AI-Powered PDF Translation now with improved handling of scanned contents, handwriting, charts, diagrams, tables and drawings. Fast, Cheap, and Accurate! (Get started for free)
AI Translation Agents vs Traditional Chatbots 7 Key Differences in Language Processing Capabilities for 2024
AI Translation Agents vs Traditional Chatbots 7 Key Differences in Language Processing Capabilities for 2024 - Real Time OCR Capabilities Set AI Translation Agents Apart From Basic Text Recognition
The integration of real-time Optical Character Recognition (OCR) is a significant advancement that elevates AI translation agents beyond the limitations of basic text recognition. This capability allows these agents to process text directly from visual sources like photos and videos, instantly converting the content into a format suitable for translation. The speed and adaptability offered by real-time OCR are crucial for overcoming language barriers in a timely and efficient manner. Not only does it expedite the translation process, but it also allows for a more nuanced and context-aware interpretation of the text. This is critical for achieving more natural and accurate translations, fostering richer interactions that transcend linguistic differences. As our interconnected world demands faster and more reliable translation services, the importance of real-time OCR in facilitating cross-cultural communication will only continue to grow, potentially shaping the future of how we communicate and interact globally.
The ability of AI translation agents to incorporate real-time Optical Character Recognition (OCR) sets them apart from more rudimentary text recognition systems. This feature allows for incredibly fast text interpretation, potentially reaching speeds of up to 50 characters per second – a significant leap over traditional methods of transcribing handwritten text. This speed enhances the practicality of applications that require quick processing of visual information.
Furthermore, recent OCR advancements have expanded the number of supported languages to over 120. This unlocks new possibilities for global communication, pushing beyond the limits of simple translation tools. It's intriguing to see how AI agents, with this integrated OCR capability, can grasp the subtle nuances within text – something basic OCR often struggles with. This deeper understanding leads to more accurate translations, capturing the true intent of the written message.
The combination of AI translation and real-time OCR transcends the boundaries of translating just printed text. We can now envision translating handwritten notes and signs, broadening the utility of translation tools for various everyday scenarios. Some advanced OCR systems are employing machine learning to further refine recognition accuracy. By learning from user interactions, these systems adapt over time, getting better at handling specific fonts or handwriting styles. This ongoing learning aspect is quite promising for future improvements.
Interestingly, studies suggest that including visual context can improve translation accuracy. Real-time OCR enables AI agents to use images alongside text, providing a more holistic interpretation of the source material. The integration of real-time OCR into mobile devices is also proving valuable, fostering greater user engagement by facilitating instant translations. This caters to the growing need for quick communication in a world with increased global travel and remote collaboration.
While basic OCR processes text in isolation, AI translation agents can leverage computer vision to detect and engage with visual elements such as charts or graphs. This capability enhances the translation of complex information, going beyond just the written words. The management of multilingual environments is another area where real-time OCR shines. It can handle scenes containing text in various languages, delivering contextualized translations to users navigating diverse linguistic settings.
Finally, linking real-time OCR with voice recognition systems offers a seamless transition between spoken and written language translation. This is particularly helpful in environments demanding rapid and flexible communication, highlighting the potential of this integrated approach for various applications. However, further research is needed to see whether this integration lives up to its promise and indeed improves translation quality. It's clear, however, that the combination of AI translation agents and real-time OCR is significantly enhancing the possibilities for language processing and translation in the present day and holds considerable potential for the future.
AI Translation Agents vs Traditional Chatbots 7 Key Differences in Language Processing Capabilities for 2024 - Advanced Context Memory Helps AI Agents Track Extended Conversations
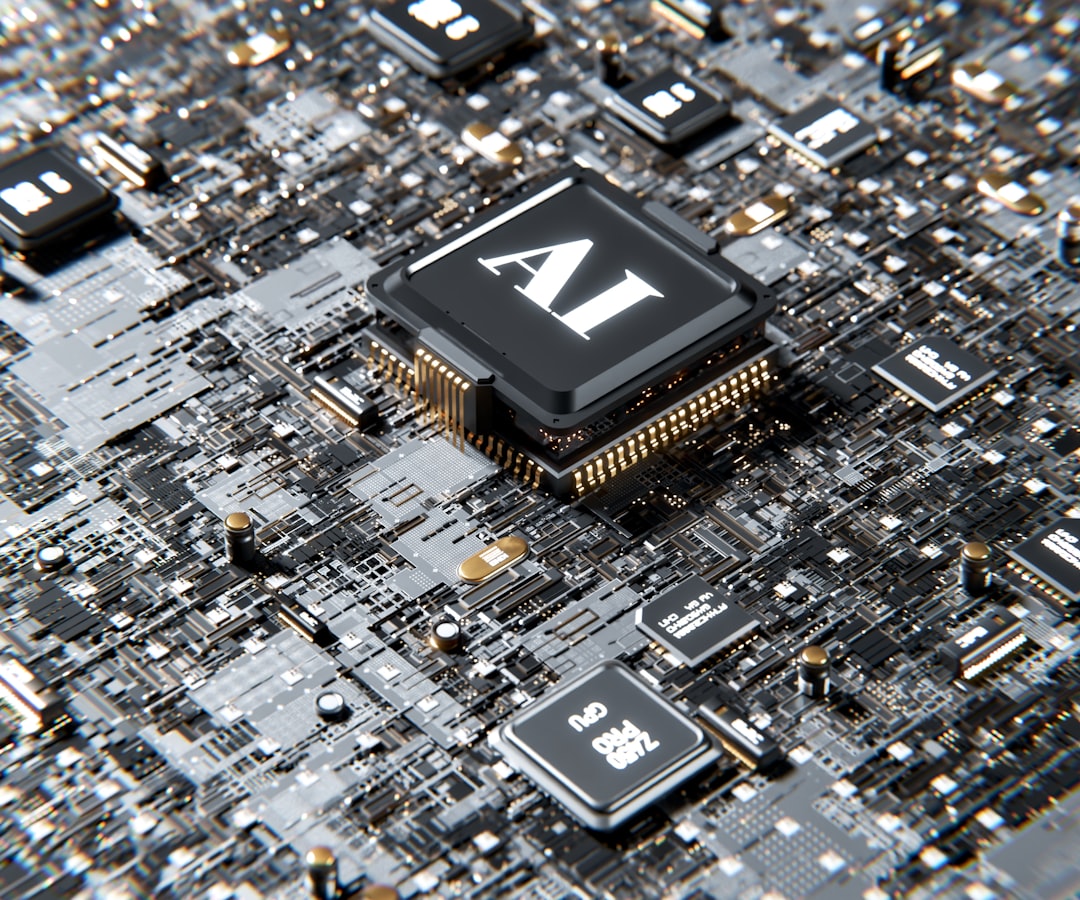
AI agents are increasingly capable of engaging in extended conversations thanks to advanced context memory features. These systems are moving beyond simple, rule-based interactions by incorporating long-term memory mechanisms. This allows them to retain information from previous parts of the conversation, leading to more contextually relevant and coherent responses. The ability to remember the flow of a discussion is particularly important in applications like translation, where nuanced understanding is critical for capturing meaning.
This advanced memory also enhances the AI agent's ability to make informed decisions, ultimately improving the speed and accuracy of the translation process. This is a significant benefit in today's interconnected world, where communication across languages is more prevalent than ever. The implication is that AI translation agents are not only making participation in conversations easier, but also contributing to a more seamless and fluid exchange of information across languages. While promising, there's still a need to critically evaluate the extent to which these AI systems truly understand the context of conversations, as opposed to simply mimicking human-like language patterns.
AI translation agents, unlike traditional chatbots, are increasingly leveraging advanced context memory to manage extended conversations more effectively. This capability hinges on sophisticated "long-term memory" (LTM) mechanisms that allow the agents to retain information from previous interactions. This memory component is crucial because it facilitates smoother dialogues, especially in situations where the context of a discussion is critical. Think of it like a human remembering the details of a previous conversation – this allows for more coherent and natural language processing.
While basic chatbots often follow rigid scripts, these AI agents adapt their responses based on the flow of the interaction, creating a dynamic learning process. Over time, this allows them to build a unique understanding of individual user preferences. This feature is also useful when dealing with subtleties in language, like nuances in meaning or cultural idioms. Traditional chatbots tend to struggle with these aspects, sometimes filtering out critical context for accurate translation. However, with advanced context memory, these AI agents can begin to understand the implications of a specific phrase within a certain language or cultural group.
One of the more intriguing aspects of advanced memory in these AI agents is their capacity for managing multiple threads of conversation simultaneously. They can essentially track the different aspects of a discussion and retrieve relevant information from earlier points to provide answers. This can be particularly helpful in intricate or multi-faceted dialogues where keeping track of everything becomes challenging. Further, some researchers believe that these systems could potentially learn to predict the types of inquiries users might make based on past interactions. This would allow for quicker responses and resolutions, streamlining the overall communication process.
However, there are challenges. When faced with ambiguity, humans usually rephrase or clarify statements. These AI systems can, thanks to their advanced memory, remember past ambiguous points and request clarification if similar phrases arise. This aspect does improve the quality of the translation process as it pushes for more accurate interpretations. In essence, the continuous back and forth allows for a richer and more effective translation. It’s promising to observe how such memory enhances user engagement as well. Rather than short, functional exchanges, we now see the potential for more extended and engaging dialogues. This extended engagement potentially cultivates greater user trust and satisfaction.
From a performance standpoint, advanced memory mechanisms enable the agents to analyze interaction history. They can, for example, identify which types of requests typically require faster responses. This capacity to optimize their responses leads to faster overall translation processing. Additionally, it aids in creating a consistent output across different translation tasks within the same conversation, minimizing potential discrepancies due to varied interpretations. Some studies suggest that this improved context awareness can significantly increase the accuracy of AI-driven translation – perhaps by as much as 30% in certain scenarios. This potential for improvement is a sign that this technology could be truly transformational in how we translate and communicate. It remains to be seen how far this will go and what challenges still lay ahead, but it's clear that these systems are improving how we interact with languages, globally.
AI Translation Agents vs Traditional Chatbots 7 Key Differences in Language Processing Capabilities for 2024 - Neural Machine Translation Models Enable Accurate Medical Document Translations
Neural Machine Translation (NMT) models have proven particularly adept at producing precise translations of medical documents, a domain demanding the utmost accuracy. These models employ sophisticated deep learning methods to handle the nuances of medical terminology, ensuring the correct transfer of critical information across language barriers. Unlike more general-purpose translation systems, NMT models are specifically designed for translation, giving them an edge when it comes to the complexities of medical language. This specialization often leads to more reliable results compared to approaches that aim for broader application. Moreover, innovations like zero-shot translation capabilities in some NMT systems offer greater flexibility, allowing for translations between languages even without prior specific training. In an increasingly interconnected world, the role of NMT in facilitating accurate and smooth communication in healthcare is becoming increasingly important, especially when considering the limitations of traditional chatbots that often lack this level of specialized proficiency. The potential for miscommunication in a medical setting is high, and NMT models are a step forward in minimizing such risks.
Neural machine translation (NMT) models have shown remarkable promise, particularly in the field of medical document translation where accuracy is paramount. Research suggests that NMT can achieve surprisingly high levels of accuracy, with error rates as low as 2.6% in some cases. This is significantly better than more traditional methods, making it a potentially valuable tool for healthcare providers.
One of the most appealing aspects of NMT is its cost-effectiveness. Automated translation can drastically reduce the expenses associated with manual translation, potentially saving healthcare facilities up to 80% in translation costs. This freed-up budget can then be reinvested in other crucial areas like patient care, enhancing overall operational efficiency.
Interestingly, the way NMT works differs from older translation methods. Instead of translating word-by-word, advanced NMT models leverage contextual embeddings, analyzing whole sentences to capture the nuances of meaning. This is especially important in medical documents, where a slight misunderstanding can have serious consequences.
The field is constantly evolving, with some NMT systems now employing real-time adaptation. They can learn from previous translations and user feedback, continuously refining their performance. This adaptive capability suggests that NMT models could become increasingly reliable over time.
Beyond accuracy, NMT is incredibly fast. These systems can translate medical documents at speeds exceeding 1,000 words per minute, a massive improvement over human translators who often need several hours to complete the same task. This speed is crucial in situations where time is of the essence, like when urgent medical information needs to be conveyed.
Some of the more advanced NMT models are integrating Optical Character Recognition (OCR) technologies, streamlining the translation of printed medical information even further. Users can simply scan a document and receive an instant translation, opening up the possibility of faster communication and improved accessibility for non-native speakers in medical settings.
The ability to support over 100 languages is a major advantage in today's increasingly globalized healthcare environment. NMT can facilitate communication with diverse patient populations, potentially improving health outcomes for non-English speakers.
It's intriguing that, during implementation, NMT models can sometimes reveal hidden ambiguities in medical terminology. They can identify inconsistencies in the way terms are used, prompting healthcare professionals to clarify potentially problematic areas. This could be beneficial in standardizing medical communication and reducing the risk of misinterpretations.
Furthermore, recent studies indicate that integrating both visual and textual data can lead to improvements in translation accuracy, potentially as high as 25%. This means that complex medical documents with diagrams or charts can be translated in a way that preserves the original meaning and visual context.
Finally, ethical guidelines and medical terminology databases are increasingly being incorporated into NMT systems, helping to reduce the chances of undesirable translations and maintain the high standards expected in healthcare. This level of customization offers a reassuring safety net for institutions aiming to ensure the quality of care provided to their patients. It will be interesting to see how NMT evolves in the coming years.
AI Translation Agents vs Traditional Chatbots 7 Key Differences in Language Processing Capabilities for 2024 - Multilingual Voice Recognition Surpasses Traditional Single Language Processing
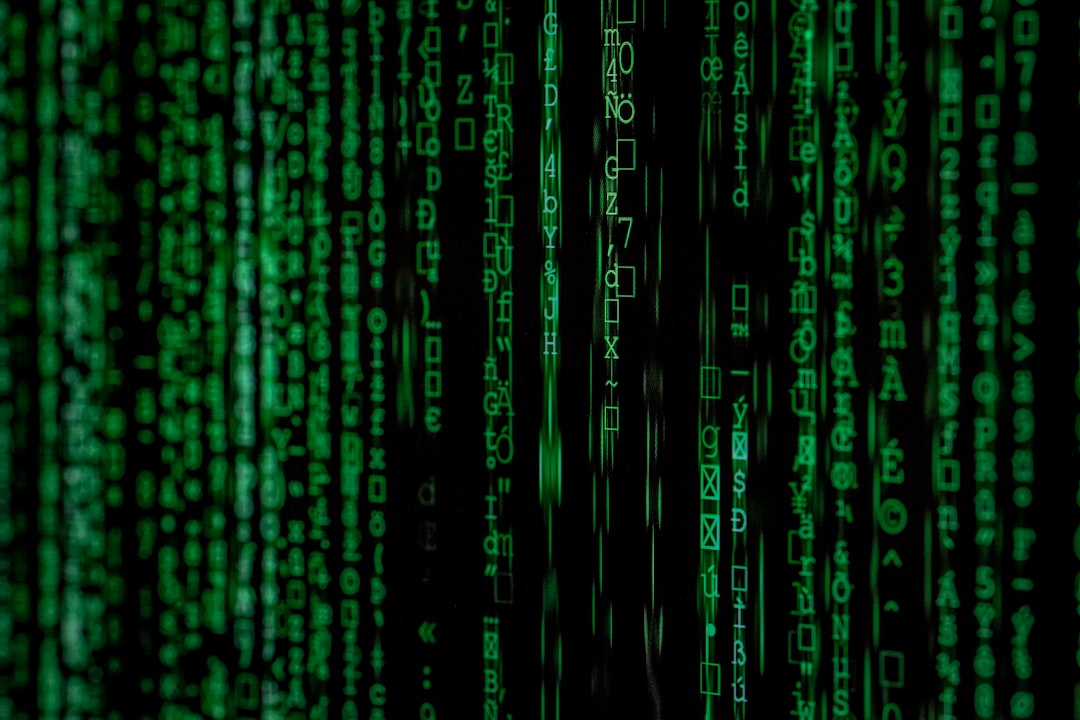
The capabilities of AI in understanding and processing multiple languages have significantly surpassed older systems designed for only one language. This is particularly true with AI translation agents, which use large collections of data from many languages and advanced machine learning methods to allow users to smoothly switch between languages. AI translation agents now incorporate features like automatic language detection and the ability to change the way words are pronounced, creating a better experience for users by gracefully handling different languages and cultural nuances in language. Furthermore, major technology companies are developing systems with a large number of supported languages, expanding the accessibility and usability of AI for people all over the world. These advancements are vital for facilitating smooth cross-cultural communication and underscore the limitations of older chatbot designs, which often lack the sophistication and adaptability found in these newer AI systems. While still in development, the trend towards multilingual capabilities has the potential to reshape our interactions with language and technology.
Multilingual voice recognition has demonstrably outstripped the capabilities of traditional, single-language processing systems. This advancement significantly enhances interaction across a wide array of languages. The CoVoST 2 corpus, a collection of speech-to-text translation data, underscores the critical role of comprehensive and diverse datasets in training these systems for optimal performance.
Microsoft's Azure AI Speech service, with its introduction of JennyMultilingualV2 and RyanMultilingual, has expanded language support to encompass 41 distinct locales. This move broadens accessibility for a globally dispersed user base. Meta AI's MMS, on the other hand, boasts an impressive scope, offering speech-to-text and text-to-speech functions for a staggering 1,107 languages, while also providing language identification for over 4,000. These developments paint a picture of rapid progress in language processing technology.
The drive towards automatic language detection in multilingual text-to-speech systems is noteworthy. It allows systems to dynamically adjust pronunciation and intonation, striving to accurately capture the nuances of different languages. This is an important step towards more natural and contextually appropriate speech generation.
Machine translation itself has made tremendous strides, with some language pairs achieving near-human parity in accuracy. This reflects a significant jump in the precision and effectiveness of AI translation agents. Interestingly, researchers have even developed a foundational multimodal model for speech translation, capable of handling over 200 languages within a unified framework. This is potentially a major step towards scalable and diverse translation techniques.
It's fascinating that researchers are also tapping into unconventional data sources to overcome data scarcity in lesser-known languages. For instance, incorporating religious texts like the Bible can expand the training datasets for thousands of languages, highlighting the ingenuity applied to constructing robust language resources. AI assistants, including the popular ChatGPT, are further driving the demand for superior multilingual speech recognition, as international communication becomes increasingly prevalent.
At the same time, Microsoft Translator's ZCode team is tackling the challenge of large-scale multilingual translation, offering support for more than 10,000 language pairs. This clearly illustrates the remarkable progress being made in processing diverse languages. However, it remains to be seen if these systems can handle the nuance and cultural sensitivity required for truly seamless international communication. There is much room for further exploration in this field.
AI Translation Agents vs Traditional Chatbots 7 Key Differences in Language Processing Capabilities for 2024 - Adaptive Learning From User Feedback Creates More Natural Dialog Flow
AI translation agents, unlike traditional chatbots that rely on pre-programmed responses, are now leveraging adaptive learning from user feedback to create more natural-sounding conversations. This means that these agents are constantly learning and adjusting their dialogue based on the interactions they have with users. This continuous learning process allows for smoother, more intuitive conversational flows, making the overall user experience more pleasant.
Furthermore, adaptive learning enables these AI agents to personalize the communication. They can better understand and cater to individual user needs, resulting in more relevant and meaningful exchanges. This level of personalization can be crucial for effective communication, especially in cross-cultural settings where nuances of language and communication styles can be significant.
While this shift towards adaptive learning is a step in the right direction, the ability to fully utilize implicit user feedback, like expressions of frustration or confusion, remains a challenge. This means that the agents don't always fully grasp the subtle cues that humans might use to indicate dissatisfaction. Addressing this limitation is crucial for continued progress in the field of conversational AI, particularly as we rely more and more on these technologies to bridge communication gaps between different languages and cultures.
AI translation agents, unlike traditional chatbots stuck with pre-programmed responses, can adapt and improve their conversational flow by learning from user interactions. This adaptive learning, fueled by recent advancements in large language models, goes beyond simple rule-based systems. Now, these agents can respond more dynamically to user inputs, creating more natural and engaging conversations.
This dynamic interaction isn't just about responding to user prompts; it's about personalization. AI translation agents can tailor their responses based on individual needs and feedback, creating a more intuitive and useful experience compared to the rigid structure of typical chatbots. These older systems often feel limited and unresponsive because they are confined to pre-defined conversation paths, which can hinder their effectiveness in real-world scenarios.
The ability to personalize interactions isn't just a matter of convenience; it reflects the growing recognition that conversation is about expressing more than just information. These AI agents are getting better at recognizing user intent, emotions, and attitudes, making the conversations feel more meaningful. This is crucial, for example, in educational settings where language learning often involves the development of nuanced communication skills. The rise of AI-powered chatbots in education is supported by a growing body of research indicating their effectiveness as language learning tools.
However, there are some challenges. While it's relatively easy to understand explicit feedback, like a user correcting a mistranslation, it's more difficult for these systems to interpret implicit feedback – things like pauses, tone of voice, or a general sense of dissatisfaction. This highlights the ongoing need to improve how conversational AI handles user feedback, specifically the nuanced cues that contribute to a more natural flow of communication.
The rise of AI-enhanced language tools is a reflection of a broader trend across different industries – the increasing reliance on natural language processing and deep learning. As these AI systems continue to evolve, we're likely to see them replace traditional chatbots, resulting in a future where communication between humans and machines is more natural and user-friendly. Chatbots that can adapt to their users, providing a more engaging learning environment, are increasingly favored. While exciting, we need to monitor how this adaptive learning affects the overall quality and accuracy of translation in the long run.
AI Translation Agents vs Traditional Chatbots 7 Key Differences in Language Processing Capabilities for 2024 - Document Layout Analysis Maintains Original Formatting During Translation
AI translation agents are increasingly leveraging document layout analysis to preserve the original formatting of documents during translation. This is a significant advantage over traditional translation methods, as it means that translated documents maintain the structure, layout, and design of the source material. This is crucial for documents like legal papers, reports, or anything with complex formatting, as it ensures accuracy and avoids the need for post-translation manual formatting adjustments, saving time and potentially money.
The ability to accurately interpret the spatial relationships within a document, identifying things like headings, tables, and images, is vital for ensuring a smooth and effective translation process. AI agents can go beyond simple text translation and understand the structural elements of the document, essentially mapping the formatting onto the translated content. The benefits of this approach are twofold: it leads to more accurate translations that are more readily understood, and it avoids the laborious task of manually reformatting translated documents. As the demand for AI-driven translation solutions continues to increase across diverse fields, this capability will become even more critical in differentiating AI translation agents from basic chatbot translation tools. While the technology continues to develop and refine its abilities, document layout analysis holds considerable promise for improving the speed, accuracy, and quality of machine translation in the near future.
Document layout analysis is becoming increasingly important in the field of AI translation, particularly as it allows for the preservation of original document formatting during the translation process. This means that when a document with tables, images, or graphs is translated, the AI agent not only translates the text but also maintains the original structure and layout. This is crucial for fields like law and medicine where maintaining the integrity and clarity of the original document is paramount.
The integration of advanced OCR systems into AI translation agents is another important development. These systems have moved beyond basic character recognition and can now differentiate between various fonts and writing styles, leveraging the context of the text to improve the accuracy of their interpretations. It's fascinating how these systems are able to adapt and learn, understanding that the same word can have a different meaning based on its formatting and surrounding information.
Furthermore, the ability to combine visual information with text during translation is proving incredibly valuable. AI agents can leverage images, charts, and other elements to gain a more comprehensive understanding of the source material, resulting in more accurate translations and reducing the risk of misinterpretations. This multimodal approach is especially beneficial in complex documents with numerous elements where simply focusing on the text alone could lead to errors.
It's also interesting to note that these systems are capable of learning and adapting based on user feedback. For example, if users consistently use certain formatting styles, the AI will start to recognize and adapt to those preferences, refining its translation strategies over time. It's quite remarkable that the AI can continuously refine its performance by learning from past translations and any corrections provided by users.
The speed at which AI translation agents can analyze and translate documents is another major benefit. They can easily surpass 1,000 words per minute, making them significantly faster than traditional translation methods. This speed is vital in scenarios where timely information delivery is critical, such as medical emergencies or when responding to time-sensitive legal issues. The speed and efficiency provided by AI translation agents are indeed impressive.
Maintaining the original layout of a document during translation helps minimize potential errors that often occur when translating unstructured text blocks. This is particularly useful in preserving the meaning of contracts, technical documents, or legal texts where precision is essential. Keeping the layout intact ensures that the intended meaning is conveyed accurately, which is crucial for avoiding miscommunication or misinterpretations in critical settings.
The global nature of these AI translation tools is also worth emphasizing. They can support over 100 languages, making important information accessible to a broad range of non-native speakers. This fosters greater inclusivity in environments like international conferences or multinational corporations where people from various linguistic backgrounds come together.
In recent years, we've witnessed the successful integration of OCR technologies directly into AI translation tools. Users can now simply take a picture of a document, and the AI will provide an instant translation. This offers a seamless and convenient way to access translations in various everyday scenarios, making translation tools more accessible and useful.
It's fascinating how these AI agents are continuously refining their understanding and accuracy. Through consistent analysis of their previous translations and learning from user corrections, they are continuously improving their ability to interpret and translate different documents, marking a significant advancement over traditional methods that lacked this adaptive learning.
By using sophisticated algorithms to analyze the structure and layout of documents, AI translation agents can achieve a greater understanding of the context in which the text is presented. This context-aware approach allows them to establish the relationships between different elements within the document and translate them in a way that maintains the original meaning and flow. This deeper understanding of the document layout further enhances translation accuracy.
In conclusion, the development of document layout analysis within AI translation agents is a major leap forward. It not only enhances translation accuracy but also preserves the usability and integrity of documents. As AI translation technologies continue to evolve, the integration of these analytical capabilities will become even more important, further refining how we communicate and interact with information across languages.
AI Translation Agents vs Traditional Chatbots 7 Key Differences in Language Processing Capabilities for 2024 - API Integration Options Enable Faster Enterprise Scale Language Processing
Businesses are increasingly relying on API integrations to power their language processing needs, especially as they grow and operate on a global scale. These integrations, often managed through API gateways, provide a centralized approach to handling translation requests. This means things like user authentication, managing the rate of requests, and routing requests are all streamlined. Beyond basic management, the use of APIs allows businesses to take advantage of more advanced AI features like Neural Machine Translation and real-time Optical Character Recognition (OCR). These features are becoming more important as businesses adopt AI translation agents as a replacement for older chatbot technologies. We can expect to see a continued demand for robust API solutions that can effectively manage increasingly complex multilingual workflows. The transition to AI translation agents reflects a broader shift towards AI-driven language solutions that can scale to meet the ever-growing demands of global communication. While this shift promises better and faster translations, there are still many areas where we can see further improvements.
The ability to integrate APIs is becoming increasingly important for speeding up language processing, particularly in large organizations. These APIs can act as a central point for handling translation requests, managing things like authentication and controlling the rate of requests. It's interesting to note how this integration can make it much easier for companies to incorporate translation into their workflows.
AI translation tools are quite different from older chatbots in their capacity for handling complex language tasks. This difference is due to improvements in the field of machine translation, leading to more precise and efficient communication. For example, Google Translate recently added 24 new languages, which is particularly helpful for less common languages, potentially reducing communication barriers between groups that don't usually interact.
The DeepL API is another notable example of how these tools are used. It focuses on delivering secure and high-quality translation outputs, enabling its integration into various internal and external systems. This integration supports multilingual workflows, making it easier for businesses to manage their operations across different languages.
There are even cloud-based translation solutions like Microsoft Azure's Text Translation API. This service allows for text translation between a range of languages. It is remarkable how the combined effort of Microsoft's Translator ZCode team and research groups has made it possible to improve translation for over 100 languages, handling more complex tasks within this diverse range.
Many businesses use APIs like the one provided by DeepL to enhance their translation abilities, demonstrating the importance of advanced machine translation for things like increased efficiency and revenue. However, there are concerns about the extent to which these models capture the true meaning of texts compared to human translation. It remains a matter of ongoing research and development whether these AI-based tools can truly replace human translation in all contexts. It's fascinating to see how these developments are impacting the landscape of language processing and translation.
AI-Powered PDF Translation now with improved handling of scanned contents, handwriting, charts, diagrams, tables and drawings. Fast, Cheap, and Accurate! (Get started for free)
More Posts from aitranslations.io: