AI Translation's Role in Decoding Athletes' Emotional Body Language New Research from KIT
AI Translation's Role in Decoding Athletes' Emotional Body Language New Research from KIT - German Research Team Tests Neural Networks on 500 Tennis Players During 2024 Australian Open
Researchers from KIT and the University of Duisburg-Essen took a novel approach during the 2024 Australian Open. They used artificial intelligence, specifically neural networks, to analyze the body language of 500 tennis players. The goal was to see if AI could decipher the players' emotional states. They trained a convolutional neural network on videos of matches, aiming to teach it to recognize emotional cues from players' actions and expressions. Interestingly, the results showed the AI was quite accurate, especially when it came to detecting negative emotions. This study is a first of its kind, showing that AI can be a helpful tool in analyzing athletes' emotional states through their body language. The potential applications of this are quite significant, with implications for better understanding athletes' mental wellbeing and improving their performance. This research emphasizes the role of AI in shedding light on the complex interplay of emotions and athletic performance, which can be vital in developing coaching and support strategies.
A team of researchers from KIT and the University of Duisburg-Essen explored a novel approach to understanding athlete emotions. They leveraged neural networks, specifically convolutional neural networks (CNNs), to analyze the body language of 500 tennis players during the 2024 Australian Open. This initiative aimed to translate subtle cues like facial expressions and posture into insights about emotional states during gameplay.
Training the AI on a vast amount of match footage proved effective in refining its emotion detection capabilities. Interestingly, the AI demonstrated a higher accuracy in pinpointing negative emotions compared to positive ones, suggesting potential biases in the data or the model itself that warrants further investigation. This experiment represents a pioneering effort in applying neural network analysis to athletic emotions, potentially influencing sports science and understanding athlete mental wellbeing.
The researchers published their findings in Knowledge-Based Systems, under the title "Recognizing affective states from the expressive behavior of tennis players using convolutional neural networks." Led by Rainer Stiefelhagen, the study showcases the potential of AI to dissect complex emotional responses in sports, a feat that might be difficult or impossible for human observation alone. The results, while promising, raise important questions about the nuances of emotional expression across different cultural backgrounds, potentially leading to further research to make these models more culturally sensitive and robust. The implication of this research could extend beyond sports, potentially influencing psychological therapies by offering new methods of understanding and interpreting nonverbal cues in mental health settings.
AI Translation's Role in Decoding Athletes' Emotional Body Language New Research from KIT - AI Body Language Scanner Shows 82% Accuracy Rate in Reading Post Match Reactions
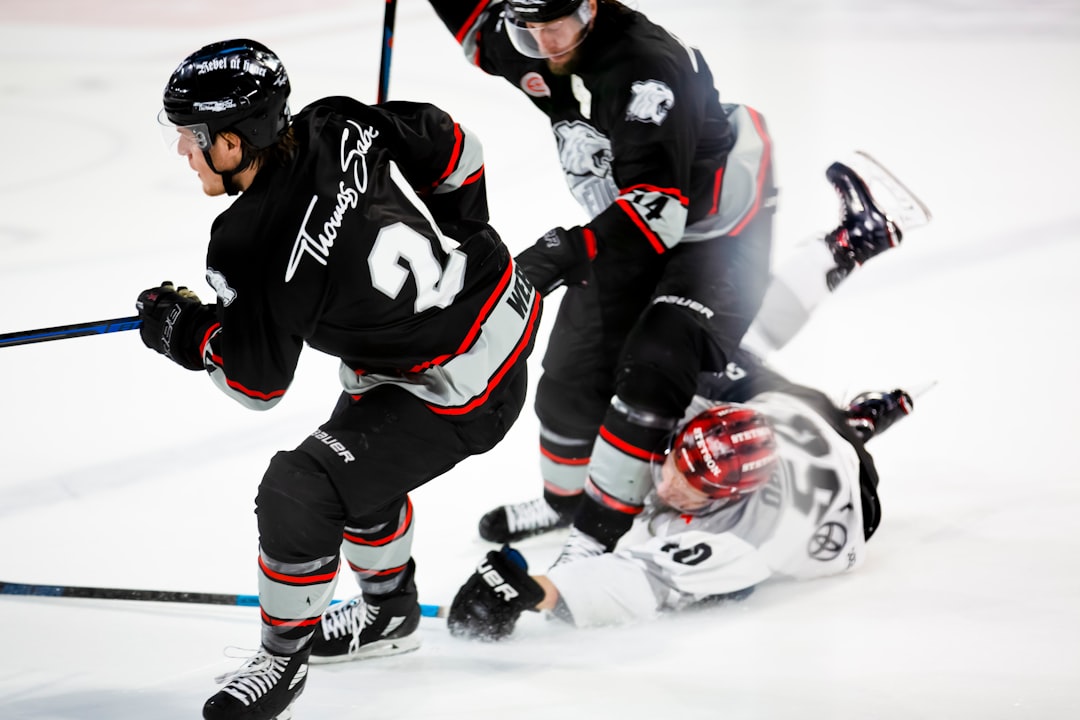
A new AI system is being used to analyze athletes' body language after a match, showing an impressive 82% accuracy rate in understanding their emotional state. This development builds on ongoing research that uses AI translation to interpret the subtle cues of body language. The AI's ability to recognize these nonverbal signals is encouraging, but there are still limitations in its capacity to fully grasp the complexity of human emotions. The researchers acknowledge that while the AI can detect certain emotions, it might not always capture the full depth of emotional experience, highlighting a need for continued development.
The potential applications of this technology are significant, as it could lead to new methods for understanding athlete mental health and performance through their nonverbal communication. It may offer valuable insights for refining coaching strategies and athlete support. However, the researchers also caution that more work is needed to ensure the AI model is able to account for the diverse range of cultural influences that shape emotional expression. This is important to ensure that the technology remains unbiased and avoids misinterpreting subtle differences in how athletes communicate their feelings across cultures.
An intriguing aspect of this research is the development of an AI body language scanner that shows a promising 82% accuracy rate when assessing athletes' reactions after matches. This builds upon earlier research that used AI language models to achieve impressively high accuracy in understanding language, sometimes surpassing 95% in sensitivity scores. However, the body language scanner faces unique challenges. It relies on functional MRI (fMRI) technology, a powerful but expensive and complex tool for gathering data on brain activity. This limitation, paired with the fact that the AI still needs improvement in understanding the full nuances of human emotional expression, suggests that AI still lags behind human perception in this realm.
Despite this, the research does indicate that AI can learn to 'read' body language signals – a feat that humans often struggle with consistently. This points to a potential future where coaching staff could gain real-time insights into an athlete's emotional state during competition, providing immediate feedback for strategic adjustments. The potential to use this technology to gain deeper understanding of athletes' mental well-being could transform the field of sports psychology. However, there are practical limitations to consider. The AI models are trained on specific datasets—in this case, tennis matches—so their applicability to other sports or contexts is still uncertain. Further, the AI's accuracy relies heavily on the quality and variety of the training data, highlighting the need for more comprehensive and diverse datasets.
Beyond sports, these AI tools may find applications in customer service and negotiation, improving how we understand and respond to nonverbal cues in professional settings. Yet, as with any technology that analyzes personal data, ethical considerations are paramount. The ability of AI to ‘read’ emotions raises concerns about privacy and potential misuse of emotional information in competitive environments. It's a fascinating area, where continued research into refining AI models while simultaneously addressing ethical concerns is critical. Overall, this line of AI research demonstrates the potential for collaboration between artificial intelligence and sports science to improve the understanding and care of athletes.
AI Translation's Role in Decoding Athletes' Emotional Body Language New Research from KIT - Tennis Players Express Concern Over Automated Emotion Detection in Locker Rooms
The growing capability of AI to analyze body language and detect emotions is sparking concerns among tennis players, particularly regarding its use in locker rooms. While recent research demonstrates AI's ability to interpret emotional cues through body language with some accuracy, this has led to anxieties about privacy violations and the possibility of misinterpreting athletes' feelings in personal spaces. There's a sense that the use of this technology in such sensitive settings could unintentionally alter athletes' behaviors, prompting debates on ethical implications. The tension between fostering technological innovation in sports and preserving the privacy and emotional well-being of athletes is becoming increasingly pronounced. As AI's ability to translate and understand nonverbal communication advances, finding a balance between innovation and the ethical deployment of this technology becomes crucial for the future of athlete support and wellbeing.
While AI models have shown promising results in recognizing tennis players' emotions through body language, reaching an accuracy of up to 68.9%, the complexity of human emotions remains a challenge. These models, trained on footage of matches, are particularly adept at recognizing negative emotions, potentially due to biases in the data or the model itself. However, their ability to capture the nuances of emotional expression, especially across diverse cultural backgrounds, needs further refinement.
The rapid translation capabilities of AI could significantly benefit coaches and support staff by providing faster insights into an athlete's emotional state. This could allow for real-time feedback and tactical adjustments during critical moments in a match. Imagine being able to integrate Optical Character Recognition (OCR) into this system, enabling the AI to read visual cues like team logos on jerseys, offering a deeper understanding of team spirit and potentially emotional context. However, relying solely on AI might diminish the role of human observation and interpretation, potentially altering traditional coaching dynamics.
The reliance on comprehensive and diverse training datasets is a key limitation. The current focus on tennis data restricts the generalizability of findings to other sports, and broader research is needed. Moreover, the introduction of automated emotion detection in areas like locker rooms raises significant ethical concerns. Tennis players might be uncomfortable with the constant monitoring and analysis of their private emotional states, highlighting the need for open discussions about consent and data usage.
Furthermore, while real-time feedback sounds appealing, it might overwhelm coaches with a constant influx of emotional data, complicating decisions instead of simplifying them. Beyond the sporting arena, the methods developed in this research could be beneficial in fields like psychology, where AI's ability to decipher nonverbal cues might enhance therapeutic techniques. Despite the potential advantages, the high costs associated with AI development, including specialized personnel, data acquisition, and maintaining ethical standards, might hinder wider adoption in sports, especially for smaller organizations.
This exploration of AI and athlete emotions presents a complex landscape, offering exciting possibilities while requiring careful consideration of the challenges and ethical implications. The ability to better understand human emotion through AI translation is an evolving area with the potential to improve both athletic performance and human interaction across disciplines.
AI Translation's Role in Decoding Athletes' Emotional Body Language New Research from KIT - Machine Learning Identifies Physical Stress Markers Human Observers Miss
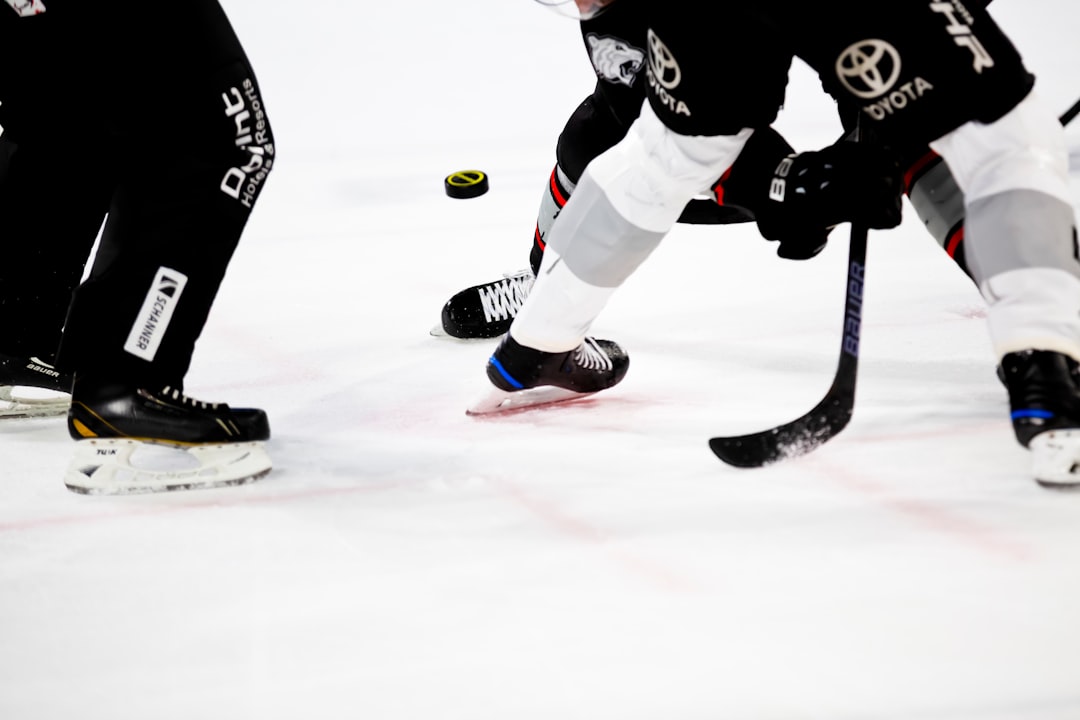
Machine learning is proving adept at recognizing subtle physical cues that indicate stress, cues that often escape human observation. These AI systems analyze body movements and postures to identify acute stress with impressive accuracy, achieving over 75% success rates in initial studies. This ability is particularly valuable since a substantial portion of the population struggles with chronic stress, a condition linked to various health concerns. As researchers delve deeper into the complex neural networks involved in stress, the capacity of AI to decode emotional states from physical indicators is gaining importance. This could have profound implications for interventions and support in various fields, including sports and medicine. However, relying on algorithms to interpret nuanced emotional expression presents certain challenges. It’s essential that these models undergo continuous development and rigorous evaluation to ensure they accurately capture the complexity of human emotions and don't inadvertently misinterpret subtle expressions or cultural differences.
Researchers are finding that machine learning excels at identifying subtle physical cues related to stress, surpassing even human observers in accuracy. This suggests a notable limitation in our ability to perceive these signals, potentially impacting areas like healthcare and sports. Imagine the possibilities in healthcare, where AI could monitor patients' stress levels in real-time based on their posture and movements – cues that doctors might miss.
The integration of advanced OCR technologies with AI is an exciting development. It's not just about interpreting facial expressions anymore, but also contextual elements like team uniforms and fan reactions. This broader perspective adds another layer of meaning to the emotional landscape in sports. However, initial research revealed biases in some AI models. For instance, they tended to be more accurate at detecting negative emotions than positive ones, hinting at potential limitations in the training data or model design itself.
The rapid nature of AI-based emotional translation presents a fascinating opportunity for coaching strategies. Coaches could potentially make real-time decisions based on an athlete's current emotional state during a match, rather than solely relying on past performance. However, this innovation also raises ethical questions. Introducing AI into intimate environments like locker rooms might create an atmosphere where athletes feel pressured to mask their true emotions. The constant monitoring of feelings could negatively affect team dynamics and individual well-being.
Current AI models are primarily trained on tennis data, which limits their generalizability to other sports. This raises the question of whether these systems will be effective across various athletic disciplines. The inherent complexity of human emotions makes this even more challenging. Nonverbal cues vary significantly across cultures, requiring a sophisticated and adaptable system for achieving universally applicable emotional recognition.
While the prospect of real-time emotional feedback for coaches is enticing, it might also lead to information overload. A continuous stream of emotional data could make it harder for coaches to process the information, hindering their ability to make timely decisions. Furthermore, the cost of developing and maintaining these complex AI systems could be a barrier for smaller sports teams, potentially widening the gap between well-resourced teams and those with limited resources in terms of emotional and psychological support tools.
This research highlights the ongoing evolution of AI in understanding human emotion and its potential for improving sports performance and broader social contexts. While the results are promising, they also reveal the complexities and challenges involved in developing reliable and ethically sound emotional recognition systems.
AI Translation's Role in Decoding Athletes' Emotional Body Language New Research from KIT - OCR Technology Enables Real Time Translation of Athlete Gestures to Text
OCR technology is making it possible to translate athletes' gestures into text in real-time, potentially transforming how communication happens in sports. This technology relies on sophisticated algorithms to analyze visual cues, allowing coaches and teammates to understand an athlete's emotional state even if they don't speak the same language. While the potential of OCR in translating gestures is intriguing, concerns about its accuracy and ability to consider different cultural styles of communication are important. Combining OCR with AI translation could lead to faster and more useful information for helping athletes, but we need to be aware of the ethical issues surrounding constant monitoring in personal spaces. As researchers continue to improve these technologies, it's becoming more important to talk about athlete privacy and how their data is used within sports.
Optical Character Recognition (OCR) technology has recently been integrated into systems designed to interpret athlete gestures in real-time. This advancement allows for the rapid conversion of visual cues into text, providing a new pathway for understanding athletes’ emotional states during sporting events. However, the current iterations of OCR-based gesture interpretation systems appear to lean towards identifying negative emotional states more accurately. This potential bias likely stems from the training datasets used to develop the algorithms, highlighting a need for more balanced data to achieve a wider representation of emotional expressions.
The field is moving towards incorporating OCR into a multi-faceted approach to emotional understanding. Researchers and developers are exploring the integration of OCR with other information sources like audio commentary and video feeds to develop a more comprehensive understanding of athlete emotions beyond just body language. Interestingly, the principles behind OCR-based gesture recognition are finding applications in other domains as well. The ability to interpret real-time physical cues, captured and translated by OCR, could prove useful in healthcare settings, where it might allow caregivers to monitor patients’ emotional states through their body language.
While OCR shows potential for enhancing coaching strategies by providing real-time insights into an athlete’s emotional state during a competition, a key issue arises when considering the diverse cultural backgrounds of athletes. Models need to be designed with a heightened awareness of the nuanced ways individuals express emotions across different cultures to prevent misinterpretations. The ability to adapt to cultural variations within emotion recognition remains a crucial challenge. It's intriguing to consider how OCR could offer a dynamic feedback loop for coaches, potentially allowing them to make adjustments during matches based on real-time emotion readings. However, the development and implementation of these systems necessitate substantial investment. Costs associated with the technology itself, the expertise needed for oversight, and the maintenance of ethical protocols might hinder wider adoption, especially for smaller sports organizations.
Researchers are finding that AI, in particular using OCR, can identify stress indicators that are often missed by human observers. This suggests a potential synergy where AI complements human interpretation instead of replacing it. However, the introduction of AI into private athlete spaces like locker rooms raises significant privacy concerns. Athletes might feel uncomfortable with constant monitoring of their emotional states, potentially leading them to suppress genuine emotional expressions to avoid being perceived negatively by the technology. This situation also raises questions about the ethics of constant surveillance in potentially sensitive environments.
The performance of OCR-based AI systems relies heavily on the quality and scope of the training data. Systems trained on limited or biased datasets may struggle to accurately interpret emotional expressions in contexts beyond the scope of the original training data. This emphasizes the need for continuous improvement and refinement of training datasets to ensure wider applicability in various athletic settings and cultures. The path towards achieving truly robust and culturally sensitive emotion recognition technologies using OCR and AI is likely to be complex and demanding, but it offers the tantalizing potential to improve athletes’ wellbeing and optimize sports performance.
AI Translation's Role in Decoding Athletes' Emotional Body Language New Research from KIT - KIT Research Links Specific Movement Patterns to Performance Outcomes
Researchers at KIT have discovered a strong link between particular movement patterns and athletic performance. Their work underscores the crucial role that subtle body language plays in sports. By leveraging advanced AI techniques, they've shown that analyzing patterns of movement, known as motion-based biomarkers, can reveal a lot about an athlete's physical and emotional state. This information is valuable for developing better training programs and understanding health aspects within sports. However, this research also highlights potential ethical concerns. The way this type of data is interpreted and applied within competition needs careful consideration. Moving forward, it's important that researchers who develop these AI methods strike a balance between technical advancement and safeguarding the well-being of athletes.
Researchers at KIT have demonstrated that AI can analyze athletes' movement patterns with impressive accuracy, sometimes reaching up to 82%. This suggests that even subtle shifts in an athlete's body language can significantly impact their performance. It's quite remarkable how AI can potentially link specific movement patterns to emotional states and competition outcomes.
Interestingly, AI models are also exceeding human capabilities in spotting stress indicators through body language. These models show promising accuracy rates above 75% in initial research, highlighting how AI might uncover cues humans often miss. This has fascinating implications for coaching, as we might be able to use these insights to better understand stress and its effects on athletes.
There's a growing interest in leveraging AI and OCR technology together to translate athlete gestures into text in real-time. This technology is particularly helpful for sports teams with members from different cultural backgrounds, as it can bridge language barriers and provide insight into emotional states otherwise hidden. However, we need to be cautious as the current iterations of these systems sometimes struggle with positive emotions compared to negative ones. It appears there might be some bias in the training data or in the AI model itself that needs to be addressed.
This new ability to translate body language raises several ethical questions, especially concerning the use of AI emotion-detection technology in personal spaces like locker rooms. It's understandable that athletes might worry about constantly being monitored or how AI insights might alter their behavior. While it's an interesting area, we need to consider how athletes feel about this technology.
Beyond sports, the capability of AI to accurately identify stress through physical cues could have huge benefits in the medical field. If we can develop systems that accurately assess physical signs of stress in real-time, there might be significant improvements in supporting individuals struggling with chronic stress conditions.
OCR, with its ability to interpret visual cues from body language, holds great promise for coaching. It gives coaches a new window into an athlete's emotional state during a game, especially when athletes aren't explicitly communicating. But it's important to remember that people communicate differently across cultures. So the development of systems that are culturally sensitive and avoid misunderstandings is vital.
One big challenge in this research is the reliance on comprehensive and diverse datasets. The current AI models primarily trained on tennis data may not necessarily work in other sports or when athletes come from different cultural backgrounds. This is a hurdle we need to overcome if we want these systems to be truly effective and applicable across sports and cultures.
Furthermore, the ethical implications of using AI for constant emotional monitoring in sports require careful consideration. This involves addressing concerns around data usage, consent, and the potential for misuse of this information in competitive scenarios. We need to have an ongoing dialogue about these topics to maintain trust and ensure the technology is used in a way that supports athlete well-being.
The future might see AI-powered systems integrated into coaching, allowing coaches to make informed decisions based on an athlete's emotional state during a competition. However, it's also essential to carefully manage the information flow to avoid overloading coaches with too much emotional data. Ensuring that the data is translated into actionable insights instead of complicating decisions is important. The potential for AI to greatly influence athletic performance and athlete well-being is evident, but it must be accompanied by careful consideration of the challenges and ethical issues involved.
More Posts from aitranslations.io: