AI Translation and the Evolution of English Alphabet Recognition
AI Translation and the Evolution of English Alphabet Recognition - Neural Machine Translation Revolutionizes Alphabet Recognition
The rise of Neural Machine Translation (NMT) has significantly impacted alphabet recognition, particularly in the realm of AI-powered translation. NMT's reliance on deep learning methods has moved beyond older, rule-based translation systems, leading to improved accuracy and a more natural flow in translated text. This progress has also revealed inconsistencies in translation quality across different languages, highlighting a gap in the accessibility and effectiveness of NMT for less-resourced languages. The development of Large Language Models (LLMs) further underscores the importance of NMT in achieving more sophisticated translations. These models suggest a future where translation can capture greater nuance and context. While NMT has proven remarkably effective, questions remain about its equitable application across the full spectrum of languages. This necessitates ongoing efforts to refine and expand the technology, ensuring future advancements benefit all language communities.
Neural Machine Translation (NMT) has significantly impacted the field of alphabet recognition, particularly surpassing traditional Optical Character Recognition (OCR) methods in accuracy, especially with handwritten text. NMT's ability to leverage deep learning and adapt to various font styles and languages, even with limited training data, offers a notable improvement in real-time text recognition. This adaptability leads to efficient handling of non-standard text formats, ultimately accelerating the translation workflow.
The synergy of NMT with OCR systems creates a streamlined translation pipeline. Images are processed through OCR, and the extracted text is instantly translated by NMT, drastically reducing the time needed for document translation. Imagine converting lengthy documents from hours to minutes – this is particularly beneficial in fast-paced environments like international business meetings.
Moreover, the introduction of transformer models in NMT has facilitated a more nuanced understanding of language, enabling the accurate translation of idiomatic expressions and subtle linguistic cues. It's no longer simply about word-for-word replacement, but rather a comprehension of the intended meaning within the context of the sentence.
Interestingly, NMT systems seem to possess a unique learning capability during deployment. Through user interaction and feedback, these systems can refine their alphabet recognition, effectively 'learning' from errors via a process called reinforcement learning. This constant adaptation allows NMT to evolve and improve its performance over time.
Furthermore, NMT demonstrates an impressive ability to incorporate new alphabets and writing systems efficiently, proving crucial in multilingual contexts. Its flexibility in incorporating new information enhances its versatility and accuracy in handling diverse languages.
When comparing NMT's efficiency to earlier translation systems, studies suggest it can deliver translations up to five times faster. This speed advantage is highly beneficial in time-sensitive industries like finance or legal fields, where immediate information processing is paramount. While the speed increase is appealing, the question remains: does this also translate into improved quality?
The transition to NMT has led to notable improvements in user satisfaction with translation results. NMT excels at capturing the essence and intent of the original text, promoting smoother cross-language collaboration, and potentially bridging communication gaps.
The field is actively exploring hybrid models, combining the strengths of both NMT and traditional rule-based methods. NMT provides adaptability and flexibility, while rule-based approaches offer high accuracy for structured text. Finding the sweet spot in these hybrid models may offer a more balanced solution for diverse translation needs.
Finally, the realm of neural translation is expanding beyond just written text. NMT's integration with advanced speech recognition technologies has made it possible to process and translate spoken language, opening doors for applications like real-time conversational interfaces and broader uses in communication. It will be interesting to see how these advancements continue to evolve in the near future.
AI Translation and the Evolution of English Alphabet Recognition - AI-Powered OCR Enhances Accuracy in Document Translation
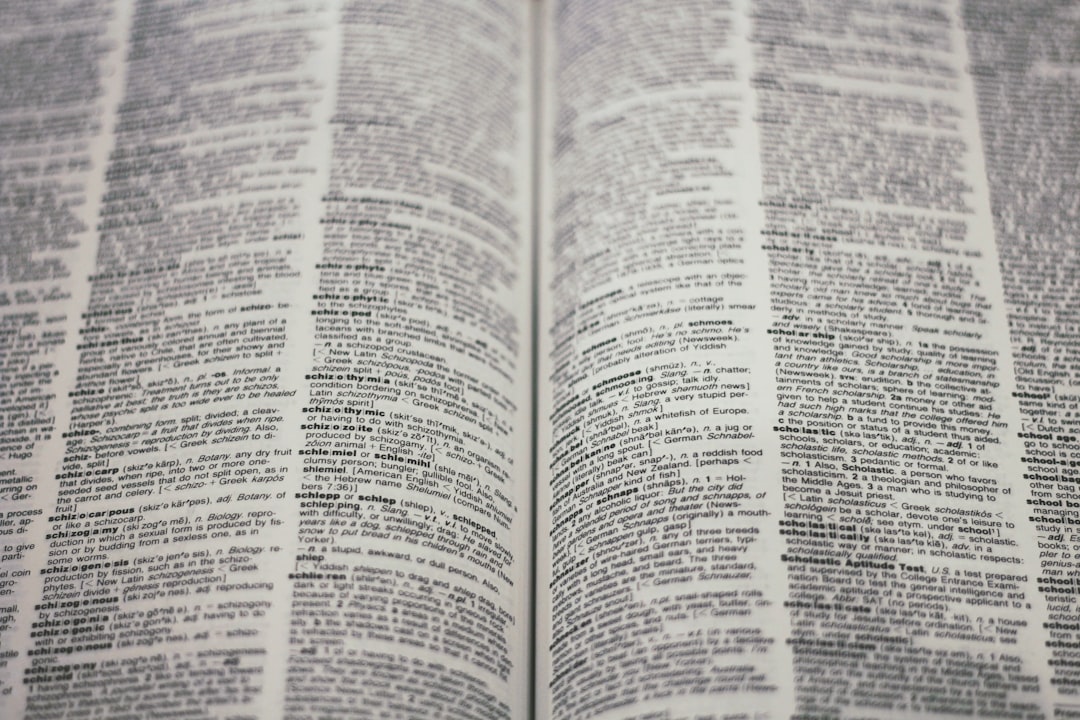
AI-powered OCR has emerged as a crucial component in enhancing the accuracy of document translation. By employing machine learning techniques, AI-driven OCR systems are adept at recognizing text across diverse font styles and even degraded or handwritten documents. This adaptability is a significant improvement over older methods, leading to more reliable extraction of data and a substantial reduction in errors during the translation process.
The increasing adoption of AI OCR across industries signifies a shift towards automated document processing. This automation brings about operational efficiencies and, in turn, helps organizations achieve cost reductions. The integration of AI technologies like OCR, NLP, and ML is fundamentally changing the way we analyze documents, enabling a faster and more precise approach to understanding text across different languages.
However, it's important to acknowledge that AI models need continual refinement. The ongoing evolution of language necessitates a steady flow of updated linguistic data to fuel AI models and maintain the high accuracy needed for reliable translation. The dynamic nature of language necessitates a consistent effort to ensure that AI-powered tools can accurately capture the ever-changing landscape of human communication.
AI-powered Optical Character Recognition (OCR) has become remarkably accurate, especially for printed text, reaching success rates nearing 99% in some instances. This leap in accuracy significantly reduces the errors that used to plague document translation processes, where human intervention was often required for correction. While the technology's focus has traditionally been on standard fonts, recent advancements in deep learning allow AI-powered OCR to adapt readily to new fonts and document layouts with minimal retraining. This adaptability broadens the scope of document types that can be processed efficiently, mitigating the need for constant manual adjustments.
Furthermore, the integration of deep learning into OCR has led to a substantial reduction in the time required for text extraction and subsequent translation. Tasks that once took hours can now be completed in minutes, greatly boosting efficiency in various fields. Legal and financial operations, particularly, have benefited from this speed increase, where timely information processing is paramount. It's intriguing to see that many leading OCR systems are now capable of recognizing not only alphanumeric characters but also a range of symbols and special characters. This broader scope of recognition is crucial for handling complex documents, such as multilingual forms or technical manuals, where such characters are prevalent.
One unexpected development in modern OCR technology is its ability to extract and translate text from complex images, such as photographs of signs or handwritten notes. This capability was a major challenge for traditional OCR methods, highlighting how AI has pushed the boundaries of what's possible in text extraction. However, it's important to acknowledge the limitations. Critics have rightly pointed out that while fast and efficient, AI-powered OCR and subsequent translation can struggle with context, especially when encountering idiomatic expressions or nuanced phrasing. These scenarios can lead to inaccuracies in the translated text, something researchers continue to address.
Interestingly, AI-powered OCR systems now utilize neural networks to learn from their errors. They can adapt and refine their accuracy over time through a process of self-correction, incorporating user feedback and new data inputs. This iterative process ensures that the technology continuously improves its performance. To enhance translation quality further, many OCR systems are now incorporating Natural Language Processing (NLP) features. This allows them to go beyond simple text extraction and analyze the sentiment and broader context within documents being translated.
The accessibility of high-quality AI-based OCR solutions has also improved due to reduced costs in recent years. This opens up these advanced translation services to smaller businesses and even individual users, making them more readily available. While a significant advancement, the question of whether AI-powered OCR can completely replace traditional OCR methods remains. Hybrid approaches, combining traditional methods with AI capabilities, have shown great promise in specific domains, such as legal documents. This combined approach seems to be particularly beneficial in situations demanding strict adherence to language and formatting requirements, suggesting that the future might lie in the fusion of both technologies.
AI Translation and the Evolution of English Alphabet Recognition - Real-Time Translation Apps Leverage Advanced AI Algorithms
Real-time translation apps are leveraging sophisticated AI algorithms to deliver faster and more accurate translations, effectively bridging the gap between languages and fostering global communication. These apps, powered by advanced machine learning, can now translate both spoken and written text in near real-time, a significant leap from earlier translation methods. These AI-driven tools adapt to individual language preferences and continuously learn from user interactions, resulting in progressively more refined and accurate translations. Yet, these advancements also raise questions about the limits of AI in handling complex linguistic nuances, especially when dealing with idioms and contextual understanding. Despite these challenges, the field of real-time translation is evolving rapidly, making cross-language communication easier and more accessible than ever before. The potential to break down language barriers is undeniable, but it's important to acknowledge the ongoing refinements needed to ensure translation accuracy across diverse language contexts.
Real-time translation apps have become increasingly sophisticated, relying on advanced AI algorithms to translate spoken or written text instantly. These apps are bridging communication gaps, allowing for immediate interactions across languages. The underlying technology is built on neural network architectures like transformers, which excel at capturing the context within sentences, leading to more natural-sounding translations.
It's fascinating how these AI systems are constantly learning and adapting. Many apps now incorporate online learning, meaning they continually refine their translations based on user feedback and corrections. This personalization element leads to a user experience that's tailored to individual preferences over time. Moreover, the integration of OCR and NMT has drastically accelerated document translation workflows. Images with text can be processed and translated in a matter of seconds, converting what used to be time-consuming tasks into highly efficient processes.
Going beyond printed text, modern OCR has progressed to interpreting text within images, like road signs or menus. It's remarkable how these systems attempt to understand the broader context of the image, something that was a significant challenge for older OCR technologies. However, the reality is that many of these apps claim to support a vast number of languages, including some with limited resources. This disparity in resource availability often results in noticeably different translation quality across languages, a kind of "ghost language" issue where the quality isn't consistent.
There are still areas where these AI-powered tools struggle, especially with images that are complex or present challenges like varying lighting conditions or handwritten notes. Despite significant strides, perfection hasn't been reached. On the positive side, the use of these tools has led to a notable decrease in the costs associated with translation, particularly in business environments. Companies can streamline operations and reduce expenses by automating certain translation tasks that previously needed human intervention.
Though these apps handle technical language well, idiomatic expressions remain a challenge. It's quite common for translations to lose cultural nuances in such scenarios, a topic that researchers are continually working on. Looking ahead, it appears we'll see more hybrid approaches combining traditional translation methods with AI solutions. These blended systems are likely to be preferred in situations demanding the highest levels of accuracy, such as legal documents. The future of AI-powered translation likely involves striking a balance between the adaptability of neural networks and the rigorous precision of traditional approaches.
AI Translation and the Evolution of English Alphabet Recognition - Machine Learning Models Adapt to Evolving English Language Patterns
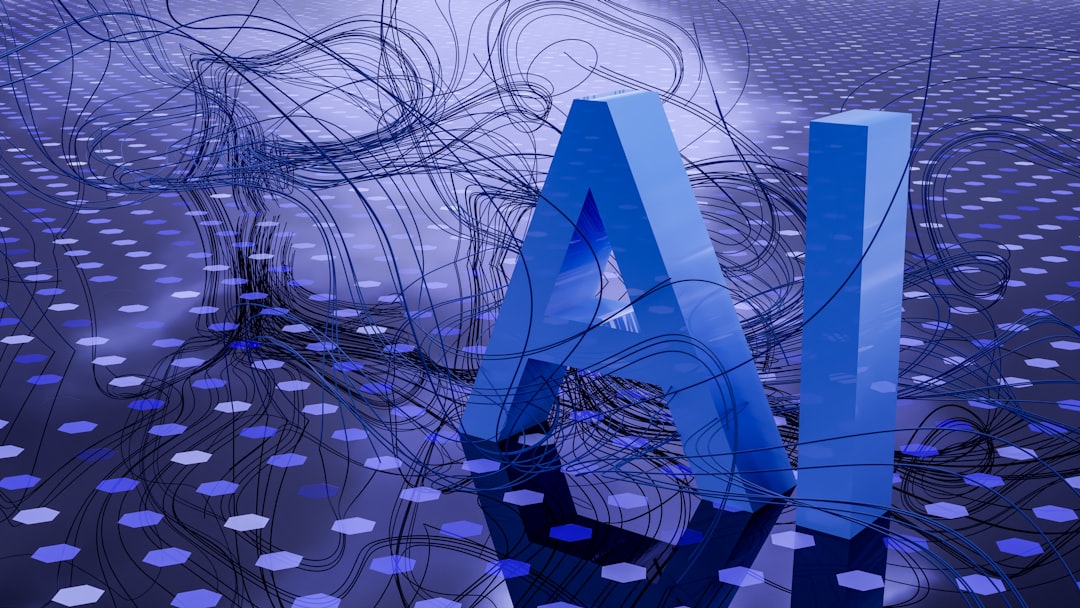
Machine learning models underpinning AI translation are becoming increasingly adept at recognizing and adapting to the ever-changing patterns within the English language. This adaptability is essential as the language evolves, with new expressions and subtle linguistic shifts constantly emerging. These models are trained on vast amounts of text data, allowing them to learn and fine-tune their understanding of these evolving patterns, leading to improvements in the accuracy of translations.
Despite their advancements, these models still face challenges when it comes to adapting in real time, particularly when dealing with specialized or technical language specific to certain industries or domains. This highlights a need for ongoing refinement of these AI systems and a reliance on user feedback to identify and rectify errors.
The inherent dynamism of language necessitates a constant cycle of improvement for these models. They must be continually refined to keep pace with the ongoing changes in how we communicate. Therefore, a delicate balance between speed and comprehensive contextual understanding is critical for any AI-powered translation tool aiming to effectively bridge language barriers in our increasingly interconnected world. The demand for accurate and responsive translation solutions is rising, and meeting that demand necessitates a continued focus on both technological advancements and user experience.
Machine learning models underpinning AI translation are becoming increasingly adept at adapting to the ever-changing nature of the English language. These models are able to learn and adjust to new slang, regional variations, and emerging terminology almost instantly, mirroring the dynamic and evolving nature of human communication. This adaptability is particularly crucial in the context of rapidly evolving internet-based communication where new phrases and styles emerge quickly.
One interesting aspect is how many AI translation systems are designed with a self-correcting feedback loop. Users' inputs and corrections directly influence future translations, refining the system's accuracy over time. Essentially, these systems "learn" from past errors and continuously improve their performance. While this shows promise, it raises a question about the potential biases that could develop within a system primarily shaped by a limited set of users.
Furthermore, the ability of neural machine translation (NMT) models to understand context has seen considerable improvement. This deeper understanding means that they're not just focusing on literal word-for-word replacements. Rather, these systems strive to interpret the intended meaning behind sentences, which is critical for translating humor, idioms, or culturally sensitive expressions. However, we still have a way to go before achieving truly natural-sounding translations that capture the subtle nuances of human expression.
Another significant development has been the remarkable advancement in Optical Character Recognition (OCR) technology, especially regarding handwritten text. AI-driven OCR has significantly reduced errors in document translation compared to older OCR systems, which frequently struggled with variations in handwriting or unique font styles. It's worth noting that while the technology has advanced greatly, it still struggles to accurately translate documents written in certain, less common scripts, and the error rate can increase for low-quality images or very obscure handwritings.
Interestingly, some real-time translation apps are now capable of adapting their translation algorithms in real-time based on individual users' interaction patterns. This level of personalized optimization means future translations are refined for specific users and their preferred language styles, a feature that could impact how people communicate across languages in the coming years. This personalized approach is interesting, but it also raises concerns about the potential for these systems to create “translation bubbles” where users primarily interact within their own linguistic circles.
Furthermore, modern AI translation often integrates both text and image processing, making it possible to extract and translate information directly from images, like signs or documents. This feature, while helpful, has its limitations when encountering complex backgrounds or highly stylized graphics within the source image. Despite these limitations, the development suggests a promising direction in which we could see increased integration of AI in multi-modal interfaces.
Despite the remarkable advancements in AI translation, there's a persistent issue of uneven quality across different languages. This disparity is largely due to the varying availability of training data. Languages with massive amounts of readily available text data, such as English, tend to have significantly more accurate translations compared to those with limited linguistic resources. This is a challenge that highlights the importance of equal and accessible translation technology for all language communities.
The speed of AI-driven translation continues to improve, particularly in recent years. Today's models can process and translate massive documents, or even spoken language, in a matter of seconds. This capability is vital in fast-paced environments like global business negotiations or immediate crisis communication. However, the rapid pace of translation should not come at the cost of accuracy.
One significant benefit is that AI-driven OCR has become more adaptable to a broader range of fonts and document formats compared to past generations. This flexibility means fewer restrictions on the types of documents that can be processed efficiently. It suggests that in the future, translating diverse types of documents in different languages will likely be a more common task. However, more work remains on developing reliable systems that can accurately interpret unusual fonts, or more importantly, fonts not included in the training data.
The future of AI translation may well hinge on the concept of hybrid models. These systems would integrate the strengths of AI-driven techniques with established, more traditional translation methods. Such models could prove crucial in areas where high accuracy is paramount, such as legal or medical translations, where errors can have significant consequences. This seems like a potentially useful path for researchers as these hybrids might combine the benefits of both AI and traditional methods in a more reliable way. While the future is uncertain, it is quite clear that advancements in AI translation technologies will continue to drive innovation in how we communicate globally.
AI Translation and the Evolution of English Alphabet Recognition - AI Translation Platforms Integrate Contextual Understanding
AI translation platforms are increasingly incorporating a deeper understanding of context, representing a notable leap forward in the accuracy and naturalness of translations. These platforms employ advanced machine learning techniques and natural language processing to not only recognize individual words, but also to grasp the meaning of phrases and sentences within a broader context. This capability is achieved by training these systems on massive datasets of text, allowing them to learn the subtle nuances of language, idiomatic expressions, and culturally specific references that previous translation systems often missed.
While this advancement is promising, significant challenges persist, especially in handling languages with limited digital resources or complex, nuanced expressions. The reliance on vast datasets and computational power can lead to biases, and the ability of AI to replicate the human understanding of cultural context remains an area needing further development. The path forward for AI translation requires a continued focus on fostering a synergy between technology and human expertise. Striking a balance between the speed and efficiency offered by AI with the nuanced understanding that humans bring to translation is crucial for ensuring accurate and culturally sensitive translations. The goal remains to bridge communication gaps effectively while acknowledging the intricacies inherent in human languages.
AI translation platforms are increasingly leveraging contextual understanding to improve their output. They are now better at grasping the nuances of language, like idioms and colloquialisms, by analyzing surrounding words. This is a shift from the more rigid, word-for-word translations of older systems. Interestingly, many platforms are also incorporating real-time feedback from users. This means future translations are tailored based on individual preferences and past interactions. This adaptive approach can result in higher accuracy but also raises questions about the potential for bias in the systems as they are primarily shaped by certain user demographics.
AI-driven OCR has advanced remarkably. In many cases, it can achieve impressive accuracy levels for printed text – nearly 99% in some applications. This has made older OCR methods seem almost outdated. Even with challenging inputs, such as blurry images or uncommon font styles, these systems are designed to minimize mistakes. However, it is still important to acknowledge that the accuracy isn't uniform, especially for handwritten texts or documents in languages with limited available data.
Beyond simply extracting text, newer OCR systems are capable of handling text within more complex images, like street signs or graphics. This is a big improvement from earlier OCR tools that struggled with these types of inputs. However, it does have limitations and the quality of the extracted text can be affected by factors like background details or lighting conditions.
There is growing interest in the potential of combining AI methods with traditional rule-based translation. This hybrid approach seeks to merge the flexible nature of machine learning with the strict accuracy needed in certain fields, such as legal or medical translations. It is thought that combining these techniques could be a path forward, leveraging the best of both approaches.
AI-based translation has brought a huge increase in processing speed. Systems now translate documents in seconds instead of the hours it took in the past. This rapid speed is essential for industries relying on quick turnaround times, like international business or breaking news reporting. However, there's a concern whether the speed is coming at the cost of translation quality in some cases.
There are still significant disparities in the quality of translations across different languages. Those languages with plenty of readily available training data, like English, tend to have more accurate translations compared to less-resourced languages. This is a worrisome situation because it highlights a need for more equitable application of these technologies across the global language community.
While these systems are becoming better at understanding context, they can still struggle with cultural nuances and idioms. This is an area of ongoing research where researchers hope to improve the systems' understanding of the subtleties of human language. Interestingly, many of these systems have built-in error correction mechanisms that learn from user feedback and adapt over time. This helps improve the quality of the system over time, but also raises questions about potential bias in future training data.
AI-driven OCR tools are showing a wider range of adaptability when it comes to various document types. This means that they can handle a more diverse collection of documents – including handwritten notes and various font styles – which is beneficial across many sectors. This capability is certainly a step forward, however it does still require ongoing development for certain, unusual fonts that might not be part of the training datasets.
The future of AI translation is likely to be a continued exploration of combining the strengths of the current approaches. This could lead to more robust and accurate translation in the long term. The field is very dynamic and, without a doubt, will continue to play a role in shaping the way people communicate across the globe.
AI Translation and the Evolution of English Alphabet Recognition - Ethical Considerations in AI-Driven Language Processing Systems
The increasing sophistication of AI-driven language processing systems brings forth crucial ethical considerations. A primary concern is the accountability for actions taken by AI systems, especially when they make autonomous choices during translation. The potential for bias embedded within algorithms is a significant issue, potentially leading to unfair or discriminatory outcomes in translated content. The use of personal data within these systems also raises significant questions around data privacy and consent. Ensuring transparency in how these systems operate is fundamental not only for achieving high-quality translations but also for building trust among users from diverse linguistic backgrounds. As AI translation becomes more prevalent, navigating the complexities of ethical considerations will be essential for responsible development and deployment, ensuring that the potential benefits of these technologies are accessible to all while minimizing potential harm.
Current AI translation systems, while impressive in their speed, sometimes stumble when encountering the subtleties of human language, like idiomatic expressions or culturally specific terms. This suggests that AI's understanding of the richness and nuances inherent in language may still be limited. It's a topic that requires deeper examination.
The continuous learning aspect of these systems, where they adapt based on user feedback, can be a double-edged sword. While it allows for personalized and potentially more accurate translations over time, it also introduces the risk of bias. If the training data primarily comes from a specific user group, the AI might inadvertently reinforce existing biases, resulting in skewed translation outputs. Understanding and addressing this potential for bias is crucial.
We often hear about how AI speeds up translations, which is certainly true. However, the question of whether speed equates to higher quality is debatable. In many cases, especially when dealing with complex texts, prioritizing speed can lead to compromises in the quality of the translation. This tension between speed and quality needs ongoing scrutiny.
One of the significant challenges in this field is the unequal distribution of resources for different languages. Many AI tools are much better at translating common languages like English due to the wealth of training data available for them. Languages with limited digital resources, unfortunately, receive inferior translation services. Addressing this disparity and ensuring equitable access to high-quality translation for all languages is a fundamental ethical consideration.
The incorporation of cultural context in translation is still a developing area. While AI is getting better at understanding the surface meaning of words, the deeper cultural significance and context that humans often rely on are still challenging for AI to fully grasp. This gap in understanding cultural subtleties can lead to translations that miss crucial aspects of the original message.
The ability of AI systems to adapt to evolving language patterns is undoubtedly impressive. But it raises a question about the future preservation of traditional linguistic norms. It's important to carefully evaluate how AI adapts to slang and new language trends, ensuring that core aspects of language are not compromised in pursuit of constant evolution.
Though Optical Character Recognition (OCR) has come a long way, it's still not perfect, especially when faced with complex handwritten text or unusual fonts. This can pose challenges in specific domains like legal or medical translation, where accurate document translation is essential. There is a risk that inaccuracies in the OCR process lead to mistranslations with potential negative consequences.
Real-time translation applications are increasingly designed to personalize the user experience based on feedback. While this can lead to increasingly accurate translations tailored to individual preferences, there's a chance it can introduce biases based on the specific user groups that frequently interact with the systems. It's something researchers need to pay close attention to.
Creating hybrid translation systems that combine the strengths of AI with traditional approaches holds promise. However, it’s proving difficult to achieve a harmonious blend. Finding that sweet spot where speed and accuracy are balanced in these hybrid systems is a critical area for future research.
Finally, while AI is becoming increasingly better at translating text within complex images, it still encounters challenges with poor image quality, confusing backgrounds, or very stylized imagery. This limitation can be a significant hurdle in situations requiring the accurate extraction and translation of text from visually complex data sources.
Overall, AI-driven translation is a dynamic and quickly evolving field. While many strides have been made, we must remain aware of the ongoing ethical considerations and limitations of these systems as they continue to develop and integrate into our lives.
More Posts from aitranslations.io: