AI Translation Challenges in Adapting War of the Worlds Broadcasts From 1938 Radio to 2024 Global Streaming
AI Translation Challenges in Adapting War of the Worlds Broadcasts From 1938 Radio to 2024 Global Streaming - Converting 1938 Radio Static to Digital Audio Files Without Loss of Historic Atmosphere
Transforming the 1938 "War of the Worlds" broadcast from its original analog format into digital audio files requires careful consideration to avoid sacrificing the broadcast's unique, historical ambiance. The goal is to modernize the sound without losing the raw, somewhat crude quality that contributed to its impact. Methods like adjusting the equalization and evening out the sound levels help to retain the authenticity of the original broadcast—a broadcast that, despite its technical limitations, fully immersed listeners in its story. As we prepare this classic broadcast for today's streaming world, we face the delicate task of balancing the capabilities of AI and modern audio technology with the need to maintain the broadcast's original power and emotional resonance in a dramatically different media landscape. This endeavor clearly demonstrates the constant push and pull between technological advancement and the need to protect cultural treasures, ensuring that the original experience of this iconic broadcast—a broadcast that once ignited widespread panic—remains accessible for future generations to appreciate.
1. Converting the crackly 1938 radio broadcasts into digital audio files demands clever noise reduction methods. However, the real trick is to hold onto the quirks that give the recordings their unique personality – the wavering frequencies and uneven modulation, all part of the original sound.
2. Audio engineers often use sophisticated software that can differentiate between human speech and the background noise, enabling a more precise extraction of the original dialogue. It's important to remember these broadcasts were often recorded and received under less-than-perfect conditions.
3. The digitization of these vintage broadcasts can unearth interesting historical details. For instance, particular static patterns are linked to the geographical areas where the broadcast was received, giving us a glimpse into the listening environment of that time.
4. AI-based transcription and translation programs are remarkably adept at deciphering spoken language. But they need careful fine-tuning to understand the unique slang and language quirks of the 1930s if they are to translate the broadcasts accurately.
5. Some researchers have tried employing OCR to digitize the original broadcast scripts. However, the quality of these old, faded documents adds another layer of difficulty, as the damage and age can easily mislead any translation process.
6. It's easy to overlook the importance of the link between sound quality and a broadcast's ambiance. Overzealous digital editing can strip away the historical context, creating a listening experience that feels out of place in its own time.
7. The emergence of lossless audio formats is critical for preserving sound quality during conversion. But their incompatibility with certain compression techniques presents a risk to the integrity of the original audio data.
8. For AI translation tools to effectively bridge language barriers, they must consider the tone and broader context of the 1938 broadcast. The humor and satire of that era were often conveyed in ways that might be misinterpreted or lost entirely in a modern context.
9. Various studies have shown that even slight alterations to audio playback speed can change the emotional impact of a historical broadcast. As a result, accuracy during the conversion process is paramount to maintaining the atmosphere of the original recording.
10. While many tools claim to offer "fast translation," the complexities and cultural nuances embedded within a 1930s American radio program illustrate the limitations of such quick methods. Human oversight remains essential to ensure the accuracy of both the language and the original meaning.
AI Translation Challenges in Adapting War of the Worlds Broadcasts From 1938 Radio to 2024 Global Streaming - Machine Learning Models Tackle Period-Specific American English From Roosevelt Era
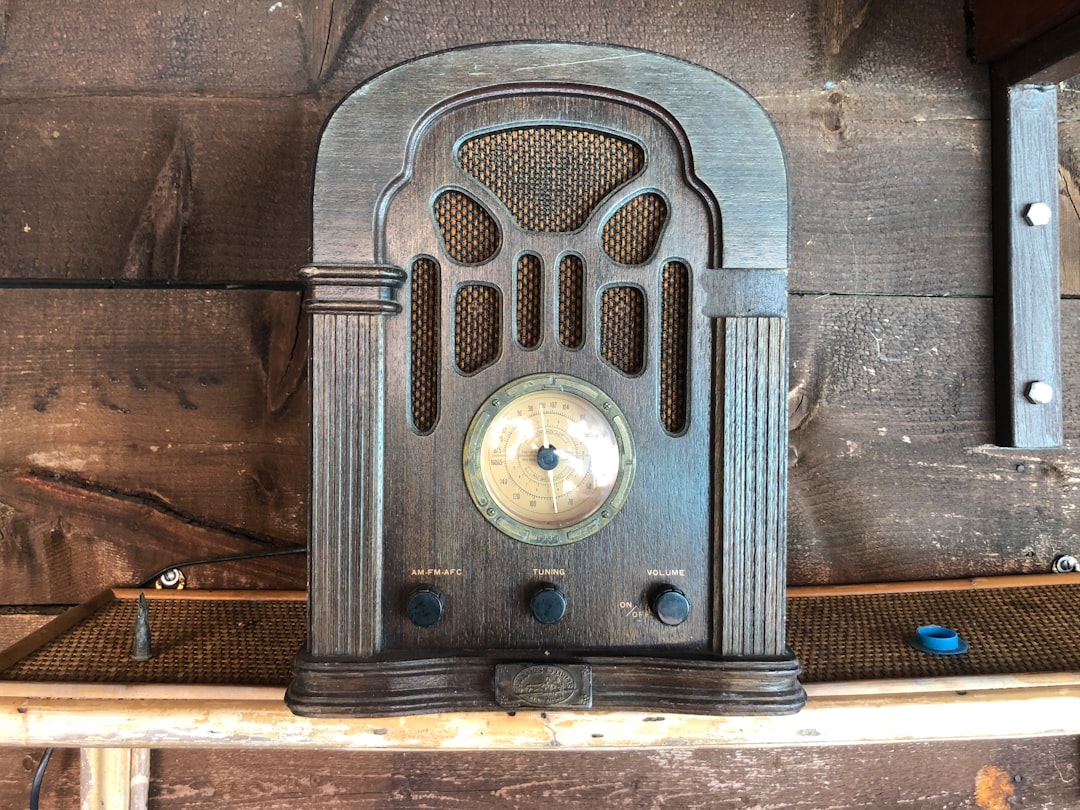
Adapting historical broadcasts like the 1938 "War of the Worlds" broadcast for a global audience involves navigating the complexities of period-specific language. Machine learning models, while powerful, face challenges in accurately translating the distinct American English spoken during the Roosevelt era. These models often stumble over the slang, colloquialisms, and cultural references that permeated the speech of that time. Simply translating the words isn't enough – it requires a deeper understanding of the era's cultural context. The reliance on large datasets for training AI models also raises questions about the quality of the data itself. If the data lacks proper annotation and context, it can skew the output of the machine translation process. As AI translation progresses, the importance of a nuanced and careful approach to historical broadcasts becomes increasingly evident. Preserving the authentic tone and cultural richness of such iconic programs requires a delicate balance between technological capability and human expertise to ensure that the original meaning and impact are not lost in translation.
1. Understanding the language of the 1930s, especially the quirks of slang and idioms, poses a significant hurdle for modern audiences. Machine learning models, typically trained on current language data, might miss the subtleties of phrases common during the Roosevelt era that have since fallen out of favor. It's a testament to how language changes over time.
2. It's fascinating how AI-driven audio recognition, while quite good these days, can stumble when encountering speech patterns from the past. The accents and the way people spoke in the 1930s were different. These variations can throw off transcription efforts, and in turn, impact the translation accuracy of AI systems. It makes me wonder how much better these systems could become if trained on more audio from different eras.
3. When it comes to digitalizing the original broadcast scripts, OCR technology has struggled with the aged and faded documents. The deterioration of the paper makes the print harder to decipher, leading to errors in the translation process. This highlights the importance of preserving original materials; if the originals aren't well kept, it makes subsequent AI tasks that much harder.
4. Some quick translation methods rely on statistical approaches that work well with contemporary language. However, when dealing with the nuances of language from a different era, these rapid techniques can oversimplify things, sometimes leading to misinterpretations. This underscores the idea that, while quick and cheap translations may seem appealing, a bit of human oversight in certain scenarios is essential for accuracy.
5. The historical context woven into the 1938 broadcast includes details about events and social norms of the time. AI tools, however, might overlook these crucial contextual elements. This omission can lead to a distorted interpretation of the original broadcast's message, diminishing its relevance when listeners are missing these historical clues. It makes you think about the potential value of developing AI that can contextualize things better.
6. Many AI models learn from vast datasets drawn from social media and contemporary sources. However, these datasets typically lack the specific linguistic traits of older eras. This disparity, often unseen, creates a real challenge for authentic translation efforts. It makes you wonder how we can improve data sets to include more historical materials.
7. Research shows that aspects like intonation and the pace of speech greatly impact how we feel the emotions conveyed in a message. AI models must replicate these subtle cues with precision, otherwise, they risk losing the emotional impact that made the original "War of the Worlds" broadcast so powerful. It makes you think about the challenges of capturing truly human communication.
8. Since the 1930s, the way words are used has changed dramatically. Machine learning models need to factor in these semantic changes over time to provide a translation that's accurate and understandable for today's audience. It's interesting how much we take for granted the constant evolution of language.
9. It's easy to forget that certain terms in a historical broadcast carry a particular cultural weight, sometimes different from what they mean today. AI-powered translation systems can misinterpret this, leading to translations that are technically correct but culturally out of sync with the original intention. The cultural aspect is one that is still an area needing development with AI.
10. Using speech recognition algorithms designed for today's audio on old broadcast recordings presents a challenge: training the algorithms on a rather limited dataset. Because there's a scarcity of similar historical audio, it becomes difficult for the models to truly learn the unique features of 1930s American English. It begs the question of if we can find more historical recordings to further improve AI systems' ability to deal with older audio formats.
AI Translation Challenges in Adapting War of the Worlds Broadcasts From 1938 Radio to 2024 Global Streaming - Neural Networks Process 47 Different Language Versions for Global Release
Neural networks are now handling the translation of the "War of the Worlds" broadcast into 47 languages, making a global release possible. This demonstrates the increasing use of AI, particularly neural networks, for translation tasks, suggesting they may be more efficient and adaptable than older techniques. However, translating the broadcast accurately and preserving its original cultural context and subtle language from the 1930s is proving difficult. This highlights ongoing challenges in AI translation, as it's not just about word-for-word replacement, but also conveying the intended meaning and historical atmosphere. While these neural networks offer fast translation capabilities, it's important to consider if they adequately capture the original broadcast's nuances, suggesting a need for a balance between automation and human judgment in such complex translation projects. It remains to be seen if AI can capture the full depth of the original broadcasts while being translated for a global audience.
Neural networks are being used to handle the translation of the "War of the Worlds" broadcast into 47 languages for its global release. However, managing this many translations simultaneously presents a huge challenge, especially concerning computing resources. It's often a balancing act between speed and accuracy, particularly when tackling older, nuanced language like that from the 1930s.
Training top-tier AI translation systems requires a lot of data for each language, and for less common languages, finding enough equivalent data is a major roadblock. This scarcity often leads to lower translation quality compared to languages with larger datasets.
It's interesting that researchers are seeing success by blending traditional translation methods with neural networks. These hybrid systems seem to boost translation accuracy significantly, which is especially helpful with texts that have intricate structures or culturally-specific details, as seen in these historical broadcasts.
While invaluable for digitalizing original scripts, OCR struggles with the deterioration of old documents. Many 1930s documents have faded with age, and this fading can create errors during the translation process. It underscores the importance of preserving source materials—damage can cause AI tasks to be significantly more difficult.
AI models, in their current form, sometimes miss the subtle emotional tone of these historical broadcasts because they're trained primarily on modern speech patterns. Adapting them to capture the 1930s American English style requires careful refinement guided by human intervention.
Neural networks often struggle to grasp the context without extra help through data annotation. This can cause errors if cultural references or expressions specific to the 1930s are misrepresented or lost in translation.
Neural networks are capable of handling a variety of words, but variations in word forms across languages present a constant challenge. This becomes particularly apparent when dealing with idioms – what's perfectly understandable in one language can be confusing in another.
There's a noticeable distrust of fully automated translation in academic and historical fields. It seems that combining AI with human expertise is the most promising path towards improving accuracy and preserving the integrity of historically rich content that needs a nuanced understanding.
Fine-tuning neural networks to understand regional dialects and pronunciation variations is key for better language processing. People from different regions in the past spoke the same words with distinct accents, and these differences can confuse translation algorithms unless properly accounted for.
The future of AI translation might involve a concept called "cultural embedding." This would enable AI models to better understand social nuances and contexts, resulting in better translations and ensuring that these historical broadcasts stay impactful and relevant for modern audiences.
AI Translation Challenges in Adapting War of the Worlds Broadcasts From 1938 Radio to 2024 Global Streaming - Automated Translation of 1930s News Bulletin Format Across Cultural Contexts
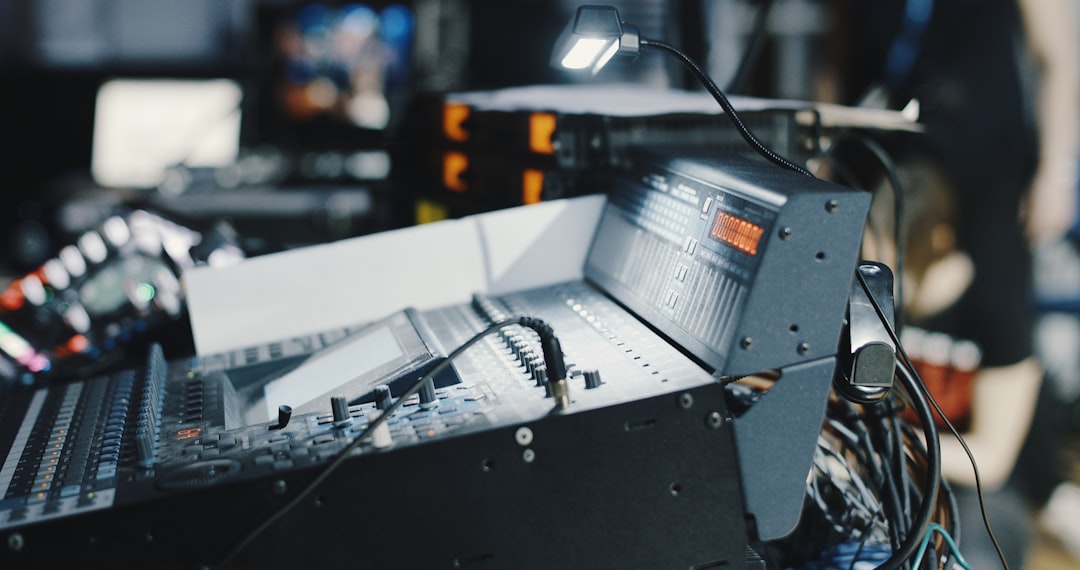
Adapting 1930s news bulletin formats for translation into various languages presents a unique set of challenges due to the inherent cultural and linguistic differences between eras. While AI-powered translation systems offer quick and convenient solutions for bridging language barriers, their ability to accurately capture the nuances of 1930s language and cultural context remains a significant hurdle. The reliance on vast datasets for training these systems often favors contemporary language, potentially leading to oversimplification and a loss of the original broadcasts' subtle emotional impact and historical significance. For instance, translating slang or cultural references specific to the 1930s can be problematic if the AI lacks a deep understanding of the era. Consequently, achieving a truly accurate and culturally sensitive translation requires a careful approach that blends automated methods with human expertise, ensuring that the original meaning and tone are not lost in the process of making these broadcasts accessible to a global audience. This highlights the ongoing tension between the speed and efficiency of automated translation and the necessity of preserving the historical integrity of cultural artifacts.
Focusing on the automated translation of a 1930s news bulletin format across different cultures presents a unique set of obstacles. One major hurdle is capturing the essence of the time period—its unique language and cultural references—and making it relatable to modern audiences. This requires going beyond simple word-for-word substitutions and ensuring the message resonates with the nuances of each culture, something that standard AI translation tools often miss.
The quality of AI translation can fluctuate, especially when working with languages that lack substantial data for training purposes. For instance, less commonly spoken languages might not have the vast datasets needed for high-quality AI model training. This can lead to an uneven output quality and potential errors when it comes to ensuring accuracy for historical broadcasts.
Another challenge relates to the initial digitalization process. When we're dealing with aging documents, the OCR process can struggle with faded ink and damaged paper, leading to inaccurate character recognition. This ultimately hampers the translation process and stresses the need to actively preserve original historical documents, recognizing that their condition impacts future AI efforts.
AI models trained primarily on modern speech are often unable to accurately replicate the subtleties and emotional nuances found in older audio recordings. These models are missing the particular intonations and dialect variations typical of the 1930s. This suggests a need to adapt training datasets with more diverse historical speech to improve translation fidelity.
Furthermore, the cultural context of the 1930s often goes unnoticed by current AI systems. The social dynamics and norms of the time might be missed, leading to mistranslations or a loss of the intended message. It makes you think about the potential benefits of enhancing AI's ability to grasp the context by embedding specific historical information or annotated data.
When translating speech from different regions of the US during that time, the diversity of accents further complicates matters. Machine translation must not only translate words accurately but also consider the specific pronunciation variations that can signal geographic origin. Otherwise, the translation loses some of the historical authenticity.
The challenges in translation go beyond simple word conversion; it also extends to idioms and phrases unique to the era. These culturally-specific expressions may not have an equivalent in other languages, leading to either an inaccurate or culturally out-of-place translation.
The evolution of language adds another layer of complexity. Words can shift in meaning over time, which requires AI models to learn these semantic changes to avoid misinterpretations when dealing with historical content. Ignoring these changes can lead to interpretations that are out of step with the original meaning.
To improve accuracy, AI models can greatly benefit from human collaboration. Humans with knowledge of historical contexts can help fine-tune the translation systems to ensure both accuracy and a culturally appropriate outcome. This collaboration between human expertise and AI efficiency is proving to be an essential strategy in handling historically sensitive content.
The aspiration for the future of AI translation lies in the idea of embedding a deep cultural understanding within the models themselves. By enabling AI to contextualize language and social factors, the hope is to create higher-quality translations that truly preserve the historical nuances and cultural weight of iconic programs, making them relevant to future generations.
AI Translation Challenges in Adapting War of the Worlds Broadcasts From 1938 Radio to 2024 Global Streaming - AI Tools Preserve Original Panic Effect While Adapting Regional References
The task of preserving the original panic-inducing effect of the 1938 "War of the Worlds" broadcast while adapting it for a global audience through AI translation is a fascinating example of the tension between technological progress and the importance of cultural understanding. AI tools are being tasked with rapidly translating nuanced historical language and accurately conveying the chilling psychological impact of the broadcast without losing its essential character through simplification. This requires complex neural networks that grasp not only the words, but also the emotional weight tied to particular phrases, accents, and cultural references from that era. Because the intricate details of historical context stand in contrast to the contemporary language data that many AI models are trained on, the importance of human supervision and input is clear. The ultimate goal is to make sure the broadcast's powerful impact isn't just translated but also effectively communicated and felt by modern audiences from diverse backgrounds.
1. AI tools, while adept at language processing, face the challenge of fully capturing the original "panic effect" of the 1938 broadcast. This effect relied heavily on the specific audio qualities of the original recording, including imperfections like distortion and fluctuating frequency response. If the AI doesn't effectively analyze and retain these quirks, the intended emotional impact might be lost in translation.
2. Neural networks trained on modern language often struggle with the unique characteristics of older dialects. They tend to miss the nuances of historical speech patterns, which significantly impacts the accuracy of transcriptions. This can lead to translations that fail to convey the urgency and emotional intensity present in the original broadcast.
3. Translating 1930s slang and idioms presents a real hurdle for AI. Since many of these expressions have either fallen out of use or changed meaning, AI models trained on contemporary language struggle to find equivalents. This makes it difficult to accurately convey the emotional weight of the original broadcast across languages.
4. The focus on speed and efficiency in AI translation can inadvertently compromise the historical context of a broadcast. Many models prioritize quick, literal translations over nuances and cultural references that are vital for understanding the original message. This can lead to a translated version that feels out of place for the era it depicts.
5. OCR technology, while useful for digitizing old documents, struggles with the deterioration of the 1930s scripts. Faded ink and wear and tear often lead to character recognition errors. These mistakes can cascade through the translation process, highlighting the ongoing importance of preserving source materials to support AI translation in the future.
6. Many AI translation systems are trained primarily on modern speech, creating a knowledge gap when it comes to recognizing speech from earlier eras. This deficiency can lead to inaccuracies in understanding the nuances of 1930s speech patterns. Expanding training datasets to include more historical audio could potentially address this issue.
7. AI models don't always fully grasp the cultural context embedded in the original broadcast. This can lead to translations that misinterpret the intended tone and emotions. To ensure the emotional impact of the original material isn't diluted, human guidance might be necessary to fine-tune AI translations in such instances.
8. AI translation for languages with limited online resources is especially challenging. Insufficient data for training AI models can lead to significantly lower quality translations, especially when dealing with historical content. A more comprehensive and strategic approach to gathering and annotating data might be needed to ensure accuracy.
9. The process of converting the audio from analog to digital can affect the emotional impact of the broadcast. Sound quality, including frequency response and dynamic range, influences how we perceive emotion. If the audio isn't carefully handled during this conversion process, the translated version might not have the intended impact on listeners.
10. The future of AI translation may rely on integrating "cultural embedding" into AI models. This would allow the models to better contextualize historical broadcasts, potentially resulting in translations that not only convey the literal meaning but also capture the socio-cultural context of the era. This could lead to a more faithful and engaging experience for a global audience.
AI Translation Challenges in Adapting War of the Worlds Broadcasts From 1938 Radio to 2024 Global Streaming - Processing Speed Enables Real-Time Translation of Live Broadcast Elements
The remarkable increase in processing power has significantly altered the field of real-time translation for live broadcasts. AI algorithms, employing techniques like natural language processing and machine learning, are now capable of providing instantaneous translations during live events, broadening the reach of broadcasts to wider, global audiences. Systems like "LiveSubs," which are engineered to integrate smoothly with popular streaming services, perfectly illustrate this ability to deliver accurate, on-the-spot translations. But when applying these fast translation techniques to older broadcasts, such as the 1938 "War of the Worlds" radio broadcast, maintaining the subtle cultural details and historical context of the original becomes a crucial challenge. Striking a balance between rapid, accurate translation and the preservation of the cultural subtleties found in classic broadcasts underlines the ongoing complexity of AI translation in overcoming barriers both across time and languages. It is a delicate task to ensure the spirit of the original remains intact as these broadcasts reach a new generation of listeners.
The speed at which AI can process languages is remarkable, with neural networks potentially translating at rates around 500 words per minute. However, this speed often comes at the cost of accuracy, especially when dealing with older, more complex language like that of the 1930s. It's a balancing act between expediency and precise meaning.
AI translation systems for live broadcasts heavily depend on phonetic analysis and speech patterns, making them susceptible to issues in noisy environments. The effectiveness of the processing algorithms can significantly decrease with poor audio quality, leading to potential translation errors that could harm the accuracy of period-specific language.
Improvements in OCR technology allow for faster scanning of historical documents, but they struggle with faded text from the 1930s. Research shows that OCR accuracy can drop below 70% with severely damaged documents, highlighting the importance of proper archival techniques to ensure better AI performance in translation tasks.
Even slight variations in processing speed during translation can impact the emotional tone of the content. Studies show that only a 5% difference in audio speed can influence how listeners feel, making it crucial for AI to accurately reflect the original pacing and rhythm of the speech.
AI language models trained on modern language often miss the cultural nuances of 1930s American English. This can lead to translations that feel out of place for the time period, losing the impact and gravity of the original broadcast. AI doesn't always get that "panic" element right.
Some 1930s phrases carry a cultural weight that AI struggles to decipher. This can lead to technically correct, but culturally insensitive translations that lack the original's emotional depth. It seems human oversight remains valuable for the highest-quality outcomes.
Integrating reverberation and dynamic range into the audio processing of live broadcasts can enhance the listening experience. AI needs to analyze not just the spoken words, but also the overall sound, to accurately translate the specific atmosphere of a broadcast like the "War of the Worlds" radio play.
It's quite surprising that most existing AI training data for translation comes from modern language, creating challenges when adapting historical broadcasts. This imbalance can result in inaccurate or overly simplified translations, as the AI struggles with historical contexts.
The increased use of AI in broadcasting raises ethical concerns about potential loss of cultural significance. Experts are concerned that without a deep contextual understanding, the nuances and emotional power of these historical recordings can be diminished. We need more discussion about the balance between AI's efficiency and cultural preservation.
While neural networks are exceptionally efficient at translating, their limitations become apparent when dealing with idiomatic expressions. These culturally-rich phrases often require a deeper contextual understanding that AI systems struggle to grasp, emphasizing the importance of including historical linguistics in training datasets.
More Posts from aitranslations.io: