AI Translation Models Bridging the Language Gap in Full-Length Films and TV Shows
AI Translation Models Bridging the Language Gap in Full-Length Films and TV Shows - M2M100 Model Revolutionizes Direct Language Translation
The M2M100 model introduces a novel approach to machine translation, directly linking any two of 100 languages without relying on English as a bridge. This capability unlocks a vast array of 9,900 language pairs, representing a major departure from earlier models often constrained by English-centric training data. By sidestepping the English intermediary, M2M100 aims for more accurate translations, reportedly improving scores considerably for non-English language pairs. This improvement in accuracy is expected to translate into more nuanced and contextually appropriate translations, a crucial factor when adapting full-length films and TV shows for diverse audiences. Furthermore, the open-source availability of M2M100 fosters wider adoption and potentially speeds up translation processes. Notably, the model's impact could be especially pronounced for less-resourced languages that have historically lacked sufficient bilingual datasets. This could lead to enhanced accessibility of media content and greater potential for authentic cross-cultural exchanges through film and television. While there are still unknowns, this development holds significant promise for expanding the reach of audio-visual content and facilitating communication across language barriers.
The M2M100 model represents a significant shift in direct language translation. It's the first model capable of translating between any two of 100 languages without requiring an English intermediate step. This approach fundamentally breaks from the traditional methods that relied heavily on English as a pivot point for many language pairs. Think about the massive increase in possible translations – this model theoretically handles 9,900 unique combinations, something that was a major limitation before.
The design itself relies on a sequence-to-sequence encoder-decoder architecture built specifically for this many-to-many multilingual context. It's been publicly released by Facebook AI, which is interesting from a research perspective as it allows for more rapid development and refinement. While its training data includes a massive set of webpages and documents, researchers are still exploring how well this approach handles nuances of culture or less common language structures.
Interestingly, results show that this model exhibits a noticeable increase in translation quality, achieving double-digit BLEU score improvements for direct translations between non-English languages. It's really interesting that models can achieve better accuracy when bypassing English altogether. For areas where data for certain languages is scarce, this approach could potentially create more equitable and effective translation solutions. This is a core benefit for projects involving full-length film or TV shows, where a focus on accurate and context-relevant translations across a broader set of languages is becoming more necessary.
Overall, it seems M2M100 sets a new standard for AI translation, particularly for languages historically lacking abundant bilingual data resources. We're still in the early days of exploring this model's full potential, especially as we're seeing a push to use this within media translation contexts that demand very fast turnaround. There's still work to do regarding optimizing performance for languages with limited training data. It will be interesting to see how this model further evolves as it's used more widely and researchers push it to address the challenges of even less-resourced languages.
AI Translation Models Bridging the Language Gap in Full-Length Films and TV Shows - Google's NMT System Enhances Accuracy in Film Subtitles
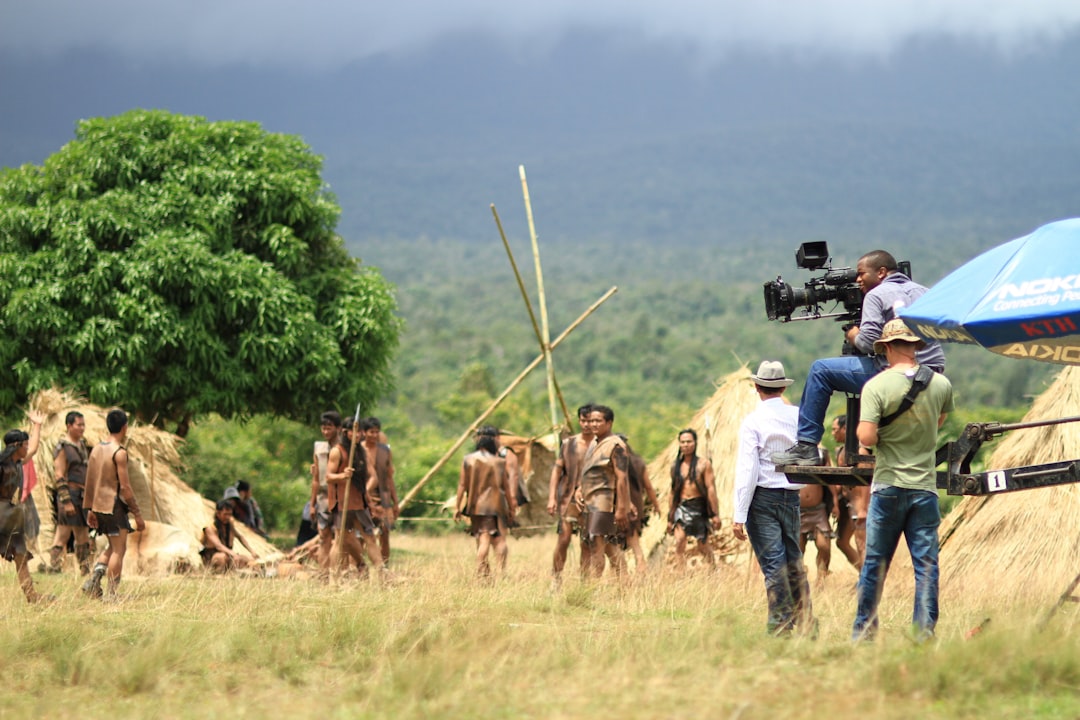
Google's Neural Machine Translation (NMT) system has significantly improved the accuracy of film subtitles by utilizing a more comprehensive learning approach. This method contrasts with older phrase-based systems, which often struggled with handling uncommon words and adapting to different sentence structures. NMT has proven highly effective, cutting down translation errors by a considerable margin, between 55% and 85%, across various widely spoken languages.
The advancements in NMT stem from techniques like beam search, which optimizes translation quality, and the system's unique ability to translate between language pairs it hasn't been specifically trained on (zero-shot translation). These features contribute to a more natural and fluid translation output. While NMT can be computationally demanding, particularly in real-time applications within film and media, it performs remarkably well with substantial datasets. It frequently outperforms older methods, offering a more reliable solution for translating film subtitles. Overall, Google's NMT is a powerful tool for making film and TV shows accessible across different language groups, fostering better understanding and communication between cultures.
Google's Neural Machine Translation (NMT) system has emerged as a promising approach to improving the accuracy of film subtitles. It leverages deep learning models, essentially intricate networks capable of processing and learning from massive amounts of data, to achieve a level of translation accuracy previously unseen in machine translation. This is particularly valuable in film subtitles, where capturing the nuances of dialogue, like humor or tone, is vital for audience comprehension.
One of NMT's intriguing aspects is its ability to learn from context. By examining the surrounding text, it gains a better understanding of common idioms or colloquial phrases frequently found in movie scripts. This contextual awareness leads to more natural-sounding translations, a key aspect in making subtitles feel less jarring and more integrated into the overall viewing experience.
The inner workings of Google's NMT system involve an attention mechanism that allows it to zero in on specific parts of the source text when creating the translation. This is especially advantageous for subtitling where precise timing is crucial for maintaining the flow of the film and viewer understanding. Additionally, NMT employs a technique called transfer learning to boost its performance. Essentially, improvements in translating frequently used languages can benefit less-common ones, which has positive implications for making media more accessible globally.
Another area where NMT excels is speed. It can churn through film subtitles incredibly quickly, processing entire movies within hours. This is a major improvement over older approaches that relied on manual translation, which could take weeks to complete. Incorporating Optical Character Recognition (OCR) further enhances the NMT pipeline by automating the process of extracting and translating text visible in video frames. This is useful for dealing with subtitles present within the film itself, like signs or captions.
The speed and automation inherent in NMT can potentially contribute to significant cost reductions in translation. Automating a large portion of the translation process limits the need for extensive manual revisions, making it a more budget-friendly alternative for film studios and distributors. Furthermore, there are efforts to explore NMT's capabilities for real-time translations. Imagine being able to instantly generate subtitles for live streams or broadcasts, creating a more seamless experience for a global audience.
However, as with any technology, NMT faces challenges. It sometimes struggles with highly specialized vocabulary or very local dialects, which raises questions about how well it can serve specific niche audiences. This can impact the localization process, as teams need to carefully balance the benefits of NMT with the need for very high-quality, culturally relevant adaptations. The constant training of the NMT models on diverse data allows the system to adapt to shifts in language and culture over time, ensuring it stays relevant to changing trends in film and media.
Overall, Google's NMT system is shaping the future of film subtitling and broader media localization. While not a perfect solution, the speed, accuracy, and automation it provides make it a powerful tool for overcoming language barriers and fostering a wider appreciation for cinematic works across the globe.
AI Translation Models Bridging the Language Gap in Full-Length Films and TV Shows - Real-Time Multilingual Translation in Video Conferencing
Real-time multilingual translation is rapidly transforming video conferencing, bridging the communication gap between individuals speaking different languages. AI-driven systems are now able to capture speech in one language and quickly generate accurate translations for participants, minimizing the potential for misunderstandings during international meetings. This capability relies on sophisticated algorithms that process and translate spoken words in real-time, making global collaboration much more feasible. While the technology is still developing, it's already having a notable impact on the user experience, offering a wider range of language support and enhancing the overall accessibility of video conferencing platforms.
The increasing integration of these translation tools highlights a rising need for efficient and inclusive communication in today's interconnected world. With people from various backgrounds engaging in online meetings more frequently, the demand for seamless cross-cultural interactions is growing. This drive is likely to see improvements in the translation accuracy and language coverage of these AI models, making real-time communication during video conferencing even smoother and more accessible in the future. Although the challenges of capturing nuanced cultural expressions and rare languages remain, real-time multilingual translation in video conferencing is showing great promise in removing communication barriers and fostering more effective global collaboration.
Real-time multilingual translation within video conferencing is a fascinating field that's rapidly evolving. It hinges on AI models, specifically recurrent neural networks (RNNs) and transformers, which are quite good at handling sequential data like speech. These models can essentially break down language structures on the fly, enabling instantaneous translation.
A big part of this technology relies on low-latency communication networks. Without these fast networks, the delay between someone speaking and the translation appearing would be too long, disrupting the flow of a conversation. Thankfully, with the expansion of 5G, this seems to be getting better.
It's interesting to see how things like Optical Character Recognition (OCR) are being integrated. OCR can grab text from the video feed, which can add extra context that the audio alone might miss, potentially improving translation accuracy. The idea of multi-modal translation is gaining traction too. By combining audio and visual inputs, we can get a more nuanced understanding of what's being said. This can really cut down on errors in those rapid-fire interactions of video calls.
One of the more surprising aspects of this technology is the speed at which it can operate. Some of these systems now deliver translation in under 300 milliseconds, which is incredibly close to human response time. That's a crucial element for ensuring that conversations feel natural and don't become stilted.
Of course, even with all this progress, these automated systems still have some limitations. Things like idioms and culturally specific references can trip them up. You might end up with translations that sound clunky or miss the true meaning altogether. That means there's still a need for human oversight to ensure accuracy, particularly for more critical communications.
On a more practical level, the cost of using real-time translation can be quite a bit lower compared to older translation methods. Automating parts of the process can lead to cost savings exceeding 50% in some cases, making this a very appealing option for businesses or organizations. Asynchronous translation is also becoming more common, where the system can handle and translate messages even if the speaker is not online at the same time, which is handy for overcoming time zone differences.
Researchers are also pushing towards more innovative methods. There's increasing work on end-to-end translation systems that can learn directly from real-time language use, without requiring a massive pre-defined set of bilingual data. This has the potential to simplify the training process, which could be especially helpful for less-common languages.
It's remarkable how far we've come from the initial days of machine translation, when systems weren't very good at capturing nuances. Now, models are utilizing attention mechanisms to better focus on specific parts of the input data, which leads to much more accurate and coherent results. These types of advancements are really crucial for maintaining smooth and effective communication during video conferences, especially when working with diverse teams and audiences across different languages.
AI Translation Models Bridging the Language Gap in Full-Length Films and TV Shows - Meta's NLLB200 Expands Language Coverage to 200 Tongues
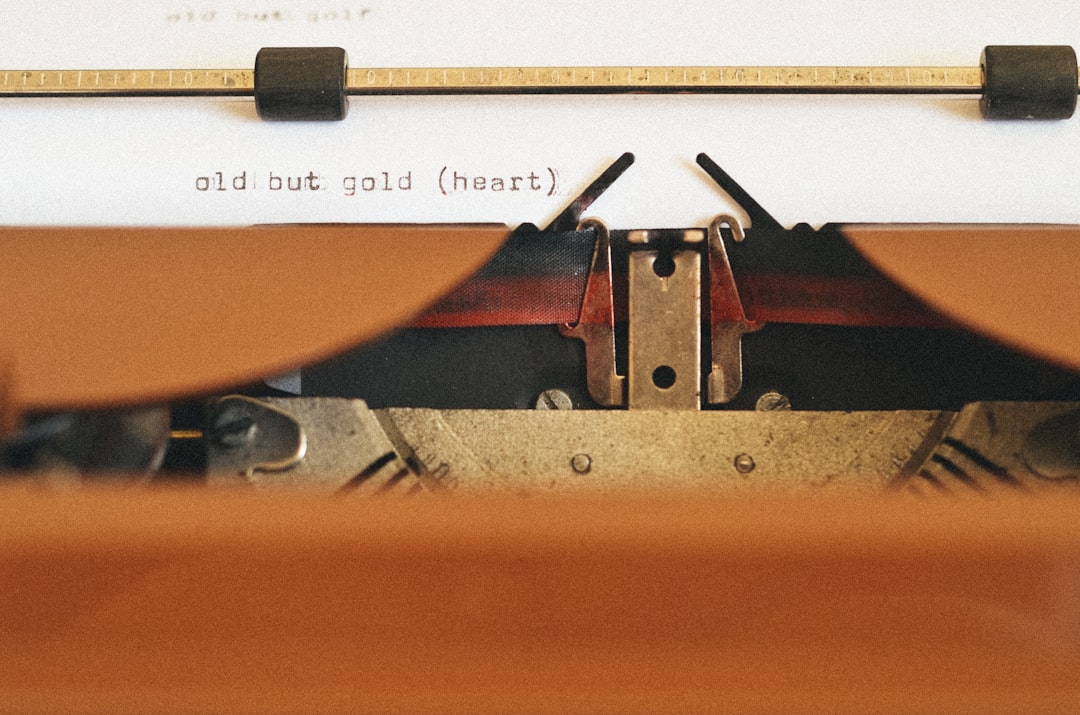
Meta's NLLB200 represents a leap forward in AI translation, expanding language coverage to an impressive 200 tongues. This model, built with a sophisticated 545 billion parameter architecture, focuses on efficiently handling real-time translations across a vast linguistic spectrum. A notable aspect of NLLB200 is its dedication to improving translation for languages often overlooked by traditional translation tools, especially in Asia and Africa, including languages like Lao and Kamba. The model is particularly noteworthy for its advancements in translating 55 African languages, significantly exceeding the previously limited selection of less than 25 languages offered by many translation tools.
Performance-wise, NLLB200 has demonstrated impressive results, exceeding comparable models by an average of 44% in benchmark tests. This accuracy is attributed to a dedicated evaluation dataset called FLORES200, specifically created for measuring multilingual translation performance. Meta's decision to open-source NLLB200 is noteworthy, offering researchers and nonprofits an opportunity to enhance the technology for various purposes, including expanding the reach of translated media, potentially facilitating subtitles and dubbing for a larger array of full-length films and TV shows. While challenges in accurately capturing cultural nuances and rare dialects persist, NLLB200 exemplifies a promising trend toward more inclusive AI translation, paving the way for broader global access and appreciation of media content.
Meta's NLLB200 model is quite impressive in its ability to tackle translations across a remarkable 200 different languages. This wide coverage is a big step forward, especially for languages that haven't had much attention from translation tools in the past. It's intriguing to see how well it can handle those lesser-known tongues.
The core of the model's design appears to be built on the same basic ideas as other advanced translation systems, relying on transformer-like structures to understand context and generate accurate translations. This focus on context is essential, especially for fields like media where subtle shades of meaning can impact how a story is perceived. In a way, it's trying to capture the true spirit of the original language, not just a literal word-for-word swap.
What's particularly interesting is that NLLB200 doesn't just rely on text to get the context. In certain cases, it seems to be capable of using visual information to understand what's going on, such as interpreting subtitles directly within a film or show. That's a clever idea that could improve things a lot for audiences used to a variety of languages.
The team working on this model has also considered the impact of dialects. By training it on a wider range of language variations, the goal is to deliver translations that feel more natural and culturally appropriate for specific groups of people. This is a valuable feature for media projects that are tailored for localized audiences.
One of the strengths of this model is its extensive training dataset. The researchers were able to feed it massive quantities of multilingual text from a wide range of areas, essentially teaching it specialized vocabulary found in different fields. This could have practical benefits for industries like entertainment or scientific research. It could help to generate more precise translations that aren't just generic word swaps.
The fact that NLLB200 can be further fine-tuned after it's deployed is quite insightful. This means the model can be continuously improved, a necessity in the world of media where languages and the way they're used are always in flux. It's adaptable to change, which is great to see.
Furthermore, the model pays close attention to low-resource languages, those that historically haven't had many tools available for translation. It's nice to see a focus on languages that haven't received the same level of attention as popular ones. This contributes to making digital content more inclusive for everyone.
It's designed with speed in mind, handling translations efficiently for real-time applications, which is a boon for live events or broadcasts. Having the capability to offer instant translation can greatly improve the experience for global audiences.
It seems the model is trying to streamline the translation process as much as possible, automating more steps than traditional systems. This could result in significant cost reductions for media production companies, allowing them to more easily reach a greater variety of audiences.
While NLLB200 shows a lot of promise, we should acknowledge its limitations. There are still situations where it struggles with things like cultural expressions or idioms. A straight translation sometimes misses the intended nuance or tone of the original language. This highlights the importance of maintaining human oversight in these types of systems to make sure that the translation truly resonates with the target audience. It would be interesting to see if that will be fully automated or a hybrid of human and AI in the future.
AI Translation Models Bridging the Language Gap in Full-Length Films and TV Shows - AI Models Improve Slang and Context Understanding
AI translation models are becoming increasingly adept at understanding slang and the broader context of language. These newer models, powered by advanced neural networks, are able to recognize and interpret idioms, cultural references, and subtle aspects of conversation that were often missed by earlier generations of AI translators. This enhanced comprehension results in more precise translations, particularly beneficial in media like films and television where accurately conveying the intended meaning and tone of dialogue is crucial for audience understanding.
While these improvements are significant, hurdles remain in dealing with the intricate nature of various languages, especially those with limited datasets or rare dialects. There's a continuing need for research and development to make sure these models can effectively handle a wider variety of linguistic styles and cultural contexts, thus further closing the gap in cross-cultural communication. The goal remains to create translation that is not just accurate, but also feels natural and culturally relevant within its target audience.
AI language models, particularly those leveraging neural networks like transformers, are becoming increasingly adept at understanding the nuances of language, including slang and context. This improvement stems from their ability to learn intricate patterns and grammatical structures from enormous datasets of text, which now often include informal communication styles found on social media and in popular culture. However, accurately interpreting the context of conversations, particularly those rife with slang, idioms, and culturally specific references, continues to present a challenge.
Recent advancements in AI-driven translation, particularly in the realm of real-time translation for video conferencing and similar applications, have shown significant leaps in contextual understanding. This is leading to a notable rise in the accuracy of translations across a range of scenarios. For example, OCR (Optical Character Recognition) has begun to be used with AI translation to allow for rapid interpretation of visual text within videos, allowing audiences to understand elements like street signs or onscreen text that are crucial to understanding the story. While impressive, these systems still require ongoing development, as some slang and colloquialisms can prove difficult for machines to process accurately.
While advancements are clear, many models are still primarily trained on datasets dominated by high-resource languages, primarily English. This can limit the effectiveness of these models when working with languages that have fewer digital resources. Interestingly, there's an emerging area where models seem to be learning to self-correct by observing user interaction with their output. While not fully mature, this capability suggests that AI models may eventually evolve beyond static training and become more dynamic, adapting to user feedback to improve the quality and accuracy of future translations.
Further complicating matters is the need for cultural sensitivity. Even the best current models can struggle to accurately translate idioms and cultural references that don't have a direct equivalent in another language. This raises questions about the ability of AI translation to preserve the spirit of the original text when translating materials meant for varied cultural contexts. It's likely that achieving truly effective intercultural communication will continue to require a balance of AI translation and human oversight, particularly in cases where culturally sensitive elements are critical to conveying the story effectively.
The pursuit of ever-improving AI language tools necessitates significant investment in both research and the development of robust training datasets. This area is still in its early stages, and continued research and experimentation are needed to fully realize the potential of AI for bridging the global language divide. There's a push to make AI translation more aligned with human values, a process termed 'pragmatic AI'. This concept aims to refine the technology so that it effectively communicates not just words, but meaning and intent in a manner that resonates with humans. Ultimately, the advancement of AI translation technologies is essential for fostering cross-cultural understanding and communication in a world increasingly interconnected through digital channels, including the rising use of film and TV to foster cross-cultural awareness.
AI Translation Models Bridging the Language Gap in Full-Length Films and TV Shows - HeyGen Platform Offers Rapid Video Translation in 75 Languages
HeyGen's platform utilizes AI to translate videos rapidly into 75 languages, potentially simplifying the process of making video content accessible across language barriers. It allows users to translate uploaded videos with a single click, resulting in videos with natural-sounding speech that can be customized for individual speakers. HeyGen also offers tools to edit and refine translations, and it boasts features such as avatar customization and voice manipulation to help enhance video creation. An intriguing new development is their voice cloning tool that can reproduce a person's voice and translate spoken content into seven languages. This tool offers a degree of personalization not typically seen in video translation. The advantage of using AI-powered methods like HeyGen is a significant increase in translation speed—as much as 100 times faster than manual methods. This efficiency and the ability to broaden video content's reach are making these types of platforms increasingly relevant to creators and distributors of international video content. While it's not without limitations—and much like other AI-based language tools, accuracy can vary depending on factors such as language complexity—it shows the potential of AI to reshape how we approach video localization and accessibility.
HeyGen's platform stands out with its ability to quickly translate videos into 75 different languages, showcasing a notable advancement in AI-driven translation. This capability has the potential to greatly expand the reach of movies and TV shows, making them accessible to audiences beyond those who speak the original language. It's quite interesting how easily it adapts content for languages that aren't frequently used in translation tools, potentially creating more opportunities for global film distribution.
One fascinating feature is HeyGen's capacity to grasp cultural nuances and idioms, leading to translations that feel more authentic and relatable. Instead of just a direct word-for-word translation, the output attempts to capture the spirit of the original, which is valuable when considering how humor or specific cultural references can be lost during simple translation. It's as if the platform tries to consider the context of the message in addition to the words themselves, making the content more engaging and enjoyable for the viewer.
The use of automation in platforms like HeyGen can drastically lower translation costs, potentially reducing expenses by half. This could make it much easier for content producers to localize their work for different regions without increasing their budget significantly. It's a compelling factor for anyone trying to reach a wider audience without creating a huge financial strain.
One of the most remarkable aspects of HeyGen is its ability to provide translations in real time. It's truly impressive that these AI systems can process and generate translated speech within about 300 milliseconds. This allows for natural-sounding and fluid conversations, which is especially important in the context of film dubbing or live broadcast translation. It's a leap forward in terms of ensuring that the experience for the viewer isn't interrupted by long pauses or awkward timing when a different language is introduced.
Furthermore, HeyGen incorporates OCR technology, enabling it to translate not just the spoken words in a video but also any visible text, such as signs, subtitles, or onscreen captions. This feature adds a valuable layer of contextual information that can significantly impact viewer understanding, making sure that no important details are lost. This integrated approach makes the translations more comprehensive.
HeyGen's multilingual support represents a change from traditional translation methods that frequently relied on English as an intermediary. It is a great example of how tools can try to avoid using English for translation. In a world with increasing global communication, being able to skip over English to deliver a translation directly to the audience in the desired language can be a real advantage.
Training these AI models on large and diverse datasets is crucial for improved performance. It allows them to learn a broader range of language styles, including slang, regional dialects, and conversational patterns, leading to more accurate and effective communication across various cultural groups. It's notable that these AI models are often trained with different types of text, including things like social media and blogs, which can aid in understanding how language is used today.
Another interesting development is the emergence of AI models that can learn from user feedback and adapt their translations over time. This kind of capability allows the AI model to continually refine its abilities based on real-world interaction. It opens the possibility for AI models to become more dynamic and personalized over time, adjusting to shifts in language usage and culture.
The promise of real-time translation extends to live events, making it possible to seamlessly facilitate multilingual communication during conferences, concerts, or broadcasts. This has important implications for international business and entertainment, as they can significantly improve the experience for audiences with diverse language backgrounds. It seems the future of live communication could see more multilingual options to suit the ever-increasing number of people online.
While AI-driven translation platforms like HeyGen have seen tremendous advances, there are still limitations. It's particularly challenging for them to handle specialized jargon or local dialects that might only be used within a specific community. This highlights a continuing need for further development in the field to ensure that these tools can adequately translate content that's culturally and contextually relevant. It remains to be seen how this aspect will evolve, whether through advanced training methods or human oversight.
In essence, HeyGen's platform and other AI-driven translation tools are a testament to how far this technology has advanced, opening the door for greater accessibility and understanding of media content on a global scale. While challenges still remain, it's exciting to see how they are transforming the way we communicate and interact with media from all over the world.
More Posts from aitranslations.io: