AI-Powered PDF Translation now with improved handling of scanned contents, handwriting, charts, diagrams, tables and drawings. Fast, Cheap, and Accurate! (Get started for free)
AI Translation Breakthrough Improving Accuracy for Filipino Regional Languages
AI Translation Breakthrough Improving Accuracy for Filipino Regional Languages - Meta's NLLB model boosts accuracy for 200+ languages
Meta's No Language Left Behind initiative, spearheaded by the NLLB200 model, signifies a major leap in AI translation. This model tackles the challenge of accurate translation across over 200 languages, with a specific focus on languages often overlooked by existing technologies. Evaluations have shown an impressive 44% average accuracy improvement over previous translation models. This is particularly impactful for lesser-known languages like those found in Africa and India, some exhibiting over 70% accuracy gains.
The potential impact on communication is substantial, as it can break down language barriers for communities previously underserved by readily available translation tools. This, in turn, promotes access to information and greater participation in online spaces. By releasing NLLB200 as open-source, Meta encourages further development and applications, potentially benefiting a wide array of fields. While still in its early stages, this AI model's impact on fostering a more inclusive digital landscape holds great promise. It remains to be seen how effectively this model can address complex nuances within certain language groups, but its potential is undeniable.
Meta's NLLB-200, a new neural machine translation model, is a step forward in tackling the challenge of bridging the language gap across a vast spectrum of languages, particularly for those with limited digital resources. This model, capable of handling over 200 languages, boasts a substantial improvement in translation accuracy, especially for languages like those found in Africa and India, where gains of over 70% have been recorded. The model's success is in part due to the creation of the FLORES-200 dataset, a massive collection of text pairs specifically designed to benchmark translation quality across numerous language pairings.
Interestingly, NLLB-200 can perform "zero-shot" translation, which means it can handle language pairs it hasn't been explicitly trained on. This adaptive capability offers a fascinating potential for translating niche dialects and language variations that previously lacked accessible translation technology. While this adaptability is promising, there's also the need to be aware of how this approach might impact accuracy in certain cases.
A core aspect of the NLLB-200 design is its focus on lower-resource languages. Previous models frequently struggled with these languages due to their limited presence in training data. NLLB-200, however, explicitly addresses this by incorporating these languages into its data balancing strategy, effectively ensuring they're not overlooked during training.
Further, Meta's open-sourcing of NLLB-200 means researchers can leverage it to explore its capabilities and potentially develop even more sophisticated tools for language understanding and translation. This openness also aligns with Meta's "No Language Left Behind" initiative, suggesting an aim to ensure AI-driven translation tools benefit a wider range of speakers.
However, while impressive, AI translation models like NLLB-200 aren't without limitations. The challenge of balancing technical accuracy with culturally nuanced language remains a hurdle. Even with its enhanced features like context understanding and error correction, the final output needs careful review for specific contexts and dialects, where human expertise can be crucial to prevent potential misinterpretations or cultural faux pas. This highlights the need for a balanced approach to using AI-driven tools, acknowledging both their capabilities and the human element still required for truly accurate and culturally sensitive translations.
AI Translation Breakthrough Improving Accuracy for Filipino Regional Languages - 44% average BLEU score improvement across 10,000 language pairs
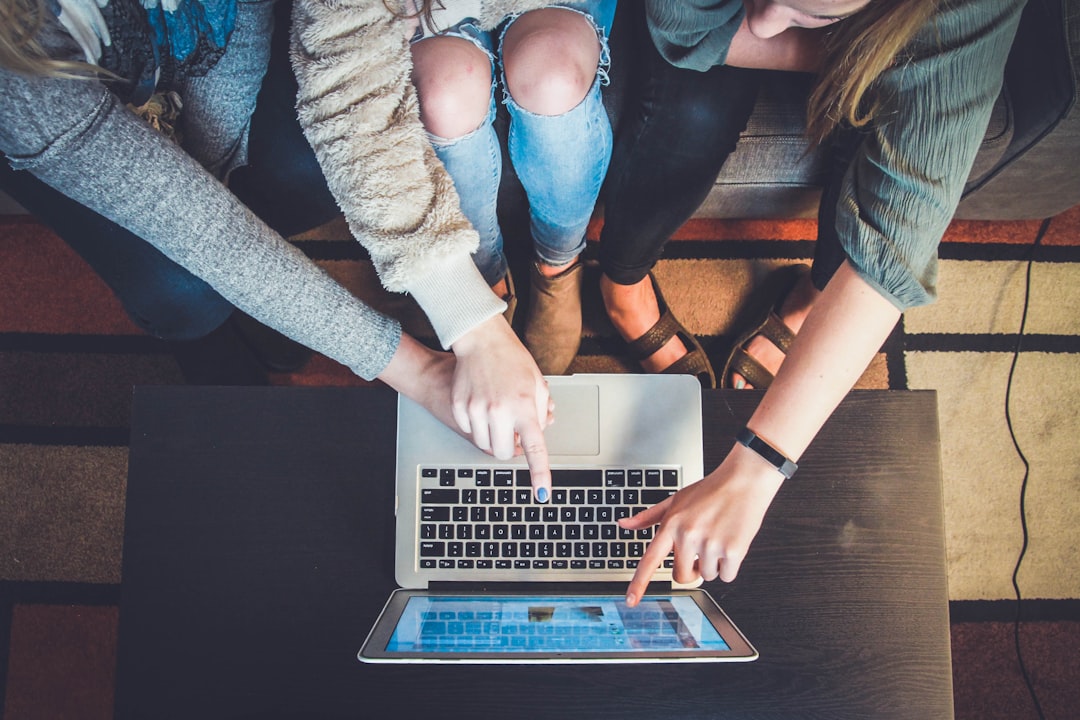
A recent breakthrough in AI translation technology has resulted in a substantial 44% average improvement in BLEU scores across a vast range of 10,000 language pairs. This represents a significant leap in the accuracy of machine translation. The progress is particularly notable for languages that have historically received limited attention from existing translation models, including various Filipino regional languages. These languages, often lacking large datasets for training, have been a challenge for AI systems.
While the advancements shown by the NLLB200 model are promising, it's important to acknowledge that AI translation is still an evolving field. The ability to accurately translate not just words but also the subtle cultural nuances within languages continues to be a challenge. The question of how to achieve both technical precision and cultural sensitivity remains a key area of discussion as these technologies progress. Despite this, the potential for these tools to foster better communication across language barriers and promote inclusivity within the digital world is substantial. It's also vital to remember that the role of human expertise in verifying and refining translations, particularly in complex or culturally sensitive contexts, remains crucial.
The 44% average BLEU score improvement across 10,000 language pairs is quite striking. This indicates a substantial leap in the model's ability to generate translations that are closer to human-quality reference translations. It's fascinating to see how this improvement spans such a wide range of language pairs, suggesting the model has a robust understanding of diverse linguistic structures. However, it also highlights that translation quality can vary greatly based on the language combination, hinting at the potential for further refinements in specific areas.
The model's ability to translate language pairs it hasn't explicitly been trained on (zero-shot translation) is truly intriguing. This capability could be particularly useful for translating less-common languages and dialects that often lack readily available translation tools. This kind of adaptability could be instrumental in integrating with Optical Character Recognition (OCR) systems, potentially allowing for faster and more accurate translations of printed documents.
It's encouraging to see the focus on lower-resource languages. This model's success in improving translations for them suggests that it's efficient with data, possibly needing less training data compared to earlier models. This aspect is especially relevant in helping to preserve linguistic diversity, as it ensures these languages aren't sidelined in the digital realm.
The potential implications of this increased accuracy are vast. Imagine the impact on real-time translation tools like instant messaging or social media platforms. Enhanced accuracy could remove significant communication barriers, making it easier for people across diverse language backgrounds to interact.
Of course, we also need to remain cognizant of the inherent limitations of AI translation. Despite impressive improvements, context and cultural nuances can still be tricky. While the model can handle aspects like context and error correction, sensitive topics or specialized dialects may need a careful human review to ensure cultural appropriateness and avoid potential misunderstandings. There's still a need for a balance between AI capabilities and human expertise in certain situations.
Looking forward, it's easy to envision a future where AI-powered translation tools like this enhance user engagement across different languages. People might be more inclined to participate in online forums and discussions if they can overcome language barriers. And for educational purposes, these improved tools could open up access to learning materials in a wider range of languages, promoting inclusivity and improving the quality of education across different cultural contexts. It's exciting to see how these developments continue to evolve and their implications for the future of communication and education.
AI Translation Breakthrough Improving Accuracy for Filipino Regional Languages - AI translation tackles unique challenges of Filipino dialects
Artificial intelligence (AI) in translation is actively tackling the complex challenges presented by the numerous dialects found in the Philippines. This is crucial not just for boosting the precision of communication, but also for successfully capturing the delicate cultural nuances woven into these regional languages. Often categorized as low-resource languages, Filipino dialects face significant hurdles, namely their limited online presence and scarcity of written materials. This makes it difficult for AI systems to efficiently translate them, creating a need for continued advancements. Researchers are diligently working on tailoring AI translation to accommodate these particular linguistic characteristics while ensuring that the translations are accurate and respect the cultural context. As this field progresses, the hope is that it will promote greater understanding and engagement within communities using these dialects, thereby fostering a sense of inclusivity.
AI translation is facing unique challenges when it comes to Filipino dialects. The intricate nature of these dialects, with their subtle nuances, idioms, and colloquialisms, makes accurate translation a complex task. Some dialects lack a standardized written form, further complicating the process for AI systems.
The issue of limited digital resources is a significant hurdle for many Filipino dialects. These languages are often classified as "low-resource," meaning there's not a lot of readily available digital text for AI models to learn from. This lack of data can lead to poor translation quality. The NLLB model's inclusion of these languages in its training data is a promising step toward addressing this challenge.
The NLLB-200 model's ability to perform zero-shot translation, handling language pairs it hasn't been specifically trained on, offers intriguing possibilities for less-common Filipino dialects. This feature could greatly expand the potential for communication and translation for speakers of these dialects.
However, just translating words isn't enough. AI must also understand the cultural context embedded within Filipino dialects. A word or phrase can have significantly different meanings depending on the region or social context, making cultural sensitivity a crucial aspect of accurate translation.
Integrating AI translation with Optical Character Recognition (OCR) systems holds great promise. It could dramatically speed up the process of translating printed materials written in various dialects, especially for documents that haven't been digitized yet.
The impressive accuracy gains shown in the NLLB-200 model indicate a shift toward more efficient machine learning techniques. This could mean that future AI models might require less training data for Filipino dialects, resulting in a more efficient use of resources.
Filipino speakers often switch between different languages and dialects during conversations, a phenomenon known as code-switching. AI models need to adapt to this dynamic to capture the context and flow of communication effectively. This is a tricky area that requires further research and development.
The 44% improvement in BLEU scores is a significant metric, suggesting the model has made progress in understanding Filipino grammar and syntax. However, the BLEU score might not fully capture the richness and subtlety of meaning crucial for meaningful communication in natural conversation.
Enhanced translation capabilities could greatly increase access to information and participation in society for those who aren't native speakers of certain Filipino dialects. This could encourage participation in education, cultural events, and even government activities, potentially leading to a more inclusive society.
Despite the impressive advancements in AI translation, the need for human oversight remains. AI systems can still struggle with understanding cultural context, which can lead to unintended errors or culturally insensitive translations. In such cases, human expertise in the specific dialect is vital to ensure accuracy and appropriateness.
AI Translation Breakthrough Improving Accuracy for Filipino Regional Languages - Zeroshot capabilities enhance Philippine language translations
AI translation is increasingly able to handle languages it hasn't specifically been trained on, a capability known as zeroshot translation. This is particularly exciting for Philippine languages, many of which have limited online resources and haven't been a focus in traditional AI training. Zeroshot translation allows AI models to translate between these languages without needing vast amounts of paired examples. The core of this technique involves teaching AI to understand the underlying patterns across numerous languages, effectively allowing it to "guess" at how a language pair should translate. This approach is especially valuable for the diverse linguistic landscape of the Philippines, where numerous regional languages and dialects exist. While this ability to translate unseen language pairs is very promising, it's important to remember that these systems are still learning. The nuances and cultural complexities within different dialects pose a continuous challenge for AI. The ultimate goal is to not only translate accurately but also sensitively, avoiding unintended misinterpretations that can stem from cultural differences. Achieving this requires continued work to refine these AI models and carefully consider the potential impact on various communities. Despite these challenges, zeroshot translation has the potential to become a significant tool in fostering better communication and inclusivity across the Philippines, bridging language barriers and promoting a more connected society.
The NLLB-200 model's zero-shot capabilities are proving quite useful for translating Filipino dialects without the need for extensive prior training on each specific language pair. This efficient approach could speed up translations in areas where these dialects are prevalent, improving communication in underserved communities. However, it's also a reminder of the challenges AI faces in truly understanding cultural nuances and idiomatic expressions within these languages. A straightforward translation might miss the intended meaning, which emphasizes the continued need to refine AI training to capture these cultural contexts more accurately.
Integrating AI translation with OCR technology holds a lot of potential, especially for translating printed documents in Filipino dialects. This pairing could accelerate the translation process and provide easier access to information from documents that haven't yet been digitized.
Interestingly, the model seems to need less training data while still improving accuracy. This is quite significant for lower-resource languages like Filipino dialects that don't have a large digital footprint. By using techniques like transfer learning, it can still achieve accuracy improvements, making sure these languages aren't ignored in the digital sphere.
Filipino speakers are known for blending different languages in their conversations, something called code-switching. It's fascinating to see how well the NLLB-200 model handles these shifting language patterns. It highlights the potential for more natural and context-aware translations, but also poses a challenge for the models.
While a 44% increase in BLEU scores is impressive, and suggests good translation accuracy, it's worth noting that these scores primarily measure surface-level similarities. The deeper aspects of communication, like capturing context and intent, might still need human refinement to ensure nuanced meaning.
Improved translation tools could open up opportunities for access to information and governmental resources for speakers of various Filipino dialects. This increased access can empower communities by providing them with the information they need to participate more actively in society.
These developments might pave the way for real-time translations in communication tools like messaging apps or social media platforms, making it much easier for people across different Filipino dialects to interact with each other, effectively reducing language barriers.
However, it's important to recognize that many of these dialects lack a standardized written form, and there's limited online content available. This scarcity makes it difficult to train AI models effectively, necessitating more innovative approaches to creating language resources to improve the quality of translations.
Finally, even with these advancements in AI translation, the human element remains crucial, especially in situations that are sensitive or culturally nuanced. AI models can sometimes misinterpret cultural references or expressions, underscoring the importance of human reviewers who can ensure translations are both accurate and culturally appropriate.
AI Translation Breakthrough Improving Accuracy for Filipino Regional Languages - AI models address concerns of language loss in digital spaces
AI models are increasingly addressing the risk of language loss within digital spaces, particularly focusing on those languages with limited digital resources, such as various Filipino dialects. These models are employing innovative techniques, like zero-shot translation, to help languages that lack extensive training data, thereby increasing their visibility and significance in the digital realm. This not only opens up new avenues for communication for speakers of less-common languages but also contributes to preserving overall linguistic diversity in our interconnected world. Although promising, the challenge of accurately conveying the subtle cultural nuances embedded within these languages continues to be a critical factor that necessitates ongoing review by humans and continuous model refinement. Ultimately, these AI models hold the promise of bridging language barriers and fostering greater inclusion across the digital landscape.
AI models are starting to address the challenges of language loss in digital spaces, particularly for languages with limited digital representation like many Filipino dialects. The development of models like NLLB-200 has spurred the creation of specialized datasets that capture the unique features of these dialects, helping AI better understand their structure and meaning. This targeted approach helps the models learn the nuances of these languages more effectively.
It's becoming increasingly clear how important it is to address the issue of low-resource languages. Languages like those spoken in parts of the Philippines are often categorized as low-resource because there's just not a lot of written content available for AI systems to learn from. This lack of data makes it difficult to get accurate translations, and finding ways to develop effective AI solutions that work even with minimal data is crucial if we want to help preserve these languages.
One fascinating development is the integration of AI translation with OCR, or Optical Character Recognition. This could be a game-changer for translating documents in Filipino dialects, especially historical or less digitized documents. AI translation, when combined with OCR, can help us make a lot more printed information accessible in these languages and potentially close some information gaps.
Zero-shot translation, the ability of AI models to translate between language pairs they haven't been specifically trained on, is showing exciting promise. It's particularly valuable for dialects with limited digital resources, as it can accelerate the development of translation tools without requiring massive amounts of training data for every single pair. This is really speeding up the process of making communication easier for speakers of these dialects.
Looking at communication in real-time, these improved AI models are paving the way for faster and better translations in messaging platforms and social media. This could break down many language barriers and make it easier for individuals from different dialect communities to interact.
Interestingly, Filipino speakers frequently switch between different languages and dialects during a single conversation—a process known as code-switching. AI models are starting to incorporate this aspect of communication, which is a big challenge. If models can effectively handle code-switching, it'll result in translations that are much more natural and reflective of how people actually speak.
While metrics like BLEU scores show impressive improvements in AI translation, we should be mindful that these scores often measure surface-level accuracy. The subtle nuances, the true heart of language, are still a hurdle for AI. Models need to become better at understanding context and intent in order to convey meaning more accurately.
AI translation models, however, can be an important tool for the preservation of languages, especially those at risk of being lost. By making them more accessible online, AI can help keep these languages alive and encourage younger generations to continue learning them, preventing them from fading away in the digital landscape.
Even with the progress being made, the need for human involvement remains vital. It's particularly critical in culturally sensitive situations, where AI might not fully capture context or nuances. Humans, with their knowledge of language and culture, are necessary to ensure that translations are not only accurate but also culturally appropriate, preventing any unintended miscommunication.
AI translation is undoubtedly a rapidly developing field with significant potential to reshape the way we communicate in a globally connected world. The future of these technologies holds potential for positive change, but it's also important to remain aware of the inherent complexities and continue to work towards achieving truly nuanced and respectful communication across all languages.
AI-Powered PDF Translation now with improved handling of scanned contents, handwriting, charts, diagrams, tables and drawings. Fast, Cheap, and Accurate! (Get started for free)
More Posts from aitranslations.io: