AI-Powered Spanish Academic Translation 7 Cost-Effective Tools for Graduate Students in 2025
AI-Powered Spanish Academic Translation 7 Cost-Effective Tools for Graduate Students in 2025 - Google Translate Professional Learns Medical Spanish Terms For Research Papers
As of May 2025, the use of readily available tools like Google Translate for navigating medical terminology in Spanish research papers remains common practice among graduate students. Its speed and zero cost offer a significant advantage when grappling with volume. However, assessments of its accuracy in specialized medical contexts present a more complex picture. While studies acknowledge its utility and sometimes decent performance, particularly when compared to earlier iterations or for general language, they consistently point out errors specific to technical and medical vocabulary. These inaccuracies can potentially lead to misunderstandings in critical research materials. Therefore, simply pasting text into such a tool and trusting the output for complex medical concepts is approached with increasing caution. Instead, a developing trend favors using these tools as a starting point, perhaps followed by validation using more sophisticated AI models trained on specialized datasets or through human review, acknowledging the current limitations in handling highly nuanced scientific language accurately.
The effectiveness of translation technologies like Google Translate when handling specialized language, specifically medical Spanish for research purposes, continues to be a subject of investigation. Recent analyses have focused on evaluating how these broadly available platforms and other AI-driven systems perform with precise medical terminology. These studies aim to map out where such tools exhibit reliability and, importantly, where they struggle or introduce inaccuracies – findings critical for academics relying on them for scholarly work.
The context for graduate students seeking efficient and affordable solutions for academic translation remains pertinent in 2025. Tools are evolving to better support the translation of research documents, with an emphasis on preserving the specific vocabulary and structural nuances required in scientific writing. While direct translation services form a baseline, there's interest in approaches that potentially combine different AI functionalities to tackle varied aspects of the translation process, reflecting a broader effort to ease the navigation of Spanish medical content for researchers.
AI-Powered Spanish Academic Translation 7 Cost-Effective Tools for Graduate Students in 2025 - AI OCR From Microsoft Lens Scans Handwritten Spanish Notes At 99% Accuracy
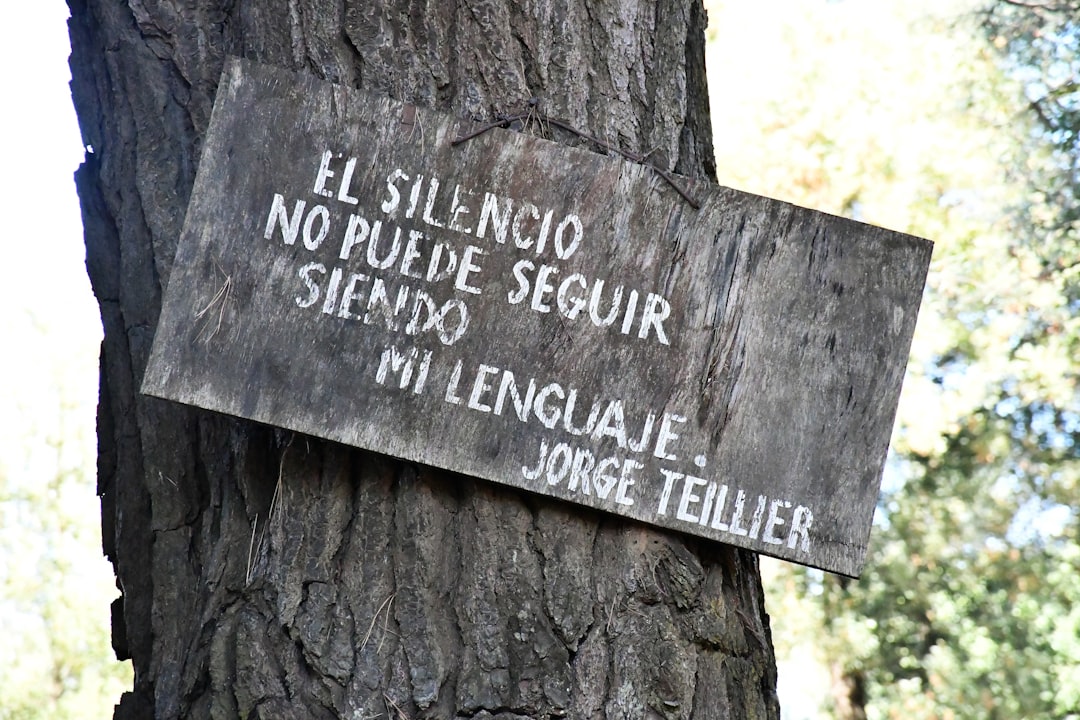
As of May 2025, reports highlight advancements in optical character recognition technology, with figures suggesting tools used via platforms such as Microsoft Lens can scan handwritten Spanish notes with high precision, potentially reaching 99% accuracy in some instances. This figure is particularly notable given that accurately recognizing varied human handwriting has historically posed a significant hurdle for automated systems, often resulting in considerably lower accuracy rates compared to printed text. Such progress offers a promising avenue for graduate students tasked with digitizing personal research notes or older documents. The continuous development in AI-powered OCR aims precisely at tackling the complexities introduced by diverse writing styles. However, the variability inherent in handwriting means that relying on automated output without careful checking can still introduce errors that might compromise downstream tasks. Consequently, a manual review step remains advisable to ensure the fidelity of the digitized text before it's used for translation or analysis.
Claims regarding AI OCR technology, specifically as implemented in tools like Microsoft Lens for handwritten Spanish notes, suggest potentially high performance in digitizing challenging input. As of May 2025, the reported accuracy for this task is notably high, sometimes cited around 99%. Achieving such a figure for handwritten text, especially given the known significant variability in human writing styles—where recognition accuracy typically varies widely and averages are considerably lower than for uniform printed characters (which themselves can exceed 99% accuracy in high-quality scans)—represents a significant technical effort.
The system is designed to process the scanned text with low latency, facilitating rapid conversion directly from the physical note to a digital format. This speed is a practical benefit for students needing to quickly access or manipulate their notes digitally. Development efforts likely include training specific machine learning models on a broad range of Spanish handwriting samples to enhance recognition capabilities across different scripts and possible regional nuances.
From a cost perspective, the OCR functionality embedded within widely available applications like Microsoft Lens generally does not incur separate charges, making it an economically accessible option. The output is typically structured to be compatible with standard digital text formats, allowing for straightforward integration into other workflows, such as feeding the recognized text into translation software or document editors.
Beyond Spanish, the underlying OCR engine often supports multiple languages, providing versatility for academic work involving diverse linguistic materials. A functional aspect that can be critical for portability and access is the ability to perform the core recognition task offline, removing reliance on internet connectivity.
Systems like this frequently incorporate mechanisms for user feedback, allowing corrections to misrecognized text. While fixing immediate errors, this interaction can also theoretically contribute to refining the underlying AI models over time, though the effectiveness of this passive learning can vary. It's important to remember that despite high reported accuracies, the complex nature of handwriting means that human verification of OCR output remains a common practice in many real-world applications to prevent downstream data issues arising from recognition errors. The technical focus continues to be on improving handwriting and cursive recognition to close the gap with printed text performance. By automating the initial transcription, such tools aim to reduce the manual overhead associated with digitizing notes, theoretically allowing more focus on the content itself. Furthermore, standard accessibility features like text-to-speech are typically supported for the digitized output.
AI-Powered Spanish Academic Translation 7 Cost-Effective Tools for Graduate Students in 2025 - Free DeepL Academic Plan Handles 500 Pages Monthly Without Subscription
Among the tools available in 2025, DeepL presents a free option that serves graduate students, reportedly facilitating the translation of up to 500 pages monthly without requiring a subscription. This service employs AI technology aiming for accuracy and fluency across many languages, which is particularly useful for translating Spanish academic papers and supports various file types. While the capability to handle a considerable page count monthly is valuable at no direct cost, this free service does come with limitations, including typical restrictions on character limits for individual translations and missing some advanced features found in paid plans. Despite these constraints, it offers an accessible pathway for students facing significant translation volumes and can be considered alongside other software designed to improve writing or process digital documents.
A free offering from DeepL reportedly provides academic users the capacity to handle up to 500 pages per month without requiring a paid subscription. As of May 2025, this strikes as a significant potential resource for students navigating foreign-language research materials, offering a non-trivial volume for translation tasks.
The system is understood to be built upon sophisticated deep learning architectures. From an engineering perspective, this approach aims to move beyond simpler word-for-word substitution, attempting to model linguistic nuance and context, which is theoretically better suited for the complexity often found in scholarly writing compared to earlier machine translation paradigms.
One practical aspect highlighted is the tool's capability to largely preserve the original formatting of source documents. For academic outputs where layout, figures, and footnotes are integral to understanding and reference, maintaining this structure automatically during translation reduces manual clean-up work significantly.
Claims about the underlying models suggest training on extensive datasets, including academic specific texts. This is critical; the quality of output for technical or specialized vocabulary directly correlates with the relevance of the training data. While it should perform better than tools trained primarily on general corpus, domain-specific performance for highly niche fields will still require careful assessment.
Users frequently note the speed of translation. Reports indicate processing times are often exceptionally fast, with texts translating in seconds. For researchers working under deadlines, rapid access to translated content is a major operational benefit, enabling quick scanning or initial comprehension of large documents.
The free tier apparently allows for translation of entire documents. This is a key functional difference from many basic services that restrict input to limited character counts or prevent file uploads. The ability to process multi-page papers seamlessly is highly valuable for comprehensive literature review, though the exact definition of a "page" within the 500-page limit might warrant clarification.
A notable point concerns data privacy; the service states that uploaded documents are not retained or used to further train the models. This is an important consideration for academics handling potentially unpublished research or sensitive data, though ultimately, users must weigh the trust placed in such policies.
The platform supports a broad spectrum of language pairs, not just the most common ones. Its reported proficiency in translating between less standard combinations, such as Spanish and German, extends its utility for students involved in international research collaborations or studying less conventional linguistic connections.
A core technical capability cited is the improved contextual understanding during translation. Rather than isolated translation of phrases, the system attempts to interpret meaning based on the surrounding text. For academic writing, where terms can be highly context-dependent, this feature is crucial for reducing ambiguity and improving accuracy, although perfection is still an AI aspiration, not a guarantee.
The widespread adoption of the free plan among academic users appears to have organically fostered informal user communities. These networks provide spaces for students and researchers to exchange practical tips, workarounds, and best practices for maximizing the capabilities and managing potential limitations within the free tier's operational constraints.
AI-Powered Spanish Academic Translation 7 Cost-Effective Tools for Graduate Students in 2025 - Spanish Translation Voice Feature In Grammarly Student Reads Papers Aloud
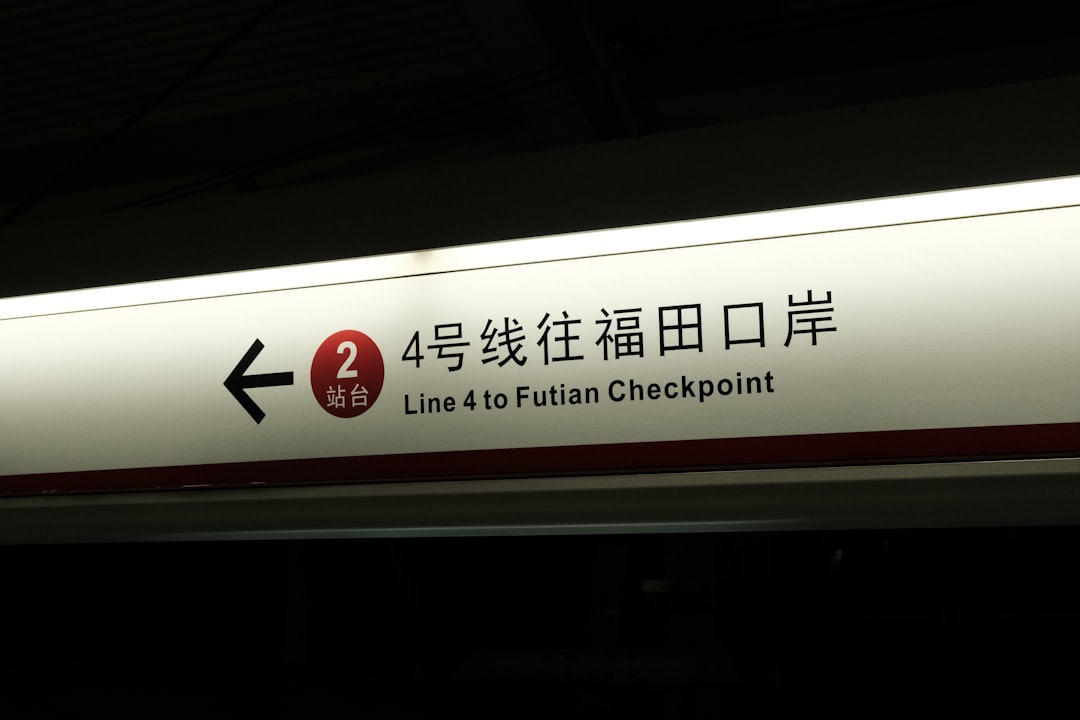
As of May 2025, Grammarly has reportedly launched a voice feature paired with its translation capability specifically for Spanish, allowing students to have their academic papers read aloud in the translated language. This functionality is intended to make written material more accessible for Spanish speakers and potentially aid comprehension by offering an auditory component alongside the text. Users are said to have the option to create unique voice profiles, which could add a layer of personalization to the experience. This AI-driven tool operates directly within the application environments where Grammarly is available and supports translation into Spanish, though it requires generative AI features to be active on the user's account. While the idea of hearing translated academic content is intriguing for learning and reviewing, how effectively the synthesized voice and translation handle the intricate vocabulary and sentence structures common in scholarly work remains a practical consideration for users.
Building on various approaches to processing Spanish academic texts using AI, another path involves integrating different modalities directly within a single tool. As of May 2025, we observe applications like Grammarly introducing functionality that couples translation with synthesized speech for Spanish content. The core idea here appears to be more than just passive translation; it's about rendering the translated text audibly.
From an engineering perspective, this merges a machine translation pipeline with a text-to-speech (TTS) engine. The complexity lies not only in the translation's fidelity to academic Spanish but also in the naturalness and clarity of the synthetic voice. Providing auditory feedback aims to offer an alternative channel for processing information, which researchers theorize could potentially reinforce comprehension compared to solely reading. There's an underlying assumption that hearing the translated output might highlight awkward phrasing or errors that are less obvious when simply scanning text, although the quality of the synthesized voice itself can introduce new cognitive load if it's overly robotic or unnatural.
Claims regarding real-time processing suggest the integration between translation and TTS is relatively tight, allowing for rapid conversion of text to speech. Achieving nuanced pronunciation and intonation, especially for specialized academic vocabulary, presents a significant technical hurdle for TTS systems. While the system might attempt some level of adaptation, true personalization to a user's specific needs or preferences in auditory output remains a complex challenge in speech synthesis research, often requiring substantial user interaction or data not typically available in a general writing assistant context.
Integrating this feature within an existing subscription service, rather than offering it as a standalone product, presents a different economic model compared to tools with per-use or volume-based charges. This bundling affects how users access the functionality, essentially distributing the development cost across a broader user base.
The approach embraces multimodal learning, which educational research often posits can enhance information retention. However, the practical effectiveness for complex academic material translated into a synthetic voice is an area where the real-world impact might vary significantly between individuals and subject matters. It's one method among others, potentially useful for certain learning styles or for initial review stages.
Addressing regional variations and accents in both translation and TTS output adds another layer of complexity. While systems might support major dialects, accurately reflecting the subtleties of numerous regional linguistic features in both the translated text and the spoken voice is a formidable technical undertaking. The potential for this to serve as an accessibility tool for students facing visual or reading challenges is evident, aligning with broader goals of making digital content more universally usable.
Ultimately, such features represent the ongoing exploration into how combining AI capabilities—translation, text analysis, and speech synthesis—can provide novel ways for students to interact with academic material. The degree to which a synthesized voice reading a machine translation truly enhances deep comprehension or refines language skills, however, likely depends heavily on the quality of both the underlying translation and the TTS, and warrants empirical evaluation rather than simply accepting theoretical benefits. The continuous refinement process relies on user interaction, but collecting and effectively leveraging feedback to improve both translation and voice quality in tandem poses non-trivial data science and engineering challenges.
AI-Powered Spanish Academic Translation 7 Cost-Effective Tools for Graduate Students in 2025 - ChatGPT Plus Offers Context-Aware Spanish Translation For Under $20
As of May 2025, considering cost-effective options for Spanish academic translation, ChatGPT Plus offers an approach priced under $20 monthly. This service tier aims to provide a more reliable level of access compared to free versions, particularly during times of high user volume, which can be important for consistent workflow. It leverages more advanced AI models than the base offering, with the goal of producing translations that are better at understanding and applying context, which is a crucial aspect for academic material where precise meaning is often nuanced. For graduate students needing to translate Spanish research papers, this emphasis on context-aware translation is a core benefit. While still machine translation and subject to potential inaccuracies, particularly with highly specialized terminology, the subscription model attempts to deliver a more capable and readily available tool. Additionally, features like browser extensions are available, designed to integrate the translation process directly into a user's research environment, aiming for smoother handling of Spanish text encountered online or within documents. This positions it as a tool offering enhanced capabilities and consistency for a relatively low monthly cost.
Among the digital tools being evaluated for academic purposes, the offering branded as ChatGPT Plus highlights a focus on what's termed "context-aware" Spanish translation. For researchers grappling with Spanish-language materials, the ability for a system to interpret text based on the broader passage rather than isolated phrases could theoretically enhance the fidelity of translations, particularly crucial where specific terminology and complex syntax are prevalent. The suggested price point for this service, reportedly less than twenty US dollars monthly, positions it as a relatively accessible option compared to dedicated human translation services, which are often prohibitively expensive for students facing substantial translation workloads.
However, achieving truly robust context awareness across the diverse and often highly specialized domains found within academic research presents a non-trivial technical challenge. While general improvements in translation quality through larger, more sophisticated models are evident, their performance in accurately capturing the nuances of highly specific Spanish academic subfields or preserving the intended meaning in intricate logical constructions requires careful scrutiny. As with many AI systems, user reliance needs to be balanced with a critical assessment of output accuracy, particularly when translating core arguments or technical data within scholarly papers. The effectiveness in practice will depend heavily on the training data supporting the model for relevant academic language.
AI-Powered Spanish Academic Translation 7 Cost-Effective Tools for Graduate Students in 2025 - Apple Visual Spanish Translator App Works Offline On Campus WiFi
As of May 2025, the built-in translation feature available on Apple devices presents itself as a tool for students navigating language barriers, particularly its reported capacity for offline use which can be relevant on college campuses where consistent internet access isn't always guaranteed. This functionality is said to support translating text, speech, and content captured through the device's camera – addressing the "visual" aspect mentioned in its application. The ability to download language packs, including Spanish, facilitates its operation independent of an active network connection. Features such as automatic language identification aim to streamline the process for users interacting with diverse Spanish-language materials. While offering convenience for quick translations of phrases or basic texts encountered during academic work, the reliability of such automated tools for capturing complex academic nuances or technical vocabulary requires careful consideration. As with any automated system, a critical approach to the output is advisable, recognizing that the subtleties essential for accurate scholarly understanding might not always be perfectly rendered. This positions it as a readily available option on devices students often already possess, suitable for initial comprehension tasks when offline access is a priority.
Here's a look at some of the engineering and design choices observed in the Apple offering for visual and offline Spanish translation, viewed from a technical perspective as of May 2025:
- A key technical approach here is providing offline translation capabilities. This design choice tackles the practical issue of unreliable network access common in institutional environments like university campuses. By enabling the download of language models, the system shifts processing requirements to the device itself, theoretically ensuring translation continuity regardless of WiFi signal strength or internet congestion, although the computational demands on the device and the size of the necessary model downloads are practical considerations.
- The integration of visual input via camera, incorporating Optical Character Recognition (OCR), adds a dimension beyond standard text or voice input. This allows the system to process text encountered in the physical environment—from printed pages to potentially handwritten notes. The challenge lies in achieving reliable recognition and subsequent translation of text captured dynamically through a camera feed, particularly with variations in lighting, font, and physical context.
- Regarding processing speed, the aim appears to be near real-time translation. Achieving low latency is critical for user interaction, especially when dealing with visual input or conversation. This implies computationally efficient models optimized for mobile hardware, balancing translation accuracy with the need for rapid output, which can be a complex optimization problem.
- The system reportedly attempts a degree of contextual awareness in its translation. While machine translation has historically struggled with nuance, especially in domain-specific language, utilizing more sophisticated models to consider surrounding phrases aims to improve fidelity. However, mastering the intricate context within varied academic disciplines remains a significant technical hurdle for general-purpose models.
- From an economic standpoint, integrating these capabilities into an existing device ecosystem potentially offers cost-effectiveness by leveraging hardware and platform investments already made. The offline feature, by reducing reliance on cellular data or premium network access, contributes to the overall utility without requiring continuous operational expenses related to network connectivity for the core translation task.
- The design emphasizes a user-friendly interface. The engineering challenge involves abstracting complex underlying processes—like OCR, language detection, and model inference—behind intuitive controls, making the tool accessible for users who are primarily focused on the translation outcome rather than the technical mechanics.
- Support for multiple languages implies an underlying framework designed for scalability across different language pairs, requiring robust linguistic models and processing pipelines adaptable to diverse grammatical structures and vocabularies beyond just Spanish.
- Voice input functionality is another modality integrated, enabling users to speak queries or content for translation. This requires accurate Speech-to-Text (STT) processing, which must handle varying accents, speaking speeds, and background noise, and then feed this transcription into the translation engine.
- Incorporating a mechanism for user feedback on translation quality is a common strategy for iterative improvement in AI systems. Technically, this involves capturing user corrections or ratings and using them as data points for model retraining or refinement, although effectively incorporating such noisy and potentially sparse feedback into complex deep learning models is non-trivial.
- The potential for integration with other applications within the ecosystem suggests efforts to enable interoperability, allowing translated text to be easily transferred or utilized in other productivity tools. This streamlines workflows for tasks like incorporating translated material into documents or presentations, requiring standardized data exchange formats and APIs.
AI-Powered Spanish Academic Translation 7 Cost-Effective Tools for Graduate Students in 2025 - Whisper AI From OpenAI Converts Spanish Audio Lectures To Text For Free
OpenAI's Whisper system presents itself as a resource for students, providing a no-cost method to transform spoken Spanish content, such as academic lectures, into written format. Built upon extensive training data covering a wide array of audio, this speech recognition technology is designed to manage different speaking styles and background sounds. Its primary function is breaking down audio streams and rendering them as text efficiently. For graduate students needing to work with recorded lectures or interviews in Spanish, this capability can significantly ease the process of creating study materials or research transcripts. While the tool offers accessibility and a rapid means of digitizing spoken words, it's prudent to review the output critically, especially when dealing with technical terms or specific academic discourse, as automated systems can introduce unexpected errors. Its availability without direct charge positions it as a practical option for those on a tight budget needing to process Spanish audio.
The AI system known as Whisper, originating from OpenAI research, demonstrates a foundational capability for converting spoken language into text across numerous languages, not solely focusing on Spanish. This underlying design, often described as a transformer-based architecture trained on vast audio datasets, appears engineered to handle diverse linguistic inputs, suggesting a potential for utility in academic environments dealing with multilingual or regionally varied Spanish audio sources.
Evaluations of its performance for spoken Spanish transcription frequently cite notable accuracy levels, potentially exceeding ninety percent depending on audio conditions. While such figures are promising for reliably capturing lecture content, practical performance can fluctuate significantly based on factors like speaker clarity, background noise, technical vocabulary density, and the recording quality itself. Achieving high fidelity in transcribing nuanced academic discourse under non-ideal conditions remains a technical challenge.
A reported feature involves processing audio streams relatively quickly, enabling near-instantaneous conversion of speech to text. This capability suggests potential for real-time transcription during live events or lectures, offering an immediate text counterpart to spoken information. However, actual processing speed and latency can depend on the computational resources available, which might introduce minor delays between speech and its transcribed output.
One aspect often highlighted is the accessibility of the core models, sometimes through open-source releases or free tiers of related services. This availability reduces the direct financial burden for graduate students needing transcription, providing a cost-alternative to professional services. Yet, utilizing the model might still involve indirect costs such as computational resources if running locally or potential limitations in free hosted versions.
The design facilitates integration, allowing developers or services to incorporate the speech-to-text functionality into other tools or platforms. This means the transcription engine could potentially be embedded within existing academic workflows or custom applications, creating possibilities for automated processing of recorded material within familiar digital environments.
Despite the complexity of the underlying AI models, access points to Whisper functionality, whether via APIs or wrapper applications, often prioritize a straightforward user experience. The aim appears to be lowering the technical threshold for non-expert users, enabling students to perform transcription tasks without requiring deep technical knowledge of the AI model itself.
The extensive training data is intended to equip the model with the capacity to recognize variations in Spanish pronunciation, including different accents and regional dialects. While this feature theoretically improves robustness in diverse settings, the degree of success can vary; accurately handling the full spectrum of spoken variations and idiosyncrasies in academic speech remains an ongoing refinement task for any ASR system.
The output generated by the system is standard text, which is readily compatible with common digital formats and tools for storage and subsequent searching or analysis. This facilitates managing large volumes of transcribed lecture content, allowing students to efficiently revisit specific topics or phrases within recorded materials.
The architecture, often involving processing audio in segments, lends itself to handling lengthy audio inputs, making it viable for transcribing full-length academic lectures or extended discussions without inherent limits on duration. Processing very large files efficiently still depends on computational power and optimization, presenting scaling considerations for batch processing extensive archives.
For AI systems trained on large datasets, mechanisms for iterative improvement are typical, sometimes incorporating forms of user feedback to refine model performance. While the specifics of such loops for public Whisper deployments can vary, the potential exists for the system to gradually enhance its accuracy, particularly in handling challenging linguistic aspects, though user-provided corrections don't automatically retrain the base public models.
More Posts from aitranslations.io: