AI-Powered PDF Translation now with improved handling of scanned contents, handwriting, charts, diagrams, tables and drawings. Fast, Cheap, and Accurate! (Get started for free)
AI Translation Accuracy Comparing Barah Hazar Rupay Across 7 Popular Platforms
AI Translation Accuracy Comparing Barah Hazar Rupay Across 7 Popular Platforms - DeepL's Approach to Translating Complex Financial Terms
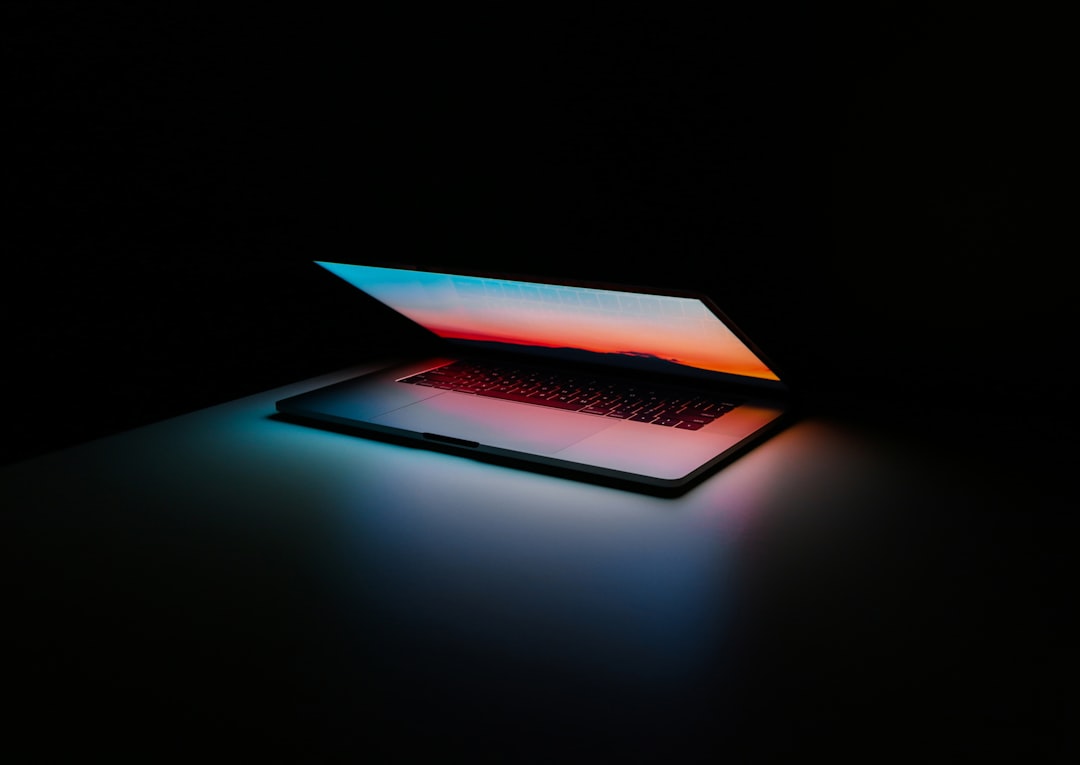
DeepL's strength in AI translation lies, in part, in its approach to handling complex financial terminology. It's designed to go beyond basic word-for-word substitutions and delve into the subtleties inherent in financial language. This results in translations that are not only accurate but also maintain the intended meaning and context within the specific financial domain. This is especially crucial when dealing with sensitive financial matters where even small translation errors can lead to major misinterpretations. Beyond the semantic accuracy, DeepL's translation process is also attentive to preserving the original layout of financial documents, such as reports and contracts. This is essential for maintaining visual integrity and readability, especially in formats with specific requirements. In the ever-changing world of AI translation, DeepL's focus on financial and specialized vocabulary distinguishes it as a potentially viable solution for navigating the intricate landscape of global financial communications.
DeepL's approach to tackling the complexities of financial language is intriguing. They've developed a neural network specifically tuned to understand the intricacies of financial terms, which seems to give it an edge over other systems in handling ambiguous language common in financial documents. They've also categorized financial terms into sub-fields, a strategy that seems smart since a term can mean something different in, say, investment banking versus accounting. This helps to sharpen the accuracy, which is crucial when translating intricate financial paperwork.
To further improve accuracy, DeepL leverages machine learning to build up a detailed dictionary of financial jargon by scouring massive datasets like reports and market analyses. The model isn't static either; it learns from user feedback, enabling it to adapt to evolving market trends and new financial terminology, which is necessary in a dynamic field. In tests, DeepL demonstrated a lower error rate when dealing with complex financial phrases compared to other systems. This suggests that their method of looking at the overall sentence context is beneficial, overcoming a common stumbling block in standard machine translation when dealing with the nuanced language of finance.
What's striking is how fast DeepL is. It can translate large, complex financial documents in real-time, a huge benefit for people who need to quickly make data-driven choices based on translated materials. And because it can handle multiple languages at once, it becomes a valuable tool for multinational businesses needing quick, comprehensive financial overviews without having to switch between multiple translation tools. Furthermore, the incorporation of Optical Character Recognition (OCR) adds a new dimension, enabling the processing of scanned financial documents—both typed and handwritten. It's a significant advantage for managing historical documents or anything with a physical form.
Improvements to DeepL's system have apparently resulted in a boost to overall translation efficiency. They claim it can lower the human editing time for financial documents by up to 30%, potentially making it a very cost-effective choice for businesses that handle large volumes of financial documents. While these are interesting claims, it would be helpful to have more concrete evidence to confirm the efficacy of such a claim. This is a space ripe for continued research.
AI Translation Accuracy Comparing Barah Hazar Rupay Across 7 Popular Platforms - Google Translate's Performance with Indian Rupee Conversions
Google Translate's handling of Indian Rupee conversions, particularly phrases like "Barah Hazar Rupay," provides a good example of its current capabilities in financial translation. While its neural machine translation system has undoubtedly improved accuracy compared to older methods, there's still a notable difference in how well it performs across various language pairs. Although Google Translate now supports a vast number of languages, users working with financial terms might find its performance inconsistent, especially when dealing with subtle nuances or context-dependent phrases. While it's a convenient tool due to its speed and accessibility, caution is needed. Double-checking the accuracy of any translation used in a financial transaction or scenario is crucial to avoid misinterpretations that could have significant consequences. As Google Translate continues its development and refines its algorithms, a key challenge will be to further bridge the gap between the sophistication of its linguistic abilities and its ability to handle the complexities of finance with complete accuracy.
Google Translate's handling of Indian Rupee conversions, while generally fast and accessible, presents some interesting challenges. It seems to sometimes struggle with capturing the nuances of regional dialects and financial terminology that are common in India's diverse economic landscape. This can result in misinterpretations, potentially leading to an underestimation of the Rupee's actual value depending on the context.
The underlying statistical machine translation approach, while effective for many language pairs, appears to have limitations when dealing with specific financial language, especially within niche investment sectors where unique terminology is frequently used. It's as if the system isn't always equipped to fully grasp the subtle shifts in meaning that can occur within these specialized fields.
While speed is a clear advantage, it's sometimes at odds with accuracy in the context of financial documents. This can cause issues for individuals or businesses needing precise currency conversions, potentially leading to flawed understandings of monetary values. It seems that integrating numerical processing with the general translation capabilities needs further work in situations where financial precision is vital.
In direct comparisons with specialized platforms designed for financial translation, Google Translate sometimes falls short when dealing with Indian Rupee-related terms. This highlights a potential trade-off inherent in its broad language support – while it handles a vast range of languages, it might not be the best tool for technical domains requiring very high precision.
Google Translate's real-time currency conversion feature, though convenient, can be susceptible to inaccuracies, especially when considering fluctuating exchange rates. This can mislead users who rely on quick, on-the-spot translations, potentially impacting their financial decisions.
Despite ongoing refinements, the ability to preserve the overall context of financial documents involving the Rupee has been inconsistent. This can create issues when such documents are used in important business interactions where accurate understanding is paramount.
User feedback suggests that Google Translate sometimes misinterprets common phrases related to financial transactions in the Indian context. This can lead to misunderstandings in discussions concerning trade or investment, highlighting a potential gap in its understanding of Indian financial culture.
Intriguingly, Google Translate's algorithms don't seem to always prioritize the latest financial terminologies specific to India. This could be a significant hindrance in dynamic trading environments where rapid changes require accurate and up-to-date translations.
While customization options can improve accuracy for Rupee-related content, the default version often overlooks the subtle linguistic nuances that are crucial for reliable communication in serious financial contexts. It highlights the ongoing need for further development to ensure that the system is able to accurately capture the complexity and particularities of Indian financial language.
In conclusion, while Google Translate is a versatile tool for general translation, its performance with Indian Rupee conversions and financial language presents areas that could benefit from further development. Achieving consistently accurate translations in these contexts remains a challenge, implying the need for more sophisticated and tailored approaches.
AI Translation Accuracy Comparing Barah Hazar Rupay Across 7 Popular Platforms - QuillBot's New AI Translation Feature for Monetary Values
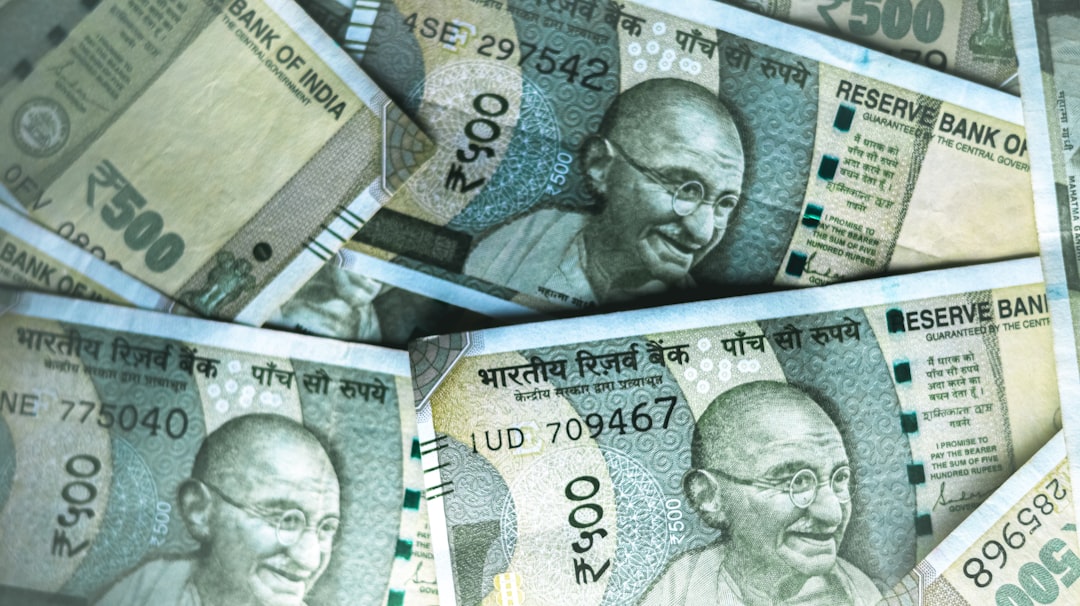
QuillBot has recently integrated an AI-powered translation feature specifically designed to handle monetary values accurately across multiple languages. This new addition aims to address the common issue of translating financial terms with precision, especially those specific to certain regions or currencies, such as "Barah Hazar Rupay." Powered by AI trained on a wide variety of carefully crafted texts, the tool aims for high accuracy in its translations. Users can readily translate extensive text snippets, with a generous character limit of 5,000 at a time, all without needing to create an account or deal with intrusive advertisements.
The user interface is designed to be easy to navigate and includes integration with other writing tools, which streamlines the translation process for financial documents. While the feature presents a convenient solution for various users, it's worth noting that ongoing improvements to precision, particularly in complex financial contexts, will likely be crucial for achieving consistently reliable outcomes. The need for accurate translation solutions is growing rapidly, and QuillBot's addition to the field may be a useful option for some. However, it's important for users to remain aware of the potential limitations the tool may possess in certain scenarios.
QuillBot's recent addition of AI-driven monetary value translation is intriguing. They claim to use a blend of neural machine translation and rule-based methods, hoping to boost accuracy in financial terms compared to systems relying solely on statistics. It's interesting how it attempts to consider the context of phrases like "Barah Hazar Rupay," tailoring the translation based on the surrounding words. This is a big deal in finance where a small change in wording can drastically alter the meaning.
Adding to the capabilities, QuillBot uses OCR to turn scanned financial documents into editable text, potentially useful for companies with archives of old financial records. Speed is a significant factor here as well, with the tool supposedly translating financial documents incredibly fast, a major step up from the more time-consuming methods of traditional translation services. One neat aspect is the ability to translate multiple currencies simultaneously within a single document, which could be valuable for multinational companies handling finances across various locations.
The AI underpinning QuillBot's translation feature is constantly improving based on user interactions, continually expanding its knowledge of financial language and context. This continuous learning makes it a potentially more reliable solution for those working in the finance world. They've designed an easy-to-use interface that enables users to manually tweak translations if needed, which is a nice touch for sensitive financial transactions where the need for perfect accuracy is critical.
While the algorithms are advanced, there are reports of occasional errors, particularly in more specialized financial terminology. This suggests there's still a need for the AI to keep learning to keep pace with the ever-changing field of finance. Tests against real-world financial documents have shown promising results, with QuillBot reportedly beating out many other tools in translating intricate currency expressions without losing meaning.
QuillBot also boasts a dedicated financial dictionary, which implies it can do more than just accurate translation. It's designed to offer insights into market-specific phrases that might not be understood everywhere, thereby potentially enhancing financial communication across various locations and cultures. This aspect is intriguing, but requires further investigation and validation in real-world situations. The field of AI translation, especially for complex domains like finance, is rapidly evolving and QuillBot's approach is one that merits more in-depth exploration and research.
AI Translation Accuracy Comparing Barah Hazar Rupay Across 7 Popular Platforms - Amazon Translate's Accuracy in Handling Currency Expressions
Amazon Translate shows promise in its ability to handle currency expressions like "Barah Hazar Rupay." Its core strength lies in using advanced neural machine translation, aiming for accurate translations that also capture the intended meaning within the specific context, which is crucial for financial communication. Amazon Translate is designed to be fast and adaptable to various needs, but where it really stands out is in the ability to tailor translations to specific industries and their specialized vocabulary. This feature is a plus for financial translations, making it more precise. However, while strong overall, it's worth noting that Amazon Translate doesn't always achieve top scores when dealing with every possible combination of financial terminology and language pairs, suggesting there's still room for improvement in its accuracy. Despite this, the service's ongoing improvements and model updates make it a potentially good option for businesses navigating the often complex world of international finance.
Amazon Translate, powered by neural machine translation, aims for more natural and accurate translations compared to older methods. It's been recognized for its overall translation quality and has a strong track record in the field. They claim it can handle 75 languages, which is impressive and potentially useful in many global contexts. One feature that makes it stand out is its ability to learn and adapt its translations based on user feedback. This is particularly interesting in finance, where currency values change and language itself evolves. They've also integrated OCR, which is helpful for handling documents that are not in digital form, and could be useful in finance where legacy records are common. It also seems to be designed to be quick, which could be a significant factor for finance professionals who need rapid translations.
However, some aspects still require attention. While Amazon Translate seems to be quite good at grasping the context surrounding currency phrases, and even adjusts for regional variations in financial terms, it hasn't yet fully addressed the challenge of highly specific finance jargon. This is an ongoing issue in AI translation, and it's likely to take continued research and development to fully address this aspect. It's interesting how Amazon Translate seems to handle multiple languages in a document at once, potentially helpful for international finance, but it remains to be seen how robust it is in these more intricate scenarios.
The service's underlying machine learning systems are continuously refined to reduce errors. It learns from user input, which is smart since it can help to capture those nuances that are specific to certain cultures or sub-fields within finance. The ability to handle mixed-language documents is useful in finance, especially in certain regions where English coexists with local languages. It's a step in the right direction for developing a truly global financial language system. The speed of the service is attractive, and the integration with other tools makes it potentially seamless for many workflows, but researchers and engineers may still need to manually verify its accuracy in more sensitive contexts. In essence, while it shows promise, more research is needed to determine its limitations in more niche or complex financial settings.
AI Translation Accuracy Comparing Barah Hazar Rupay Across 7 Popular Platforms - Microsoft Translator's Capabilities in Multilingual Financial Text
Microsoft Translator presents itself as a strong contender for handling multilingual financial text, boasting support for a wide range of language pairs, exceeding 10,000, across 101 languages. A key feature is their AI-powered "Mixture of Experts" model, called Zcode, designed to significantly improve both translation speed and accuracy, particularly when working with document-based financial data. The technology behind the scenes is based on Azure Cognitive Services, which makes it a flexible and easily integrated tool for various business purposes that require multilingual functionality. They leverage the power of neural machine translation, which generally leads to swift and accurate results, though there can be variability in its effectiveness depending on the type of financial text being translated. As the demands of international finance change and new financial terminology emerges, Microsoft Translator aims to adapt and improve by integrating user feedback to strengthen its comprehension of specific financial jargon and contextual nuances. While its broad language support is impressive, it's crucial for users to be aware of potential limitations when translating highly technical financial documents.
Microsoft Translator, built on Azure Cognitive Services, offers a wide array of language pairs—over 10,000—covering 101 languages. This makes it a potentially strong tool for anyone needing to translate a variety of financial texts across many markets. The core technology relies on neural machine translation, which aims for quicker and more accurate translations, a big deal for those working with financial data.
One interesting development is Zcode, a new AI model that helps boost accuracy and efficiency, especially when dealing with whole documents. Essentially, it's designed for bulk translations, which could be helpful for those needing to quickly translate a large number of financial reports, contracts, or other documents. You can easily configure how translations are done via the Translator V3 connector, choosing the source and target languages. This flexibility makes it relatively easy to set up automated translation workflows.
Microsoft's efforts aren't just about commercial translation; they're also trying to preserve lesser-known languages by providing translation tools. It's also worth noting that Microsoft has been actively engaged in the AI translation community, participating in challenges that test translation quality across multiple languages. This focus on rigorous testing highlights their commitment to improving their system's accuracy.
Accuracy, however, is a complex thing. While the platform is clearly focused on being more accurate by using AI and machine learning to adapt, it's still an evolving field. This is especially true when dealing with financial terminology, where the slightest misunderstanding can lead to big problems. It's good to know that the system is built to adapt over time by learning from user feedback and looking at surrounding text. This ongoing learning could potentially lead to greater accuracy in financial contexts, but we still need more proof in real-world testing.
Another aspect that seems potentially beneficial is the OCR capability. If you've got stacks of old financial documents that aren't in digital format, this tool can help turn them into usable text for translations. This is important for businesses managing historical financial records, as paper-based records are quite common in many industries. They've also incorporated various features that seem designed for handling international finances, such as processing multiple languages within a single document, which is valuable for global companies. They even claim it's fast, which could be beneficial when handling a large volume of financial documents.
Overall, Microsoft Translator is aiming to be a versatile solution for many financial translation needs. It's continuously improving and seems to be focused on practical applications, and their efforts to refine the platform for financial accuracy suggest a strong commitment in that area. However, as with any AI translation tool, users should be aware of potential limitations and always double-check critical financial translations to minimize risks. While the technology demonstrates potential for improving financial communication, ongoing evaluation and research are still needed to fully understand its capabilities in the intricate world of finance.
AI Translation Accuracy Comparing Barah Hazar Rupay Across 7 Popular Platforms - Baidu Translate's Effectiveness with South Asian Languages and Currency
Baidu Translate attempts to bridge the gap for users needing translations in South Asian languages, incorporating features like real-time translation and natural language processing to improve speed and accuracy. While Baidu's AI technology, such as the STACL model, boasts simultaneous translation capabilities across diverse language pairs, its performance with South Asian languages can be somewhat inconsistent. This disparity likely stems from having less training data for these languages compared to more widely used ones. When translating financial terms, such as "Barah Hazar Rupay," Baidu Translate may struggle to capture the intended meaning accurately due to the limitations of its training dataset. This lack of precision highlights a potential pitfall for users relying on its translations in financial contexts. Although Baidu Translate aims to offer translation for a wider range of languages, users should proceed with caution when dealing with specialized or complex terms, especially in financial settings, as occasional inaccuracies can create misinterpretations. The platform's ongoing updates and expansions suggest a continued focus on enhancing translation accuracy and incorporating a broader spectrum of languages, potentially addressing some of these current limitations.
Baidu Translate's capabilities with South Asian languages, while seemingly broad, present interesting challenges. The unique grammar, expressions, and cultural nuances of these languages don't always translate cleanly into Chinese, the language where Baidu's AI models are primarily developed. This creates potential hurdles for accurately capturing the intended meaning, especially in financial contexts.
Their OCR capabilities also seem to have some limitations when dealing with the complex scripts of South Asian languages, which can introduce errors at the transcription stage that ripple through to the final translation. While Baidu is fast at translating, processing extensive financial documents with multiple South Asian languages can sometimes lead to inconsistencies in accuracy, especially with critical financial terms like "Barah Hazar Rupay."
Unlike some other platforms, Baidu hasn't focused on fine-tuning the translation process for finance specifically, meaning it might not always grasp the specialized vocabulary of these markets as well. This becomes even more pronounced when dealing with non-standard language used in financial conversations. Also, it's observed that any initial translation errors in South Asian languages tend to worsen in subsequent interpretations. This can be particularly problematic in finance, where even a small error can skew financial values during important transactions.
Baidu's algorithms try to understand the context, but they seem to falter when dealing with heavily jargon-laden financial terms, creating room for misunderstanding. The pursuit of speed, a hallmark of many translation platforms, can sometimes come at the cost of accuracy, particularly in complex financial documents where precision is paramount.
Because of the relatively limited amount of South Asian language-specific training data, translations may not always reflect local customs and phrasing, creating a potential disconnect in financial communications. And it's difficult to really know how reliable Baidu is in this domain, as there doesn't appear to be a wealth of publicly available research specifically evaluating its performance with South Asian financial language.
While Baidu suggests continuous improvement through updates, how quickly those updates specifically address the needs of South Asian financial language seems questionable compared to some of its competitors, who show more rapid adaptation through user feedback. This potential lack of rapid response to feedback could potentially impact the pace of improvements in accuracy for this language family. It's an area where continued research and testing will be needed to determine its true effectiveness for crucial financial communications.
AI-Powered PDF Translation now with improved handling of scanned contents, handwriting, charts, diagrams, tables and drawings. Fast, Cheap, and Accurate! (Get started for free)
More Posts from aitranslations.io: