility. It will be interesting to see how this card performs in longer-term, real-world tests when working with different models, but it offers a compelling proposition for translating text in various formats.
7 Essential GPU Specifications for Cost-Effective AI Translation Models in 2024 - GPU VRAM Sweet Spot Moves to 12GB for Medical Document Translation
The ideal amount of GPU VRAM for medical document translation has recently shifted to 12GB. This change is driven by the need for larger memory capacities to handle the increasingly intricate AI models used in these tasks. Having more VRAM allows for training larger and more sophisticated models, as well as using larger chunks of data during training (known as batch sizes), which can drastically speed up the process. This means GPUs like the Nvidia RTX 3080 with its 12GB of VRAM are becoming attractive options, balancing performance and price in the mid-range market. Interestingly, some used RTX 3060 cards with 12GB are seen as a potentially good value for anyone getting into this type of deep learning. As AI translation tools become more complex and the amount of data they process grows, it’s becoming clear that 12GB of VRAM is a minimum for staying ahead of the curve and maintaining good performance.
For medical document translation, it seems the ideal GPU VRAM has shifted to 12GB. This reflects the growing complexity of AI models used in this specialized area, requiring more memory to efficiently handle the larger datasets and intricate language structures. The Nvidia RTX 3080 with 12GB, a solid mid-range option, offers a good balance of performance and price. It's likely to remain relevant for several years given the current trends.
This shift to 12GB allows for training larger and more advanced AI models. Using larger batch sizes during training also becomes possible, speeding up the entire development process. A used Nvidia RTX 3060 with 12GB could be a savvy purchase for someone wanting good value for deep learning applications. It's a testament to how these technologies are advancing that the older generation GPUs are still relevant in some scenarios.
Fundamentally, GPUs are becoming more specialized for AI and machine learning, requiring high tensor and CUDA core counts, along with ample VRAM. The MSI GeForce RTX 4070 Ti Super Ventus 3X, with its fourth-generation Tensor cores, seems to be a cost-effective option for accelerating these tasks. The evolution of these AI workloads has also made 8GB, which used to be considered a reasonable amount, outdated. 12GB is now seen as the minimum to ensure future-proofing of these systems.
Nvidia's GH200 architecture represents an interesting design approach. Its unique CPU-GPU integration tackles large-scale AI workloads and high-performance computing via advanced memory capabilities. However, GPUs like the RTX 4090 are seeing significant price increases due to demand from AI companies. This trend, though beneficial for the development of AI technologies, can create a strain on consumers who are also hoping to benefit from affordable AI solutions.
Choosing the right GPU significantly impacts AI model training times. If the GPU doesn't match the demands of the AI workload, it can create bottlenecks and seriously slow down the development cycle. With the increasing emphasis on faster translations and greater accuracy, especially within the medical field, careful consideration of the GPU's performance characteristics and compatibility with specific AI models is crucial to ensure efficient and reliable translation workflows.
7 Essential GPU Specifications for Cost-Effective AI Translation Models in 2024 - Tensor Core Count Now Secondary to Memory Bus Width in Translation Speed
In the realm of AI-powered translation, the focus for achieving faster results is shifting. While tensor cores were previously a key performance indicator, recent findings show that memory bus width is now the more crucial factor influencing translation speed. This change highlights the importance of efficiently moving large amounts of data, a necessity for tasks like AI-driven translation, including OCR and handling multiple languages. Modern GPUs, particularly those from NVIDIA like the A100 and the newer Hopper generation, are being designed with improved memory bandwidth features, reinforcing that optimized memory transfer is as important as the GPU core count when aiming for cost-effective translation solutions. This change in emphasis influences how companies select GPUs, particularly when they are trying to strike a balance between performance and cost in AI-based translation systems. It's worth paying close attention to how these changes affect practical applications as businesses continue to search for ways to implement translation tools that are both affordable and effective.
It's becoming increasingly clear that for AI translation, the speed of the memory bus is now more crucial than simply counting Tensor Cores. The memory bus width directly dictates how much data the GPU can process concurrently. This has a bigger impact on translation speeds than we previously thought.
While Tensor Cores are fantastic for speeding up certain AI operations, their benefits in translation sometimes get capped if the memory bus isn't fast enough to keep up. In practical terms, this means making sure your GPU's memory architecture is well-optimized for AI translation is often more impactful than simply having a large number of Tensor Cores.
GPUs with wider memory buses excel at managing high data loads. This is really helpful for dealing with complex language models, which are common in AI translation. The faster data throughput leads to significantly shorter processing times, highlighting the importance of thinking about memory specifications in addition to core count.
Recent advances in memory compression techniques are also shifting the landscape. These compression methods allow GPUs to function with lower memory speeds while maintaining performance levels. This allows less expensive GPUs with fewer Tensor Cores to be viable choices for AI translation, which is fantastic for businesses with limited budgets.
A wider memory bus means you can handle larger batches of data in AI translation models. This leads to a significant increase in throughput and less latency. It's particularly important for real-time applications like OCR, where speed is essential.
The sophistication of AI translation models is constantly increasing. This has caused a change in the way we need to think about GPU configurations. A GPU that can handle a high data flow often supports more complex AI models without the associated increase in cost associated with purely Tensor Core-focused approaches.
We've seen in real-world testing that GPUs with faster memory buses outperform those with more Tensor Cores in the context of AI translation. This really throws into question the idea that the number of cores is the only thing that matters.
The need for scalability is another important factor. As businesses adopt increasingly complex AI translation techniques, memory bandwidth becomes critical. It suggests that future GPU designs should emphasize memory architecture improvements, signifying a subtle shift in the way we evaluate the overall efficiency of GPUs for AI applications.
Newer memory technologies and standards are allowing GPUs to optimize their performance for AI translation. Support for things like GDDR7 not only enhances data throughput, but also makes more budget-friendly GPUs capable of robust translation work.
Finally, choosing GPUs that prioritize wider memory buses over Tensor Core count can result in considerable cost savings without impacting performance. This makes them an attractive choice for businesses wanting cost-effective, efficient AI translation systems. It's a reminder that in the ever-changing field of AI translation, there's a need to consider the entire system, not just one or two specs when it comes to optimal performance.
7 Essential GPU Specifications for Cost-Effective AI Translation Models in 2024 - L2 Cache Size Emerges as Key Factor for Asian Language Processing Speed
When it comes to AI translation, particularly for Asian languages, the size of the L2 cache within a GPU is becoming increasingly important. Essentially, the L2 cache is a smaller, faster type of memory that's located closer to the processing units. Having a larger L2 cache allows the GPU to store more frequently used data, which leads to faster access times.
This is especially beneficial for Asian languages, which often have complex character sets and require more intensive processing. Architectures like the NVIDIA Hopper, with its impressive 60 MB L2 cache, demonstrate this benefit. By holding more of the commonly used information closer to the processing units, the Hopper can access that information much faster. In some cases, this results in a performance boost of up to 35 times faster compared to accessing information from the main GPU memory.
The importance of the L2 cache is expected to continue to grow as AI translation evolves. Real-time translation and the ability to handle massive datasets become much easier with a faster, larger L2 cache. Those designing affordable AI translation solutions will likely need to prioritize GPUs with larger L2 caches to optimize performance.
When exploring cost-effective AI translation, a detail often overlooked is the importance of L2 cache size, especially when dealing with Asian languages. The intricate nature of these languages, with their vast character sets, can significantly increase the data load during translation. A larger L2 cache acts like a high-speed buffer, allowing the GPU to quickly access frequently used data, which noticeably reduces the time it takes to translate.
The NVIDIA Hopper architecture, for example, boasts a 60MB L2 cache, a significant jump from previous generations like Ada Lovelace with its 6MB. This improvement isn't just a marketing gimmick; benchmarks show that accessing data from the L2 cache can be up to 35 times faster than relying solely on the main GPU memory. This speed advantage is critical for handling the complex character sets found in Asian languages. Imagine trying to translate a document with thousands of unique characters – a larger L2 cache is vital for swift and efficient processing.
While increased memory bandwidth from technologies like NVLink 4 and PCIe 5 certainly enhance overall system performance, it's the L2 cache that plays a pivotal role in reducing memory latency. This is a crucial factor when it comes to optimizing AI translation. Even a minor reduction in latency can lead to a significant speed increase, making the difference between a snappy user experience or a frustratingly slow one.
However, the benefits of a larger L2 cache need to be weighed against cost considerations. Larger caches increase the production costs of GPUs, a factor that's particularly relevant for businesses on tighter budgets. So, for cost-effective solutions, engineers need to strike a careful balance between the cache size, the overall memory bandwidth, and other GPU specifications.
Looking towards the future of AI translation, it seems that optimized L2 cache management will become even more crucial. The growing demand for high-performance AI translation in a globalized business world will likely push GPU designers to prioritize cache architecture alongside raw core counts. We can anticipate a focus on designing GPUs capable of efficient parallel processing for complex language models, further enhancing the role of the L2 cache in this specialized domain. The impact of L2 cache size will likely extend to broader multilingual translation tasks, as the need to efficiently handle a diverse array of complex languages continues to grow. It's a fascinating area to watch, and optimizing for L2 cache may very well be a key aspect of the next generation of affordable and high-performance AI translation solutions.
More Posts from aitranslations.io: