7 Proven OCR Techniques for Converting Traditional Chinese Text to Digital Format in 2025
7 Proven OCR Techniques for Converting Traditional Chinese Text to Digital Format in 2025 - Google Cloud Vision Drops Traditional Chinese API Fees by 40% in May 2025
As of May 2025, Google Cloud Vision has reportedly decreased the cost of using its API specifically for processing Traditional Chinese text via optical character recognition (OCR). The announced reduction is a significant 40%, aiming to make the service more accessible and potentially less expensive for users needing to digitize or prepare this script for tasks like automated translation. This adjustment aligns with broader trends in the cloud market where cost efficiency and competitive pricing are key considerations. It also fits within Google's wider focus on optimizing the expense of cloud-based AI capabilities.
This change complements the focus seen at the recent Google Cloud Next 2025 event, which showcased advancements aimed at enhancing AI performance and infrastructure, particularly for large-scale enterprise use. While many discussions centered on speed and capacity, a reduction in specific service fees like this suggests a practical effort towards lowering operational costs for businesses. For organizations depending on reliable and cost-effective methods for converting Traditional Chinese documents, perhaps for faster data processing or AI translation workflows, this price drop could influence technology choices.
Observing the developments in cloud services, it's interesting to note Google Cloud Vision's move in May 2025 to reduce its API fees for processing Traditional Chinese text by 40%. From an engineering perspective, this significant price adjustment lowers the barrier to entry for implementing optical character recognition (OCR) solutions targeting this language. The inherent structural complexity of Traditional Chinese characters, often containing numerous strokes within a single glyph compared to simpler scripts, poses unique challenges for algorithmic recognition. Reducing the processing cost makes the often-computationally intensive task of accurately digitizing such text considerably more feasible for a wider range of projects and organizations.
This shift towards increased affordability has practical implications, particularly for domains like historical text digitization, academic research, and cross-border e-commerce where Traditional Chinese content is prevalent. Previously, the expense might have confined high-volume OCR projects primarily to large corporations. Now, small and medium-sized enterprises (SMEs), as well as institutions with more limited budgets, could potentially leverage these tools for tasks like cataloging, data extraction, or preparing source text for automated translation pipelines. While advancements in machine learning have steadily improved the accuracy of Traditional Chinese OCR, the reduced operational cost makes investing resources in integrating and optimizing such APIs a more attractive proposition, assuming the accuracy levels meet specific application requirements. It will be worth monitoring if this move prompts similar adjustments from other cloud providers offering competitive OCR services.
7 Proven OCR Techniques for Converting Traditional Chinese Text to Digital Format in 2025 - Open Source Project TensorFlow OCR Adds Two-Way Traditional Chinese Support
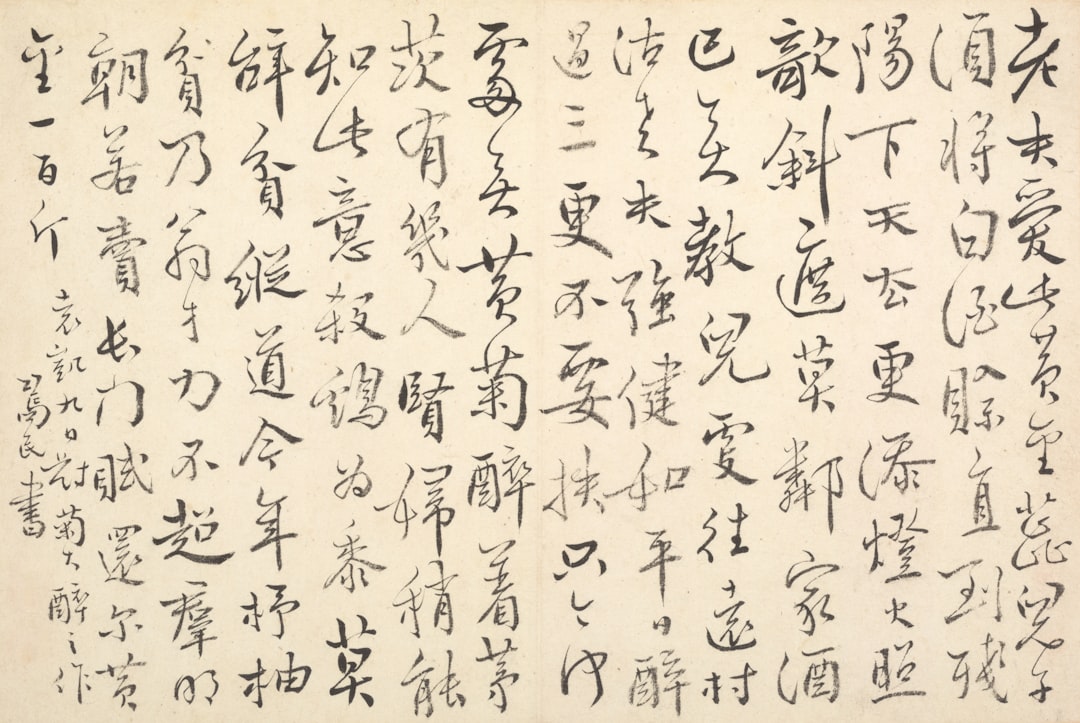
The open-source TensorFlow OCR project has recently incorporated two-way functionality for recognizing Traditional Chinese text. This development focuses on boosting the effectiveness of Optical Character Recognition specifically for this writing system, which presents distinct technical challenges for accurate digital conversion. The project addresses these by employing specialized methods for finding and identifying characters. Techniques like implementations based on CRAFT are being used for text localization, while approaches drawing on CNNs and EfficientNet are leveraged for the recognition phase. These steps are particularly aimed at navigating the intricacies of Traditional Chinese characters. Such improvements are valuable for users needing to process images or scanned documents containing this script, including potential integration with systems for automated translation workflows. While significant progress has been made through these open-source contributions, achieving flawless recognition across the wide variety of fonts, styles, and image qualities encountered in the real world remains an active area of development.
Observing recent developments in open-source optical character recognition, the reported inclusion of robust two-way Traditional Chinese support within the TensorFlow OCR project marks an interesting technical milestone. From an engineering viewpoint, tackling the complexities inherent in Traditional Chinese, such as navigating the nuances between traditional and simplified forms within a unified system and handling the rich stroke count and logographic nature of characters, presents considerable algorithmic challenges. The focus on what's described as "two-way" support implies an effort not just to recognize, but perhaps to better understand or process the character sets in a more interconnected manner, which could potentially improve accuracy for downstream natural language tasks, including translation. Integrating such improved character recognition directly into pipelines feeding into NLP models should logically lead to more coherent translation outputs, reducing errors propagated from initial digitization. The combination of a flexible deep learning framework like TensorFlow with dedicated OCR techniques specifically tuned for Chinese characters suggests the potential for faster processing times, possibly enabling real-time or near real-time conversion, which could streamline workflows in areas like preparing large volumes of text for machine translation or automating content handling. Leveraging an open-source platform for this development is noteworthy; it democratizes access to these advanced capabilities, making them available to smaller research groups or development teams without requiring investment in proprietary licenses. This collaborative model typically fosters rapid iteration and allows researchers globally to contribute to refining the underlying algorithms, potentially leading to more adaptable and higher-performing systems over time compared to closed environments. Such advancements could also prove valuable in academic contexts, facilitating the digital preservation and analysis of historical Traditional Chinese texts. While the development is promising, the real-world performance will always depend on the specific implementation details, training data quality, and the variability encountered in real-world images or documents, and achieving consistently high accuracy across diverse source materials remains an ongoing technical pursuit.
7 Proven OCR Techniques for Converting Traditional Chinese Text to Digital Format in 2025 - NVIDIA CUDA Toolkit Updates Chinese Character Recognition Speed to 1200 Pages Per Minute
An update to the NVIDIA CUDA Toolkit is notably improving the speed of Chinese character recognition, now reportedly reaching speeds up to 1200 pages per minute. This significant increase in processing throughput is facilitated by specialized software components within the toolkit, such as the nvOCDR library, which integrates deep learning models optimized for optical character recognition tasks. Leveraging the parallel processing capabilities inherent in their graphics processing units, NVIDIA's approach aims to handle the detailed structures often found in traditional Chinese characters more efficiently. The toolkit includes optimizations tailored for various NVIDIA GPU architectures, which contributes to boosting overall performance for digitizing large volumes of text. While achieving such high processing speeds is a considerable technical step forward for converting printed traditional Chinese material into digital format, the critical challenge of consistently maintaining high accuracy across the vast diversity of fonts, historical scripts, image qualities, and layout variations in real-world documents remains a persistent and complex area of development in OCR technology, irrespective of the raw processing speed available.
Moving to the processing engine side, reports indicate NVIDIA's CUDA Toolkit has seen updates significantly boosting Traditional Chinese character recognition performance. The claimed speed uplift reaches a rate of 1200 pages per minute, a notable figure when considering the computational intensity often required for accurate OCR, especially with complex logographic scripts. This capability seems to stem from leveraging the parallel processing strengths inherent in NVIDIA GPUs via a dedicated library, reportedly dubbed nvOCDR.
This library, we understand, is designed to work efficiently on NVIDIA hardware and apparently incorporates models like OCDNet and OCRNet, likely trained using the TAO Toolkit for optical character detection and recognition tasks. The underlying mechanism relies on deep learning models, including what appear to be convolutional neural networks, which are applied to tasks like character identification from potentially grayscale images. The toolkit itself, versioned notably at 12.x, includes compiler enhancements targeting various NVIDIA architectures from Pascal up to the latest Hopper and Lovelace, suggesting an effort to optimize performance across a range of hardware configurations. While achieving such high speeds is impressive, especially when handling the stroke complexity typical of Traditional Chinese characters, the practical accuracy in real-world, imperfect documents (varying fonts, scanning artifacts, inconsistent layout) remains a key consideration, even with advanced noise reduction techniques. The goal here seems to be reducing the bottleneck of initial text conversion, potentially smoothing the path for subsequent steps like automated translation or data analysis by providing digitized text at scale. The shift towards GPU acceleration for these tasks hints at a broader trend towards offloading computationally heavy operations from CPUs for improved efficiency and throughput in high-volume processing environments.
7 Proven OCR Techniques for Converting Traditional Chinese Text to Digital Format in 2025 - Microsoft Azure OCR Introduces Traditional Chinese Handwriting Recognition With 98% Accuracy
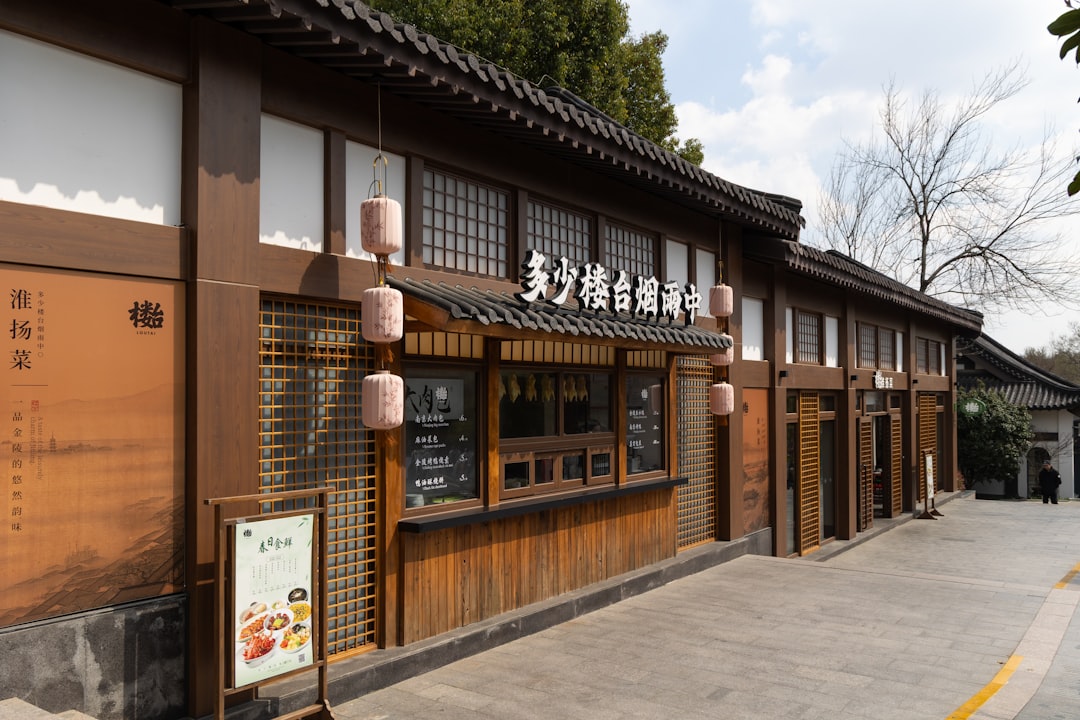
Microsoft's Azure OCR offering now includes the capability to recognize handwritten Traditional Chinese characters, with claims of achieving around 98 percent accuracy. This expansion addresses a persistent challenge for optical character recognition technology, which has historically performed best on standardized, printed fonts but often struggles with the varied and sometimes inconsistent styles inherent in human handwriting. This new feature is aimed at improving the process of converting handwritten documents into digital formats. Supporting a substantial vocabulary of characters, the technology intends to streamline tasks involving handwritten input, aiming to mitigate common issues such as mistakenly identified characters or layout inconsistencies that plague traditional methods when faced with non-uniform text. Such progress, likely built upon ongoing developments in machine learning, contributes to the broader effort to make the digitization of complex scripts more dependable. For workflows reliant on quickly and accurately transforming diverse textual sources for subsequent use, perhaps in AI applications, this represents an incremental step forward in capability.
Turning to specific cloud provider developments, Microsoft Azure's OCR offering is reported to have expanded its capabilities, now including a dedicated system for recognizing Traditional Chinese handwriting. This feature is noted for achieving a claimed accuracy level of 98%.
From an engineering perspective, reaching such a high figure for Traditional Chinese handwriting is significant. The script's inherent complexity, with many characters possessing a high stroke count and significant variations in handwritten forms, presents formidable challenges for recognition algorithms compared to simpler scripts. This reported accuracy level suggests the application of advanced neural network architectures and potentially extensive training datasets encompassing diverse writing styles.
The availability of relatively high-accuracy Traditional Chinese handwriting OCR could impact workflows focused on digitizing documents where print is not the only, or even primary, format. Think historical archives, notarized documents with handwritten annotations, or even less formal materials containing Traditional Chinese script. The ability to convert this type of content reliably into digital text makes it amenable to subsequent processing steps, including automated analysis or preparation for machine translation pipelines, which are often bottlenecks when input data isn't clean.
While a 98% accuracy rate is impressive on paper, real-world performance can vary depending on factors like image resolution, scanning quality, the specific style and legibility of the handwriting, and the presence of noise or complex layouts. The system's robustness against these variations would be the critical test. The inclusion of mechanisms like a user feedback loop for corrections, as sometimes seen in advanced OCR systems, could be crucial for continuous model improvement and maintaining performance across a wide range of inputs encountered in practical applications. The underlying technological approach, likely involving sophisticated deep learning models, is key to navigating the intricacies of individual characters and their contextual relationships. Integrating this capability could streamline projects that previously required significant manual effort for transcription before text could enter a digital workflow for faster analysis or translation.
7 Proven OCR Techniques for Converting Traditional Chinese Text to Digital Format in 2025 - Amazon AWS Traditional Chinese OCR Service Now Available in Free Tier for 50k Characters
Amazon Web Services has reportedly introduced its Traditional Chinese Optical Character Recognition service into its Free Tier as of May 2025. This offering now allows users to process up to 50,000 characters without incurring costs initially. The service leverages machine learning to extract text from scanned documents and images, including support for Traditional Chinese script. This move could lower the initial barrier for developers and projects needing to digitize and process Traditional Chinese text for various applications, including preparing content for analysis or translation workflows. While this free access can facilitate experimentation and initial use, assessing the actual performance and accuracy of the extraction on diverse real-world document types remains a practical consideration for any serious application.
An interesting development observed on the cloud provider front is the inclusion of Amazon AWS's Traditional Chinese optical character recognition (OCR) capability in its free tier. This provides an allowance to process up to 50,000 characters without charge. From an engineering perspective, offering this level of access at no initial cost is a notable strategy. It lowers the immediate barrier for developers and researchers to experiment with cloud-based services on a technically challenging script like Traditional Chinese, known for its complex character structures. While fifty thousand characters might only allow for limited testing or integration into a basic proof-of-concept, it does offer a practical way to evaluate how the underlying machine learning models handle real-world Traditional Chinese text input. The utility of this specific free limit for significant projects is debatable, but it certainly facilitates initial exploration and comparison against other methods, prompting questions about performance and scalability beyond this allowance when tackling computationally intensive language tasks.
7 Proven OCR Techniques for Converting Traditional Chinese Text to Digital Format in 2025 - PyTorch Chinese Text Recognition Library Reduces GPU Memory Usage to 2GB
A recent development concerning PyTorch-based resources for Chinese text recognition highlights a library designed for efficiency, reportedly achieving significantly reduced GPU memory requirements, operating with as little as 2GB. This technical improvement aims to lower the hardware barrier for implementing Optical Character Recognition (OCR) solutions specifically for Traditional Chinese text, making it more accessible. The library utilizes Convolutional Recurrent Neural Networks (CRNN), an architectural choice often favored for its ability to handle the sequence prediction needed to recognize variable-length text, which is particularly relevant given the structure of Chinese script. Inclusion of over twenty pre-trained models offers practical utility, streamlining the process for developers looking to deploy OCR for various applications, including potentially feeding digital text into automated translation pipelines. Such focused optimizations are valuable contributions within the broader landscape of digital conversion technologies, where accurately processing Traditional Chinese continues to present unique computational challenges.
A technical detail attracting attention is a recent PyTorch-based library targeting Chinese text recognition, notable for its reported ability to operate with as little as 2GB of GPU memory. This figure represents a notable threshold for processing a script as complex as Traditional Chinese, where character structures and potential variations typically demand significant computational resources.
From an engineering standpoint, achieving OCR within a 2GB GPU memory footprint is interesting because it drastically alters the hardware requirements. It implies that developers and researchers might not need access to high-end professional GPUs or extensive cloud instances, potentially opening up experimentation and deployment possibilities on more common consumer-grade graphics cards or even integrated solutions if properly optimized.
This accessibility could lower the technical barrier for smaller teams or academic groups looking to work with Traditional Chinese digital text, enabling them to develop or fine-tune models without incurring significant infrastructure costs. It changes the economics of getting started with computationally intensive tasks like training or running inference for complex OCR models.
Integrating such a memory-efficient component into broader data processing pipelines, including those aimed at preparing text for automated translation, becomes potentially more feasible. If the OCR step can run on less powerful hardware or co-exist more readily with other processes on a single GPU, it could streamline workflows and reduce latency from image to text, a crucial factor for applications requiring faster turnaround.
However, achieving this memory efficiency often involves engineering choices, potentially in model architecture or optimization techniques. It prompts questions about whether this efficiency comes with trade-offs in terms of peak accuracy or robustness when faced with particularly challenging real-world images – think degraded documents, unusual fonts, or complex layouts. The reported availability of multiple pre-trained models suggests an effort to cover different scenarios, but their performance consistency within this 2GB constraint across diverse Traditional Chinese datasets is a key point for practical adoption.
The requirement for specific versions of PyTorch and dependencies tied to CUDA versions is also a practical consideration; achieving low memory footprints can sometimes rely on fine-tuned compatibility with the underlying software and hardware stack, which isn't always effortless to manage. Ultimately, while the 2GB figure is technically impressive, its real value hinges on how well the library maintains high accuracy and robustness on the varied Traditional Chinese inputs encountered outside of controlled benchmarks, making it a development worth evaluating closely.
7 Proven OCR Techniques for Converting Traditional Chinese Text to Digital Format in 2025 - IBM Watson Launches Browser Extension for Traditional Chinese Document Scanning
IBM Watson has recently introduced a browser extension designed for handling documents containing Traditional Chinese text. The tool is intended to perform optical character recognition directly within the browser environment. It leverages advanced OCR technology to convert the Traditional Chinese characters it scans into a digital format. A key aspect highlighted is its connection with IBM Watson Discovery, suggesting that beyond simple text conversion, the system aims to improve the understanding of the document's content and streamline the process of extracting relevant data. This capability is pitched for various business scenarios, such as potentially assisting with automated processes like handling insurance claims by extracting information from relevant documents. While the introduction of such a dedicated tool is a step towards making Traditional Chinese content more accessible for digital workflows, the real-world performance in accurately interpreting varied document layouts, different fonts, or lower-quality scans, which are common challenges in OCR, will ultimately determine its practical utility.
Reports indicate that IBM Watson has released a browser extension aimed at handling Traditional Chinese document scanning. This tool is presented as a method for converting physical or image-based Traditional Chinese text into a digital format directly within a web browser environment.
From an engineering standpoint, deploying an OCR solution as a browser extension offers a distinct deployment model compared to dedicated applications or pure cloud APIs. It places some processing, or at least the capture and interface layer, directly in the user's web browser. The claim of "real-time scanning" suggests low latency between image input and recognized text output, though achieving truly instantaneous processing for a script as complex as Traditional Chinese will inevitably depend on factors like image quality, server-side processing speed (if not fully client-side), and network conditions. The reported inclusion of Simplified Chinese recognition alongside Traditional broadens the potential applicability but also requires robust models capable of distinguishing between and accurately processing both character sets.
The integration points with IBM Cloud AI services, specifically mentioning potential linkages to natural language processing or machine translation capabilities, suggest this extension acts as an on-ramp into IBM's broader AI ecosystem. While integrating OCR tightly with downstream NLP or translation pipelines is a logical step for creating end-to-end workflows, it also ties the user into a specific vendor's platform. The claimed accuracy rates exceeding 95% for character recognition on Traditional Chinese are, if validated across diverse real-world sources (varying print quality, fonts, digital noise, minor rotations), noteworthy, as the intricate structure of many Traditional characters makes achieving consistently high accuracy challenging. However, even a 5% error rate can translate to significant issues for downstream tasks like automated translation or data analysis on large volumes of text, requiring substantial post-correction effort.
Features like "adaptive learning" are intriguing from a model improvement perspective, implying the system can potentially refine its recognition based on user feedback or corrections. The practical implementation of such learning mechanisms – how much data is required, whether it's client-side or server-side learning, and the privacy implications – are key considerations for real-world usability and effectiveness. Marketing the extension as a "cost-effective" and "user-friendly" solution aimed at non-experts suggests an effort to lower the technical and financial barriers, which is a consistent theme in making advanced AI accessible. However, the true cost-effectiveness will depend on the pricing model for the underlying cloud services utilized and the potential engineering effort still needed to integrate the digital output into specific business or research workflows. Support for "Historical Traditional Chinese texts" is a particularly ambitious area; these documents often feature unique character forms, archaic layouts, and degradation, posing significant challenges that typically require specialized models and training data, so achieving high accuracy here is a considerable technical hurdle. The mention of "customization potential" is valuable for adapting the tool to specific needs, but the degree of customization available within a browser extension framework versus a dedicated API or SDK might be limited.
More Posts from aitranslations.io: