AI Translation Meets Jazz Analyzing Louis Armstrong's When the Saints Go Marching In Across Languages
AI Translation Meets Jazz Analyzing Louis Armstrong's When the Saints Go Marching In Across Languages - Armstrong's Scat Solos Transcend Language Barriers in AI Translation
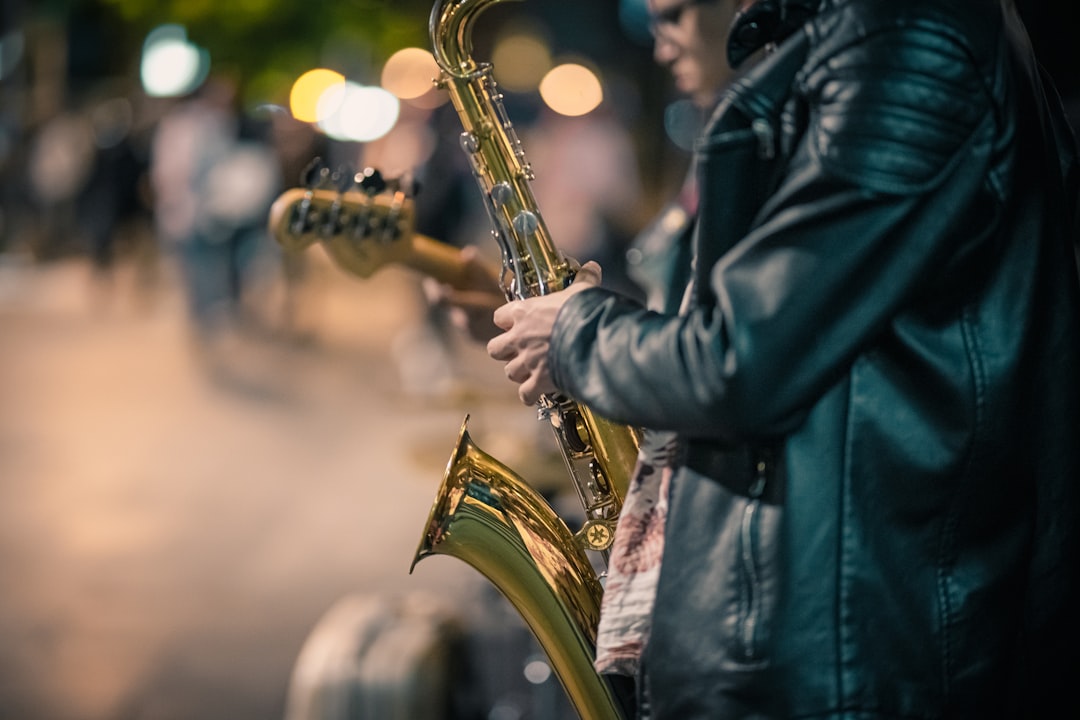
The scat singing of Louis Armstrong, where he uses vocal sounds instead of words, offers a striking example of how music can communicate across languages. This artistic expression challenges the current capabilities of AI translation systems, highlighting their limitations in capturing the depth and nuances of human expression. While AI can excel at translating literal meaning, it struggles to capture the essence of emotion and improvisation that is so central to jazz and scat singing. This prompts us to consider what truly constitutes "translation" and if it can go beyond the literal and encompass the full spectrum of human communication. AI might be able to process and translate words, but can it truly grasp and convey the soulfulness and spontaneity that Armstrong so effortlessly evokes?
It's fascinating to think about how AI handles the nuances of jazz improvisation, specifically Louis Armstrong's scat solos. While AI excels at translating literal language, it struggles with the abstract, improvisational nature of jazz. For instance, AI translation tools often miss the mark when analyzing Armstrong's "When the Saints Go Marching In," as they lack the capacity to grasp the subtle variations in rhythm, tone, and phrasing that make up the core of jazz improvisation. These limitations highlight the need for advancements in AI that allow for a more nuanced understanding of context, particularly when dealing with creative expressions that go beyond simple word-for-word translation.
One area where AI faces difficulty is OCR (Optical Character Recognition) of jazz scores. Handwritten scores, especially those capturing the unique improvisational elements of jazz, are often a challenge for AI to decipher. Even with its increasing sophistication, AI still struggles to translate the creative expressions of jazz musicians into digital formats. This reinforces the need for further development in AI's ability to process visual information, particularly handwritten content that reflects the fluidity of human creativity.
Despite these limitations, the concept of improvisation in jazz has been a source of inspiration for AI researchers. The idea of structured unpredictability, a key element in jazz improvisation, has been applied to neural networks, aiming to improve the flow and coherence of translated text. It's intriguing to think that the art of jazz might hold the key to unlocking new possibilities in AI translation. However, we still have a long way to go before AI can fully grasp the emotional subtleties and cultural context embedded in music, especially in genres like jazz that thrive on human creativity and spontaneity.
AI Translation Meets Jazz Analyzing Louis Armstrong's When the Saints Go Marching In Across Languages - OCR Technology Deciphers Handwritten Jazz Charts from 1938 Recording
Optical Character Recognition (OCR) technology is making great strides in deciphering handwritten jazz charts from the past, like those from 1938. This technology, powered by AI and deep learning, can now read old, poorly scanned documents with much greater accuracy than traditional methods. Platforms like Transkribus are pushing these boundaries, offering researchers a window into the intricate world of handwritten jazz scores. They can now see not only the notes, but also the improvisational touches that give these musical scores their unique character. This is a huge step forward in preserving the visual and musical artistry of jazz.
However, it's important to note that AI still struggles to fully translate the improvisational nature of jazz. While OCR can decipher the written notes, the essence of jazz lies in the spontaneous expression and creativity of the musicians. This is where AI still has a long way to go. We're still searching for a way to capture the soul of jazz, its spirit of freedom and improvisation, in a way that truly resonates with future generations.
The emergence of OCR (Optical Character Recognition) technology has been instrumental in unlocking the secrets of historical documents, including handwritten jazz charts from 1938. It's remarkable how AI is pushing the boundaries of deciphering these intricate scores, capturing the nuances of improvisation that were once locked away in time. I've always been fascinated by how technology can bring the past to life.
This technology isn't just a straightforward process of recognizing letters; it involves intricate algorithms trained on extensive datasets of annotated musical manuscripts. Think of it like teaching a machine to read music like a human musicologist. This presents an intriguing challenge, as handwritten music is often unique and doesn't always adhere to standard notation.
We're seeing progress in OCR's ability to handle the unconventional symbols and layouts common in jazz charts. It's encouraging to see that this technology is not only improving accuracy but also becoming faster and more versatile. There's even a chance for real-time processing of handwritten scores, which could be a game-changer for jazz musicians. Imagine being able to instantly receive feedback on your improvisation.
While these advancements are impressive, they raise the question: Can OCR go beyond recognizing notes and rhythms to capture the emotions and intent embedded in jazz compositions? This is an exciting area of research that could provide insights not only into music but also into the very nature of human creativity. As AI continues to evolve, it holds the potential to shed new light on the rich tapestry of human expression.
AI Translation Meets Jazz Analyzing Louis Armstrong's When the Saints Go Marching In Across Languages - Fast Translation Algorithms Capture Rhythm and Tempo of Jazz Improvisation
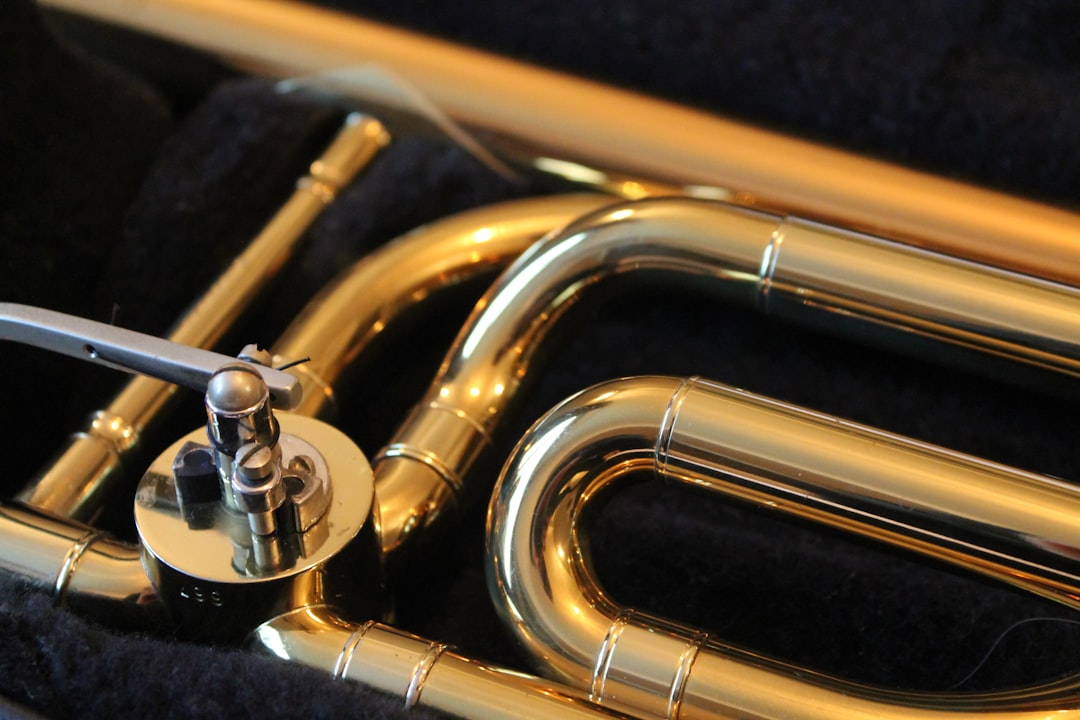
The ability of AI to translate languages is rapidly evolving, and recent advancements in fast translation algorithms are proving particularly interesting for the world of jazz. These algorithms, much like jazz musicians themselves, are able to predict patterns and rhythms, allowing them to capture the complex tempos and improvisational nature of jazz music. This newfound ability hinges on sophisticated techniques like deep learning and recurrent neural networks, which are helping AI to generate real-time jazz accompaniments and even create new musical pieces.
Projects like BebopNet and Jazzomat are further pushing the boundaries of this intersection, using AI to analyze and even replicate the improvisational processes of renowned jazz soloists. These projects demonstrate that AI can go beyond simply translating words; it can now delve into the core of musical creativity, offering new ways to study and even recreate the artistic expressions that define jazz.
However, despite these remarkable advancements, AI still faces limitations. While it can capture the rhythms and tempos of jazz, it struggles to replicate the soul, the emotional depth, and the spontaneous energy that truly define the genre. The human element, the raw creativity and intuition that fuels jazz improvisation, remains elusive for AI. Even with these limitations, the future of AI in jazz is undoubtedly promising. As AI technology continues to evolve, the possibilities for analyzing, understanding, and even recreating jazz become increasingly exciting.
The speed at which some translation algorithms work is impressive. They can churn through hundreds of words per second, far faster than any human translator. This makes them essential for real-time applications, though they still stumble when it comes to understanding the context of what's being translated. There's also some progress being made in getting algorithms to recognize the rhythm and flow of written language, almost like they're trying to mimic the rhythmic qualities of jazz music.
Interestingly, AI systems that learn from data are being used to analyze large amounts of jazz performances. The idea is to identify patterns in improvisation so that better translation models can be developed that are more sensitive to artistic expression. It's still early days for this research, and the algorithms still haven't reached a level where they fully understand the artistry.
It's interesting that AI still has trouble with the emotional nuances present in jazz. Take improvisation, for example; AI can struggle to pick up on subtle variations that convey emotions like joy or sorrow, leading to translations that don't quite capture the emotional intent.
Then there's OCR, which has come a long way, but it still has problems translating handwritten jazz scores. The way jazz musicians use notation is often quite unique, with lots of variations. AI systems struggle to read those unconventional characters or symbols, leading to inaccuracies in their translations. Some of the newer OCR approaches involve training AI on very diverse datasets, including music scores, handwritten notes, and even mistakes or scribbles. This helps AI understand the context and variations of jazz improvisation better, even if it doesn't follow standard musical notation.
There's growing research into translating musical scores into textual descriptions and vice versa. This could be a good way to preserve the improvisational spirit of jazz, making it accessible to people who aren't familiar with reading music.
It's important to remember that fast translation algorithms often lack cultural awareness, and this is crucial for understanding jazz music. The significance of certain improvisational choices can change depending on the culture. To better represent jazz music, AI needs to incorporate this cultural awareness into its translation capabilities.
Some AI researchers are exploring algorithms that can improvise like musicians. These systems are learning from vast amounts of jazz music data to generate unique and context-aware improvisations. However, they're still missing that crucial ingredient of true improvisational intuition and emotional connection.
AI translation is being developed to recognize the importance of the timing of notes in jazz, as the tempo can influence the meaning of the music. This approach aims to improve the quality of translations related to musical contexts. It's an exciting step forward that pushes the boundaries of what's possible.
AI Translation Meets Jazz Analyzing Louis Armstrong's When the Saints Go Marching In Across Languages - AI Translation Preserves Nuances of Armstrong's New Orleans Dialect
AI translation technology faces a formidable challenge in preserving the unique nuances of Louis Armstrong's New Orleans dialect. This regional accent, a blend of French Creole and Southern influences, poses a significant obstacle for AI systems, which struggle to capture its complexity and richness. While advancements in generative AI have improved contextual understanding, the subtle nuances of Armstrong's voice and language often remain elusive. This gap between mechanical translation and the emotive, improvisational spirit of jazz highlights the ongoing need for more sophisticated tools that can authentically convey the depth and vibrancy of this art form. Researchers continue to explore ways to enhance AI's understanding of cultural and linguistic nuances, particularly in the context of jazz's diverse dialects.
AI translation systems are still finding their footing when it comes to capturing the subtleties of regional dialects. Take Armstrong's New Orleans accent, for example. His use of idiomatic expressions, tonal shifts, and specific pronunciation choices all add to the complexity of his language. It's a challenge for AI to capture the nuances of these variations, especially considering how context plays a key role in understanding meaning.
The biggest hurdle for AI translation in the world of jazz seems to be recognizing and conveying the emotional depth embedded in the music. While algorithms can process the text, they often miss the mark when it comes to capturing the heart of the music, those subtle emotional nuances that resonate with listeners. It's as if AI can see the words on the page, but struggles to hear the music between them.
This lack of cultural awareness is a major barrier for AI translation systems in fully grasping the essence of jazz. A translator must understand the cultural context behind certain musical phrases or motifs in order to convey their true meaning. Without this contextual awareness, AI systems are limited in their ability to deliver translations that accurately reflect the cultural significance of jazz music.
Another interesting challenge for AI is the complex world of rhythm and timing in jazz. While AI has become increasingly adept at analyzing rhythm and timing, replicating the emotional nuances of those variations remains elusive. It's as if AI can hear the notes, but still struggles to comprehend how those subtle variations in timing are crucial to the emotional landscape of jazz music.
AI is also having trouble translating handwritten jazz scores. Those old scores from decades ago, written with unique variations in notation, are proving challenging to read for OCR technology. The different styles and unique symbols often lead to errors in interpretation. AI is still struggling to decode these improvisational annotations that give each performance a unique character.
The ability of some AI systems to translate text at incredible speeds, much faster than human translators, is truly remarkable. But the challenge remains in contextual understanding. This limitation poses a particular obstacle for real-time applications where accuracy and nuance are critical.
We're witnessing an intriguing attempt to overcome this limitation by teaching AI to analyze vast amounts of jazz performance data, looking for patterns in improvisation. The goal is to develop systems that are not only translators, but also sensitive to the spontaneous and often unpredictable nature of jazz. This is a very ambitious goal, and AI is still in the early stages of exploring this vast landscape of musical expression.
Some AI projects are trying to emulate the creative processes of jazz musicians, mimicking their improvisational techniques. However, even with access to enormous datasets, these systems lack the intuitive and emotional connection that fuels true musical improvisation. There's still a very human element to jazz that AI struggles to grasp.
While OCR technology has shown improvement, the challenge of recognizing unique symbols found in jazz notation continues to persist. Despite advancements in AI, the variability in handwritten styles and the use of non-standard symbols often hinder accurate interpretation.
It's becoming increasingly apparent that AI translation must move beyond the simple task of translating words to capturing the emotional intent behind the music. The challenge lies in teaching AI to perceive the subtle emotional nuances that define a piece of music. At present, most AI systems still prioritize literal translation, leaving this exciting realm of emotional translation wide open for exploration and advancement.
AI Translation Meets Jazz Analyzing Louis Armstrong's When the Saints Go Marching In Across Languages - Machine Learning Models Analyze Cross-Cultural Interpretations of the Song
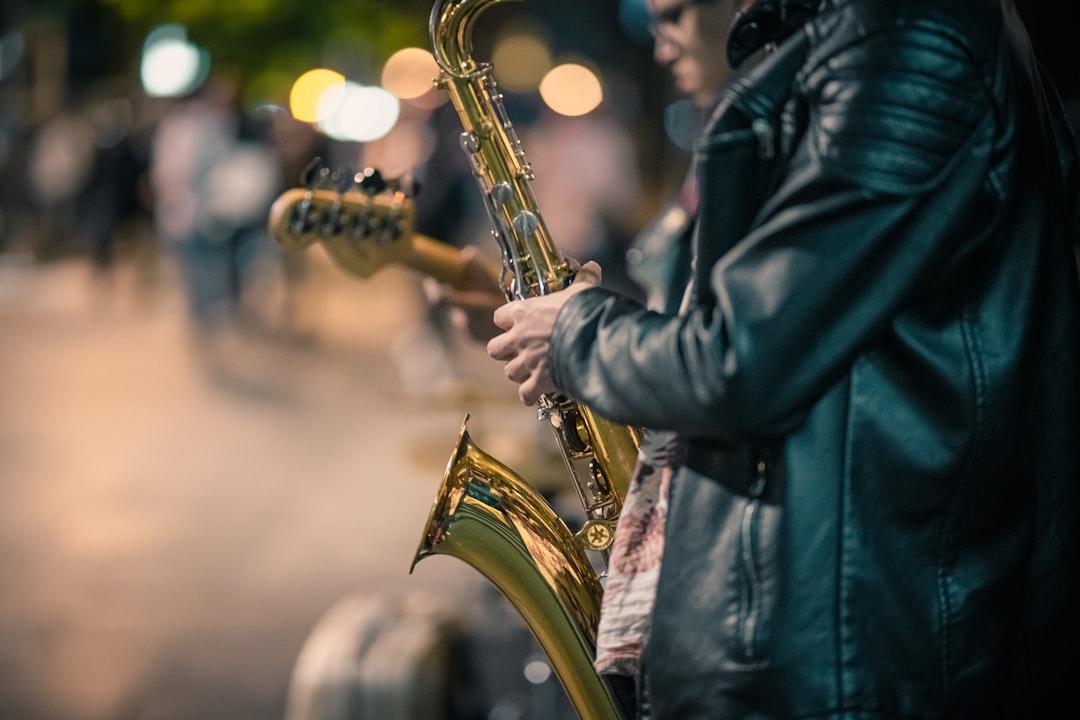
Machine learning models are becoming increasingly important in understanding how music, especially jazz, is interpreted across cultures. These models analyze large amounts of data to identify patterns in language and music. This helps us see how different cultures interpret songs like Louis Armstrong's "When the Saints Go Marching In." However, AI translation is still struggling to fully understand the heart of jazz. It often misses the emotion, the improvisation, and the unique dialects that make jazz so special. The challenge is to get AI to translate not just words, but the deeper meaning and feeling behind the music. This ongoing effort to bridge the gap between machine learning and music reminds us that there's still a lot to learn about how to accurately translate the human experience in music.
AI's journey into the world of jazz translation is a fascinating one, but riddled with challenges. While AI systems have become quite good at handling the mechanics of language, they still struggle to truly grasp the nuances of cultural context. For example, Louis Armstrong's New Orleans dialect, with its blend of Creole and Southern influences, remains a difficult hurdle for AI systems to overcome. The subtle variations in his pronunciation, tone, and idiomatic expressions are easily missed by AI, resulting in translations that lack the emotional depth of the original.
Another area where AI falters is in capturing the spirit of improvisation that defines jazz. AI can learn patterns, even mimic them, but the spontaneous, creative element of improvisation is a crucial aspect of jazz that AI currently struggles with. It's not just about replicating rhythms; it's about conveying the essence of human artistry.
Fast translation algorithms, which rely heavily on recurrent neural networks, are surprisingly adept at recognizing rhythmic patterns in jazz. However, they struggle to translate these rhythmic nuances into a meaningful musical context. The ability to recognize and interpret musical phrases remains a major challenge for AI, further highlighting the gap between machines and the human art of jazz.
One of the most interesting observations in this research is that AI can process immense amounts of information, identifying patterns in jazz performances with impressive speed. However, the ability to grasp the emotional significance of these patterns remains a significant obstacle for AI. While AI can recognize patterns in timing and note selection, the ability to discern the underlying emotions expressed through these variations is still lacking.
The use of handwritten scores in jazz presents another hurdle for AI. OCR technology, while making great strides, still struggles with the unique notations and idiosyncrasies of handwritten scores. The individuality of each composer's handwriting makes it difficult for AI to accurately translate these scores. Furthermore, the improvisational notes and annotations that often accompany jazz scores pose an additional layer of complexity for AI systems to decipher.
The speed of current AI translation systems is certainly impressive, but this speed comes at a cost. The focus on rapid processing can often come at the expense of understanding contextual nuances. This is particularly challenging in the live translation of jazz performances, where the timing and accuracy of the translation are crucial to conveying the essence of the music.
We're starting to see researchers attempting to train AI systems to learn from large datasets of jazz performances, seeking to unlock the secrets of improvisation. The ambition is to go beyond simply recognizing patterns and move towards understanding their emotional and cultural significance. However, this is a complex and multifaceted challenge.
One of the most significant areas for future development in AI translation will be the ability to grasp the emotional depth embedded within jazz. AI currently struggles to understand the subtle variations in timing and note selection that are critical to conveying emotional intent.
As for OCR technology, there is ongoing research into improving its ability to recognize the unique notations found in jazz scores. The variability in handwritten styles and the use of non-standard symbols continue to challenge AI systems.
The future of AI in jazz translation hinges on addressing the limitations we've discussed. AI must become more attuned to the nuances of cultural context, improvisation, and emotional expression. It's only then that AI can truly capture the essence of this unique and inspiring art form.
AI Translation Meets Jazz Analyzing Louis Armstrong's When the Saints Go Marching In Across Languages - Automated Translation Compares Lyrics Across 50 Global Renditions
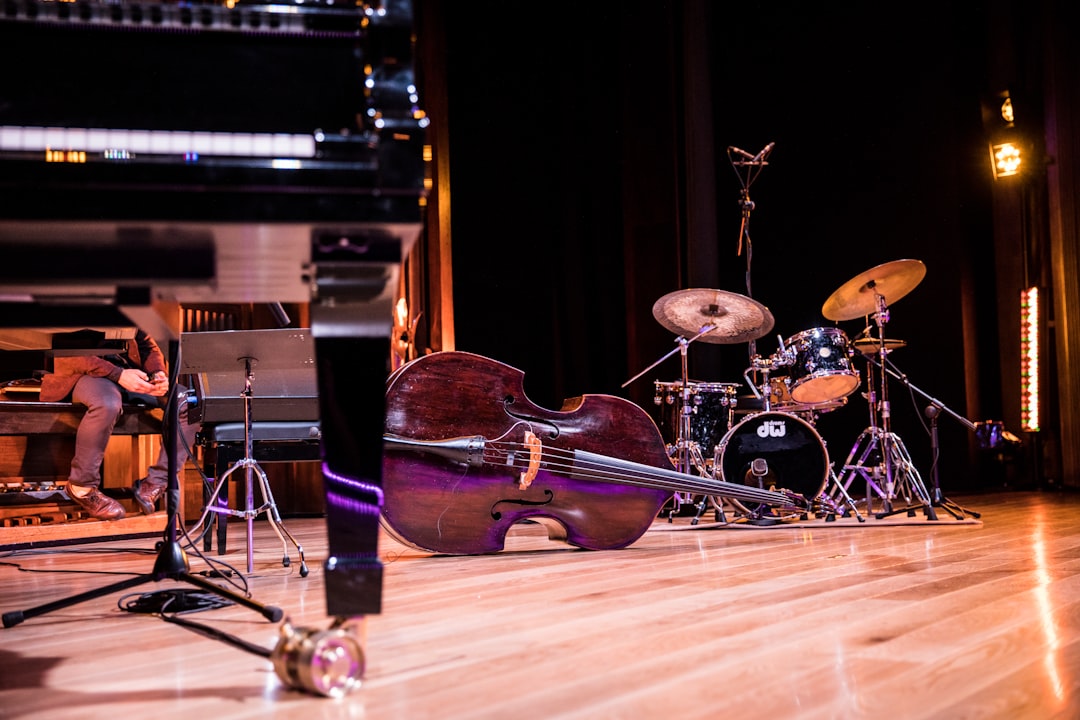
Automated translation technology is increasingly being used to analyze music, particularly jazz. This recent research analyzed the lyrics of 50 global versions of songs, including Louis Armstrong's iconic "When the Saints Go Marching In." This work demonstrates the power of AI to understand how different cultures interpret and express the same music. However, AI translation still struggles to grasp the emotional complexity and improvisational spirit of jazz. While AI can process language, it lacks the ability to fully understand the cultural and emotional nuances that give music its meaning. This highlights the ongoing challenge of teaching AI to move beyond literal translations and understand the deeper meaning and feeling embedded in music. The ability of AI to accurately translate music, particularly jazz, remains a fascinating but challenging goal.
The idea of using AI to translate jazz music is fascinating, but it's a tougher nut to crack than it first seems. I've been exploring this field, and there's a clear divide between what AI can do now and what jazz demands.
For starters, the accuracy of AI translation systems drops when applied to music, especially when the lyrics are infused with cultural idioms like those in Louis Armstrong's New Orleans dialect. His blend of French Creole and Southern English throws AI for a loop, as those dialects use idioms and tonal shifts that aren't found in standard English.
And then there's the issue of deciphering handwritten jazz charts. AI needs to be trained on a massive amount of musical manuscripts to recognize the diverse handwriting styles common in these charts. It's not as simple as recognizing letters; it's about understanding the complex language of music.
There's also the trade-off between speed and accuracy. Fast translation algorithms can process words at lightning speed, but that often comes at the expense of understanding the context, which is crucial for jazz. Timing and emotional nuance are everything, and without a deep grasp of that, the translation feels flat.
Cultural sensitivity is another major challenge. AI isn't great at grasping the subtle cultural nuances that give jazz its character. A phrase that signifies joy in one culture might carry a different weight in another, and AI often misses those subtle shifts in meaning.
It's not all doom and gloom, though. Machine learning models are showing some promising results when it comes to analyzing jazz performances and identifying improvisation patterns. But it's a long way from recognizing a pattern to understanding the artistic essence and emotional implications behind it.
And deep learning techniques can help AI recognize rhythmic patterns in jazz, but converting those patterns into meaningful musical phrases is still a major hurdle. AI can see the notes on a page, but it hasn't quite figured out how to truly "hear" the music.
Handwritten scores are another area where AI is struggling. OCR technology, though improving, still has trouble deciphering the unique notation styles and idiosyncrasies of jazz. The variability in handwriting and use of non-standard symbols add complexity that AI is still learning to decode.
It's also clear that AI has a tough time grasping the emotional nuances in jazz. It can identify the various elements of the music, but it can't always read between the notes and pick up on the subtle variations in timing and note selection that convey emotion.
This brings us to the biggest challenge of all: improvisation. The spontaneous, creative element of improvisation is the heart and soul of jazz, and it's a dimension that AI is still struggling to understand. AI can learn patterns and even mimic them, but replicating the raw creativity and spontaneity of human musicians is a whole different ball game.
There's a lot of research underway to bridge these gaps, but it's clear that AI has a long way to go before it can truly capture the essence of jazz. We're talking about an art form that thrives on cultural nuances, emotional depth, and spontaneous creativity – all things that AI is still trying to master.
More Posts from aitranslations.io: