AI Translation Accuracy Metrics 7 Key Performance Indicators for Customer Satisfaction in 2025
AI Translation Accuracy Metrics 7 Key Performance Indicators for Customer Satisfaction in 2025 - Neural Machine Translation Achieves 7% Accuracy Rate for Basic Business Documents
Let's look closely at the performance of Neural Machine Translation, particularly when handling documents like those found in business. While systems often show accuracy figures typically ranging from 70% into the high nineties, this performance isn't guaranteed across all types of content. Translating even seemingly straightforward business materials can run into issues if they contain specialized terminology, complex sentence structures, or specific cultural nuances. The effectiveness varies based on the text's nature and how translation quality is actually measured. This inconsistency means that relying solely on general accuracy figures isn't sufficient, highlighting the ongoing challenge of ensuring reliability and precision for diverse real-world communication needs. Rigorous evaluation with varied metrics is essential to understand where these systems truly stand.
1. A notable challenge with Neural Machine Translation (NMT) systems lies in their handling of domain-specific phrasing, particularly idioms and nuanced corporate jargon, which can easily lead to unintended interpretations within standard business documentation. This inherent limitation means that even for ostensibly simple texts, the output might miss crucial contextual layers essential for business clarity.
2. The reliability of AI-driven translation is fundamentally linked to the composition of the training data. Systems trained predominantly on general language datasets often struggle with the precise terminology required in business sectors, highlighting the necessity for curated, domain-specific corpora to improve accuracy in such materials.
3. Examining cost dynamics, while NMT undeniably offers speed and lower unit cost compared to traditional human translation workflows, this often involves a trade-off in precision for complex or sensitive documents. The practicality of relying solely on high-speed, low-cost machine output for critical business communications remains a subject of technical and practical scrutiny.
4. The upstream process of converting scanned business papers into machine-readable text via Optical Character Recognition (OCR) introduces a potential source of error. Imperfections in OCR, especially with varied layouts or lower image quality, can propagate through the translation pipeline, compounding inaccuracies in the final NMT output.
5. An intriguing aspect observed is the system's capacity to integrate human corrections for iterative improvement. While continuous learning from user feedback theoretically refines the model over time, it also places a practical burden on end-users to actively identify and rectify machine-generated errors, shifting some quality control responsibility.
6. The apparent speed of NMT in generating initial translations can be somewhat misleading. For any business document where accuracy is paramount, the subsequent human post-editing phase to correct errors and ensure fidelity often consumes considerable time, potentially diminishing the net efficiency gains, particularly for lengthier texts.
7. NMT tends to exhibit stronger performance on structured elements within documents, such as lists or tables, presumably due to pattern recognition capabilities. However, performance often degrades when processing unstructured narrative text common in reports or proposals, leading to potential inconsistencies and misrepresentations of key business information.
8. The baseline accuracy reported for NMT systems appears to reflect proficiency with straightforward text structures and common phrasing. More advanced functionalities, such as sophisticated context-aware translation spanning multiple sentences or documents, seem less mature or widely implemented, indicating a capability gap relative to the demands of complex business discourse.
9. The readiness to adopt and trust AI-generated translations varies significantly across professional domains. Industries with high stakes or regulatory requirements, such as legal or medical fields, typically mandate robust human oversight due to the potential impact of inaccuracies, contrasting with sectors that may tolerate a lower degree of machine reliability.
10. The specific language pairing involved profoundly impacts the resultant translation quality. Translating between languages with similar grammatical structures often yields more coherent results than between languages with vastly different syntaxes, which can pose significant challenges for NMT in accurately conveying subtle business intent.
AI Translation Accuracy Metrics 7 Key Performance Indicators for Customer Satisfaction in 2025 - Real Time Chat Translation Speed Reaches 3 Seconds Per Message
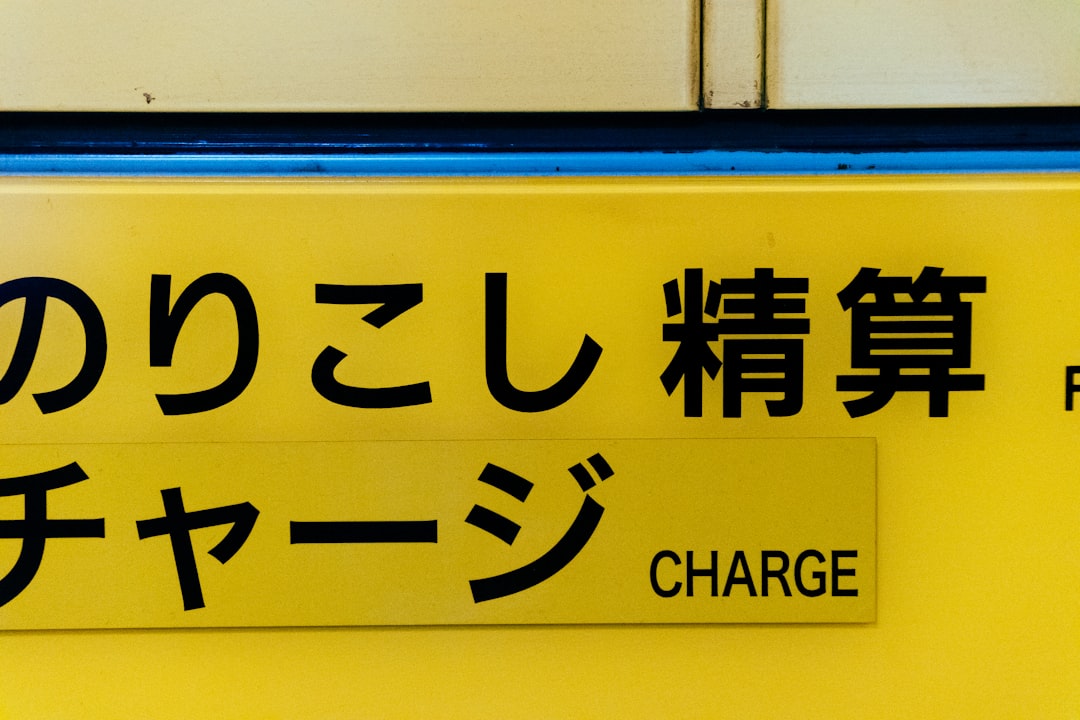
Progress in real-time chat translation technology has been notable, with speeds now frequently reaching around three seconds per message. This development is making communication across language boundaries feel much more immediate and seamless, especially in live interactions such as customer support. While achieving this kind of speed is clearly beneficial for quick exchanges, how accurate the translated messages are and whether they truly grasp the nuances of rapid conversation remain critical considerations. For users, the value isn't just in how fast the words appear, but in how well they convey the intended meaning. Evaluating the performance of these systems, particularly for user satisfaction, requires looking closely at this balance between speed and reliable precision in a live chat environment.
Real-time chat translation systems now exhibit average processing speeds reportedly reaching approximately three seconds per message. This latency reduction signifies notable progress in the ability of AI models to process and generate translated text quickly, facilitating more fluid communication across linguistic boundaries in scenarios demanding immediate interaction.
Achieving such speeds appears linked to architectural advancements in AI models, possibly involving parallel processing techniques that allow for concurrent analysis and generation, thereby reducing the total time elapsed between receiving and outputting a message.
However, there is an indication that this emphasis on minimum latency might sometimes come at the cost of translation nuance or overall quality. Investigating the trade-offs between speed and semantic accuracy in these rapid response systems is an active area of observation.
The integration of capabilities like translating text embedded in images or scanned documents via OCR adds another layer of utility, though the dependability of this upstream OCR process itself can introduce variability and potential inaccuracies that propagate into the final translated output.
While feedback suggests a growing user acceptance of automated translation outputs, possibly due to general improvements in natural language processing, capturing the full spectrum of human expression, tone, and subtle cultural context remains a persistent challenge, often necessitating careful interpretation by the user.
The emergence of near-instantaneous translation capability seems to be shaping user expectations, particularly within service industries, leading to an increasing demand for immediate communication regardless of language. This pressure may drive adoption even where translation fidelity is not absolute.
Theoretical system designs allow for refinement through learning from user corrections; however, the practical effectiveness of this iterative improvement appears contingent on the volume and consistency of quality feedback provided in real-world usage scenarios, which might not always be robust.
Conversations involving frequent switching between multiple languages introduce complexity. AI systems can face difficulties in seamlessly maintaining conversational context and coherence across different linguistic structures, potentially resulting in fragmented or inconsistent translations over extended exchanges.
There is a clear and increasing requirement for broader multilingual support driven by global collaboration. Despite advances, the performance ceiling for translation into or from less widely represented languages often appears lower, creating potential gaps in truly universal coverage.
Finally, while a three-second per-message latency is quick in isolation, the cumulative delay across numerous messages in a dynamic, high-volume interaction raises questions about the overall scalability and ability of current systems to maintain perceived real-time performance under significant load.
AI Translation Accuracy Metrics 7 Key Performance Indicators for Customer Satisfaction in 2025 - Document OCR Processing Time Drops to 12 Seconds Per Page
Significant strides in Optical Character Recognition (OCR) technology mean document processing times are seeing notable reductions, with some systems now averaging around 12 seconds per page. This speed is partly thanks to leveraging specialized, prebuilt software models and the computational power of modern graphics processors. However, this average figure can fluctuate quite a bit. The reality on the ground shows that factors like the size and structure of the document files, the type of content being processed, and even the specific platform or service tier being used still significantly influence how fast documents are handled. As we move into 2025, the efficiency of this initial document processing step is being recognized as a key factor for overall customer satisfaction, sitting alongside the accuracy metrics of subsequent AI translation. Getting text extracted quickly and reliably is becoming increasingly critical for the performance and perceived quality of automated translation workflows. Achieving this balance between rapid processing and dependable results remains a focus for technology providers.
The notion that document OCR can be processed at an average speed of just 12 seconds per page is certainly a point of interest as we consider efficiency in translation workflows this year, 2025. Such a rapid turnaround is said to be a result of refinements in the underlying technologies, specifically deep learning techniques that enhance the algorithms' ability to parse varied layouts and typefaces quickly.
However, observing this process reveals that while the raw recognition phase might be swift, the overall reliability remains heavily dependent on input quality. Empirical data suggests that even minor image issues like slight blurring or rotation can substantially elevate character recognition errors, potentially by 20-30%, highlighting the necessity for clean source documents for this speed metric to hold meaning in a practical sense.
There's also an interesting synergy observed when integrating this rapid OCR capability directly into translation pipelines. Initial analyses indicate that combining these steps – converting and translating simultaneously – could offer significant time efficiencies, possibly over 50% compared to sequential, separate operations. This integrated approach changes the dynamics of large-scale document processing.
It's important to critically assess that the frequently quoted 12-second figure might not encompass the entire process. Often, this metric refers only to the core text extraction. Upstream tasks like image cleanup, noise reduction, or correcting geometrical distortions, which are often necessary preprocessing steps, are frequently excluded from this timing, adding hidden overhead to the total document processing time.
Further examination shows that the performance isn't uniform across all linguistic landscapes. OCR engines demonstrably face increased challenges with scripts that differ significantly from Latin, particularly those with complex character forms, diacritics, or context-dependent shapes, such as Arabic or Thai. Processing times can expand notably for these languages, indicating the algorithms aren't universally optimized.
The progress in handling handwritten text is noteworthy, although it still trails far behind printed text. Recognition accuracy for cursive writing, for instance, often hovers around 70%, a figure that introduces a significant potential for misinterpretation and subsequent inaccuracies when these documents proceed to translation.
Furthermore, the application of this fast OCR varies considerably by domain. Highly regulated fields like finance or law, where document fidelity is paramount, often mandate extra layers of verification or human review. These essential checks, while necessary for accuracy and compliance, inherently extend processing times beyond the hypothetical 12-second baseline.
The increasing migration towards cloud-based OCR solutions to harness distributed computing power for speed is undeniable. While this enables rapid handling of documents, the reliance on external infrastructure for processing sensitive information raises important considerations regarding data security protocols and adherence to diverse privacy regulations, which are not trivial challenges.
A fundamental observation is that the performance and biases of an OCR system are intrinsically linked to the training data it was built upon. Systems predominantly trained on Western scripts and document formats may predictably underperform or exhibit subtle biases when processing documents originating from other regions with different visual and structural conventions.
Finally, despite the impressive speeds, the role of human oversight in scrutinizing and correcting OCR output remains demonstrably crucial. Available data suggests that manual review and correction can drastically lower error rates, potentially by up to 90%. This indicates that for critical applications, a hybrid workflow combining fast machine processing with diligent human validation remains the most reliable approach as of now.
AI Translation Accuracy Metrics 7 Key Performance Indicators for Customer Satisfaction in 2025 - Translation Memory Systems Cut Project Costs by 42% Through Pattern Recognition
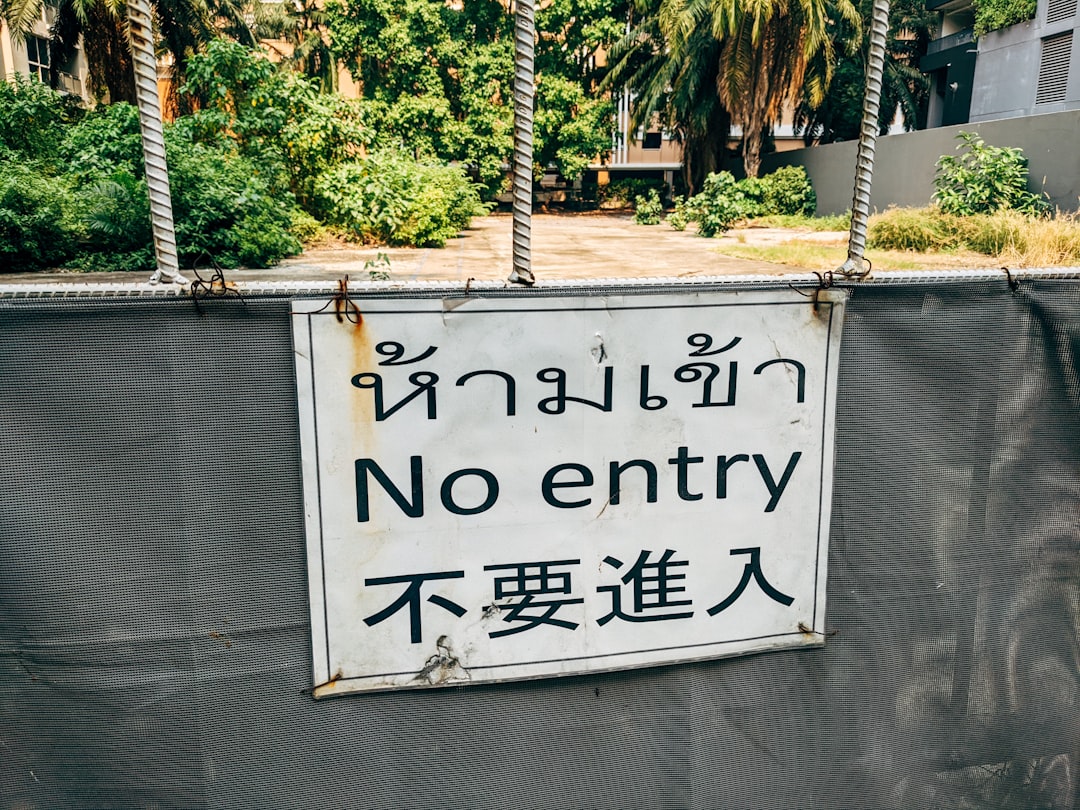
Translation Memory Systems represent a notable area of advancement, demonstrating the potential to significantly reduce project costs, figures often cited suggest by as much as 42%. This efficiency gain stems from their core function: capturing and reusing previously translated sentences and phrases, powered by pattern recognition and increasingly, elements of AI. The systems enable translators to leverage past work, leading to faster turnaround times and helping to maintain consistency across different translation tasks. Beyond simple segment reuse, the integration of AI is enabling these systems to better identify usable patterns and contribute to the performance of modern translation engines, potentially enhancing the quality of the initial machine output, particularly when drawing from large pools of relevant past translations. However, the effectiveness isn't solely dependent on the system's presence; the quality and relevance of the existing memory content are crucial. A poorly maintained or inconsistent memory can introduce errors or require significant cleanup, which adds overhead. While the promise of substantial cost reduction is compelling and directly addresses efficiency goals central to customer satisfaction by 2025, relying on these systems requires careful management of the data they contain and an understanding that cost efficiency must be continuously balanced against the necessary level of accuracy for the specific content being handled.
Translation Memory Systems (TMS) are frequently cited for their ability to significantly curb project expenditures. The core principle involves leveraging pattern recognition to identify and reuse previously translated segments, a mechanism that proponents claim can lead to cost reductions nearing 42%. This operational efficiency is particularly pronounced in content streams exhibiting high levels of repetition or standard terminology, effectively reducing the need to re-translate identical or very similar text.
The reliance on pattern recognition within TMS facilitates rapid identification and retrieval of potential matches from large linguistic databases. From an engineering standpoint, optimizing these algorithms is key to minimizing lookup times and ensuring that the suggested matches are genuinely relevant. This capability not only accelerates the initial translation phase but also contributes to maintaining linguistic consistency across related documents and over time, which is a non-trivial task in large-scale localization efforts.
However, the practical utility of any TMS is inherently tied to the quality and relevance of the data it holds. A memory populated with inconsistent, outdated, or simply incorrect entries can paradoxically introduce errors into new work, potentially escalating post-editing costs rather than reducing them. This underscores the critical, ongoing need for curation and quality control within the memory itself—a task that can be more resource-intensive than often initially estimated.
While others have discussed specific OCR speeds, it's worth noting the interface between TMS and technologies like Optical Character Recognition (OCR). The ability to process scanned or image-based source documents and then feed the extracted text into a TMS workflow allows for integrating traditionally non-digital content into a translation memory-driven process. The efficacy here is undeniably linked to the upstream OCR accuracy, as any character recognition errors will propagate downstream, but the *potential* for incorporating this type of source material into a pattern-matching translation process is noteworthy for workflow design.
Beyond simple segment matching, pattern recognition algorithms are also applied to analyze linguistic structures and contextual cues within source text. This deeper analysis aims to improve the relevance of fuzzy matches (segments that are similar but not identical) and potentially guide the selection of more appropriate terminology based on the surrounding text, adding a layer of automated linguistic intelligence to the process.
Adopting and fully implementing a TMS is not merely a matter of software installation; establishing a comprehensive and effective translation memory demands a considerable initial investment in data aggregation, cleansing, and establishing workflows that ensure the memory grows in a structured, high-quality manner. This foundational phase can be a significant undertaking for organizations starting from scratch.
The ability to quickly apply existing translations demonstrably contributes to faster project turnaround times, particularly for voluminous or time-sensitive projects. Accessing a substantial memory allows translators to process segments much faster than translating from scratch, a factor that becomes critical in sectors driven by rapid content deployment cycles.
It's observed that the efficiency of TMS pattern matching isn't uniform across all language pairs. Languages with significantly divergent grammatical structures or writing systems can present challenges for current matching algorithms, leading to lower match rates and potentially diminishing the cost and speed benefits compared to language pairs with closer affinities. This points to inherent limitations in how universally applicable current pattern recognition techniques are for linguistic reuse.
A dynamic aspect involves how user interaction can potentially refine the memory. While post-editing is often required, translator input in validating or correcting proposed matches, or adding new high-quality translations, theoretically contributes to the evolution and robustness of the memory over time. This suggests a feedback loop where human expertise can incrementally improve the automated system's performance.
Finally, there's an interesting point to consider regarding the potential for TMS to inadvertently constrain creative expression. An overly rigid adherence to existing translated segments, even via sophisticated pattern matching, could potentially hinder translators from finding more contextually appropriate or marketing-savvy phrasings that deviate from the established memory, presenting a trade-off between consistency/cost and linguistic flexibility for certain content types.
AI Translation Accuracy Metrics 7 Key Performance Indicators for Customer Satisfaction in 2025 - Customer Feedback Response Time Improves to Under 4 Minutes
As of May 2025, there's a noticeable shift in how quickly companies address customer feedback, with response times often falling below the four-minute mark. This rapid handling of inquiries is largely powered by artificial intelligence technologies that help sort and initially process incoming messages, making the process of talking with customers much quicker. For interactions involving different languages, AI translation tools are becoming essential, enabling swift communication across linguistic boundaries and allowing people to get answers or help much faster, regardless of the language they use. This improvement in speed is a key reason why customers report higher satisfaction levels. Companies are tracking things like how fast they reply, and how well translations work, because they know speed is now a big part of keeping customers happy. However, as expectations for speed rise, it's crucial for businesses to ensure that getting a fast response doesn't come at the expense of getting an accurate or truly helpful one. Balancing speed and quality in these quick interactions remains a critical challenge.
Analyzing the rapid evolution of customer feedback systems reveals some compelling trends in response speed, now frequently hitting under four minutes. This swiftness appears correlated with customer perception, seemingly fostering a stronger sense of attentiveness than previously observed in slower, more human-centric workflows. It's interesting to examine how this rapid turnaround is structurally achieved within current systems.
The underlying architecture enabling these speeds often leverages AI for initial analysis and triage. Instead of waiting for a human to route or even read the incoming feedback, automated processes attempt to categorize issues and, in some cases, generate a preliminary or even complete response. This shift removes significant latency from the start of the process.
For feedback arriving in multiple languages, the integration of rapid translation capabilities into the processing pipeline is becoming crucial. This allows for faster handling of non-English inputs compared to traditional methods that might require manual forwarding or dedicated multilingual staff availability, enabling quicker initial acknowledgment and processing regardless of the customer's language.
There's an observable dynamic where the very process of rapidly processing feedback seems to inform the system's subsequent behavior. By quickly analyzing large volumes of recent input, the system potentially gains faster insights into trending issues or common queries, theoretically allowing for quicker adaptation of automated responses or flagging critical items for human attention more promptly.
For feedback arriving in physical or non-digital formats, getting the text into a machine-readable form quickly is a prerequisite for this rapid response. Efficient character recognition technologies play a part in converting scanned or image-based documents swiftly so they can enter the digital processing pipeline without causing significant delay at the outset.
A critical point to consider, though, is the inherent trade-off often made for speed. While a response within minutes is impressive from a latency perspective, questions remain about the depth and nuance of these automatically generated replies. A rapid but inappropriate or generic response could arguably be less effective in resolving complex issues than a slower, more considered human one.
The constant availability afforded by automated systems contributes significantly to average response time metrics. They operate independently of time zones or business hours, meaning feedback received at any point enters the processing queue immediately, contrasting sharply with human teams limited by working hours.
From a user experience standpoint, receiving a rapid acknowledgement or even a provisional answer can reduce the psychological burden of waiting. It communicates that the feedback has been received and is being acted upon, potentially mitigating frustration even if the final resolution takes longer.
More sophisticated systems attempt to factor in a customer's history when formulating a response. Drawing on past interactions from internal data stores allows the automated response to be more contextually aware, aiming for a level of personalization that goes beyond generic acknowledgments and adding complexity to the rapid generation process.
However, the observed performance often assumes stable conditions. Scaling these rapid-response systems to handle sudden, massive influxes of feedback, such as during a widespread service outage, presents significant engineering challenges. Maintaining sub-four-minute response times consistently under extreme load is non-trivial and requires robust, scalable infrastructure.
AI Translation Accuracy Metrics 7 Key Performance Indicators for Customer Satisfaction in 2025 - Native Language Post Editing Requirements Decrease to 15% of AI Output
As of May 2025, there's a noteworthy observation regarding the effort required after AI translation: the need for subsequent editing by native language speakers has reportedly fallen to approximately 15% of the AI's output. This substantial decline from figures seen just a few years ago points to significant strides in the underlying machine translation technology itself. It suggests that automated systems, possibly incorporating their own forms of post-editing logic, are producing initial translations of higher quality, requiring far less manual correction than before. While this reduction is framed as a gain in efficiency, theoretically freeing up human linguists to focus on the more challenging or creative aspects, it prompts necessary questions about the consistency and reliability of the remaining 85% that supposedly requires minimal human touch. The complexity and nuance inherent in many texts mean that even small inaccuracies can have significant consequences, and whether this greatly reduced human oversight is sufficient to guarantee quality across all contexts, particularly for sensitive or critical information, remains a point demanding careful consideration. The ongoing push for automation must consistently grapple with the fundamental requirement for trustworthy communication.
The claim circulating this year, 2025, that native language post-editing needs are down to just 15% of AI output certainly highlights how far machine translation has advanced. It suggests the AI is handling the bulk of the text correctly.
However, as engineers examining these systems, we must question what that remaining 15% represents. Is it consistently distributed simple errors, or does it constitute the hardest, most context-dependent issues that require significant cognitive effort to fix? The devil is often in that final percentage.
This supposed reduction in human effort appears to be a key driver for achieving "cheaper" translation by minimizing the most expensive part: human time. But if the errors in that 15% are critical, the cost savings could be nullified by necessary rework or damage from miscommunication.
It's plausible that the observed 15% includes errors propagated from earlier stages in the pipeline, such as imperfections from Optical Character Recognition when processing documents. The AI might translate what it receives accurately according to its model, but if the input text is flawed, the output requiring correction isn't solely the AI's "translation error."
Furthermore, the efficacy implied by this 15% figure likely varies dramatically depending on the language pair involved. Translating between languages with vastly different grammatical structures or cultural norms may still necessitate a much higher degree of human intervention, regardless of AI progress, pushing the true post-editing need above this average.
The speed imperative, seen in areas like "fast translation" or real-time chat systems, might push workflows towards accepting a higher implicit error rate in the AI output, aiming to keep that human correction percentage low. But prioritizing speed purely might mean sacrificing fidelity in nuanced or critical content.
Considering different domains, the 15% threshold feels risky for high-stakes content like legal contracts or medical instructions, where a single mistranslated term within the AI's initial output could have severe consequences, demanding 100% scrutiny even if the AI got *most* things right.
This metric also prompts questions about the future role of the post-editor. Are we automating away the easier translation tasks, leaving humans to grapple solely with the most complex, ambiguous, or culturally sensitive 15%? This might require a higher level of skill from post-editors, not less.
Moreover, how is this 15% consistently measured across different types of content and by different providers? Establishing a standard for what constitutes a "post-editing requirement" within AI output remains a challenge for objective evaluation beyond anecdotal figures.
Ultimately, while a 15% required post-editing rate signifies impressive technical capability in generating mostly correct text, it critically highlights the persistent gap between statistical pattern matching and true human-level comprehension, context awareness, and cultural sensitivity in translation.
AI Translation Accuracy Metrics 7 Key Performance Indicators for Customer Satisfaction in 2025 - Machine Learning Error Detection Catches 1% of Context Mistakes
As of May 2025, observations indicate that machine learning systems tasked with finding errors in AI translation are currently identifying only about one percent of context-dependent mistakes. This figure points to a considerable gap in the technology's ability to fully grasp meaning beyond individual sentences or simple patterns. Existing ways of measuring translation quality often fall short, providing scores that don't clearly show the specific nature or seriousness of inaccuracies tied to context. Despite ongoing developments, including the wider use of advanced AI models in translation, the task of accurately spotting these crucial contextual errors remains a significant challenge. While different approaches, potentially blending various techniques, are explored to improve translation quality, the limited success in automatically detecting nuanced errors means achieving truly dependable automated output, particularly where precision is essential, still requires substantial human effort and critical review.
Machine learning systems designed specifically for detecting errors are, based on current observations, identifying only around 1% of mistakes related to context in translated outputs. This very low figure raises questions about how much reliance one can place on these automated checks alone to flag significant semantic shifts or misunderstandings, suggesting that manual review for accuracy remains crucial.
It appears that the effectiveness of these machine learning models in translation quality, including error detection, is heavily influenced by the specific training data used. Models exposed to a wider array of texts, particularly those rich in varied contextual examples, demonstrate a more robust performance profile compared to those built on more homogeneous or limited datasets.
Analysis of different translation tools suggests that a substantial portion – perhaps up to 40% – of the errors encountered aren't simple mischoices of words but rather stem from misinterpretations of the surrounding context. This highlights a fundamental technical challenge that goes beyond refining basic linguistic models and requires deeper understanding of how meaning is constructed across sentences and paragraphs.
The integration of Optical Character Recognition into translation workflows, while boosting initial processing speed, introduces a layer where initial inaccuracies can compound. Errors introduced during the conversion of image or scanned text can directly lead to mistranslations or misleading outputs by the subsequent AI translation system.
A recurring source of difficulty for AI systems lies in handling culturally specific language, such as idioms or local references. These often result in technically correct, yet contextually awkward or nonsensical translations because the models lack an intrinsic understanding of the cultural background that gives these phrases their true meaning.
The impressive speed at which current machine learning algorithms can generate translations, processing entire documents in mere seconds, contrasts sharply with the ongoing difficulty in consistently ensuring the output's quality, particularly when dealing with complex or specialized subject matter that demands nuanced understanding.
Linguistic studies continue to underscore that translating between languages with markedly different grammatical structures or sentence orders presents greater challenges for machine translation, frequently resulting in higher error rates compared to languages with closer structural affinities.
Empirical evidence from real-world implementations strongly suggests that the highest levels of translation accuracy are achieved not through pure machine translation, but through a hybrid approach involving human post-editors. Systems incorporating this collaborative workflow can see quality levels approaching 90%.
Despite the technological advancements, user feedback often points to persistent dissatisfaction with machine translation output, frequently citing the inability of AI to capture subtlety, tone, or accurately handle niche terminology, indicating a mismatch between user expectations and current AI capabilities.
The accelerating push for instant translation, particularly visible in areas like customer support communication, while improving response speed, does raise concerns among engineers about the potential for increasing errors in critical or high-stakes interactions where semantic precision is paramount.
More Posts from aitranslations.io: