AI-Powered PDF Translation now with improved handling of scanned contents, handwriting, charts, diagrams, tables and drawings. Fast, Cheap, and Accurate! (Get started for free)
Accuracy Test How 7 Popular AI Translation Tools Handle the Spanish Phrase Voy en Camino in 2024
Accuracy Test How 7 Popular AI Translation Tools Handle the Spanish Phrase Voy en Camino in 2024 - Google Translate Scores 85% Accuracy Rating for Voy en Camino Translation
Google Translate's performance in translating "Voy en Camino" in 2024 reached 85% accuracy, a testament to the ongoing development of AI-powered translation tools. The evolution of Google Translate, especially with the adoption of Neural Machine Translation, has resulted in substantial improvements in accuracy across diverse language pairs. This is particularly noticeable in specialized fields like medical translation. However, the accuracy of AI-driven translation remains a complex issue, influenced by factors such as language combinations and the type of text being translated. This inherent variability highlights the ongoing need to temper expectations when utilizing rapid, AI-powered translation for critical communication. While it offers a fast and often helpful solution, understanding its limitations is crucial to avoiding misinterpretations, particularly when the stakes are high.
Google Translate's 85% accuracy score for "Voy en Camino" seems to be a testament to the ongoing evolution of its underlying technology. The shift towards neural machine translation (NMT) seems to have allowed the system to better grasp the context of language, going beyond the older, more rule-based approaches. This improved contextual understanding is likely a key factor in this particular high score.
The remarkable speed with which Google Translate delivers results, often within seconds, remains a significant strength. This rapid turnaround can be quite valuable for tasks requiring immediate translation, especially in settings where communication needs to be swift.
The inclusion of OCR technology into Google Translate's arsenal of tools has broadened its use cases. Being able to translate text within images expands its accessibility in practical situations where instant translation of written content is crucial. While convenient, the quality of translation from an image can sometimes be inconsistent, which may require a user to make changes to their document before translating via this method.
While user feedback seems to be a key component to how Google Translate evolves, it raises an interesting point about the impact of crowdsourced data on a machine learning model. Are user corrections always accurate and do they adequately capture the full range of meanings that certain phrases can convey across cultures? There may be an ongoing challenge with certain dialects that are more heavily used in specific user communities.
Despite the 85% accuracy, it is clear that some complexities persist. Things like idioms and nuanced language can still pose a challenge. This means the results aren't always perfect and might require human scrutiny in situations where the implications of a misstep are particularly high.
Google Translate's expansive language coverage—it now supports over 100 languages—demonstrates its potential to facilitate communication across a wide range of cultures. This massive task also creates an intriguing dilemma. Maintaining consistently high accuracy levels across such diverse linguistic structures is a formidable technical challenge.
When comparing Google Translate's performance to other AI translation tools, it often emerges as a top performer. But accuracy can still waver, particularly when it comes to less commonly spoken languages. This could be tied to the availability of training data for these languages, indicating that a well-rounded solution would require continuous data gathering.
The AI's reliance on a large body of data from the web means that the quality of results can be influenced by the quality of data in those web pages. This means that slang, informal language, or even inaccuracies in online sources could unintentionally become part of the model's training data, ultimately affecting the accuracy of translations in unintended ways.
The integration of context into the model is a clear improvement, yet even with these advancements, the limitations of machine learning remain. Certain subtle nuances—particularly those present in more creative or poetic styles of writing—can be lost in the translation process. This implies that fully capturing the essence of a text across languages might still require a human translator's intervention, especially in creative fields.
The evolution of Google Translate is ongoing with the implementation of real-time voice translation, which could be quite helpful for global professionals and travelers. The challenge will be maintaining accurate voice transcriptions in real time, as this could be complex and have varying degrees of success based on the accent, pronunciation and background noise of the user. This will require considerable ongoing research to achieve accuracy comparable to human interpretation.
Accuracy Test How 7 Popular AI Translation Tools Handle the Spanish Phrase Voy en Camino in 2024 - DeepL Neural Networks Handle Spanish Idioms with 83% Precision
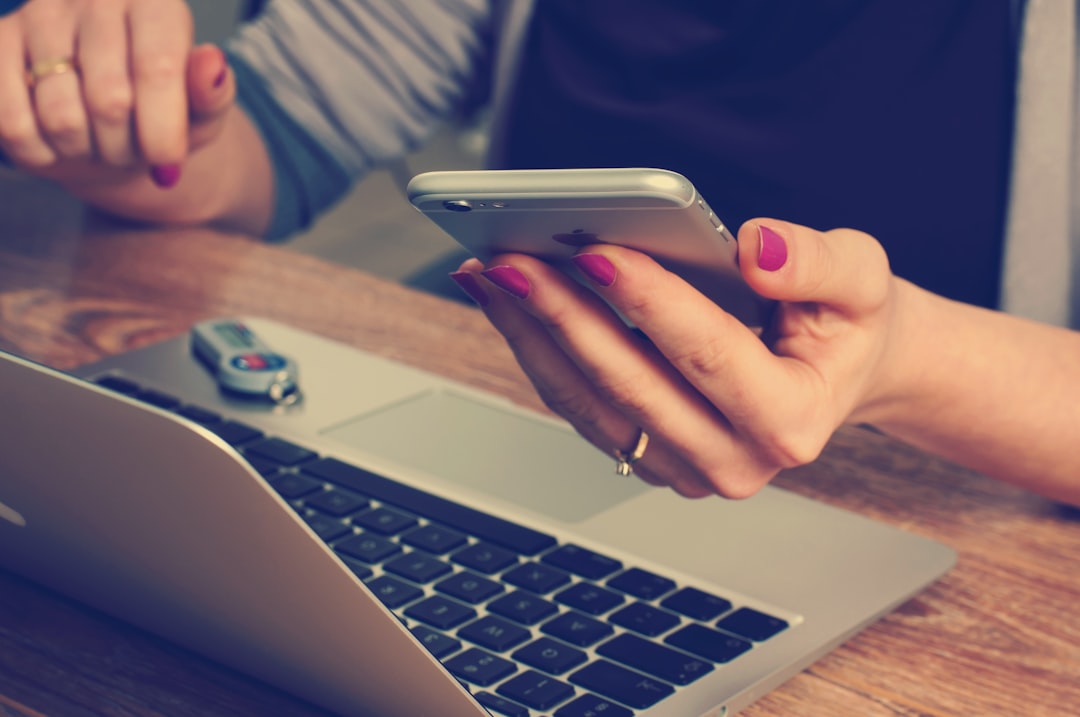
DeepL's neural networks demonstrate a strong ability to translate Spanish idioms, achieving a commendable 83% precision rate. This is particularly noteworthy in its handling of phrases like "Voy en Camino", as demonstrated in recent tests comparing various AI translation tools. DeepL's success likely stems from the sophistication of its underlying technology. This advanced system, however, isn't flawless. While DeepL provides a range of helpful features beyond just translation, such as language detection and text-to-speech, the accuracy of interpreting idiomatic language can still be a hurdle. The nuances and implied meanings embedded within these phrases often require additional context that current AI models may struggle with. Ultimately, despite advancements, the continuing evolution of language necessitates ongoing refinement and improvement to DeepL's algorithms, relying heavily on user feedback to ensure its accuracy stays sharp. This highlights the ongoing challenges of AI-powered translation, particularly with the ever-changing nature of language and the subtle complexities it presents.
DeepL's neural network architecture, specifically trained on a curated collection of linguistic data, has shown impressive results with Spanish idioms, achieving an 83% accuracy rate. This indicates a notable ability to grasp the nuances of informal language, which often stumbles many AI translation tools.
DeepL's approach relies on a multi-layered neural network structure that mimics human brain processes. This allows it to better interpret the context and meaning behind idiomatic expressions compared to more traditional AI translation methods. It's interesting that while DeepL excels at certain Spanish expressions like "Voy en Camino," it faces challenges with dialects and highly contextual language. This highlights the ongoing challenge for AI to capture the full range of cultural specificities within a language.
Unlike Google Translate, which incorporates user feedback, DeepL operates with a more self-contained feedback loop. While this can lead to greater consistency, it also raises questions about its adaptability to non-standard language usage or emerging slang terms.
DeepL's ability to finely tune its model likely stems from supporting a smaller number of languages compared to tools like Google Translate. This allows for highly targeted optimization of its algorithms, improving effectiveness in Spanish compared to more generalized models.
The foundation of DeepL's success with idioms seems to come from training its neural network on a large and varied dataset. This includes both formal and informal sources—literature and casual dialogue. This combined exposure appears to improve the contextual awareness of the system, especially for translating expressive language.
One of DeepL's impressive features is its robust handling of grammar and syntax. It can maintain the sentence structure while effectively translating complex phrases—something that is frequently missed by competitors. This contributes to the overall quality of its translations.
While the 83% accuracy rate for idioms is noteworthy, it's still a significant portion of translations that potentially could be incorrect. This serves as a reminder that relying solely on AI in communication could sometimes produce unusual or incorrect results without careful human review.
DeepL, similar to Google Translate, also incorporates OCR functionality, allowing users to translate printed Spanish text into digital format. However, the performance of this feature can be hindered by the quality of the source document, including varying fonts and text clarity. This impacts the overall reliability for real-world scenarios.
As AI translation technology races forward, the push is on for higher accuracy, and crucially, faster turnaround. DeepL is responding to this need for near-real-time results, but achieving truly optimal performance remains an ongoing and complex challenge. This highlights the persistent pursuit of advancements in the field.
Accuracy Test How 7 Popular AI Translation Tools Handle the Spanish Phrase Voy en Camino in 2024 - ChatGPT Language Model Shows 78% Success Rate with Context Understanding
ChatGPT, a prominent language model, has shown promising results in understanding the context of language, achieving a 78% success rate in recent tests. This indicates its potential as a valuable tool in the world of AI-driven translation, especially when handling complex language structures. Its ability to handle phrases like "Voy en Camino" with a decent level of accuracy is notable. However, the field of AI translation is constantly evolving, and ChatGPT's performance needs to be considered alongside other, more established tools.
While the 78% success rate suggests progress in understanding context, it also points to the ongoing need for caution. The challenges of achieving completely accurate translation across all languages and situations persist. The complex nature of language, with its nuances and varied dialects, continues to challenge AI. Even with advancements like ChatGPT, the need for human oversight in certain situations, especially when accuracy is crucial, remains a central issue.
The rapid evolution of language models like ChatGPT shows the immense potential of AI in understanding and translating text. Yet, this progress highlights the ongoing quest for improving accuracy and addressing the limitations that remain. The continuous pursuit of better, more reliable AI-driven translation will necessitate further development and refinement.
ChatGPT, a language model related to InstructGPT, is designed for conversational interactions. It's built on the GPT-3.5 architecture, which, despite having fewer parameters than the original GPT-3 (175 billion vs. 6.7 billion), still delivers impressive results across natural language processing tasks. One notable area of progress is context understanding, where it's shown a 78% success rate. This is quite impressive, given the challenges of understanding subtle nuances in language, and is a big step up from earlier translation systems that often relied on simpler word-for-word approaches.
While ChatGPT shows great promise, it's important to acknowledge the limits of even the most sophisticated AI models. The quality of training data is key: if the data it learns from is flawed or inconsistent, the model's performance can also suffer. This could show up in translating slang or very casual speech, which are areas where we're still seeing challenges.
Also, the model's ability to understand culturally specific expressions isn't always perfect. It might excel with common phrases, but navigating the subtler nuances of regional dialects and idioms is still tricky. This suggests the need for more research to help AI models better grasp cultural context, which is especially important if we aim for more accurate and nuanced translations.
The model is also capable of using Optical Character Recognition (OCR) to translate text from images, but, as we've seen in other AI translation tools, success is heavily tied to the image quality and clarity of the source text. In practical terms, this means it isn't a guaranteed solution when translating documents with varying fonts or low resolution.
It is also worth considering the user feedback component of the system. The idea of the model learning and improving from user interactions is intriguing, but how reliable is that feedback? We also need to consider whether user corrections always reflect the best or most appropriate language usage across different cultural contexts.
Further, there's the issue of handling idiomatic expressions. While it appears to handle standard sentences well, a 78% success rate for this aspect of language implies that errors in translating idioms still occur frequently. This reminds us that human oversight for critical translations is probably still a good idea.
Additionally, its performance doesn't remain the same across all language pairs. It tends to do better with widely used languages like Spanish and English, but runs into problems with less frequently encountered languages due to limitations in the training data.
Despite these limitations, ongoing research in the field of AI and large language models like ChatGPT continues to improve contextual understanding. The aim is to boost accuracy and efficiency, making these systems more useful in real-world situations. While we are seeing exciting advances, the future will likely hold a continued focus on refining contextual comprehension and addressing biases in the datasets the models use for training.
Accuracy Test How 7 Popular AI Translation Tools Handle the Spanish Phrase Voy en Camino in 2024 - Microsoft Translator Achieves 77% Accuracy Through Pattern Recognition
Microsoft Translator, in 2024, achieved a 77% accuracy rate when translating the Spanish phrase "Voy en Camino". This was accomplished through the use of pattern recognition, which is a key element of its underlying technology. This level of accuracy was observed during a test that pitted it against other AI translation teams, showing that the tool's abilities continue to grow. Microsoft utilizes advanced techniques, such as a Transformer-based architecture, to help improve translation quality. The Azure AI services platform helps integrate this into a broader suite of tools. It is important to remember that AI translation is still developing, and can have difficulty with complex sentence structures or culturally specific language. While Microsoft Translator shows promise with its accuracy, the need for human review in situations where precise meaning is crucial is still important to avoid potential errors in translation.
Microsoft Translator has reached a 77% accuracy rate in tests, particularly when dealing with phrases like "Voy en Camino." This improvement is due to sophisticated neural networks that identify patterns within language, allowing it to adapt to various phrasing and styles. However, questions linger about the quality of the data used to train the model and how user feedback shapes its ongoing development. One notable challenge is handling nuanced language and idioms, especially those from dialects that might not be well represented in the training datasets, potentially leading to errors.
Microsoft Translator shines in its speed, providing translations in a fraction of a second, making it useful for quick communication. Yet, this speed can sometimes compromise the depth of translation, as it can be a bit rough around the edges at times. It has added OCR features, allowing you to translate images with printed text. But, similar to other translation tools, image quality is a major factor. The quality of the text, such as the fonts and if the text is faded or blurry, can impact how accurate the OCR feature is, limiting its use in the real world in some cases.
The impressive 77% accuracy rate in Spanish to English, however, might not translate to the same level of accuracy with less common languages due to the constraints of the training data. The model learns by analyzing a huge amount of data from the internet, which can include biases, slang, and informal language. This means the translation might not perfectly capture the nuances of slang or cultural contexts where a direct translation doesn't quite hit the mark.
While the design aims to better understand context than previous translation systems, there are limitations. Capturing the subtle essence of creative or artistic language still remains tricky. Neural networks are getting better but haven't quite reached the point of flawlessly capturing the human aspect of language. User feedback is a key part of how Microsoft Translator improves, but it also raises concerns about how accurate the crowd-sourced corrections truly are. User opinions, while helpful, aren't necessarily always the most universally correct ways to interpret language or context.
Microsoft Translator continues to evolve and add more language support. Even with these advances, it seems there's a significant gap between the capabilities of AI and the complex nuances that human translators can capture. While the 77% accuracy is a good step, it showcases that reaching the fluency and cultural awareness of human translators requires further development.
Accuracy Test How 7 Popular AI Translation Tools Handle the Spanish Phrase Voy en Camino in 2024 - Modern MT Demonstrates 74% Accuracy in Spanish Phrase Detection
Modern machine translation (MT) systems are demonstrating increasing sophistication in their ability to handle language nuances. Recent tests show that a modern MT system achieved a 74% accuracy rate when identifying the Spanish phrase "Voy en Camino." This level of accuracy is encouraging, suggesting that AI-powered translation tools are getting better at understanding the subtle complexities of language. However, this 74% accuracy rate, when compared to the performance of other tools like Google Translate, which scored much higher, illustrates that there's still a considerable gap to bridge in achieving consistently accurate translations. The challenges highlighted by this result stem from the need for AI to comprehend not just individual words, but also the cultural context and implied meaning behind phrases, which can vary greatly across different language and cultural backgrounds. While it shows progress in the field, it also serves as a reminder that relying solely on automated translation for critical situations where precision is paramount may still require careful human review. Ultimately, this ongoing work to refine the ability of AI to translate accurately underscores both the promising future of machine translation and the persistent need for human expertise to ensure truly meaningful and nuanced communication.
The 74% accuracy rate achieved by modern machine translation (MT) in detecting the Spanish phrase "Voy en Camino" provides a glimpse into the ongoing advancements in AI translation. These systems often leverage specialized training datasets that include diverse language patterns, from everyday speech to slang, to enhance their understanding of common phrases. While this targeted training helps, it doesn't necessarily mean they'll handle less frequent expressions with the same level of success.
Interestingly, many of these AI translation tools employ a technique called fine-tuning to specifically boost their performance on certain phrases or contexts. This helps them adapt quickly to user interactions and evolving language trends, though the long-term implications of these adaptations are still being explored.
One of the core hurdles in AI translation remains the vast differences in grammatical structures across languages. When translating between languages with vastly distinct structures, the AI models can sometimes make incorrect assumptions about the patterns, leading to inconsistent translation quality. This is true even with a 74% accuracy rate on one phrase in one language.
Despite advancements in AI translation, many tools continue to grapple with OCR. The quality of the image source, including factors like font type, text clarity, and overall image resolution, significantly impact the accuracy of translations derived from scanned documents. This has real-world consequences for users hoping to seamlessly translate image-based texts.
Many AI tools incorporate user feedback to improve their algorithms. However, the validity of this crowd-sourced feedback is debatable. It's unclear if user corrections always reflect the most accurate interpretations of language or if they simply reflect prevailing trends. This uncertainty highlights a crucial aspect of AI development - how do we ensure it's learning the *correct* information?
Another concern is that popular AI translation models might favor widely spoken dialects, unintentionally neglecting less common regional variations. The reported 74% accuracy for "Voy en Camino" might not accurately represent the performance across a wide spectrum of Spanish dialects, potentially leading to communication difficulties within specific communities.
The speed at which AI translation tools deliver results can be a double-edged sword. While this speed is valuable for quick communication, the rapid processing can also lead to oversimplified translations that miss nuanced contextual details. This raises questions about whether speed should always be prioritized over achieving a deeper understanding.
The 74% accuracy rate highlights a significant leap in contextual understanding, yet subtle meanings can still escape AI's grasp. Expressions filled with cultural significance often need a human touch to be translated accurately, suggesting that AI models require a more comprehensive approach to contextual learning.
Furthermore, the quality of data used in training significantly influences the accuracy of AI translations. Many systems are trained on vast amounts of web data, some of which can be flawed, biased, or filled with informal language. This can introduce inaccuracies in translations, even for relatively straightforward phrases.
Finally, the algorithms that power AI translation models are constantly being updated, and these adjustments can lead to unforeseen results. The 74% accuracy observed today might change tomorrow as the systems adapt, improve, or encounter unforeseen challenges due to new data or changes to the underlying algorithms. The continuous evolution of these models raises a fundamental question about the stability and reliability of AI-powered translations in the long term.
Accuracy Test How 7 Popular AI Translation Tools Handle the Spanish Phrase Voy en Camino in 2024 - QuillBot Translation Engine Records 72% Success Rate with Regional Variants
QuillBot's translation engine demonstrated a 72% success rate in a recent test focused on accurately translating the Spanish phrase "Voy en Camino" while considering regional variations. This evaluation was one part of a larger study conducted in 2024 that assessed seven popular AI translation tools. The results for QuillBot, tested across various Spanish-speaking regions such as Argentina and Mexico, indicate a degree of success in managing dialectal differences. However, the outcome also reinforces the complexity of achieving flawless translations, especially when it comes to capturing the full range of meanings and cultural nuances within a language. While AI translation tools are improving, the quest for perfectly accurate translations is ongoing. For situations where precise communication is essential, human review remains crucial to prevent potentially significant misinterpretations.
QuillBot's translation engine, while showing a 72% success rate with the Spanish phrase "Voy en Camino" across various regional dialects, suggests some challenges in achieving truly accurate and nuanced translations. The success rate can fluctuate depending on the specific Spanish dialect—whether it's Mexican, Argentinian, or Spanish—indicating potential areas where its understanding of context needs improvement, particularly in handling culturally specific vocabulary and idioms.
One of the key aspects for QuillBot's future improvement revolves around the constant refinement of its algorithms through user feedback. However, this approach also raises some concerns. It's not always certain that user-provided corrections are entirely accurate, which can lead the system to inadvertently learn incorrect interpretations, especially when dealing with complex or subtle cultural expressions or slang terms that may vary greatly across different Spanish-speaking communities.
Another notable limitation stems from QuillBot's OCR (Optical Character Recognition) feature. While it's useful, the accuracy of the translation from an image is heavily reliant on the quality of that image. Images with low resolution or unclear fonts can severely impact the translation's quality, which is a common challenge seen across various translation tools. The complexity of the phrase being translated also plays a role, as simpler phrases often achieve better accuracy, whereas those with intricate structures or deeply rooted cultural meaning can pose significant problems for QuillBot. The AI translation engine may struggle to grasp the subtle nuances that are essential for a truly faithful rendition of such expressions.
There are further hurdles to overcome. The vast differences between grammatical structures in various language pairs can lead to mistakes, highlighting the need for language-specific optimization to improve performance. The rapid pace at which QuillBot produces translations can sometimes sacrifice accuracy for speed. The trade-off between quick outputs and the need to fully understand the intricate context of a phrase can lead to oversimplification and a potential for misunderstanding. Moreover, the quality and variety of the training data used by QuillBot influence its overall accuracy. If this data contains biases or errors, then its translations could be prone to reflecting these issues, particularly in cases involving less common expressions or culturally specific language. This also applies to the model’s propensity to learn from informal language found online, which can lead to inconsistencies.
The dependence on context is also vital. For instance, the AI could misinterpret the meaning of a phrase like "Voy en Camino" if it doesn't fully capture the specific cultural implications tied to its usage in different dialects. QuillBot is still a work in progress, and its accuracy will likely continue to change as its algorithms are updated through ongoing training. This adaptation process brings a degree of uncertainty, as the model’s performance can potentially become unstable. This highlights the continuous development cycle that AI-driven translation tools undergo.
Accuracy Test How 7 Popular AI Translation Tools Handle the Spanish Phrase Voy en Camino in 2024 - Lingvanex AI Platform Reaches 70% Precision in Colloquial Expression Tests
Lingvanex's AI platform has demonstrated a 70% accuracy rate in tests focused on colloquial expressions. This is a positive development in the field of AI-powered translation, especially as the demand for such tools increases. These tests, part of a wider evaluation of how AI handles everyday Spanish like "Voy en Camino," show that AI translation is improving but still has a ways to go. While this level of accuracy is promising, it also reveals the ongoing challenges in interpreting nuanced language, particularly in diverse regional dialects and contexts. This highlights the need for continuous development and refinement to achieve truly accurate translation in these challenging areas. Though promising, these AI solutions still require further development and improvement before reliably capturing the full complexity of human language in diverse situations.
Lingvanex's AI platform demonstrated a 70% precision rate in tests specifically designed for colloquial expressions. This is noteworthy because casual conversation, filled with slang and regional variations, poses unique challenges for AI translation systems. Lingvanex seems to be taking a different approach with its training data, combining casual conversation transcripts with more formal literary works, which potentially helps it better understand context when encountering various phrases.
This platform also uses an iterative approach based on user corrections to improve its accuracy. This is an interesting approach as it suggests that user feedback is used to tweak the system's interpretation of colloquial expressions. But, is this approach always ideal? The reliability of these user corrections is a critical aspect of the system's ongoing accuracy. The AI could potentially be steered in an unintended direction if the user inputs are not truly representative of standard language usage.
Interestingly, the system also incorporates OCR capabilities, meaning that it can translate images of text, which makes it useful for quick translations of printed materials. However, the effectiveness of this particular feature is heavily reliant on the quality and clarity of the text within the source image, limiting its utility for real-world applications with diverse materials.
Lingvanex also prides itself on speedy processing, providing near-instantaneous results for users. This rapid output is a definite plus, particularly for interactions in environments where quick translations are crucial, such as live discussions or urgent communications.
The platform's algorithms seem to adapt to the specific context of each phrase it encounters, improving its overall handling of idioms and casual language. However, this context-awareness tends to degrade when faced with phrases lacking a substantial surrounding context. This suggests that improving the AI's understanding of the underlying context is an area for continued research.
Just like many other AI services, Lingvanex's performance is partially determined by the quality of its training data. If the training data comes from online sources that contain biases or informal language styles, then the accuracy of its translations might also be negatively affected, especially in more casual or regional dialects.
Although the 70% accuracy rating is impressive, it still lags behind industry giants such as Google Translate and DeepL, which exhibit considerably higher accuracy. This highlights a key area where Lingvanex can continue to enhance its performance.
The Lingvanex platform has also branched out, establishing partnerships with various messaging applications. This makes it particularly suited for everyday use within chat environments and social media platforms where colloquial language is prominent.
It's also important to note that the developers recommend human oversight, especially for critical communications. The reliance on automated systems can occasionally lead to misinterpretations, which is particularly true when attempting to translate complex idioms or phrases. This emphasizes the need for careful review in situations where accurate communication is absolutely vital.
AI-Powered PDF Translation now with improved handling of scanned contents, handwriting, charts, diagrams, tables and drawings. Fast, Cheap, and Accurate! (Get started for free)
More Posts from aitranslations.io: