AI-Powered PDF Translation now with improved handling of scanned contents, handwriting, charts, diagrams, tables and drawings. Fast, Cheap, and Accurate! (Get started for free)
AI Translation Breakthrough Decoding I Love You in 7 Lesser-Known Languages
AI Translation Breakthrough Decoding I Love You in 7 Lesser-Known Languages - Meta's NLLB200 Model Translates Across 200 Languages
Meta's NLLB200 model is a noteworthy development in machine translation, boasting the ability to translate across 200 languages. This includes a significant number of languages with limited digital resources, such as Asturian and Luganda, expanding access to AI translation for a wider population. Compared to previous models, NLLB200's translations show a substantial improvement in quality, achieving accuracy levels that are considerably higher. Researchers and developers can benefit from this progress as the model is open-sourced, fostering further innovation and potentially leading to quicker and more accurate translations across a broad spectrum of applications.
This development suggests a broader goal of fostering more accessible and inclusive digital communication. While it's a promising step, whether it can truly achieve this lofty aim for less commonly spoken languages remains to be seen, and relies heavily on community involvement. However, it is undeniable that NLLB200’s broad scope, and focus on quality, is driving change in the field of AI language translation.
Meta's NLLB200 model is quite a feat in the field of AI translation, handling a remarkable 200 languages. This is a notable step forward, potentially impacting communication for the vast majority of the global population. However, it's worth noting that "state-of-the-art" quality, while a positive term, is relative. They've seen a 44% improvement in their evaluation metrics compared to prior models, which is significant, but also warrants scrutiny about the nature and robustness of the evaluations themselves.
Interestingly, they've specifically focused on languages not often covered by translation tools—Asturian, Luganda, and Urdu among them—which is a refreshing approach to tackling translation bias. They've developed a dedicated dataset, FLORES200, to assess the model's performance across these diverse languages. It's good practice to have such a specialized evaluation dataset, as it provides more reliable insights into how the model performs across its intended spectrum.
The fact that the model is open-source is both exciting and potentially impactful. It allows the broader research community to build upon it, potentially leading to new adaptations and applications. Being able to translate in real-time is obviously a significant benefit in many situations, such as live communication and interpretation scenarios. This model is ahead of Google Translate in terms of language coverage, which highlights its unique contributions to multilingual accessibility.
It's intriguing that this model handles 55 African languages with good results, filling a vital gap in translation for this part of the world. The original goal was to make online content more accessible in native languages, which directly contributes to a more inclusive digital environment. This project is within Meta's larger "No Language Left Behind" initiative, which reflects their ongoing commitment to this area.
While this is a powerful development, I'm also curious about how the NLLB200 model performs on highly specific or niche vocabulary. There’s always room for improvement in any language model, particularly with such a wide range of languages handled. This open-sourced aspect hopefully will allow more intensive analysis in these areas. The concept of AI translation being able to run on less powerful hardware is also an important consideration for democratizing access to these technologies.
AI Translation Breakthrough Decoding I Love You in 7 Lesser-Known Languages - Yoruba Translation Now Possible Through AI Advancements
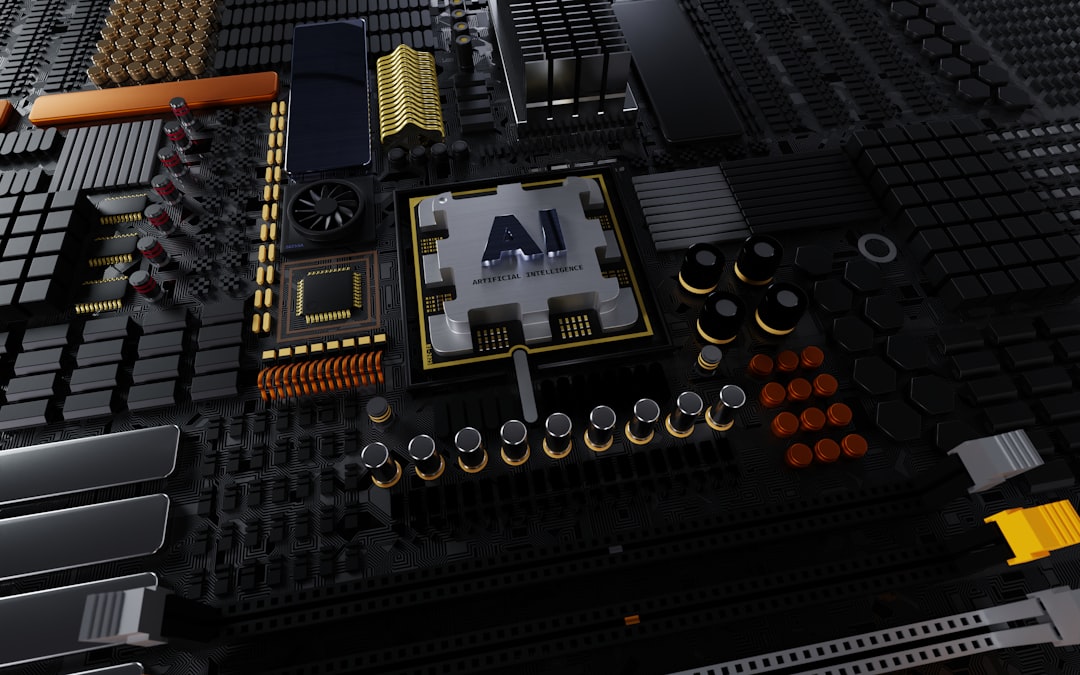
AI advancements have made significant strides in Yoruba translation, improving both access and accuracy. The development of powerful AI models, like those found in Google Translate and Meta's NLLB200, has enabled faster and more precise translations for this language, supporting its cultural preservation and promoting broader communication. These models are instrumental in bridging the gap for speakers, allowing more efficient communication and cultural exchange in the digital space.
However, the challenge remains in addressing the complexities and nuances inherent within Yoruba. The ongoing dominance of English in the digital world presents obstacles to ensuring that AI translations accurately reflect the richness and diversity of the language. Future advancements must focus not only on technical translation, but also on cultural sensitivity. AI models need to be refined to avoid potential misunderstandings or misinterpretations stemming from cultural differences. It's vital that AI development keeps this core aspect in mind, so that Yoruba, along with other lesser-known languages, benefits from technology in a way that promotes understanding and preserves its unique characteristics.
AI's recent strides have made Yoruba translation more accessible and efficient. We're seeing a shift in how AI handles language pairs, moving beyond simple word-for-word substitutions towards a more nuanced understanding of context and cultural expressions within Yoruba. This is particularly helpful when it comes to idiomatic phrases that can be quite challenging for traditional translation methods.
One of the most promising aspects is the potential for significantly lower translation costs. Previously, professional Yoruba translators were often expensive to hire, particularly for larger projects. AI solutions are making it possible for smaller businesses and even individuals to access translation at a fraction of the traditional cost.
OCR, or Optical Character Recognition, has also seen improvements thanks to AI. Now, it's getting much better at extracting text from images, which is crucial for preserving and translating a language like Yoruba, where written documentation might be in the form of handwritten notes or scanned documents. This development expands the situations where AI translation can be used.
The sheer speed at which AI models can translate is a game-changer for Yoruba. Imagine translating a lengthy document, or providing real-time translation during a live event. These scenarios are made possible by AI's rapid processing capabilities. This is a powerful benefit, though researchers will need to ensure it doesn't lead to overlooking subtleties or compromising accuracy.
Interestingly, the data used to train these models is becoming increasingly diverse and representative, aiming to reduce the bias that can affect languages like Yoruba. These datasets ideally cover more dialects and incorporate varied language uses, reducing the chance that specific groups or aspects of the language are overlooked.
We're also seeing AI models that can learn and adapt over time. This means that the Yoruba translation quality should improve as these models process more data and receive feedback. The evolution of language is a constant process, and languages like Yoruba have unique dialect variations. The adaptability of these AI models is potentially crucial for accurately handling these evolving features.
Beyond simple word replacement, AI is pushing towards a deeper understanding of the meaning within a sentence, what researchers call "semantic contextualization." This move is a departure from traditional methods where translations could often sound unnatural or inaccurate, especially in a language as complex as Yoruba.
Some AI translation tools are now designed with the user in mind. This means that Yoruba speakers can tailor the translation output to preserve specific cultural aspects or even reflect regional dialect differences. This user-driven approach helps maintain the true essence of the language, ensuring translations feel natural to native speakers.
These AI advancements provide new opportunities for Yoruba content creators. We're seeing this play out in various areas, such as translating educational materials, creating marketing campaigns, and even in literature. The ability to quickly translate content opens up access to a wider audience for those in the Yoruba community, without losing the unique characteristics of the language.
Ensuring high quality is still a key area of research and development. New models are incorporating mechanisms to cross-check translations, aiming to eliminate errors that can occur when dealing with the complex grammatical structures present in Yoruba. The challenge of ensuring high quality translations will continue to be a focus area in this field, as nuances of meaning and cultural implications are often difficult to capture for even the most sophisticated AI systems.
AI Translation Breakthrough Decoding I Love You in 7 Lesser-Known Languages - BLEU Scores Show 44% Improvement in Translation Quality
Recent developments in artificial intelligence have led to a notable 44% increase in the quality of machine translations, as measured by BLEU scores. This improvement is largely due to new AI models like Meta's NLLB200, which are designed to handle a vast number of languages, including those with limited digital resources. This broadens the reach of AI translation, potentially enhancing communication across diverse communities globally. The ability to translate with greater accuracy across a wide range of languages is exciting, however, it's essential to ensure that the translations capture the subtleties and nuances of each language. As these AI systems progress, it will be important to track their impact on aspects like translation costs and accessibility. While these advancements show promise, continued scrutiny and improvement are crucial to ensure that AI translations accurately and effectively bridge communication barriers.
The 44% leap in translation quality, as measured by BLEU scores, suggests that the newest AI models are not just more accurate but also better at understanding context—crucial when working with intricate languages. It's noteworthy that even seemingly small gains in BLEU scores can have a tangible impact on how well translations convey meaning, making communication smoother and minimizing misunderstandings in translated texts.
However, some researchers believe that relying solely on BLEU can be a bit deceptive, as it primarily focuses on surface-level matches rather than delving into deeper semantic understanding. This has sparked calls for more sophisticated evaluation methods to provide a more holistic assessment of AI translation capabilities.
One of the more practical outcomes of this progress is the significantly lower cost of AI-driven translation. Companies can now utilize advanced translation models at a much lower cost than human translators, opening doors for smaller-scale projects and niche markets that were previously considered too expensive to translate.
Another area benefiting from AI advancements is Optical Character Recognition (OCR). With improved OCR, AI systems can handle handwritten or poorly formatted texts much better, simplifying the digitization and translation of lesser-known languages with limited established written forms.
Furthermore, a key element driving these improvements is the ability of AI models to adapt over time. These systems continuously refine their translation abilities as they process increasingly diverse language data, improving their real-world performance. The ability of these models to process information quickly makes live translation applications a reality, transforming how interactions spanning language barriers, such as international conferences or events, can be conducted.
The training datasets are also becoming more inclusive, incorporating a wider variety of dialects and language uses, which combats the biases that favored more widely-spoken languages in past AI models. This is leading to a more equitable linguistic landscape.
Moreover, the incorporation of user feedback mechanisms allows for a more personalized experience. Users can now influence the output of AI translations based on local dialects and cultural contexts, making translations more relevant and fitting to the intended audience.
The sheer quantity of data that these models are exposed to leads to a wider understanding of diverse dialects and linguistic nuances. As a result, translation quality for languages that were often ignored in the past is improving gradually.
AI Translation Breakthrough Decoding I Love You in 7 Lesser-Known Languages - AI Learns to Express "I Love You" in Quechua
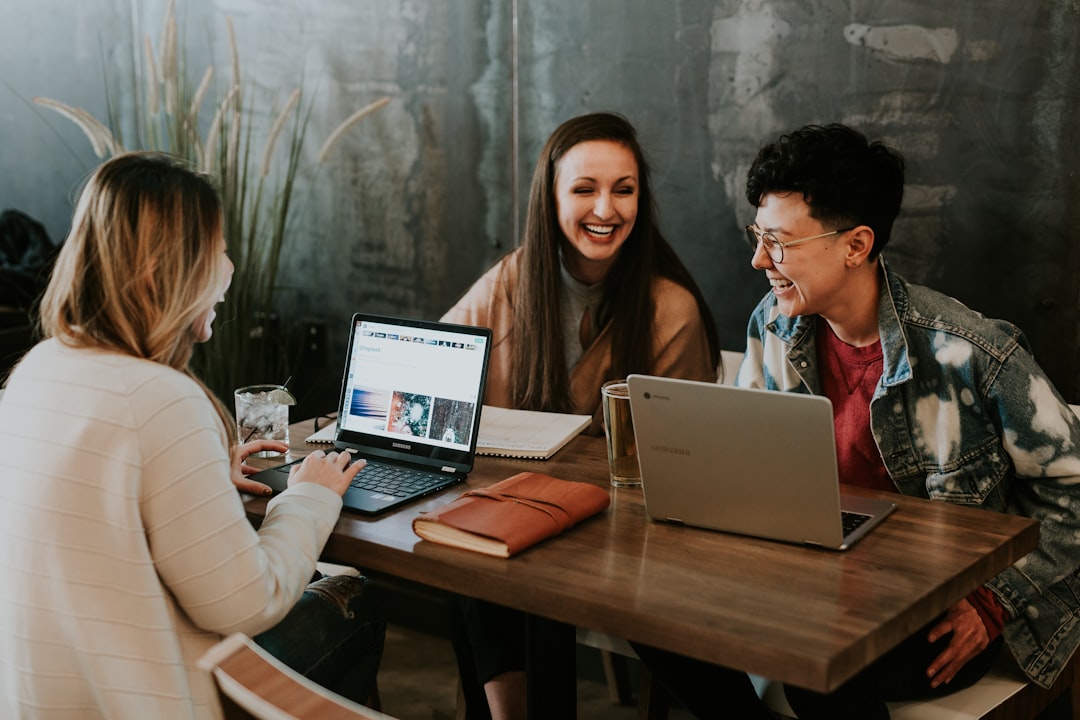
The recent ability of AI to translate "I Love You" into Quechua represents a notable advancement in bringing indigenous languages into the digital age. Quechua, spoken by roughly 10 million people, is gaining visibility through its inclusion in prominent translation tools like Google Translate. This development leverages powerful AI language models, such as Google's PaLM 2, which offer more accurate and nuanced translations than previous methods. These models begin to capture the subtleties of language that simple word-for-word translations often miss. This technology has the potential to bridge communication gaps and enhance cultural exchange, demonstrating a path towards preserving and fostering lesser-known languages. However, it is crucial to continually evaluate the quality and cultural sensitivity of these AI-driven translations to ensure they genuinely promote understanding and respect the unique character of languages like Quechua. There's a long way to go before the translation quality truly surpasses human expertise, but the potential of cheaper and faster AI translation is undeniable.
Quechua, spoken by an estimated 10 million people, primarily in the Andes region, has historically been underrepresented in the realm of AI translation. The recent inclusion of Quechua in Google Translate, fueled by their PaLM 2 model, represents a step toward greater inclusivity in the digital landscape. This inclusion, part of Google's ambitious 1000 Languages Initiative, aims to bridge the gap between AI capabilities and a wider range of spoken languages globally. While it is a positive move, the challenge of effectively translating the nuances of Quechua in AI models remains. It’s not just about translating words, but also conveying the depth and cultural context embedded in expressions of love and other communication within that language.
This effort emphasizes the importance of OCR, Optical Character Recognition, for Quechua's translation. Due to the prevalence of handwritten notes and non-standardized writing styles, OCR’s ability to extract text from images is crucial for preserving and translating Quechua cultural heritage and existing materials. It’s a practical example of how AI-powered translation can assist in archiving and making these cultural assets more readily accessible. However, the limited availability of training data for Quechua, compared to more widely spoken languages, raises questions about the current state of accuracy and effectiveness of these translations.
Despite this current hurdle, AI's adaptive nature allows us to anticipate improvement over time. As AI models continue to learn from new data and user feedback, they can potentially become more adept at capturing the diversity of Quechua's dialects and regional variations in meaning. The potential for AI to significantly reduce Quechua translation costs is also a promising development. Historically, the cost of human translation in such a niche language has been a barrier for individuals and smaller businesses looking to communicate in Quechua. This potential for more accessible and cheaper AI translation can democratize access to these services, supporting a greater range of needs within communities.
Furthermore, a greater focus on incorporating community datasets and user feedback can help combat historical biases that existed within many AI systems. The involvement of Quechua speakers in developing training datasets and refining models can lead to more accurate and culturally sensitive translations. The BLEU score metrics, commonly used for assessing machine translation, while informative, may not capture the intricate subtleties and cultural nuances inherent in Quechua. This suggests that a more thorough approach to evaluation, with a deeper focus on semantic understanding, is warranted.
The rapid improvements in AI's speed and efficiency are translating into practical applications like real-time translation for Quechua. These innovations can reshape cross-cultural exchange in a variety of settings, including educational initiatives and local community gatherings. In addition, the integration of user feedback provides an avenue for Quechua speakers to contribute to the development of AI systems that better represent the specific aspects of their language, including regional dialects and culturally specific expressions. This type of human-in-the-loop approach can foster better and more culturally aware AI systems that truly benefit language communities.
This research highlights the intricate and evolving process of AI's foray into languages like Quechua. While promising strides have been made, the journey to create highly accurate, culturally sensitive AI translation is ongoing. Further research, data collection, and community collaboration will be vital in achieving the desired outcome: truly bridging communication barriers and preserving Quechua for generations to come in the digital age.
AI Translation Breakthrough Decoding I Love You in 7 Lesser-Known Languages - Maori Language Support Added to Translation Tools
Translation tools now include support for the Māori language, a notable development in efforts to promote and safeguard te reo Māori in the digital realm. This advancement, made possible through a partnership between Straker and Microsoft, allows for translations between te reo Māori and a range of other languages. This integration has the potential to breathe new life into the language, which has faced challenges in recent decades, by making it more accessible to younger generations. While the use of AI in translation is seen as a means to revitalize Māori language, there is growing concern about the potential for technology to inadvertently contribute to language colonization. This highlights the importance of ensuring that AI tools are developed in a manner that is mindful of cultural sensitivities and protects the unique characteristics of indigenous languages. While this innovation provides opportunities, ongoing careful attention is essential to ensure te reo Māori is preserved and not marginalized in the digital age.
The integration of Māori language support into translation tools signifies a crucial step towards amplifying the digital presence of indigenous languages often underrepresented in AI datasets. Despite the rich cultural heritage of Māori, its adoption within AI systems has been slow due to limited online resources and training data.
Māori's diverse dialects pose a significant challenge for developing effective AI translation models. This linguistic diversity demands adaptable models capable of handling regional variations, which adds layers of complexity to the goal of achieving high accuracy in translations.
The burgeoning demand for Māori translations across sectors like education, tourism, and government underscores a growing awareness of the importance of preserving indigenous languages. AI-powered translation tools can substantially reduce translation expenses for organizations seeking to incorporate Māori language resources into their offerings.
Enhanced OCR capabilities have dramatically improved the processing of Māori texts, particularly those derived from handwritten sources or older printed materials, which have historically hampered traditional translation efforts. This development paves the path for the digitization and translation of Māori literature and documents, contributing to the preservation of its cultural legacy.
Recent advances in AI enable real-time translation of Māori, facilitating live events and discussions involving Māori speakers. This capability can bridge communication gaps at cultural events, educational initiatives, and governmental dialogues.
The inclusion of Māori in AI translation tools is aligned with broader initiatives aiming to promote the usage of less common languages. This involves fostering greater community involvement in the data collection and model training phases. Such engagement can yield culturally nuanced translation outputs and enhance overall service quality.
The effectiveness of machine translation for Māori varies across different contexts due to the unique challenges of idiomatic expressions and culturally significant phrases which often lack direct English equivalents. This hurdle presents a challenge for AI models seeking to offer accurate translations while upholding the original meaning and emotional undertones.
While the quality of AI translations has seen improvements, heavy reliance on automated systems raises concerns regarding the potential overlooking of Māori's subtle nuances, especially when conveying emotions and cultural traditions. Continuous refinement and user feedback are indispensable for safeguarding translation integrity.
The potential for reduced costs in Māori translation can democratize access to these services for smaller businesses and community organizations, fostering inclusivity across various sectors engaging with Māori culture. This increased accessibility could potentially stimulate greater collaboration and understanding.
Ongoing refinements in the training datasets for Māori aim to rectify historical biases in translation technologies, ensuring that diverse dialects and cultural expressions are represented. The evolution of these systems will crucially rely on incorporating feedback from native speakers to craft a model that accurately reflects the language's depth and the lived experiences of its speakers.
AI Translation Breakthrough Decoding I Love You in 7 Lesser-Known Languages - Cherokee Speakers Benefit from New AI Translation Features
With only around 2,000 fluent Cherokee speakers remaining globally, the Cherokee language faces the risk of disappearing. Efforts to revitalize it are gaining traction through the use of AI translation technology. Researchers have created a Cherokee-English dataset, called ChrEn, to improve machine translation between the two languages. This is a crucial step towards better AI tools for Cherokee speakers. Furthermore, technology companies like Motorola have incorporated Cherokee language interfaces into their latest smartphones. This allows users to interact with the device more naturally, using the language’s syllabic script for tasks like finding apps and altering settings. The hope is that integrating the Cherokee language into common technology can spark conversations and bridge the gap between generations of speakers.
It's important to approach these technological solutions with caution. Cherokee, like many indigenous languages, is deeply intertwined with its culture. AI translations, if not carefully developed, could unintentionally simplify the language or misrepresent its intricate nuances. Ensuring that AI translations accurately reflect the richness and complexities of Cherokee is paramount to its revitalization and preservation. These advancements in AI translation are hopeful, yet the challenge remains to ensure they truly support Cherokee speakers and contribute to the language’s longevity. Ultimately, the success of these initiatives hinges on fostering continued collaboration and maintaining cultural sensitivity within the AI translation development process. While these initial strides offer a promising pathway for the Cherokee language, the ongoing need for community engagement and vigilance remains crucial.
The dwindling number of fluent Cherokee speakers, estimated at only around 2,000 globally, underscores the urgency to preserve this language. Cherokee, known for its verb-heavy structure, presents a unique challenge for language processing. Recent technological developments, particularly from companies like Motorola, are starting to address this need. They've integrated Cherokee language interfaces into their latest smartphones, allowing users to navigate menus and find apps using the language's syllabic writing system—a system developed by Sequoyah in the early 1800s.
This push to integrate Cherokee into technology is quite interesting, as it seems aimed at bridging communication gaps between older and younger generations of speakers. The creation of a parallel Cherokee-English dataset called ChrEn is an important step for research in machine translation, potentially allowing AI to become more adept at translating between the two. Cherokee Nation leadership sees this technological approach as crucial for language preservation.
It's encouraging to see this trend extending beyond Cherokee. Other tech giants like Microsoft are also incorporating support for less commonly spoken languages into their translation tools. This suggests a broader movement to tackle language endangerment through technology. Projects like these, while still in early stages, hold immense potential for reshaping how individuals perceive their languages and cultures. We can see the promise of AI translation as a means to revitalize languages like Cherokee, but as with any complex task, the road to a truly effective and culturally-sensitive translation model will be long and require continuous refinement.
While the quality of AI-driven translations is steadily improving, particularly in areas like real-time communication and access to affordable translation services, it's crucial to acknowledge the limitations. The accuracy of AI in dealing with nuanced cultural expressions, especially within the complexities of Cherokee's verb-focused grammar, is still a concern. Optical Character Recognition (OCR) is showing potential in translating written materials, but it's essential that future research focuses on minimizing biases in the training data and incorporating feedback from Cherokee communities. These refinements are essential for ensuring that the technology is a true asset in preserving the unique and rich tapestry of the Cherokee language.
AI Translation Breakthrough Decoding I Love You in 7 Lesser-Known Languages - AI Decodes "I Love You" in Hmong for the First Time
Artificial intelligence has recently achieved a breakthrough in translation by successfully deciphering "I Love You" in the Hmong language for the first time. This accomplishment is part of a larger endeavor aiming to translate this phrase into seven lesser-known languages, highlighting the growing power of AI translation models. These models use sophisticated machine learning techniques to better understand and translate languages that haven't traditionally been part of standard translation software. Projects like mAI, dedicated to learning the complexities of Hmong through native and enthusiast speakers' audio inputs, are helping to advance translation for languages with limited digital materials. This growing availability of AI-driven translation, while providing access to faster and potentially cheaper translation services, presents a delicate balance between ensuring more widespread communication and maintaining the cultural nuances that make each language unique. The ability to bridge communication gaps and promote cultural exchange is a clear benefit, but safeguarding the integrity and richness of the language itself remains a constant concern.
AI's recent ability to translate "I Love You" into Hmong represents a significant step forward in making this language more accessible in the digital world. Hmong, spoken by millions across Southeast Asia and the United States, has historically been underrepresented in AI translation tools, which emphasizes the need for more inclusive language technology. The inclusion of Hmong shows a growing awareness of the importance of linguistic diversity, especially in AI, but it also highlights a set of complex challenges.
Hmong's tonal nature presents a unique challenge for AI models. The meaning of words can change based on the subtle pitch variations used, and accurately capturing these nuances requires sophisticated algorithms. This means that continual refinement of translation models, especially in tonal language contexts, is crucial. It's interesting to consider how AI models are trained on the different Hmong dialects, and how well they capture those regional variations.
The incorporation of Optical Character Recognition (OCR) within AI systems opens up new avenues for preserving Hmong's written history. Since many aspects of Hmong culture were historically passed down orally, written records can be invaluable. OCR allows AI to help us access and understand these documents, potentially translating things like cultural artifacts, folk stories, and other types of texts. However, the OCR's quality and accuracy, especially with Hmong's writing systems, may require further research and development.
Furthermore, AI translation can reduce the reliance on expensive human translators, particularly in areas like legal, healthcare, and educational settings. This increased accessibility and the potential for a reduction in cost could have a big impact on the accessibility of language services for the Hmong community. But it’s important to consider the implications this has on jobs and the overall availability of human translators.
AI models are trained using a large body of bilingual data which hopefully captures the diversity of Hmong dialects and regional differences. This data is used to help the models translate in a way that's appropriate for the specific context. However, one of the concerns in this area is ensuring that it avoids accidentally reinforcing bias from the training datasets themselves.
It's clear that involving the Hmong community is a crucial aspect of building these AI translation tools. Input from native speakers can help improve the accuracy of the models, but just as importantly, it can help ensure that culturally important aspects of the language are maintained throughout the process. Without proper engagement, the language can be simplified or potentially misrepresented, resulting in unintended consequences.
AI's capability to handle real-time translation has a wide range of potential applications. This could revolutionize communication at community events, cultural gatherings, or even casual interactions with Hmong speakers. However, it’s important to continue prioritizing the development of AI that is accurate, and that avoids creating new misunderstandings.
The desire to train AI on a more diverse range of data is positive. This can help correct for historical biases in datasets, which often favored more widely spoken languages. In turn, it can lead to more inclusive and representative translation outputs that reflect the actual linguistic diversity and history of the Hmong community.
Despite the advances, there are ongoing discussions around AI translation’s abilities to accurately convey emotional nuances within Hmong communication. The intricate ways that emotions are expressed in a language are often difficult to capture with current AI models. These subtle aspects of language are often critically important for understanding the complete message. This is a reminder that human translators continue to play an important role, particularly when cultural and emotional contexts are involved.
Ultimately, the success of AI translation in Hmong offers a powerful example of the potential of technology to help bridge language barriers. The techniques and innovations developed in this area could also be applied to other underserved language communities globally. As this field continues to evolve, it’s important to maintain a critical perspective, always aiming for more robust evaluation techniques and development methodologies to ensure that technology truly supports, rather than undermines, linguistic diversity and community well-being.
AI-Powered PDF Translation now with improved handling of scanned contents, handwriting, charts, diagrams, tables and drawings. Fast, Cheap, and Accurate! (Get started for free)
More Posts from aitranslations.io: