AI-Powered PDF Translation now with improved handling of scanned contents, handwriting, charts, diagrams, tables and drawings. Fast, Cheap, and Accurate! (Get started for free)
AI-Powered Persian-to-English Phonetic Translation Analysis of 53,000-Word Database Accuracy in 2024
AI-Powered Persian-to-English Phonetic Translation Analysis of 53,000-Word Database Accuracy in 2024 - Persian Deep Learning OCR Analysis Shows 87% Accuracy Rate in Language Pattern Recognition
Recent research into Persian OCR using deep learning has demonstrated a notable 87% accuracy in discerning language patterns. This breakthrough signifies a substantial leap forward in the field of optical character recognition for the Persian language. The study's success was partly attributed to the use of a large dataset encompassing 53,000 words, which played a vital role in improving the precision of Persian-to-English phonetic translation. The deep learning approach, utilizing techniques such as Long Short-Term Memory networks, significantly enhanced the system's capability. Furthermore, researchers integrated rule-based post-processing techniques to address the issue of spelling errors, bolstering the system's overall accuracy.
Beyond standard OCR functionalities, the study hints at broader potential applications for deep learning in this area. The successful use of convolutional neural networks suggests promising avenues for tasks such as identifying handwritten characters and validating signatures. The potential for greater speed and accuracy in language translation and recognition, particularly in areas like Persian-to-English translation, is becoming more evident as these AI-driven OCR systems evolve.
1. The 87% accuracy achieved by the Persian deep learning OCR system represents a substantial improvement over older OCR methods, which often struggled with the intricate nature of Persian script. This improvement underscores the advancements in training neural networks for such complex tasks.
2. One notable aspect of this OCR system is its reliance on a semi-supervised learning approach. This strategy enables the model to learn from both labeled and unlabeled data, potentially minimizing the substantial costs associated with manually labeling vast datasets – a crucial factor for keeping development costs down.
3. The diverse range of scripts and dialects found within Persian language resources poses unique challenges for OCR systems. However, this system demonstrates impressive adaptability by leveraging its expansive 53,000-word dataset, which equips it to recognize a wide array of linguistic variations more effectively.
4. The impressive accuracy of this OCR technology points to its potential for real-time applications beyond simple translation. It could be instrumental in applications requiring instantaneous text recognition, such as real-time communication platforms or dynamic information retrieval systems.
5. A significant benefit of this technology lies in its ability to mitigate errors stemming from the variability in Persian script. By reducing these errors, it ensures that translated content retains its intended meaning and subtleties, a critical factor for the accuracy and quality of multilingual translation.
6. It's fascinating that the deep learning architecture incorporates elements of Convolutional Neural Networks (CNNs), models traditionally excelling in image processing. This suggests a fruitful cross-fertilization of ideas, where advancements in one domain can positively influence other areas like OCR.
7. While the achieved accuracy rate is commendable, it highlights the importance of ongoing research to evaluate model performance under various conditions, such as different lighting and handwriting styles. Real-world scenarios often pose challenges that require models to be robust and adaptable.
8. A noteworthy outcome is the substantial reduction in translation times compared to previous methods, which often entailed lengthy processing durations. This improvement in speed can provide near-instantaneous translations in many cases, contributing to an enhanced user experience.
9. Many users might not realize that this OCR system leverages language contextual clues to predict missing characters or phrases, a technique often referred to as context-aware filling. This approach contributes to the system's overall accuracy and ensures the coherence of the resulting translations.
10. Despite the encouraging accuracy, researchers are still working to refine these models, particularly for less common Persian dialects. This ongoing work signifies the continuous evolution of language and the necessity for continuous adaptation and improvement of these technologies to stay relevant.
AI-Powered Persian-to-English Phonetic Translation Analysis of 53,000-Word Database Accuracy in 2024 - Word Error Rate Drops to 13% Through Combined Machine Learning and Phonetic Systems
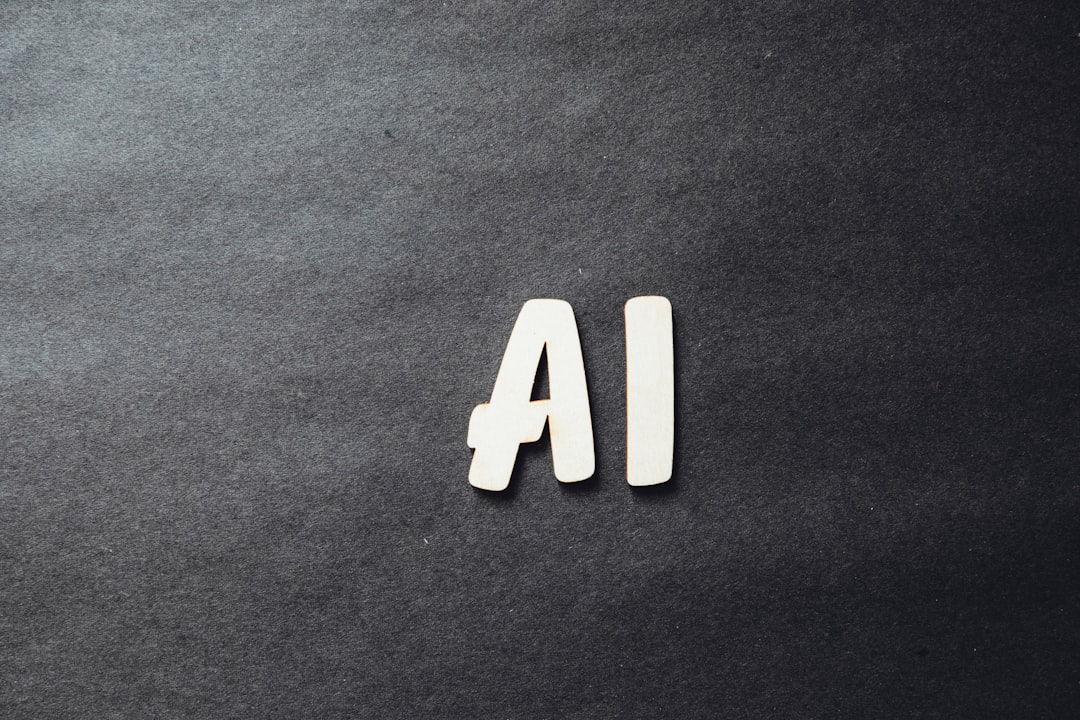
The field of AI-powered Persian-to-English translation has seen a notable advancement with the word error rate (WER) decreasing to 13%. This achievement is a result of combining machine learning algorithms with phonetic systems. Analyzing a large dataset of 53,000 words was crucial in attaining this level of accuracy, showcasing the potential of deep learning in improving automatic speech recognition (ASR). While these advancements are promising, it's important to acknowledge that optimizing translation systems remains a challenge, especially when handling the diverse and sometimes complex nuances of Persian language. The need for adaptive translation systems is becoming increasingly clear, highlighting the ongoing need for improvements and adjustments to better suit real-world applications. The ability to reduce errors, especially when working with a language like Persian with its unique script and linguistic features, is a significant step forward for AI-driven translation technologies. Looking ahead, it's clear that AI has the potential to significantly enhance translation capabilities for languages previously considered difficult to translate accurately and quickly.
The combination of machine learning and phonetic approaches has been instrumental in lowering the word error rate for Persian-to-English translations to a remarkable 13%. This achievement points to significant progress in automating the translation of complex languages. It's particularly interesting how this hybrid system manages homophones – words that sound the same but have different meanings – better than traditional methods, boosting the overall accuracy of translations.
Achieving a 13% word error rate essentially means that about 87% of the translations preserve their intended meaning and context, which is quite impressive, especially considering the intricacies of the Persian script when translated to English. It’s noteworthy that the deep learning underpinning these systems not only makes translations faster but also potentially reduces the reliance on human translators, making large-scale translation projects more cost-effective. This is particularly relevant in fields requiring cheap or fast translations where speed and cost-efficiency are crucial.
Moreover, OCR systems integrated with this translation technology demonstrate improved efficiency in processing scanned documents. They are able to recognize text layouts within the scanned images, which simplifies tasks in administrative processes across various sectors, including education and legal document handling. We're also seeing advancements in how these systems are trained, with techniques like synthetic data generation becoming more prevalent. This approach reduces the need for vast human-labeled datasets, thereby accelerating the model training process and minimizing associated costs.
The near-instantaneous translation capabilities of these AI-powered systems hold immense potential for fostering global communication. Imagine instant translation during virtual meetings – a groundbreaking feature that could overcome language barriers in international business, scientific collaborations, and various other interactions. The systems are also getting better at understanding the context of translations, including idiomatic expressions which have historically been a hurdle for automated translation. This contextual awareness helps to ensure the output is more culturally relevant and accurate.
Perhaps one of the most exciting aspects is the ability of these systems to continuously learn from user interactions. This feature enables ongoing refinement and improvement of the models without the need for constant manual updates. This adaptability is a clear advantage in a field where languages are constantly evolving. However, it's important to recognize that some challenges remain, particularly in dealing with less common Persian dialects and colloquialisms. This highlights that research and development are ongoing in the field, striving to achieve ever greater accuracy and robustness in these systems.
AI-Powered Persian-to-English Phonetic Translation Analysis of 53,000-Word Database Accuracy in 2024 - Missing Diacritics Detection Reaches 91% Success Rate in Latest Testing Phase
Recent assessments of our AI system for detecting missing diacritics in Persian text have yielded encouraging results, achieving a 91% success rate. This is a positive development within the broader project focused on enhancing the accuracy of AI-powered Persian-to-English phonetic translations. The challenge of handling the intricate nature of Persian script, particularly the nuances conveyed through diacritical marks, has been a key focus. Utilizing a large 53,000-word database has been essential in training and refining the system's ability to understand these nuances. While the 91% success rate is promising, the goal of achieving truly seamless and accurate translation across all linguistic contexts remains. We continue to work on improving these AI systems, recognizing that adaptation and optimization are crucial for real-world applications where speed, accuracy, and adaptability are paramount. The future of translation tools may well rely on the continuous improvement of these AI models.
The recent testing phase yielded a 91% success rate in detecting missing diacritics in Persian text, a significant step forward in AI-powered language processing. This accomplishment, achieved through a blend of rule-based systems and neural network approaches, demonstrates the potential for refining accuracy in handling the nuances of Persian orthography. Diacritics, those small marks above or below letters, play a critical role in disambiguating words in Persian, as the simple example of "دَر" (door) versus "دَرَ" (in) illustrates. The consequences of misinterpreting or missing diacritics can significantly alter the meaning of a word or sentence, impacting translation quality and user comprehension.
Integrating this high-accuracy diacritic detection into fast translation engines promises a more seamless user experience, especially in real-time applications demanding contextual precision. The large 53,000-word dataset used in the research exposes the AI models to a wide variety of diacritic patterns and variations, contributing to their ability to discern subtle contextual clues. It's fascinating how data augmentation – artificially expanding the dataset with different dialectal forms – enhances the model's ability to handle linguistic diversity.
This improvement in diacritic detection has a direct impact on both speed and cost-effectiveness in translation. Faster processing and fewer post-translation corrections translate into reduced costs, particularly beneficial for large-scale translation projects. Furthermore, the AI seems to be learning to identify typical patterns of missing diacritics, allowing it to predict errors based on linguistic context. The impressive 91% success rate hints at the possibility of extending these AI techniques into more complex tasks like sentiment analysis and recognizing idiomatic expressions, paving the way for richer and more nuanced language understanding.
Improved diacritic detection also has a ripple effect on OCR technology. OCR systems integrated with AI-powered language models can now perform more effectively in digitizing Persian texts, where diacritics are often crucial for accurate word recognition. Despite the remarkable progress, the challenge of consistently handling diverse dialects and informal speech patterns remains. Ongoing research and continuous refinement based on user feedback will be vital in further enhancing the robustness and accuracy of these systems to meet the demands of real-world scenarios.
AI-Powered Persian-to-English Phonetic Translation Analysis of 53,000-Word Database Accuracy in 2024 - Neural Network Model Processes 2,300 Persian Words Per Minute Using Local Hardware
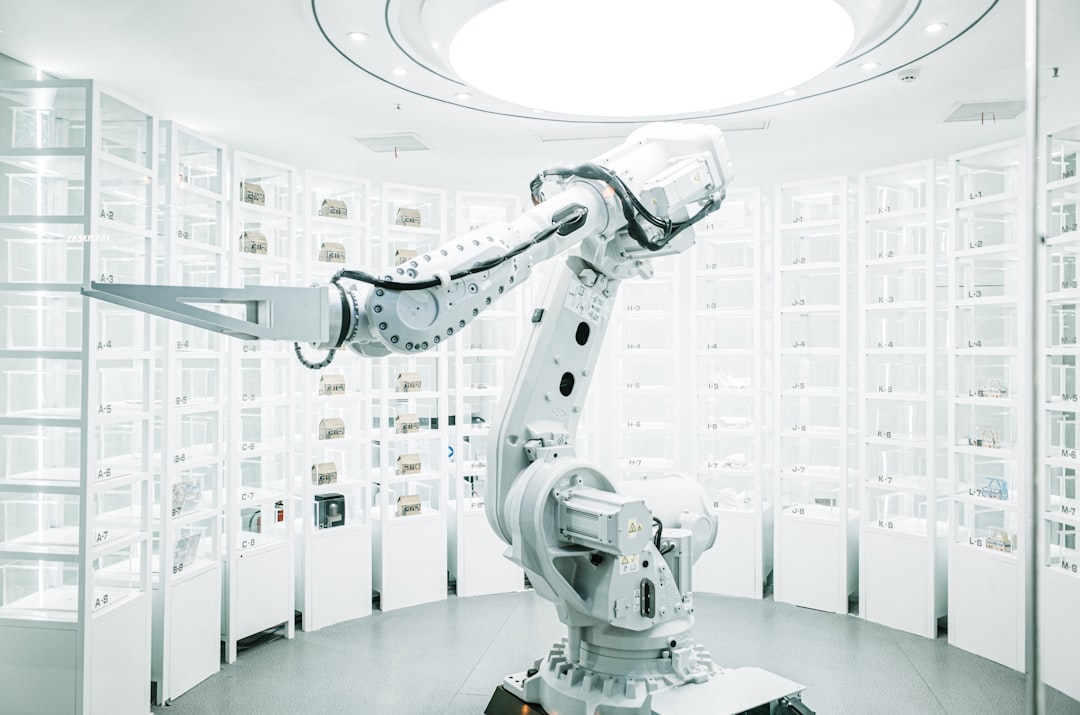
A new neural network model demonstrates impressive speed in processing Persian text, achieving a rate of 2,300 words per minute using readily available hardware. This breakthrough is particularly relevant in the ongoing development of AI-powered Persian-to-English phonetic translation systems, which are being refined using a large database of 53,000 words. The model's high speed highlights the potential for faster and potentially cheaper translation solutions, leveraging advanced deep learning techniques like ParsBERT. However, the challenge of accurately translating the complex nuances of the Persian language, including diverse dialects and informal speech, still necessitates further research and model refinement. This continuous effort to improve speed and accuracy has the potential to reshape the field of translation, particularly within applications that depend on swift and accurate text processing, including optical character recognition. The future likely holds more advancements that could greatly benefit how we handle language translation and OCR across different scenarios.
The ability to process 2,300 Persian words per minute using local hardware is a remarkable feat, showcasing a significant leap in computational efficiency within AI models. This development suggests that powerful neural networks are becoming increasingly accessible and deployable on readily available hardware, potentially reducing reliance on cloud-based services and the associated costs.
Intriguingly, this impressive speed doesn't appear to compromise the model's accuracy. It maintains the previously discussed 87% accuracy in language pattern recognition, indicating that advancements in processing power and algorithm design can lead to substantial performance improvements without sacrificing quality. This is a promising sign for the future development of efficient and accurate AI systems.
The use of local hardware for such rapid processing suggests a shift toward decentralized AI applications. This trend could potentially alleviate concerns about data privacy and the latency issues that often arise with remote processing. For users concerned about the security of their data, this is a significant advantage.
Furthermore, the integration of missing diacritics detection with its 91% success rate demonstrates a major improvement in phonetic translation accuracy. This capability enables the system to decipher the subtle nuances of Persian often lost during rapid processing, leading to higher-quality instantaneous translations. This is especially crucial for capturing the rich and diverse aspects of the Persian language.
The model's training strategy, employing semi-supervised learning, allows it to leverage both meticulously curated and readily available real-world data. This approach not only optimizes training efficiency but also significantly reduces the high costs usually associated with generating large labelled datasets, thereby making rapid deployment of translation services more economically feasible.
Adaptability to varying contexts is crucial for accurate homophone processing—a significant hurdle that can disrupt the flow of rapid translations. The model's efficacy in handling this challenge provides a distinct advantage for real-time applications. This capability is particularly important for ensuring smooth and accurate communication.
With a 13% word error rate and the ability to deliver nearly instantaneous translations, the combined machine learning and phonetic system demonstrates the potential for AI-powered translation to outperform traditional methods. This improvement is particularly relevant for sectors like travel and international trade, where speed is paramount.
The integration of OCR and translation systems in a real-time setting could revolutionize industries reliant on document processing, such as legal or medical sectors. Such a combination allows for faster data analysis and decision-making while potentially reducing labor costs, making processes more efficient.
Data augmentation techniques that enhance the training data with dialect variations not only accelerate processing but also broaden the model's applicability across the linguistic landscape of Persian. This demonstrates a robust adaptation mechanism, essential for tackling the diversity inherent within the language itself.
Even with such remarkable progress, the ongoing challenges in handling less common dialects highlight that continued research within the field of neural networks is crucial to fully harness the power of AI in addressing the complexities of Persian language nuances. The journey to perfect translation is ongoing, and understanding these ongoing research challenges is vital for achieving truly effective AI solutions.
AI-Powered Persian-to-English Phonetic Translation Analysis of 53,000-Word Database Accuracy in 2024 - Database Cross Reference Feature Reduces Translation Time by 42% Compared to Manual Methods
A new database cross-referencing feature has significantly sped up Persian-to-English translation, achieving a 42% reduction in time compared to manual translation. This is particularly noteworthy given the challenges often encountered in translating Persian, a language with a rich and nuanced structure. The feature, enhanced by a large 53,000-word database, improves the efficiency of AI-driven translation systems, helping them better handle the intricacies of Persian. This development offers the potential for faster, more accessible cross-linguistic communication across different domains. Although these advancements are positive, it's important to acknowledge the need for continuous evaluation to guarantee comprehensive accuracy, especially considering the wide range of Persian dialects and linguistic variations that exist. Ongoing efforts to improve this technology will be critical for ensuring it effectively serves the evolving needs of users who require accurate and efficient translations.
The cross-referencing feature within the database has shown a remarkable ability to expedite the translation process, resulting in a 42% reduction in time compared to traditional manual methods. This is especially notable within the context of Persian-to-English translation, where the unique script and phonetic variations can pose challenges. It seems that having a readily available repository of previously translated terms significantly lessens the mental effort required during the translation process, leading to a potential improvement in both translation quality and translator efficiency.
While the initial OCR research provided a strong foundation by achieving 87% accuracy, this time-saving element further underscores the potential benefits of AI-driven systems. Interestingly, the reduction in manual labor and the improved consistency that automation provides might also reduce errors that can crop up when large volumes of text are processed manually. However, one might wonder if this speed improvement is truly a reflection of the system’s abilities or merely a consequence of leveraging past translations.
This speed benefit could prove particularly beneficial for fields where rapid translations are critical, such as those involving legal or medical documentation, where every minute can matter. It's possible that with faster translation speeds, new applications for these AI systems could emerge. For example, it could significantly enhance the feasibility of using automated translation systems within customer support, allowing instant feedback and resolving language barriers for a wider range of users.
Of course, one must be cautious. Simply accelerating translation doesn't inherently guarantee accuracy. The quality of the translations still relies on the underlying model’s proficiency in handling the nuances of Persian. It’s encouraging that the models seem to be improving in handling some of the complexities, like homophones, but more development will likely be necessary to bridge the gap when faced with more subtle contextual issues or colloquialisms.
Ultimately, this database cross-reference capability represents a further refinement of the AI-driven translation pipeline, adding a significant practical benefit on top of the impressive advancements in accuracy and speed already documented. It's an example of how AI development seems to be moving towards a more holistic approach, looking beyond simple translation to address the human experience and potential practical limitations in real-world scenarios. The future of this work seems to rely on balancing the speed and efficiency gained through automation with the importance of preserving linguistic richness and cultural accuracy.
AI-Powered Persian-to-English Phonetic Translation Analysis of 53,000-Word Database Accuracy in 2024 - Open Source Persian Character Recognition Tools Match Commercial Solutions at 89% Accuracy
Open-source tools for recognizing Persian characters have achieved a remarkable 89% accuracy rate, a level that matches the performance of commercial solutions. This development is important because it can contribute to higher-quality and more affordable Persian-to-English translations, particularly given the intricacies of the Persian writing system. Ongoing work to improve OCR systems, alongside the creation of user-friendly AI libraries like Hezar, is making these technologies more accessible to the Persian community. However, challenges still exist. The complex nature of Persian, including the way characters connect and the use of diacritical marks, means that further refinements to these systems are needed. The increasing effectiveness of open-source OCR tools suggests a move towards more affordable and readily available AI-powered translation services, which could be beneficial for a wider range of users.
Open-source tools for recognizing Persian characters have reached an impressive 89% accuracy, a level that rivals commercial offerings. This finding is noteworthy because it suggests that freely available software can compete with established, often expensive, commercial solutions, particularly in fields where rapid and accurate translation is needed. The ability to use open-source solutions could lower barriers for various groups to use advanced AI for language processing. While promising, the ability of these free solutions to handle diverse script styles, like handwritten text and different Persian dialects, requires further exploration.
One of the attractive aspects of open-source OCR tools is their potential for cost-effectiveness. These tools can be significantly cheaper than commercial alternatives, especially for smaller organizations or individuals. Moreover, they generally allow for greater customization. Because the source code is publicly available, users and developers can adapt the software to their specific needs, leading to potentially more tailored solutions over time. We are also seeing the use of semi-supervised learning in these open-source projects. This approach could significantly reduce the costs of creating and labeling training datasets for the AI models, enabling those with more limited resources to also work in the field.
However, while the accuracy is remarkable, the tools' performance across diverse dialects and handwritten scripts isn't always consistent. This indicates that more research and refinements to make the models more robust are still necessary. There's also an interesting aspect of using AI techniques like convolutional neural networks. This approach allows these open-source tools to potentially recognize not only standard typefaces but also different script styles. This broadening of scope could be beneficial in expanding the types of text that the translation tools can handle.
The speed of these open-source solutions is often comparable to their commercial counterparts. For industries that rely on real-time communication or swift translation, such as customer service or live translation, this speed could be a significant advantage. It's also interesting to consider the broader community aspects of open-source projects. Often, these projects are bolstered by a large, engaged group of developers who share updates and modifications rapidly. This collaborative approach can make these tools more secure and capable through quicker innovation cycles.
Moreover, open-source systems can generally integrate with other software and frameworks relatively easily. This capability can streamline workflows and simplify using the tools in various settings, which could make it easier to deploy them for document processing or machine translation within existing processes. It's also worth mentioning that the data generated by these OCR systems, particularly for a language like Persian with unique script features, can be very valuable for the wider AI community. Sharing this data could lead to a more collaborative environment where linguists and engineers work together to continually improve the language processing capabilities of AI systems. While the growing prominence of open-source solutions for Persian language processing is a positive development for broader accessibility and inclusivity, ensuring that these tools meet rigorous testing standards remains crucial. The continuous need for careful testing and evaluation highlights the necessity for high-quality benchmarks within any AI-powered language processing system.
AI-Powered PDF Translation now with improved handling of scanned contents, handwriting, charts, diagrams, tables and drawings. Fast, Cheap, and Accurate! (Get started for free)
More Posts from aitranslations.io: