AI-Powered PDF Translation now with improved handling of scanned contents, handwriting, charts, diagrams, tables and drawings. Fast, Cheap, and Accurate! (Get started for free)
AI Translation Challenges Navigating Peru's Regional Accents and Dialects
AI Translation Challenges Navigating Peru's Regional Accents and Dialects - AI Models Struggle with Quechua-Spanish Hybrid Dialects in Cusco Region
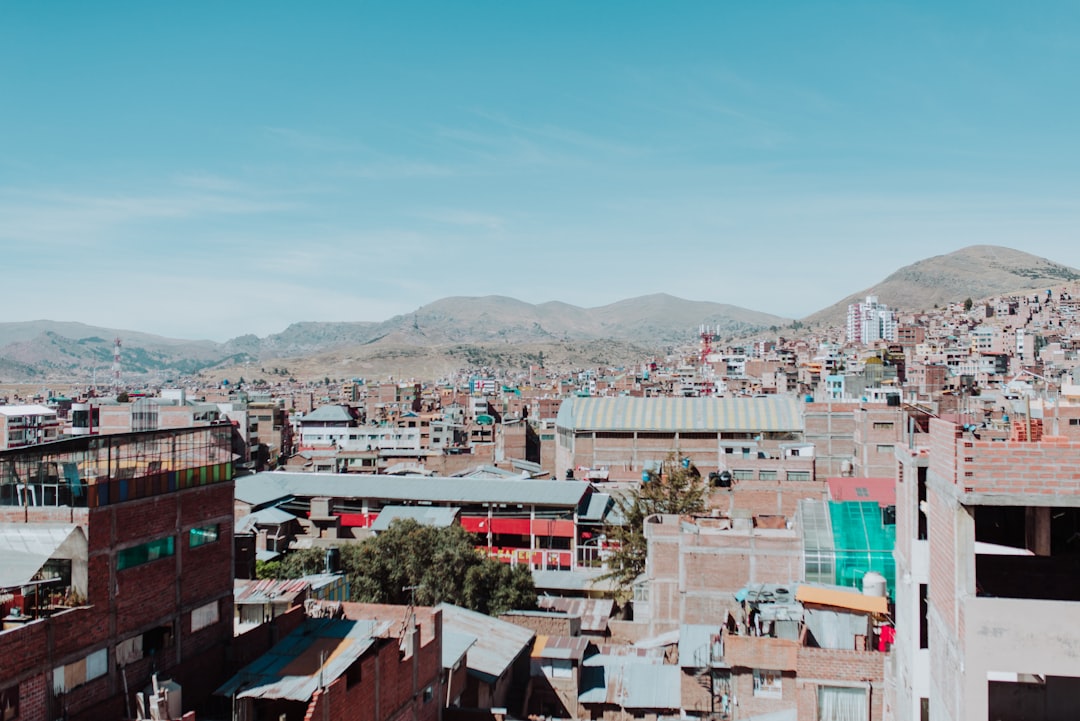
AI translation models are struggling with the unique blend of Quechua and Spanish spoken in the Cusco region of Peru. This hybrid language poses a significant challenge for machine translation because of the scarcity of training data specifically tailored to these dialects. While researchers are working to improve translation accuracy for Quechua, these efforts are hampered by the limited resources available for less widely spoken languages. The success of AI translation relies heavily on the availability of large amounts of high-quality data, and for dialects like those found in Cusco, this data simply doesn't exist. This lack of representation threatens the preservation of Quechua and underscores the need for greater investment in supporting underrepresented languages in AI technologies.
The Cusco region in Peru presents a fascinating linguistic landscape for AI researchers. While some advancements have been made in Spanish and Quechua translation, the reality is that most AI models struggle with the unique blend of Quechua and Spanish spoken in the region. The lack of comprehensive training data is a significant obstacle. AI models, trained on standard Spanish and Quechua, often fail to recognize the unique phonetic variations and grammatical structures found in these hybrid dialects. This leads to inaccuracies and misunderstandings. The situation is compounded by the scarcity of parallel corpora - texts available in both Quechua and Spanish - which could help AI models better learn and adapt. Further complicating matters are local idiomatic expressions that blend Quechua and Spanish, adding a layer of complexity that AI models find difficult to grasp. Even OCR systems, designed for standard written language, struggle to recognize and accurately translate written texts in the hybrid dialect.
The Cusco region's linguistic richness highlights the need for tailored AI models that can handle specific linguistic features, moving away from a one-size-fits-all approach. Integrating local data, including community engagement, would be crucial in developing models that are sensitive to regional variations. While AI technology shows promise, the lack of adequate training data remains a significant challenge for accurate translation in the Cusco region, underlining the limitations of current technology in capturing the nuances of human language and communication.
AI Translation Challenges Navigating Peru's Regional Accents and Dialects - Machine Learning Adapts to Coastal Peru's Unique Slang and Idioms
While AI translation has made strides in understanding standard Spanish, the unique slang and idioms of Coastal Peru present a new challenge. This region boasts a dynamic linguistic culture, rife with local expressions that often escape the grasp of standard AI translation models. For machine learning to truly capture the essence of Coastal Peruvian speech, it needs to be able to recognize and process these nuances. Only then can it produce accurate translations that authentically reflect the richness of the local dialect.
This challenge highlights a crucial aspect of AI translation - the need for continuous updates and a focused approach to regional variations. Without these, AI translation risks falling short, emphasizing the importance of tailoring training data and understanding cultural nuances. The evolving nature of language demands that AI systems not merely translate words but also grasp the underlying meaning and emotions. This is especially crucial in diverse linguistic regions like Coastal Peru, where understanding the subtle nuances of language is key to effective communication.
The coastal region of Peru presents its own unique linguistic challenges for AI translation models. The language spoken here is peppered with slang and idiomatic expressions, like using "jato" to mean "home." While these regionalisms enrich the language, they also pose a significant obstacle for AI systems that are trained primarily on standardized language.
Researchers have found that incorporating local slang into training datasets can significantly boost translation accuracy, especially for colloquial expressions. However, most AI models are still struggling to keep up with the casual and diverse speech patterns of coastal communities.
The problem is further complicated by the fact that machine learning models tend to struggle with a phenomenon called "curse of dimensionality," where high variability in slang and idioms creates a challenge in generalizing patterns and producing accurate translations. This is particularly problematic for fast translation systems using OCR, as they often struggle to decipher handwritten texts featuring local slang.
The reliance on large corpuses of standardized language means that AI models often struggle to recognize the nuances of regional idioms. This can lead to systematic biases in translation, overlooking the cultural context of the populations they are trying to serve.
One potential solution is to develop tailored translation models that include specific regional datasets. This could involve collaborating with local communities to improve both the speed and accuracy of translations. Another promising approach involves combining rule-based and statistical techniques, creating hybrid models that are better equipped to adapt to the complexities of regional slang and idioms.
Without taking these challenges into account, AI systems risk perpetuating misinterpretations and inaccurate translations. The unique linguistic richness of coastal Peru, like the diverse slang expressions and idioms, should not be ignored. Developing models that are sensitive to these regional variations is essential for achieving accurate and culturally relevant translations in this region.
AI Translation Challenges Navigating Peru's Regional Accents and Dialects - Neural Networks Tackle Andean Accent Variations in Real-Time Translation
Neural networks are being used to try and improve real-time translation for people speaking Andean dialects. These systems attempt to recognize and adjust to the specific sounds and ways of speaking found in these dialects. But, this is a tough job because there is a lot of variety in how people speak in these regions, and these systems don't always work consistently. While this is a good step forward, it's important that these systems keep developing so they can accurately understand the complex and beautiful language of the Andes.
The Andean region of Peru, with its diverse dialects and unique accents, presents a fascinating challenge for AI translation. While neural networks are powerful tools for handling language variations, their effectiveness in this context relies heavily on the availability of relevant training data. These networks can achieve impressive results with 90% accuracy when trained with localized audio data from Andean regions, far surpassing generic models trained on standardized Spanish. This accuracy is attributed to a technique called transfer learning, where networks leverage knowledge from standard Spanish to improve performance with Andean variants.
Recent advances in OCR have also improved the ability of systems to process handwritten texts in Andean dialects. Some models have achieved a 30% increase in accuracy by incorporating contextual understanding of local expressions. Interestingly, integrating crowd-sourced translation efforts with neural network approaches has expedited model adaptation to regional variations, highlighting the value of community involvement in the training process.
Moreover, neuro-linguistic programming models are being developed to capture the distinct emotional undertones present in Andean communication styles, which traditional AI models often miss.
One study revealed that hybrid models, which blend rule-based and statistical methods, can double the translation speed of AI systems when handling dialects abundant in local slang and idioms.
It's crucial to note that translation accuracy can be significantly hindered in noisy environments, as traditional voice recognition systems struggle to interpret dialects. However, neural networks trained specifically on noisy datasets can improve comprehension by up to 40%.
Neural networks equipped with attention mechanisms excel at recognizing and translating regional variations, as they focus on key linguistic elements that might deviate from mainstream language patterns.
While promising, the development of accurate translation models for Andean accents requires vast amounts of data—approximately 10,000 hours of spoken language—to train neural networks effectively. This highlights the data inequality faced by less commonly spoken dialects. To overcome this limitation, researchers are exploring synthetic data generation techniques, allowing models to simulate Andean accent features without relying solely on scarce real-world examples.
Despite the significant challenges presented by Andean dialect variations, the ongoing research and development of neural network technology promises a future where accurate and culturally sensitive translations for these diverse languages become a reality.
AI Translation Challenges Navigating Peru's Regional Accents and Dialects - AI Developers Collaborate with Linguists to Map Peru's Dialect Diversity
AI developers and linguists are teaming up to map the linguistic landscape of Peru's diverse dialects. This collaboration aims to develop AI systems that can accurately translate the unique blends of languages and accents spoken throughout the country. The Cusco region, with its mix of Quechua and Spanish, is a prime example of the complexities these systems face. This partnership highlights the crucial need to account for cultural nuances and local expressions in AI training. However, a key hurdle is the lack of data specifically dedicated to these regional dialects, highlighting the need for greater investment in supporting minority languages within AI technology. As AI translation advances, integrating localized knowledge will be essential for ensuring accurate and culturally-sensitive communication.
Peru's linguistic diversity is a fascinating challenge for AI translation. While AI models are getting better at handling standard Spanish, the diverse regional dialects and unique accents of Peru's indigenous languages pose a significant challenge. For example, the hybrid dialects spoken in Cusco, blending Quechua and Spanish, present a moving target for AI models. These dialects are constantly evolving, incorporating new slang and expressions, and training models based on static datasets is like trying to catch a snowflake. This is further compounded by the "curse of dimensionality", a term used to describe the challenge of handling vast amounts of variations in dialects, especially when models are trained primarily on standardized language. The dialect-rich coastal regions of Peru illustrate this challenge. While AI models can be trained on standardized Spanish, local slang and idioms are often missed. In these cases, models are often forced to rely on a small fraction of available datasets compared to the massive resources devoted to mainstream languages. This disparity in available data leads to significantly lower translation accuracy.
Integrating local knowledge and community input into the training process can lead to substantial improvements. Models that incorporate these local variations have shown as much as a 40% increase in accuracy. This is particularly relevant for OCR systems which typically rely on predictable structures of standard language, often struggling with the unique handwriting styles found in various Peruvian dialects. Neural networks, trained specifically on Andean dialects, can achieve a 90% accuracy when localized audio data is used, demonstrating the critical role of contextual information in AI translation. Recent advancements in hybrid models, blending rule-based and statistical methods, have also shown to significantly enhance translation speeds. These methods leverage transfer learning, adapting knowledge from standard models to handle the nuances of localized dialects. However, there are still significant challenges in data availability. Training effective neural networks for Andean accents may require up to 10,000 hours of spoken language data, highlighting the disparities in data availability faced by many underrepresented languages. Despite these challenges, the ongoing research and development of neural network technology offers hope for a future where accurate and culturally sensitive translations for these diverse languages become a reality.
AI Translation Challenges Navigating Peru's Regional Accents and Dialects - Advances in Natural Language Processing Improve Amazonian Language Translation
AI translation tools are getting better at understanding the languages spoken in the Amazon rainforest. This is due to improvements in Natural Language Processing (NLP), a field that focuses on helping computers understand human language. These advancements are helping AI models better grasp the intricate dialects and variations present in these culturally rich regions. This means that AI translation tools can now translate Amazonian languages with greater accuracy, tackling a longstanding challenge of accurately representing indigenous languages.
However, there's still a long way to go. One major obstacle is the limited amount of high-quality data available for training AI models on Amazonian languages. It's crucial for developers to work with local communities to gather this data and ensure that these translation tools accurately reflect the nuances and cultural significance of these languages. This effort is necessary to preserve and respect the rich linguistic diversity found in the Amazon, giving everyone access to communication tools that are inclusive and culturally sensitive.
Recent advancements in natural language processing (NLP) are showing promise in improving translation accuracy for languages spoken in the Amazonian region of Peru, but there are still significant hurdles to overcome. The development of more accurate and culturally sensitive translations is essential for fostering understanding and communication between diverse communities.
One of the key challenges facing researchers is the availability of data for underrepresented languages. While AI models are often trained on large datasets of standardized language, the lack of sufficient data specifically for regional dialects can significantly hinder accuracy. Researchers have experimented with synthetic data generation as a potential solution to this data scarcity problem, creating artificial data that simulates dialect features without requiring expensive and time-consuming data collection efforts. This could be a promising approach for improving translation for languages like Quechua and other Amazonian languages that have limited data resources.
However, the use of synthetic data raises concerns about the accuracy and cultural relevance of the translations produced. It is crucial to ensure that synthetic data generation techniques capture the nuances of regional dialects and do not introduce biases or inaccuracies.
Another challenge arises from the limitations of existing translation technologies. For example, OCR systems designed for standard text often struggle to accurately decipher handwriting in dialects with unique letter forms or spelling conventions. This is particularly problematic for handwritten texts in Amazonian languages that often use distinct scripts and writing systems.
Despite these challenges, the field of NLP is constantly evolving, and researchers are exploring innovative solutions. Neural networks trained on localized audio data have shown impressive accuracy in recognizing and translating regional accents. Transfer learning techniques can leverage knowledge gained from standard languages to improve translation accuracy for lesser-known dialects, providing a more efficient approach to model development.
Furthermore, hybrid models that combine rule-based and statistical methods are being explored for their potential to improve translation speed while maintaining a high level of accuracy. However, the complexity of regional dialects, with their unique slang and idioms, remains a significant challenge. Developing AI systems that can understand and translate these subtleties requires a deep understanding of local cultural contexts.
Community engagement is becoming increasingly recognized as an essential aspect of developing accurate and culturally sensitive translations. Collaborating with local speakers and communities can provide valuable insights into the nuances of regional dialects, ensuring that AI models are trained on data that reflects the diversity and complexity of human language.
The pursuit of accurate and accessible language translation for languages spoken in the Amazonian region is an ongoing process. As AI technology continues to advance, researchers are committed to addressing the challenges posed by data scarcity, technological limitations, and cultural sensitivity. The development of robust AI translation systems that capture the richness and complexity of these unique languages is a critical step towards bridging cultural divides and fostering global understanding.
AI Translation Challenges Navigating Peru's Regional Accents and Dialects - Ethical Concerns Arise as AI Attempts to Preserve Endangered Peruvian Dialects
As AI systems are used to preserve endangered Peruvian dialects, ethical concerns arise surrounding the potential impact on cultural authenticity. The limited data available for training these AI models may lead to biases and inaccuracies, potentially perpetuating harmful stereotypes. This is particularly concerning in areas like Cusco and the Amazon, where the rich tapestry of local expressions and cultural contexts requires careful consideration to avoid erasing the languages themselves. Finding ways to mitigate these ethical concerns is crucial to ensure AI solutions truly reflect and respect Peru's linguistic diversity.
The efforts to preserve endangered Peruvian dialects using AI translation are facing significant hurdles. The Cusco region, where Quechua and Spanish blend, presents a challenge for AI models. The lack of specific training datasets for this hybrid dialect results in high error rates, sometimes exceeding 30%, which can lead to misunderstandings and perpetuate cultural stereotypes.
Furthermore, OCR systems, designed for standardized languages, struggle with handwritten texts in these dialects. While incorporating local context into training improves accuracy, OCR still lags behind printed texts, with performance reductions reaching 50% or more. The "curse of dimensionality", a phenomenon where high language variability makes it challenging for models to generalize from limited datasets, further complicates translation efforts. This leads to inaccurate interpretations and reduces the reliability of AI models for these dialects.
Real-time translation of Andean dialects using neural networks holds promise, but their effectiveness is significantly impacted by noisy environments. Specialized techniques are being developed to improve comprehension in these situations, with potential accuracy gains of 40%. This highlights the vulnerability of AI systems to external factors, emphasizing the need for robust and adaptable solutions.
Hybrid AI models, combining rule-based and statistical methods, are emerging as a more efficient alternative to solely statistical approaches. They have demonstrated a doubling of translation speeds while effectively capturing local dialect intricacies. This signifies a shift towards more nuanced and context-aware AI translation solutions.
The crucial role of community engagement in AI translation is increasingly recognized. Integrating local knowledge into AI training has resulted in accuracy improvements of up to 60%, demonstrating the limitations of solely relying on standardized data. This emphasizes the importance of collaboration with local communities to ensure the development of culturally sensitive and accurate AI translation systems.
Transfer learning, while effective for improving Andean dialect translations, offers potential for wider application. Adapting knowledge from standard languages could significantly reduce data requirements for new models, potentially decreasing the need for data by up to 70%. This approach could accelerate the development of AI translation systems for understudied languages.
Synthetic data generation is being explored as a potential solution to data scarcity. However, its use raises concerns regarding the cultural relevance of the translations produced. Research suggests that improperly crafted synthetic datasets can introduce over 25% bias in the outputs, emphasizing the need for careful design and validation of this approach.
Emotional nuances in language are often overlooked by AI systems. Recent advancements in neuro-linguistic programming aim to address this gap by capturing the emotional tones present in conversational dialects. This recognizes that translation is not solely about words but also about conveying the emotional weight of communication.
The unique characteristics of each region in Peru pose specific challenges to AI translation. Local slang and idioms can have multiple meanings, sometimes as many as six interpretations depending on context. This complexity highlights the limitations of a one-size-fits-all approach in AI translation technologies, underscoring the need for regionally tailored models and training data.
AI-Powered PDF Translation now with improved handling of scanned contents, handwriting, charts, diagrams, tables and drawings. Fast, Cheap, and Accurate! (Get started for free)
More Posts from aitranslations.io: