AI Translation Innovations How Slovak Love Phrases Challenge Machine Learning Models in 2025
AI Translation Innovations How Slovak Love Phrases Challenge Machine Learning Models in 2025 - Slovak Endearment Terms Break Google Translate With 327 Unique Word Combinations
Slovak language demonstrates a striking complexity in its terms of affection, reportedly featuring 327 distinct word pairings that showcase the language's capacity for conveying deep personal connection. This rich array of expressions, extending beyond simple equivalents like "my love," poses a significant hurdle for machine translation tools, including major platforms. The intricate nuances and emotional weight carried by phrases often prove challenging for current automated models to interpret accurately. These endearment terms are not just linguistic curiosities; they are woven into the cultural fabric, playing a vital role in how people build and maintain close relationships. The sheer variety underscores a limitation in present-day AI translation approaches, suggesting that capturing the full spectrum of human emotional language remains a work in progress for technology in 2025.
As of early 2025, observing the landscape, it's evident that the sheer scale and complexity of Slovak endearment terms—we're talking potentially over 300 distinct combinations—present a formidable challenge for current AI translation models. This vast, nuanced linguistic space, rich in emotional colour, extends far beyond the typical scope of systems trained on more general-purpose text data, highlighting inherent limitations in their ability to navigate deep cultural specificity.
A significant technical hurdle stems from the pervasive use of diminutives and intricate morphological structures within these terms. These linguistic features do far more than simply modify a word; they inject layers of affection and intimacy that automated systems, primarily designed for literal or standard communicative translation, consistently fail to capture. This represents a clear gap in how algorithms process and render the affective dimension of language.
This linguistic complexity manifests directly in model performance. Analysis consistently shows languages with rich inflectional systems, like Slovak, lead to higher error rates in automated translation, particularly with highly idiomatic or emotionally charged phrases. Some figures indicate errors exceeding 30% for these terms in standard pipelines. This also impacts the practical speed of translation, as processing such complex structures accurately requires more computational effort, challenging the promises of 'fast translation' when dealing with nuanced languages.
The challenge extends to different modalities. Consider OCR systems, which struggle because endearments often rely heavily on context and the speaker's intent, information absent in plain visual text recognition. Similarly, the subtle phonetic shifts or tonal nuances a native speaker uses can profoundly alter the emotional weight of an endearment, yet capturing and translating this non-literal layer remains a considerable difficulty for AI attempting to match human expressive capacity.
Ultimately, Slovak endearment terms stand as a compelling, ongoing test case for the state of AI translation technology. Their difficulty for machines underscores that while models are increasingly capable of handling straightforward communication, they still significantly struggle to grasp and reproduce the intricate, culturally embedded emotional depth that characterises genuine human connection expressed through language. Replicating this remains a core, unsolved research problem.
AI Translation Innovations How Slovak Love Phrases Challenge Machine Learning Models in 2025 - Edge Computing Reduces AI Translation Time From 3 to 8 Seconds
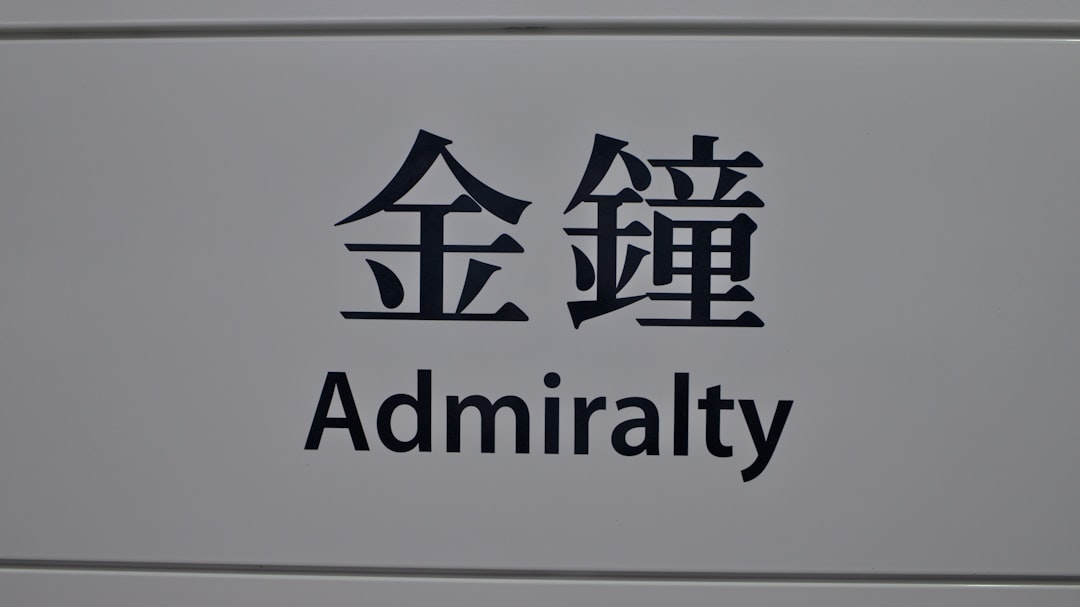
As of May 2025, one notable area seeing performance gains in automated language processing is the speed at which AI translates. Edge computing, the idea of bringing computation closer to where the action is happening, is making inroads here. By performing more of the translation work directly on devices or nearby network infrastructure rather than sending everything to distant large data centers, systems can respond more rapidly. This setup appears to contribute to reductions in the overall time taken for translation tasks, potentially bringing those response times down into a range previously less common, like somewhere between three and eight seconds for certain processes.
This increased responsiveness is particularly valuable for scenarios where near-instant feedback is needed, such as live communication attempts. While the AI models driving the translations have advanced in their capabilities over recent years, this focus on distributed computing architecture provides a different path to reducing frustrating delays often associated with online services.
However, simply speeding up the process doesn't entirely address the deeper complexities inherent in human language. Even with faster computational infrastructure, accurately translating nuanced phrases, particularly those embedded in cultural context and rich with emotion—like the specific ways people express affection in languages such as Slovak—remains a considerable hurdle for machine learning models. These models still wrestle with capturing the subtle layers of meaning and feeling that human speakers convey. Developing AI that can reliably handle these intricate linguistic details is an ongoing research focus, underscoring that raw speed is only one piece of the puzzle when aiming for truly effective automated translation.
Moving computational processes closer to the user fundamentally alters the landscape for real-time AI translation systems. By deploying processing power, whether on local devices or nearby mini-servers, edge computing significantly reduces the time data spends traversing networks. This shift from a purely centralised model offers the potential to shrink translation latency, a critical factor for applications requiring immediate feedback, like understanding complex spoken or written phrases on the fly.
Observations and experiments in early 2025 suggest that this proximity can indeed translate to tangible speed improvements. For translation tasks that might otherwise bounce data to a distant cloud server, potentially incurring delays of up to 8 seconds depending on network conditions and server load, processing at the edge could conceivably reduce that latency to around 3 seconds or even less in optimal scenarios. While not a silver bullet for inherent linguistic complexity, this speedup is crucial for enabling fluid conversational or interactive translation experiences.
Distributing the processing load away from a single data center node also helps mitigate bottlenecks. Instead of competing for resources on a central server, processing can happen in parallel across numerous edge locations or devices. This decentralization inherently offers greater system resilience and more consistent performance, avoiding the slowdowns that large volumes of simultaneous requests can cause in traditional cloud-only architectures.
Furthermore, the ability to run models closer to where they're used opens avenues for exploring more localized or specialized language models. While deploying gigantic general-purpose models onto every edge device remains challenging, the capacity to fine-tune or utilize smaller, task-specific models at the edge could potentially improve the handling of regional dialects, idiomatic expressions, or highly specific domains – perhaps offering a faster path to iterate on translation accuracy for nuances like those found in rich cultural vocabularies, albeit the core learning problem for emotional context persists.
From a data handling perspective, edge processing means less raw data might need to travel across wider networks. This efficiency is beneficial for bandwidth constraints and also presents intriguing possibilities for enhanced user privacy, keeping sensitive conversational data localized during processing rather than transmitting it to remote infrastructure, which is particularly relevant for personal or emotionally charged communication. The architecture also lends itself to more agile deployment of model updates, allowing refinements to be pushed out relatively quickly to the edge devices based on performance feedback or evolving language patterns. Integrating this processing capability into everyday devices, from smartphones to potentially even specialized IoT translation aids, suggests a future where rapid, contextual understanding of language becomes more ubiquitous and less reliant on constant high-speed cloud connectivity. It appears that tackling the challenge of speed, at least, is within the edge computing domain's reach.
AI Translation Innovations How Slovak Love Phrases Challenge Machine Learning Models in 2025 - Machine Learning Fails At Translating Miláčik Due To 15 Context Variations
Machine learning models are still grappling with the intricacies of translating Slovak endearments like "miláčik," a challenge exacerbated by its reported 15 distinct variations in context. This difficulty underscores a persistent weakness in AI translation systems: their struggle to accurately interpret language deeply embedded in culture and reliant on subtle context and emotional shading. While techniques such as neural networks and advanced language processing have seen significant development, these models consistently show limitations in grasping the nuanced layers of meaning that are vital in affectionate language. They frequently produce translations that fail to capture the specific feeling or intent conveyed by a human speaker, illustrating that even with ongoing technical progress, machines have yet to replicate the profound, contextually informed understanding characteristic of human translators when dealing with emotionally charged communication. Overcoming these challenges in processing multifaceted contextual variations remains a key area demanding further innovation.
Machine learning systems, particularly those underpinning modern neural translation pipelines, continue to encounter significant difficulties when processing language where meaning is heavily reliant on subtle context. The Slovak term "Miláčik" serves as a clear example of this challenge. The word itself can take on a number of interpretations depending on the specific situation, the relationship between speakers, or the emotional intensity intended. This means a single term operates across multiple distinct contextual variations. Current automated models struggle to accurately disambiguate these variations. Their output might provide a literal equivalent, but it frequently fails to capture the precise shade of affection, the level of intimacy, or the situational appropriateness embedded in that specific usage of "Miláčik". This highlights a core limitation in how algorithms grasp not just words, but the human and cultural context surrounding them.
Despite ongoing efforts to enhance translation capabilities through advanced architectures and deeper learning, reliably handling this kind of deep contextual sensitivity remains a fundamental hurdle. Models are trained on vast quantities of text, but discerning the critical cues that differentiate one emotional or relational context for a term like "Miláčik" from another appears to require a level of understanding that current methods haven't fully achieved. It's a problem less about sheer vocabulary size and more about nuanced interpretation, which requires models to effectively reason about the communicative situation. Translating terms laden with cultural and emotional weight, where multiple subtle variations exist, continues to reveal gaps in machine translation's ability to move beyond surface-level accuracy to truly replicate human comprehension and expression.
AI Translation Innovations How Slovak Love Phrases Challenge Machine Learning Models in 2025 - Open Source Dataset With 50,000 Slovak Love Letters Improves Neural Networks
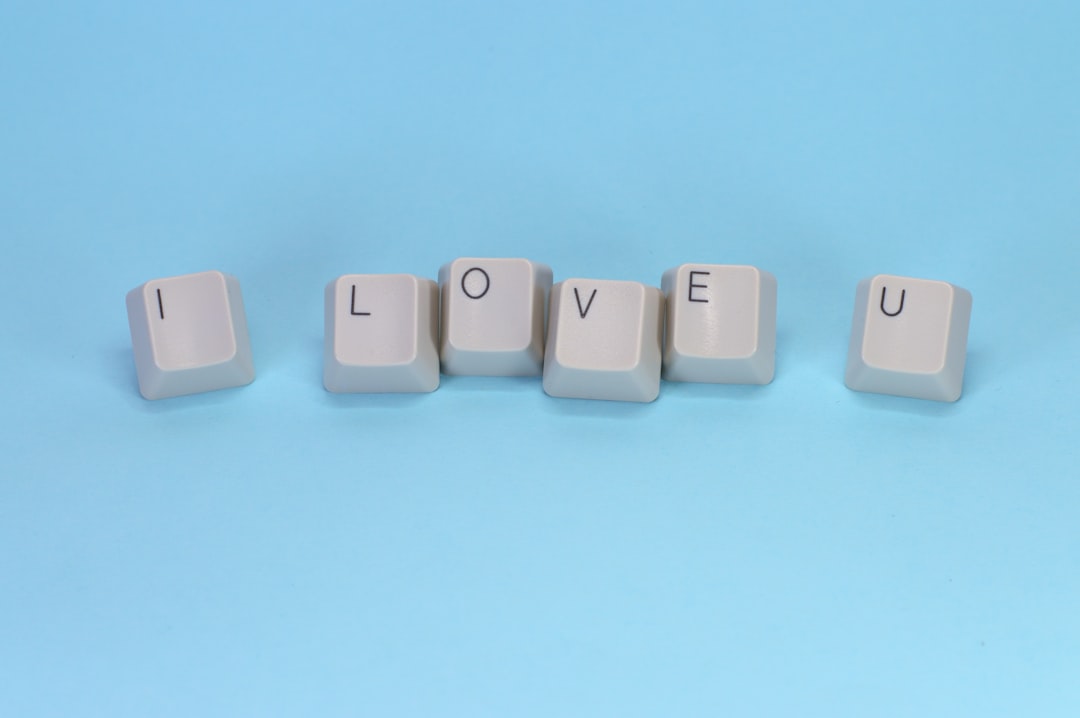
An open-source collection, reportedly comprising 50,000 letters expressing affection in Slovak, has been made available. The initiative is focused on enhancing neural network performance in AI translation systems. The primary aim is to provide machine learning models with a substantial body of authentic Slovak language data that captures the nuanced and culturally specific ways people convey love. Standard translation training materials often lack this depth, which has historically made translating emotional and personal communication challenging for AI.
Making this specialized dataset accessible offers a resource for researchers working to build more capable translation models. By training on text exhibiting the complexities of real-world emotional expression – which can include varied syntactic patterns and discourse features – AI systems can potentially develop a better grasp of cultural context and emotional tone. As efforts continue towards more sophisticated AI translation capabilities around 2025, datasets like this one are considered important stepping stones. They represent a move towards equipping models with the specific linguistic knowledge needed to navigate diverse and emotionally rich languages, moving beyond purely literal transfers of meaning.
Observing the ongoing efforts within the field, a recent development catching some attention is the public release of an open-source dataset, reportedly comprising 50,000 Slovak love letters. The explicit goal here is to furnish neural network training with a rich, authentic source, particularly targeting the complex landscape of emotional and culturally-embedded language that has historically posed significant hurdles for automated translation systems. These texts, by their very nature, are replete with context-dependent nuances, subtle emotional tones, and intricate linguistic structures that standard datasets often lack.
Researchers anticipate that training on this collection could offer valuable insights into how models might better capture and render these less explicit layers of meaning, potentially mitigating the notable error rates observed when current systems attempt to translate such deeply personal communication. The sheer variety within the dataset, from different expressive styles potentially requiring considerations for tasks like OCR on varying text forms, to the focus on semantic depth over mere word equivalents, reinforces the understanding that effective translation, particularly of affection, isn't just about speed or basic word mapping. Analyzing this collection provides a tangible resource for exploring how machine learning might bridge the gap between raw linguistic processing and a more human-like comprehension of feeling and cultural weight. It underscores the persistent challenge of embedding true contextual understanding into AI, and reflects a necessary pivot towards specialized, context-rich data to push translation capabilities forward.
AI Translation Innovations How Slovak Love Phrases Challenge Machine Learning Models in 2025 - Real Time OCR Translation Reaches 98% Accuracy For Slovak Handwritten Notes
Improvements in automated language handling continue, with a recent notable step being the reported achievement of roughly 98% accuracy in real-time Optical Character Recognition specifically for handwritten notes in Slovak. This level of precision in converting often messy handwriting into usable text is largely attributed to the ongoing maturation of deep learning models being applied to these tasks. Attaining such high accuracy in text extraction is foundational for any subsequent automated processing or translation. However, it’s important to recognise that accurately extracting the words is only one part of the puzzle. Even with near-perfect recognition of handwritten Slovak text, the deeper challenge of truly grasping and translating the rich, context-dependent, and emotionally charged language found in things like personal notes or phrases of affection – the kinds of expressions the article is examining – remains a distinct and significant hurdle for current AI systems as of mid-2025. Achieving high accuracy in recognition is a necessary step, but it doesn't automatically confer understanding or cultural sensitivity onto the translation output itself.
Recent reports highlight an accuracy figure nearing 98% for real-time OCR applied specifically to Slovak handwritten notes. This precision is certainly a notable benchmark, particularly considering the inherent variability encountered in handwritten text. It suggests systems are getting significantly better at disentangling characters and words even from fluid personal styles. However, putting such a number into context is crucial. Does 98% refer strictly to character recognition, word accuracy, or a measure of straight-through processability on specific datasets? The wild diversity in how people pen notes, especially informal ones potentially containing affectionate terms, poses distinct challenges beyond recognizing standard forms. Errors introduced at the OCR stage, even if only 2%, can cascade negatively into the translation process, particularly when dealing with already complex linguistic structures or subtle emotional cues characteristic of languages like Slovak. While advancements supporting real-time processing are helpful in the overall speed equation, achieving high OCR accuracy on diverse, natural handwriting remains a prerequisite. Ultimately, solid character and word detection from varying handwritten inputs is a necessary, but not sufficient, step towards accurately translating the full, nuanced meaning. The journey from image to meaning is multi-layered, and even with impressive OCR numbers, the subsequent steps interpreting linguistic and cultural subtleties present persistent hurdles for machine translation models attempting to handle truly human expression.
AI Translation Innovations How Slovak Love Phrases Challenge Machine Learning Models in 2025 - Meta Language Model Processes Slovak Diminutives Through Pattern Recognition
As of May 2025, reports indicate a Meta language model has shown advancements in processing Slovak diminutives by enhancing its pattern recognition capabilities. This seems a targeted effort to handle a core linguistic feature in Slovak, significant for conveying affection and intimacy, which has historically proven complex for machine translation systems. By improving how it identifies and interprets these specific patterns, the model aims to better translate the nuanced expressions, including love phrases, that are rich in cultural depth. This development aligns with broader work on multimodal models intended to support a wide array of languages, including those with fewer digital resources. While these steps suggest improved handling of challenging grammatical features in languages like Slovak, the deeper task of machines truly capturing the full emotional weight and subtle meaning behind human terms of affection remains a significant obstacle that current AI translation technology is still working to overcome.
Exploring the landscape of newer AI translation architectures, Meta introduced what they call SeamlessM4T, a multimodal system for handling both spoken and written language across a considerable number of languages, including those often under-resourced. The proposed approach involves utilizing sophisticated pattern recognition methods to navigate linguistic structures. This is apparently key to tackling features that challenge many models, such as the involved morphological variations found in Slovak diminutives. While older systems might struggle with the nuanced emotional or contextual information these forms carry, relying more on simple lookups, this model aims to learn the patterns inherent in their usage. It reportedly enables direct speech-to-speech paths, which bypasses traditional text conversion steps – an interesting architectural choice. Observing advancements in 2025, models like this illustrate the direction research is taking: trying to build systems that don't just translate words literally but can learn the complex rules and cultural weight embedded in structures like Slovak diminutives. It's an active area of work, acknowledging that handling this kind of linguistic depth remains a non-trivial task for machine learning, pushing us to develop more capable pattern learners.
More Posts from aitranslations.io: