AI-Powered PDF Translation now with improved handling of scanned contents, handwriting, charts, diagrams, tables and drawings. Fast, Cheap, and Accurate! (Get started for free)
AI Translation and the Precision of Timecode Enhancing Video Localization Workflows
AI Translation and the Precision of Timecode Enhancing Video Localization Workflows - AI-Driven OCR Enhances Timecode Precision in Video Subtitling
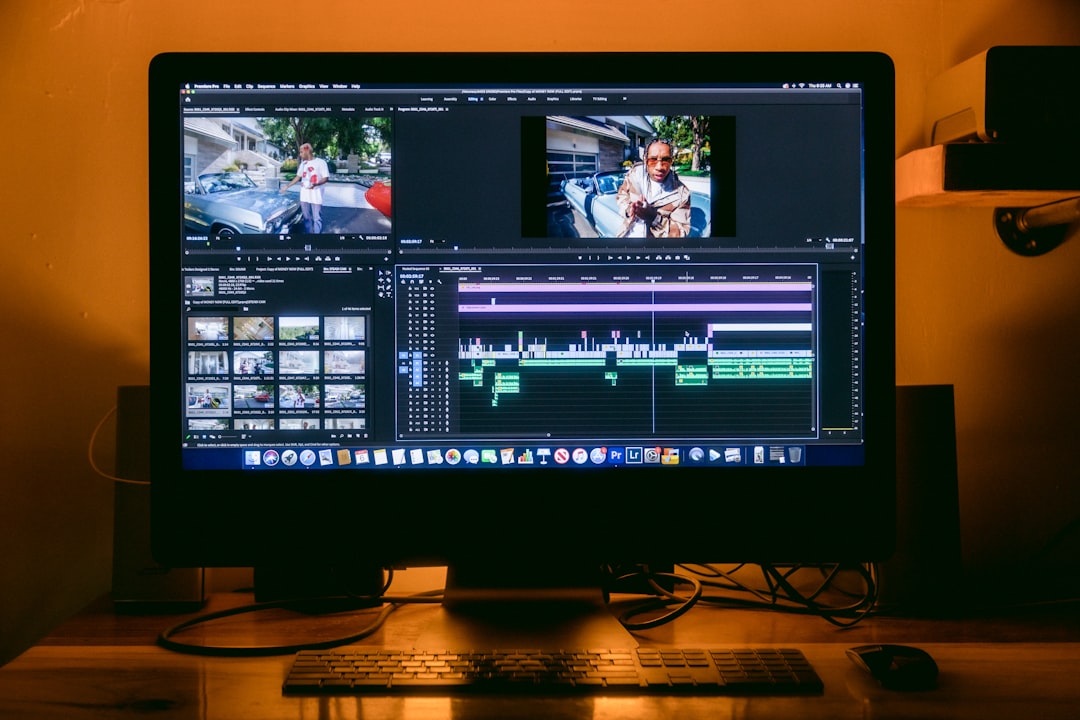
AI-powered OCR has revolutionized how timecodes are handled in video subtitling. It's no longer just about transcribing the words; AI can now precisely match the subtitles to the spoken dialogue. This automation streamlines the process, leading to faster turnaround times for subtitled videos without compromising on accuracy. The integration of these tools is especially crucial in an increasingly globalized media landscape where content needs to be quickly and readily accessible to diverse audiences. The result is a more seamless viewing experience for everyone, fostering a greater sense of inclusion through accessible video content. While the technology is still developing, the current state of AI-driven OCR significantly impacts how video localization workflows are structured, paving the way for a future where multilingual content creation is both efficient and accurate.
AI-driven OCR has become increasingly adept at extracting text from video frames, pushing accuracy levels remarkably close to 99%. This ability is proving pivotal for generating highly precise subtitles. While traditional methods often involve manually tweaking subtitle timing, AI can now leverage sophisticated algorithms to automatically assign timecodes based on a deeper understanding of speech patterns and context. This automation significantly reduces the potential for human error and the time required for post-production.
Furthermore, contemporary OCR systems demonstrate an impressive capability to parse multiple languages within a single frame. This is vital for efficiently creating multilingual subtitles and making international content readily accessible. These systems often leverage intricate deep learning models trained with vast quantities of data, enabling them to accurately distinguish between diverse fonts and styles commonly encountered across different video sources.
Beyond accuracy and multi-lingual support, the speed at which AI-powered subtitling systems operate has revolutionized workflows. Tasks that once took hours are now often completed within minutes. This speed advantage holds the potential to considerably reduce the overall time commitment of video localization projects. And this efficiency isn't limited to post-production. Combining AI OCR with speech recognition technologies allows for real-time subtitling, providing a critical advantage for live broadcasts or scenarios where immediate captioning is needed for accessibility.
The ongoing refinement of AI OCR is evident in its improved ability to detect and correct timecode inconsistencies. This feature is particularly beneficial for maintaining seamless subtitle synchronization with spoken dialogue, leading to a richer viewer experience. It's fascinating to see the cost barrier to entry for AI OCR solutions steadily decrease, making it increasingly viable for organizations with limited budgets who might have previously relied exclusively on manual subtitling.
Interestingly, many modern AI OCR tools offer custom dictionaries. This allows users to integrate specialized terminology or even slang unique to their content, further enhancing the accuracy and overall contextual relevance of the generated subtitles. Research continues into making AI OCR even more robust, particularly when dealing with demanding environments such as those with poor lighting or fast-moving imagery. These scenarios can easily confuse traditional methods of optical character recognition. The pursuit of greater AI OCR resilience across varied conditions is an ongoing challenge that researchers are striving to overcome.
AI Translation and the Precision of Timecode Enhancing Video Localization Workflows - Fast Translation Turnaround Using Neural Machine Learning
Neural Machine Translation (NMT) has become a dominant force in the translation field, particularly when speed is crucial. Utilizing deep learning methods, including the Transformer model, NMT has surpassed older translation techniques, offering a significant boost in both speed and accuracy. The recent advancements in NMT have made fast translation turnaround times a reality, particularly when paired with specialized tools. This acceleration is notably useful for video localization, where keeping subtitles precisely synchronized with video is critical for a good user experience. NMT has the potential to considerably reduce processing time, enhancing both speed and the overall quality of translations, thanks to ongoing developments in artificial intelligence. While it's still an active area of research, overcoming the computational challenges inherent in NMT could further accelerate translation processes and lead to more sophisticated and efficient workflows in various areas.
Neural machine translation (NMT) has become a dominant force in the field, largely replacing older methods due to its improved performance, particularly in speed. The core of this improvement lies in the use of deep learning algorithms, like the Transformer architecture, which enable the system to learn complex language patterns. It's quite remarkable how advancements in NMT have allowed for significantly faster translation turnaround times, especially when paired with features like translation memory. However, relying on translation memory does introduce computational overhead, and researchers are actively exploring ways to make this process even more efficient.
The shift towards end-to-end neural network architectures in machine translation has led to both better efficiency and improved accuracy. This shift is particularly important for video localization workflows, where precise timecode synchronization is critical. Interesting approaches like efficient minibatching and softmax fusion have been implemented to further boost the performance of these systems. Beyond simple translation, researchers are also investigating how to visualize what these models are doing during translation, using methods like interactive beam search and attention weights to help understand the decisions they make, potentially aiding in post-editing.
The landscape of NMT has also been fundamentally altered by the arrival of pre-trained large language models. These models have greatly increased the capabilities of NMT, particularly in terms of handling complex language nuances and understanding context. However, there is still work to be done. For instance, NMT models, despite their speed, can still make mistakes, such as mistranslations or losing context when dealing with ambiguous phrases. This is an active area of research, with the aim of refining NMT systems to handle these tricky aspects of language with greater precision.
There are also compelling applications of NMT extending beyond text. For example, the integration of OCR with machine learning has led to impressive advances in the translation of handwritten text. Neural networks are increasingly adept at deciphering even complex scripts like cursive, broadening the applicability of AI translation. Furthermore, the development of transfer learning techniques has enabled the quick adaptation of NMT to new languages, even those with limited training data. This is particularly valuable for making translation accessible for low-resource languages and making localization efforts more inclusive.
One of the more exciting developments is the ability of these models to utilize attention mechanisms. This allows them to pay more attention to relevant parts of the source text when translating, leading to better quality translations, particularly in lengthy documents where context is crucial. Another benefit is the changing business model. Rather than exorbitant upfront costs, NMT systems are often priced on a usage basis, opening the door to high-quality translation for a wider range of individuals and smaller organizations. Moreover, advances in parallel processing have enabled NMT systems to handle large-scale video localization projects with much greater speed, as they can translate multiple text segments simultaneously. Finally, the integration of speech recognition into these translation pipelines opens up the possibility for multi-channel localization workflows. This allows for the simultaneous localization of audio, text, and video streams, significantly reducing overall turnaround times. This dynamic field of research shows great potential for improving the accessibility of information across languages.
AI Translation and the Precision of Timecode Enhancing Video Localization Workflows - Cost-Effective Localization Through Automated Workflows
Cost-effective localization through automated workflows is changing how businesses approach translation, especially in today's world where speed and accuracy are vital. Leveraging AI allows for the automatic processing of large quantities of content, which dramatically cuts down on the time it takes to complete projects without sacrificing quality. Furthermore, the use of machine learning and natural language processing automates repetitive tasks, minimizing the need for extensive human intervention and allowing for better resource management. As AI continues to improve localization, businesses are empowered to effectively reach global audiences by tailoring their messages to suit diverse markets. Nevertheless, the need for a balance between automation and human review is a vital aspect. Relying solely on AI systems could sometimes miss intricate linguistic details that require a human touch.
Thinking about localization costs, it's clear that automated workflows can offer significant savings. Some research suggests that using these methods can cut costs by as much as 60% compared to doing everything manually. This is quite appealing, especially for smaller companies that are looking to expand globally but might have limited budgets. The reduction in manual labor is also a key aspect here. These automated systems can drastically decrease the time needed to finish a localization project. Instead of spending dozens of hours on translation and related tasks, it's possible to condense this into just a few hours, freeing up teams to focus on other aspects of the work.
One interesting thing about these workflows is the ability to handle several languages simultaneously. This can really speed things up when delivering multilingual content, as it avoids the need for repeating tasks multiple times. It's also worth noting that the quality of machine translation keeps improving, and studies show that when these automated systems are combined with the newest translation models, the need for human post-editing can be significantly reduced by around 30%. This obviously has a huge impact on both time and cost. Real-time subtitling for live events is another exciting area where automation shines. Imagine the applications in live broadcasts or events where having subtitles synced with spoken content is essential for accessibility. The speed and accuracy that automation offers are far superior to more traditional methods in such cases.
Furthermore, the adaptability of AI-powered translation systems to different linguistic environments, including lesser-used languages, is noteworthy. This is particularly helpful in addressing the gap in resources for languages that haven't traditionally received as much attention. The precision in terms of subtitle synchronization is remarkable. Automated workflows can now align subtitles within milliseconds of dialogue, ensuring a far more seamless experience for viewers. This level of precision often outperforms older, manual methods which relied heavily on educated guesses. It's also worth observing that automation dramatically reduces errors. Some studies indicate a decrease in translation errors by up to 40% thanks to AI integration. This improved accuracy helps ensure the localized product's overall quality.
The ability to scale localization efforts is also a powerful benefit. These automated workflows can easily handle thousands of subtitles at the same time, which becomes incredibly valuable during peak seasons or for larger projects. Many automation systems now include customizable dictionaries, allowing users to add specific jargon, industry terms, or even regional variations. This ensures the localized content closely matches the expectations of the target audience. While there's still plenty of ongoing research and development in this field, these automated workflows have clearly demonstrated that they can help create more efficient and cost-effective solutions for localization—a vital element in a globalized world.
AI Translation and the Precision of Timecode Enhancing Video Localization Workflows - Real-Time Language Adaptation with Deep Learning Algorithms
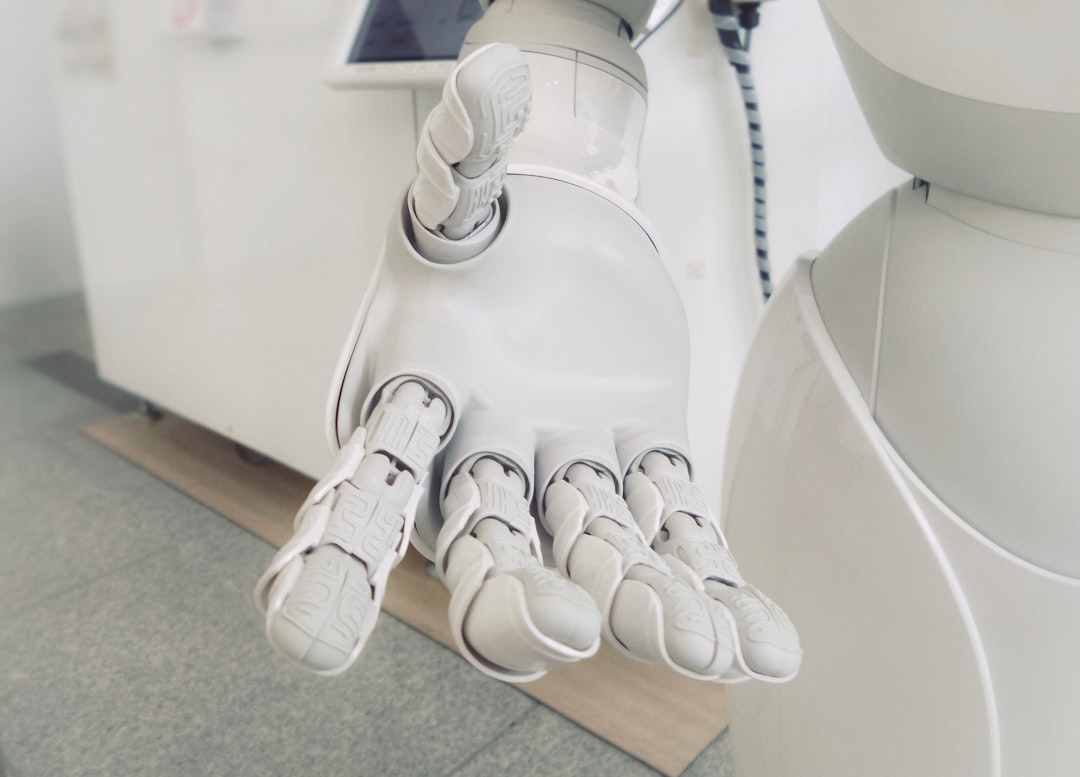
Real-time language adaptation using deep learning is rapidly evolving the field of AI translation, particularly for video localization. These algorithms, often incorporating neural networks, strive to provide immediate and precise translations, enhancing the fluidity of communication in diverse settings like live broadcasts and virtual gatherings. The development of techniques like Region-aware Temporal Graph-based Neural Networks is a prime example of this push, as it demonstrates the capacity for AI to accurately translate sign language in real-time, showcasing an attempt to bridge communication gaps for a broader range of users.
While promising, there are persistent hurdles to overcome, especially in consistently achieving contextual accuracy across the complexities of multiple languages. The continuous evolution of AI translation is not only about increasing speed, but also about consistently refining the overall quality of translated content. The ultimate goal is to foster a more interconnected and understanding global community, where language barriers are increasingly minimized through advancements in artificial intelligence. There is however a lingering concern about quality which is not addressed by simply improving speed. This remains a challenge that is being addressed but not yet solved in a way that everyone agrees is sufficient.
Deep learning algorithms, especially those using transfer learning, are showing promise in rapidly adapting to new languages. By leveraging data from well-resourced languages, these algorithms can quickly improve their performance for languages with limited data, making translation more accessible globally. Interestingly, the fusion of optical character recognition (OCR) and neural machine translation (NMT) creates a powerful multimodal approach, allowing the system to not only translate text but also understand visual cues within video content. This means translations can capture cultural nuances and deliver more context-sensitive results. Furthermore, deep learning has facilitated real-time translation collaboration, particularly for remote teams. Through cloud-based tools, multiple translators can now work on a project concurrently, making revisions and updates in real time, significantly accelerating the editing process.
This improved speed and collaboration is also facilitated by NMT models incorporating attention mechanisms. These mechanisms help the models focus on relevant parts of the source text, improving the accuracy of translations, especially in complex and lengthy texts. This focus on context is important because it helps minimize common translation errors that occur when models struggle to grasp the nuance of language. Furthermore, the cost-effectiveness of automated workflows driven by AI is quite notable. Studies show that automation can decrease localization costs by as much as 60%, making AI translation much more accessible for organizations of all sizes. Moreover, these automated systems can handle large amounts of content simultaneously, allowing organizations to scale their localization efforts effectively.
Beyond speed and cost reduction, the integration of OCR and NMT enables a system to dynamically adjust timecodes based on real-time speech recognition. This means subtitles are not just translated but also precisely synced with spoken dialogue, leading to a far smoother experience for the viewer. It's worth noting that research suggests that when these AI systems are used, the need for human post-editing can be reduced by up to 30%. This indicates that the quality of automated translation is improving, leading to higher-quality outputs and faster workflows. The improved robustness of modern AI algorithms also stands out. These algorithms show good performance in complex environments, such as video with poor lighting or fast movement, something that was a challenge for older translation technologies. These are critical improvements for maintaining subtitle integrity in diverse media formats.
It is also interesting that many contemporary translation tools allow users to build custom dictionaries for industry-specific terms and jargon. This means businesses can adapt the AI to better match their brand voice and the terminology relevant to their industry. This customization is a positive step towards ensuring the accuracy and consistency of translation. Finally, the advancements in real-time subtitling are significant, allowing live translation of events, like conferences and broadcasts, which are essential for both accessibility and audience engagement. This area of translation continues to mature, showcasing the potential of deep learning to break down language barriers in dynamic settings. While there's still ongoing research, these recent advancements in deep learning-based translation workflows are quite intriguing. There is a strong sense that these techniques will continue to enhance efficiency, quality, and accessibility of translation services in the years to come.
AI Translation and the Precision of Timecode Enhancing Video Localization Workflows - Multilingual Voice Cloning Preserves Original Speaker Tone
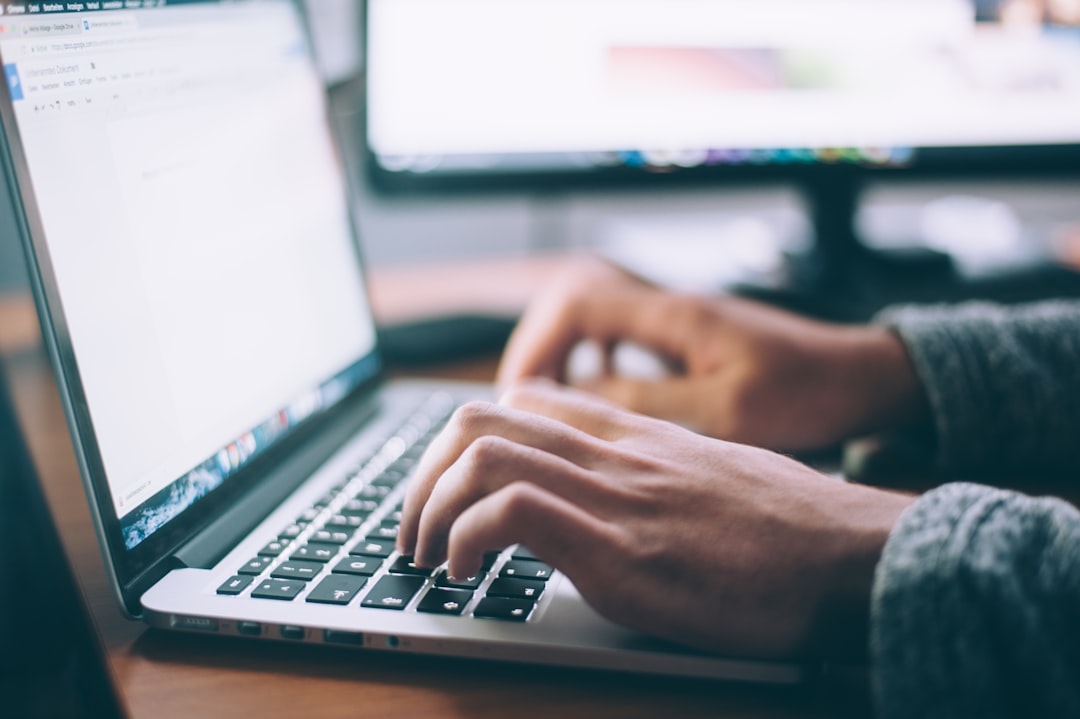
The ability of AI to clone a speaker's voice across multiple languages is a notable development in AI translation. These systems, powered by advanced neural networks, strive to mimic the original speaker's tone and style while delivering the translated content. This approach offers a more natural and engaging experience compared to traditional dubbing, preserving the emotional nuances and contextual richness of the original message. The prospect of accurately replicating a voice in multiple languages holds the potential to significantly alter how video localization is handled, fostering greater accessibility and cultural sensitivity in translated materials. While the potential is substantial, there are ongoing limitations, particularly in maintaining a high degree of accuracy with both the translation itself and the emotional conveyance of the cloned voice. This pursuit of natural-sounding, multi-lingual voice cloning is a promising area of research that could redefine audience engagement with translated content.
Recent advancements in AI translation are increasingly focused on replicating a speaker's voice across languages, maintaining the original tone and even emotional nuances. This "voice cloning" approach is fascinating because it strives to deliver translations that feel authentic and retain the speaker's unique character. It's achieved through sophisticated analyses of a speaker's phonetic patterns, allowing AI systems to essentially mimic their voice across languages. However, the quality and breadth of the training data used are crucial to the effectiveness of this technology. Models trained on a diverse range of voices and accents are better at adapting to new dialects, showcasing the importance of a comprehensive dataset.
One of the current challenges with voice cloning is the delay, or latency, in real-time scenarios. When it comes to applications like live broadcasts where simultaneous translation is needed, the speed and accuracy of the voice synthesis process are critical, and achieving both simultaneously can be tricky. Furthermore, the rise of this technology brings ethical considerations to the forefront, including concerns about consent and potential misuse of someone's voice. Ensuring that individuals' voices are not replicated without permission is vital as we navigate this emerging area of AI.
The interesting thing about some of these voice cloning technologies is their adaptability. They are not limited to just mimicking a speaker's voice in a neutral way. There's research on tailoring the outputted voice based on the translated content itself, adjusting parameters like age, gender, or even emotional state to provide a more personalized viewing experience. This raises questions about the impact on how translated content is perceived by viewers, as the delivery of information itself can be altered.
However, there are still limitations in voice cloning technology, particularly when it comes to replicating non-standard speech. Individuals with unique accents, speech impediments, or dialects require more specialized training data for the AI to accurately replicate their voices. This is a critical area for research, highlighting the need for AI systems that are inclusive and can accommodate a greater variety of speech patterns.
Voice cloning has the potential to disrupt existing workflows in creative industries like film and theater. Instead of needing actors to record lines in numerous languages, AI-powered voice cloning could streamline the process, reducing costs and accelerating localization. Moreover, these voice cloning systems can be integrated with other AI tools, such as OCR, to deliver seamless translations across multiple media types. And there's the potential for voice cloning technology to power interactive applications like voice bots in customer service, aiming to create more natural and contextually aware interactions for users.
It's a truly dynamic field, with promising developments and challenges alike. While the initial breakthroughs are exciting, it's also crucial to continue critically evaluating the ethical and practical implications of voice cloning to ensure responsible development and deployment. The potential benefits in bridging language gaps are undeniable, but equally important is ensuring that this innovation aligns with human values and respects individual autonomy.
AI Translation and the Precision of Timecode Enhancing Video Localization Workflows - AI-Powered Subtitle Generation Supports 280+ Languages
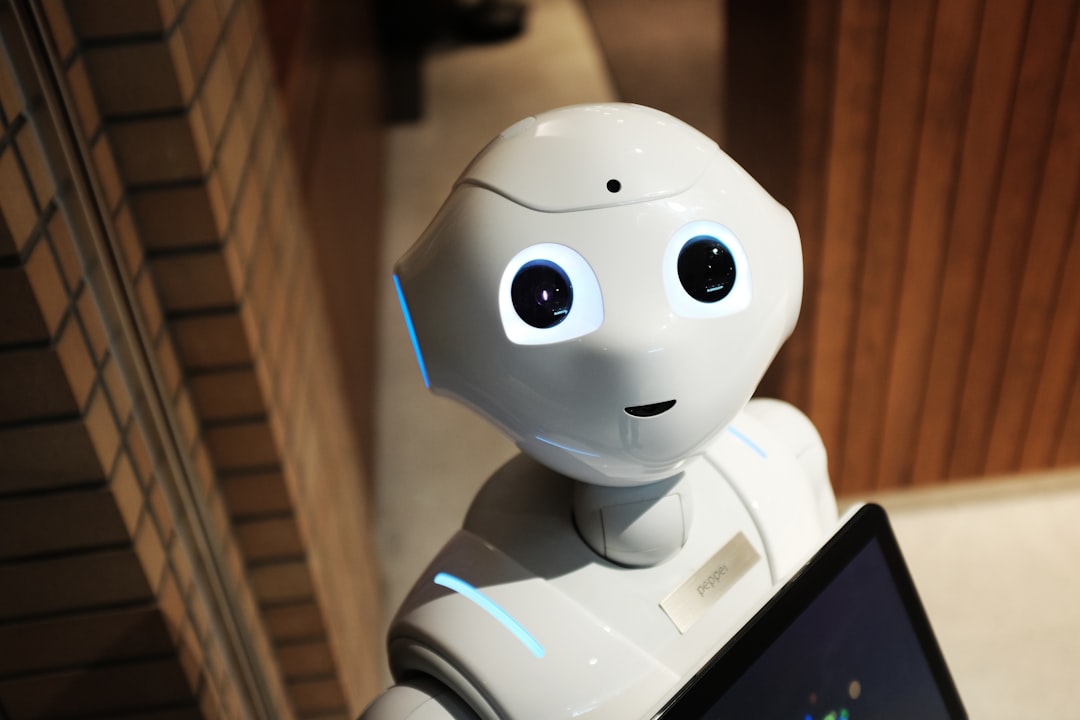
AI has made a significant impact on video subtitling by enabling the generation of subtitles in over 280 languages. This means that video content can now reach a far broader audience, breaking down language barriers. The speed at which AI can create subtitles is impressive, leading to faster turnaround times without sacrificing accuracy. Some services offer a centralized way to handle translation and localization, simplifying the process for users. It is now easier to upload videos from different platforms and quickly generate subtitles. The quality of the subtitles is also improving, with some services claiming near-perfect accuracy. However, it is still a technology that is evolving, and there's a potential for errors which might need human review. The use of AI to create subtitles allows for more accurate timecodes, resulting in subtitles that are better synchronized with the spoken dialogue. This enhanced synchronization improves viewer experience and can make videos more accessible to a wider range of viewers. Overall, AI-powered subtitle generation has greatly enhanced the efficiency and inclusivity of video localization. It's an area of AI development that is having a profound impact on how people access and consume video content around the world. While still evolving, these tools have made video localization more feasible and accessible to a much larger community.
The ability of AI to generate subtitles in over 280 languages is quite impressive, expanding the reach of video content to a truly global audience. It's not just about translating words anymore; AI systems are getting better at understanding different dialects and regional variations within languages. This development is especially interesting when considering the sheer variety of linguistic nuances across the world.
While the promise of real-time subtitling for live events is exciting, it also presents challenges in terms of maintaining accuracy and speed. Thankfully, AI systems have become much better at integrating speech recognition and handling fast-paced interactions with minimal delays. It seems that AI-powered subtitle generation offers a promising path toward greater accessibility for people with different language backgrounds.
However, a key aspect of AI-powered translation that researchers continue to work on is the need for contextual understanding. Traditional machine translation often falls short when it comes to properly interpreting the intended meaning of phrases, particularly when there's ambiguity. The fact that modern AI systems can incorporate context into their algorithms to a certain degree is promising, with some studies showing error reduction of up to 40%. But there's still a need for constant refinement of these systems to address nuanced language patterns.
Beyond just reducing error rates, AI subtitle generators are also integrating tools that allow users to customize their lexicons. This feature is useful for maintaining consistency in branding and terminology, particularly when translating content for specific industries or regions. This is a helpful tool that potentially helps these translation systems handle the vastness of terminology across industries and across different languages.
The inclusion of voice modulation within AI subtitle generation is an interesting development. It allows AI to mimic not just the spoken words, but also the tone and nuances of the original speaker in dubbed content. While we're not yet at the point where we can seamlessly replace a speaker's voice with a perfectly convincing AI replica across all languages, this area holds a lot of potential for more immersive and authentic video translation.
Researchers continue to improve AI's ability to quickly adapt to new languages using techniques like transfer learning. This approach utilizes data from languages that are well-studied to help "bootstrap" the learning process for less-common languages. This aspect of AI is promising in terms of broadening the reach of quality translation and helping with efforts to make language learning accessible for under-resourced languages.
Furthermore, these AI-powered systems can process and generate subtitles for multiple languages simultaneously, accelerating the localization of video content without significant losses in accuracy. It's impressive that these systems are able to balance speed and quality in such a demanding task.
The application of deep learning to continuously refine the translation models within these systems is promising. AI models are becoming increasingly complex, allowing them to understand the intricacies of grammar and language, which contributes to greater accuracy in translations.
It's encouraging to see how the efficiency of AI is helping to make translation services more accessible to a wider audience. The automation provided by these systems leads to cost savings of up to 60% for businesses, reducing the barriers to producing translated content. It's interesting to consider how much this decrease in the cost barrier can help accelerate the globalization of media and the exchange of information globally.
AI-Powered PDF Translation now with improved handling of scanned contents, handwriting, charts, diagrams, tables and drawings. Fast, Cheap, and Accurate! (Get started for free)
More Posts from aitranslations.io: