AI-Powered PDF Translation now with improved handling of scanned contents, handwriting, charts, diagrams, tables and drawings. Fast, Cheap, and Accurate! (Get started for free)
AI Translation Embracing Uncertainty in Language Processing
AI Translation Embracing Uncertainty in Language Processing - Metax's AI Model Tackles Overlooked Languages in Translation
Meta AI's NLLB200 model tackles the challenge of translating languages that are often overlooked by standard translation tools. With thousands of languages spoken globally, most translation systems fail to support the vast majority. NLLB200 distinguishes itself by using a singular system for translation across 200 languages, instead of the typical multi-model chain approach. This single system makes translations faster and potentially more accurate.
To assess the quality of translations, especially for less-common languages, Meta AI developed a new benchmark called Flores101. This benchmark provides a standardized way to evaluate how well NLLB200 handles these languages. Further, the model's ability to translate directly between language pairs without relying on English as a bridge is a noteworthy innovation. It signifies a move towards more inclusive translation technology that can directly benefit individuals and communities speaking underrepresented languages. This is important in a world where internet access and information sharing shouldn't be limited by language barriers. However, it remains to be seen how well NLLB200 can translate nuanced, cultural or contextual language within its 200 supported languages, as it could face hurdles in properly handling diverse and complex linguistic information.
Meta's AI team has made strides in addressing a critical gap in translation technology: the neglect of less-common languages. While thousands of languages are spoken globally, the majority are underserved by existing translation tools. Their model, dubbed NLLB200, tackles this challenge head-on by enabling translation between 200 languages. This is a substantial leap forward compared to prior approaches that typically chained multiple, specialized models together for translation. The innovative aspect of NLLB200 is its single-system design, which simplifies the process.
To gauge the model's proficiency, especially with low-resource languages, they've introduced a new benchmark called Flores101. This benchmark utilizes 3,001 sentences from English Wikipedia, strategically chosen to evaluate translation quality across a diverse range of languages with limited digital presence. Importantly, this also means more robust evaluation of many-to-many translation systems. The developers at Meta have meticulously designed NLLB200 to ensure high accuracy across the board, validating its performance across all the languages it supports. They've introduced a companion dataset, Flores200, to further assess and refine the model's performance.
Furthermore, NLLB200 offers the ability to translate directly between any two of its supported 200 languages, dispensing with the necessity for English as an intermediary. This is a substantial departure from previous models that primarily relied on English data, highlighting Meta's dedication to inclusivity. This represents a significant move toward a more inclusive translation landscape, expanding accessibility to internet resources for individuals who speak languages that are not widely used. The potential impact on language accessibility and online inclusivity is undeniable. It's a key step toward dismantling barriers for a greater diversity of language speakers. However, the challenge remains on how well this scales with continued growth of languages and dialectal variations and how to efficiently integrate user feedback into future iterations.
AI Translation Embracing Uncertainty in Language Processing - Deep Learning Enhances Natural Language Processing Capabilities
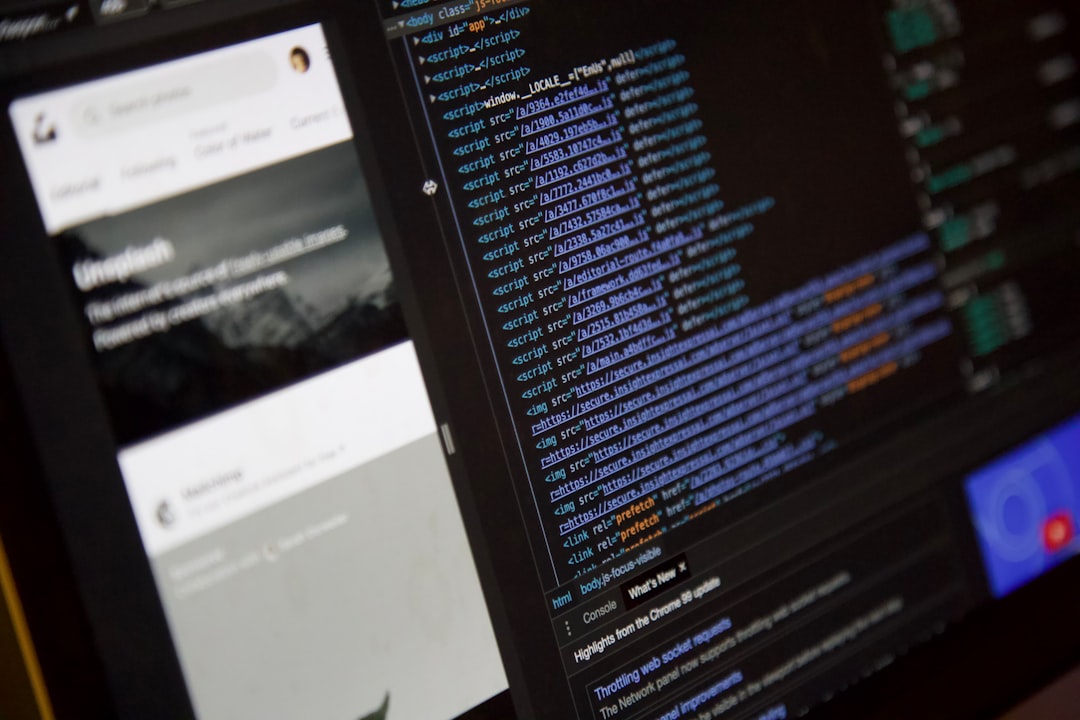
Deep learning has significantly advanced the field of natural language processing (NLP), driving progress in AI translation systems. These advancements stem from the use of complex neural networks that allow language models to perform tasks akin to human comprehension, like summarizing text or translating language with context. This has led to noticeable gains in machine translation, creating more accurate and contextually aware translations. Despite these advances, challenges persist, especially when dealing with languages that have complex cultural or nuanced aspects. As deep learning continues to refine NLP, we can anticipate future innovations that may fundamentally alter AI translation in a world with an increasingly diverse range of languages. It remains to be seen whether this trend will lead to improved accuracy and understanding of a wider variety of languages, or if it will primarily result in faster but less nuanced translations.
Deep learning has significantly boosted the capabilities of Natural Language Processing (NLP) by using neural networks to understand and generate human language in ways we couldn't before. This has led to improvements in the accuracy and fluency of machine translations.
These deep learning models are trained on massive datasets, allowing them to pick up on the subtle nuances present in different languages, such as idioms and cultural references. This is important for making AI translations more contextually relevant and less literal.
However, deep learning models still face challenges when dealing with languages that don't have a lot of data available for training. This can lead to inaccuracies and errors in translations. Striking a balance between the power of deep learning and the availability of training data for all the world's languages remains a key challenge.
OCR has also seen benefits from deep learning through improved accuracy in extracting text from images. This can help integrate written content from various languages into translation models more easily. It's interesting how these two fields (NLP and OCR) influence each other.
The advent of transformer models in deep learning has sped up translation processing considerably. We can now see real-time translations that adapt quickly to changes in context or user input. It’s fascinating how quick these models have become.
Deep learning approaches are still struggling with words that have multiple meanings depending on the context, such as homonyms and polysemous words. This makes it difficult for the AI to pick the correct meaning. Disambiguation is a major area of ongoing research in translation.
Traditional rule-based methods often rely on huge dictionaries and grammar rules. Deep learning models can learn patterns from data, which makes them more flexible and scalable for a wider range of languages. This difference is an interesting point of comparison.
Some deep learning models, like transformers, use attention mechanisms. This lets the system focus on particular parts of the input sentence during translation. This helps make the final output clearer and more coherent. Understanding how these mechanisms function and how they can be optimized is important.
Creating models that can handle multiple languages can not only improve translations between common languages but also make it easier to translate directly between less common languages. This helps promote more linguistic diversity and communication across cultures.
Finally, there's the issue of data bias in training datasets. This can affect the output of the deep learning models, leading to biased translations that perpetuate stereotypes. Carefully curating the training data is essential to ensure fair and equitable language support. The ethical implications of this bias cannot be overlooked.
AI Translation Embracing Uncertainty in Language Processing - Ethical Debates Surround AI-Driven Translation Advancements
The rapid progress of AI in translation, while promising faster and more efficient communication across languages, has sparked important ethical discussions. Concerns about bias within these systems are central, as they can inadvertently perpetuate stereotypes and misrepresent cultures through skewed translations. The potential for these technologies to exacerbate existing inequalities, especially in access to information, is a significant worry. Developing these tools necessitates careful consideration of ethical implications, particularly in areas like data management, preserving cultural nuances, and respecting user privacy. Moving forward, it's crucial to strike a balance between the drive for technological advancement and the need for ethical considerations that prioritize inclusivity and accuracy in translation across all languages. The ultimate goal should be to ensure that these advancements benefit all communities and promote a more equitable global communication landscape.
The rapid increase in the use of AI-powered translation tools has sparked worries among some language experts about the fate of languages facing extinction. Over-reliance on these tools might inadvertently hinder efforts dedicated to preserving linguistic diversity and cultural heritage across the globe.
A curious side effect of AI translation systems is their ability to inadvertently reinforce existing language biases. These biases often stem from the datasets used to train the systems, prompting ethical inquiries about the role of developers in carefully selecting and curating training data.
AI translation technology, when applied incorrectly, can lead to comical and inaccurate results. It occasionally struggles to capture the subtleties of cultural context, leading to possible misunderstandings that can strain relationships between individuals communicating in different languages.
The capacity of Optical Character Recognition (OCR) to extract text from images varies considerably among languages. This variation is particularly pronounced when comparing languages that use complex scripts, such as Chinese or Arabic, with those employing simpler alphabets.
Studies have indicated that AI translation systems can achieve better accuracy when translating specialized or domain-specific language, such as legal or medical texts, in comparison to more casual conversation. This highlights the crucial role context plays in determining the quality of a translation.
Recent breakthroughs have allowed certain translation models to adapt to user feedback in real-time. This suggests that interactive translation tools could enhance their performance over time by learning individual user preferences and specific language quirks.
One major shortcoming of current AI translation technology is its difficulty with complex, nuanced expressions. These systems often produce overly literal translations that can misinterpret subtle communication cues like sarcasm or humor, which are crucial elements of effective communication.
Contrary to popular assumptions, machine translation hasn't entirely replaced human translators. Instead, it frequently acts as a valuable assistant for professionals, providing initial translations that often require further refinement by humans to align with specific contextual demands.
The introduction of deep learning within translation processes can sometimes create inconsistencies in translation speed. While deep learning allows for incredibly fast translation, this speed can occasionally come at the cost of accuracy, especially when dealing with idiomatic or culturally-rich phrases.
Ethical questions regarding user privacy are becoming increasingly relevant. Numerous AI translation tools necessitate users to input potentially sensitive information, but the methods by which these providers manage and utilize this data remain unclear, raising concerns.
AI Translation Embracing Uncertainty in Language Processing - NLP Algorithms Break Down Sentences for Accurate Translations
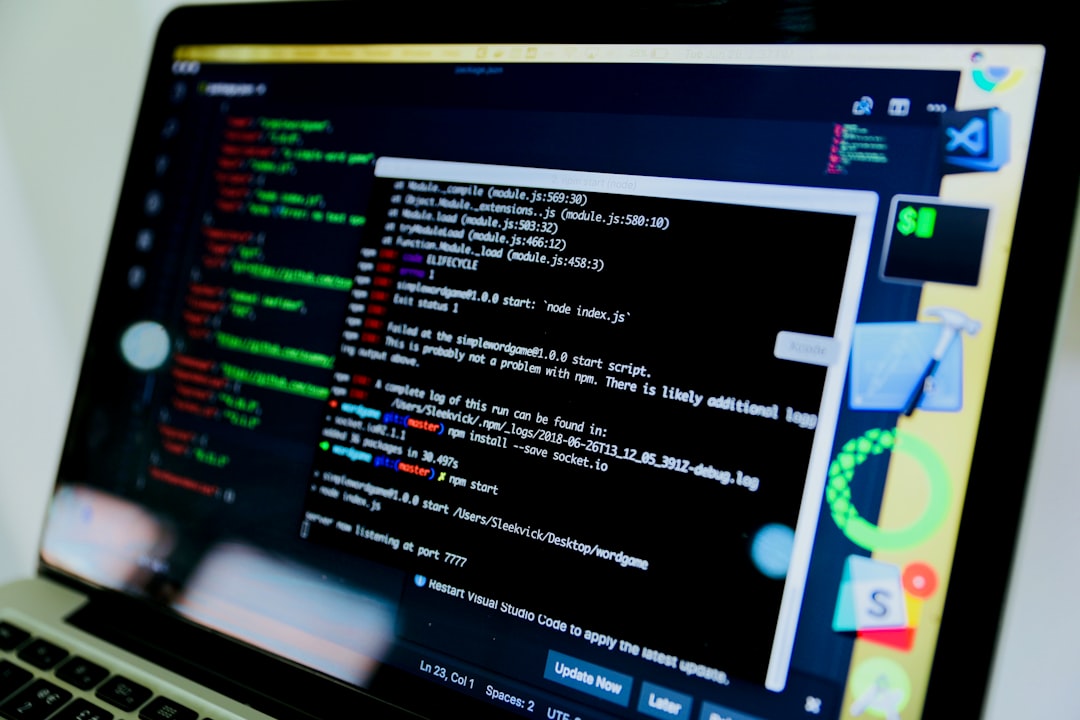
AI translation systems increasingly rely on Natural Language Processing (NLP) algorithms to achieve more accurate translations. These algorithms dissect sentences into their core components: words, phrases, and grammatical structures. This breakdown allows for a more granular analysis, enabling the AI to better comprehend the meaning and context of the text. NLP's effectiveness stems from machine learning models that continuously refine their understanding of diverse languages, leading to faster and more sophisticated translations. However, the complexity of human language, with its inherent ambiguity and cultural nuances, presents a continuing challenge. Simply breaking down a sentence is not always enough, as nuanced meanings and subtle expressions can easily be misconstrued. Therefore, ongoing development in NLP must strive to balance the speed of translation with a deeper, more context-aware understanding of language if AI translation tools are to become truly effective in a world of diverse languages.
AI translation systems, powered by Natural Language Processing (NLP), are steadily improving their ability to accurately translate languages. NLP algorithms break down sentences into smaller parts, like words and phrases, which helps them understand the grammatical structure and context. However, this process isn't without challenges. For instance, languages that lack clear punctuation or have complex sentence structures can be difficult for algorithms to dissect properly. This can lead to mistakes in understanding the overall meaning of the text, making accurate translation more difficult.
Another tricky aspect is understanding words with multiple meanings. NLP algorithms need to figure out the right meaning of a word based on its context, which can be challenging, especially when dealing with languages with many homonyms. This is a key area where research is ongoing.
Additionally, idiomatic expressions are often stumbling blocks for translation systems. If they simply translate word-for-word, they can end up with funny or inaccurate results. It's important for algorithms to not just understand individual words, but to grasp the cultural context behind them.
The use of pre-trained models has significantly improved the capabilities of AI translation. These models are trained on vast quantities of text data and learn patterns in language, helping them become better at translating. However, their effectiveness depends heavily on the availability of data for each language. Some languages have more training data, leading to better translation quality, while others are less well-represented.
Integrating OCR technology with NLP is also an interesting development in AI translation. OCR extracts text from images, making it easier to process visual content like scanned documents or images. But the accuracy of OCR varies widely depending on the language. For instance, languages with intricate writing systems, like Chinese or Arabic, can be harder for OCR to decipher, which can lead to translation errors.
Deep learning has definitely increased the speed of translation, but it has also created a tradeoff between speed and accuracy. Fast translation systems might not always produce the most nuanced or contextually accurate outputs. This is particularly true in scenarios where there are subtleties in language or culturally rich expressions, where understanding the underlying meaning is key.
Fortunately, some new AI translation models can learn from user feedback, adapting their translations in real time. This could potentially improve translation accuracy over time as the models learn individual user preferences. It's an intriguing area, but it also raises questions about how the models will maintain consistency and ensure user preferences don't unduly shape translations.
A significant challenge remains in handling language diversity. Models trained mostly on common languages may struggle with regional dialects or less commonly spoken languages. This underscores the need for more inclusive training datasets that capture the variety and richness of languages across the world.
Cultural nuances are still an issue for current AI translation. Expressions that are meaningful in one culture often lose their impact when translated literally into another. This shows that AI translation, while promising, needs to incorporate more cultural understanding into its algorithms for truly accurate communication.
Finally, the use of AI translation tools raises important privacy concerns. Users provide potentially sensitive information when translating, so there's a need for more transparency about how this information is collected and used by AI translation providers. As AI translation technology continues to advance, it's important to consider not only the technological improvements but also the ethical and societal implications of these innovations.
AI Translation Embracing Uncertainty in Language Processing - CUBBITT Model Narrows Gap Between Machine and Human Translation
The CUBBITT model represents a noteworthy advancement in AI translation, bridging the longstanding divide between human and machine translations. Developed through a collaboration between researchers at Charles University and the University of Oxford, CUBBITT utilizes a deep learning approach within its architecture. This approach allows it to excel at capturing the essence of text, showcasing superior performance to human translators in certain instances, like translating English into Czech. While human translations are generally perceived as more fluid, CUBBITT has demonstrated improved fluency over earlier AI models, making it a potentially viable option for quick, dependable translations. This achievement highlights a developing understanding within the field that machine translation has the potential to effectively grapple with the inherent complexity and variability of human language. The ongoing development of this field leads to important inquiries about the future of AI translation in terms of finding the balance between rapid output and a comprehensive understanding of language nuances and cultural contexts.
Researchers from Charles University and the University of Oxford have developed the CUBBITT model, a deep learning system aiming to bridge the gap between machine and human translation. It challenges the notion that machines can't achieve the same level of quality as humans, particularly in tasks like preserving meaning during translation. Interestingly, CUBBITT has outperformed professional translators in certain scenarios, especially when translating English into Czech, demonstrating impressive performance in maintaining the core message of a text.
CUBBITT utilizes a standard encoder-decoder architecture, consisting of six layers for each component. The encoder converts sentences into numerical representations, allowing the model to understand the input, while the decoder generates the translation. Despite this success, human translation still holds an edge in terms of fluency, although CUBBITT has exhibited significant improvement in this aspect compared to previous top-performing AI translation systems.
To further assess CUBBITT's quality, human judges were tasked with a blind evaluation of the model's output versus professional translations. Their feedback highlighted that CUBBITT frequently yielded superior results in capturing the original text's context. These findings, published in Nature Communications, are a significant contribution to the field.
CUBBITT's abilities extend beyond English-Czech translations. It has been tested and validated on other language pairs, including English-French and English-Polish, indicating its broader applicability. The development of CUBBITT highlights the increasing focus on accommodating uncertainty and variations within languages, an area where AI translation is pushing boundaries.
While it seems the model is quite adept at understanding context, especially with the nuances of language, it remains to be seen how CUBBITT would manage with significantly different languages and how it could potentially integrate human-in-the-loop feedback to adapt in a meaningful way. This research pushes forward the boundaries of AI translation and underscores how important it is for machine translation tools to better accommodate a broader range of language structures and styles. The challenge remains on how CUBBITT would perform in translating very specialized language or dialects.
AI Translation Embracing Uncertainty in Language Processing - AI Translation Tools Bridge Global Linguistic Divides
AI translation tools are playing a crucial role in bridging the gaps between languages spoken globally. These tools, powered by machine learning and vast amounts of language data, can quickly translate text and speech, making communication across language barriers easier. While these technologies are improving rapidly, they still face limitations. A large number of languages are still under-represented in the training data used by these systems. This can result in inaccurate translations, especially when dealing with subtle cultural aspects or idioms. The expanding global interconnectedness makes powerful AI translation tools increasingly important for providing equitable access to information. Ensuring that these tools can effectively translate the full spectrum of human language, including lesser-known languages and their cultural nuances, remains a key area of development. As these technologies continue to evolve, it is essential to critically examine their impact on linguistic diversity, access to information, and global communication.
AI translation tools are increasingly being seen as bridges across the world's diverse linguistic landscapes, but they still have a long way to go before truly overcoming the barriers they aim to eliminate. While many tools can translate between a handful of popular languages, a huge portion of the world's languages remain underserved. This is a significant challenge as the accuracy of AI translation often relies heavily on the availability of training data, and many languages simply don't have enough readily available online text.
The accuracy of text extraction through Optical Character Recognition (OCR) also varies widely across different languages. While tools might be able to accurately extract text from documents in English or French, dealing with scripts like Chinese or Arabic often leads to a noticeable drop in accuracy, affecting the overall translation process.
Interestingly, some of the newer AI translation tools are experimenting with real-time feedback mechanisms. This user-centric approach allows the translation systems to learn from individual preferences and adapt to user behavior, potentially leading to more tailored and effective translations over time.
However, one major hurdle for AI translation systems is accurately interpreting context, especially when words have multiple meanings. While NLP algorithms are getting better at analyzing language, it's still difficult for them to consistently differentiate between various shades of meaning based on the surrounding text, leading to mistakes in translation.
This challenge is especially acute for languages with fewer online resources. Many indigenous dialects or less-common languages struggle to get accurate translations because the AI models haven't been trained on enough data from those languages.
The introduction of transformer-based models has accelerated translation speed dramatically, but this increase in speed sometimes comes at the cost of nuanced understanding. Culturally rich language or phrases with intricate meanings are sometimes simplified or misrepresented in the rush for rapid outputs.
Despite improvements in AI-powered translation, capturing cultural nuances and context within language remains a significant challenge. These systems can often deliver literal translations that fail to convey the intended emotional or contextual tone of the original text, which can lead to misunderstandings.
Additionally, we can't ignore the issue of bias within the datasets used to train these AI models. Datasets might reflect existing societal biases, which can lead to the perpetuation of inaccurate or harmful stereotypes in translation outputs. The responsibility falls on developers to carefully curate these datasets and ensure they reflect a wider range of linguistic and cultural backgrounds.
Rather than eliminating the need for human translators entirely, AI systems are increasingly being used as a collaborative tool. They can provide quick initial translations that are then reviewed and refined by human experts to ensure accuracy and adherence to the original message.
One fascinating aspect of AI translation is that these systems seem to perform better with specific kinds of language, like legal or technical documents. Possibly because the language in these domains is more structured and precise, which AI systems can parse more accurately. Casual conversation, on the other hand, can be trickier to handle, with its slang, colloquialisms, and diverse communication styles.
In summary, AI translation is an evolving field showing great promise in connecting people across languages. However, it still faces significant challenges in understanding the complexities of human language and accurately capturing its various nuances. The ethical considerations surrounding training data, potential biases, and the need for human-AI collaboration highlight the ongoing importance of carefully monitoring the development of these tools for the benefit of everyone.
AI-Powered PDF Translation now with improved handling of scanned contents, handwriting, charts, diagrams, tables and drawings. Fast, Cheap, and Accurate! (Get started for free)
More Posts from aitranslations.io: