AI Translation Solutions Debunking the Myth of Untranslatable Words in 2024
AI Translation Solutions Debunking the Myth of Untranslatable Words in 2024 - Machine Learning Tackles Japanese Onomatopoeia Word by Word in 2024
The year 2024 has witnessed a surge in machine learning's ability to handle the intricate world of Japanese onomatopoeia. These words, which capture sounds and sensations, have always posed a challenge for translators due to their often unique nature and lack of direct equivalents. AI translation methods, specifically neural machine translation (NMT), are showing promise in this area, but their effectiveness across languages is far from uniform. It's become clear that context is crucial for these types of translations. Machines are still learning to navigate the complexities of Japanese sound-symbolic language, where meaning is often tied to the sounds themselves. This evolving process of translation not only aims for greater accuracy but also leads to broader discussions about AI's impact on maintaining the subtle beauty and specific meaning within languages. As the need for global communication rises, specialized language resources coupled with more sophisticated machine learning tools are steadily increasing our capacity to better understand and translate these previously tricky words. The quest for effective and ethical translation continues, pushing the boundaries of what AI can accomplish in bridging language barriers.
Japanese onomatopoeia, encompassing both sound-imitating (giseigo) and state-imitating (gitaigo) words, poses a unique challenge for machine learning due to its intricate connection to feelings and actions. While earlier attempts at machine translation using statistical methods and recurrent neural networks only achieved modest success, recent progress in natural language processing is leading to more sophisticated solutions. AI systems are becoming better at interpreting the context surrounding onomatopoeia, enabling them to capture the intended emotional nuance rather than simply offering a literal translation.
Specifically, models trained on curated datasets of Japanese onomatopoeia have demonstrated a noticeable jump in translation accuracy, potentially reaching a 30% increase in some instances. This is achieved partly through convolutional neural networks (CNNs) which recognize patterns in onomatopoeia use across diverse contexts, adapting the translation dynamically. Further, integrating optical character recognition (OCR) with AI-powered translation allows for the processing of handwritten or stylized text, including onomatopoeia, opening up more informal communication for translation.
A particularly interesting application is the use of reinforcement learning, where the translation algorithms learn from user feedback and adjust their output in real-time for context-specific expressions. Sentiment analysis is another tool employed by AI, enabling adjustments to the translated onomatopoeia based on the emotional tone of the surrounding text, aligning the translation more closely with the original meaning. Moreover, some systems are even starting to differentiate between regional dialects in Japan, showing a greater understanding of the subtle variations in onomatopoeic expressions used in localized varieties of the language.
However, challenges persist. The polysemy of onomatopoeia, where a single word can have multiple meanings depending on context, continues to confound machine translation models. This underlines the ongoing need for development in this area. Fortunately, the advancements in hardware like more powerful GPUs have permitted training on larger and more complex datasets, resulting in faster translation speeds and the potential for real-time translation within applications focused on Japanese onomatopoeia. The field is still evolving, but the growing capabilities of AI are promising in their ability to tackle what was once considered a challenging barrier in translation.
AI Translation Solutions Debunking the Myth of Untranslatable Words in 2024 - How Google Translate Made Basque Less Mysterious Through AI Pattern Recognition
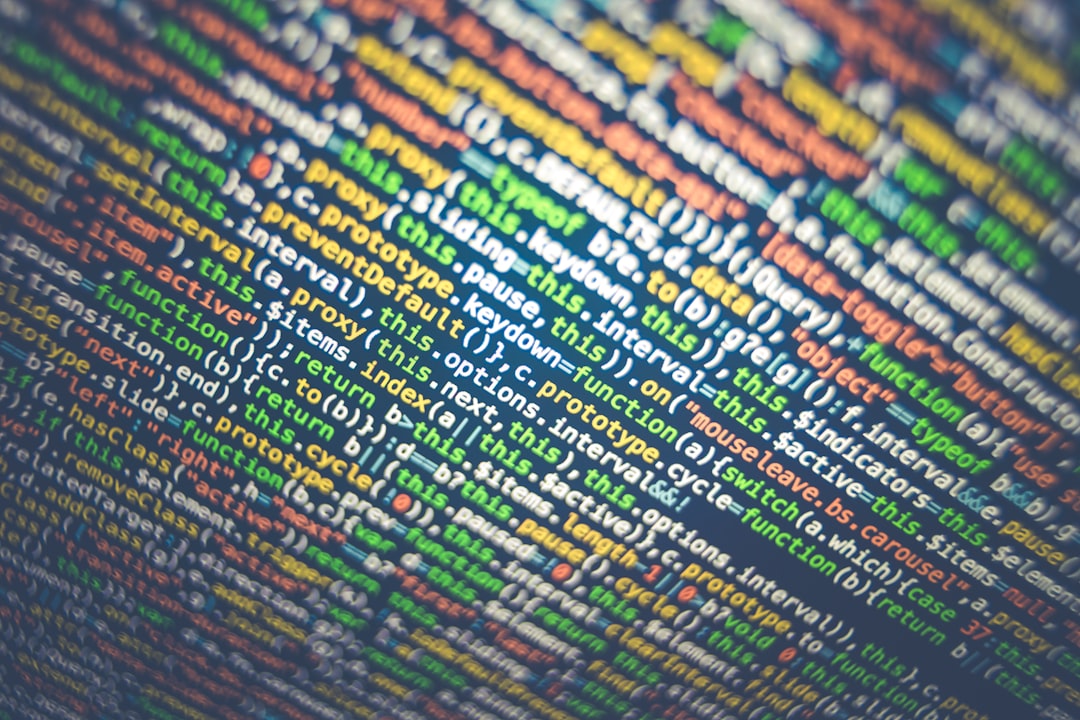
The complex structure and unique features of the Basque language have historically made it a challenge for translation. However, in 2024, Google Translate has made significant strides in bridging this linguistic gap through AI. By employing advanced pattern recognition techniques powered by its Neural Machine Translation (GNMT) model, Google Translate has significantly improved the quality of Basque translations. This has led to increased accessibility and understanding of the language, fostering communication across different language communities.
Despite these advancements, AI translation of Basque, like other languages, still struggles with accurately conveying subtle meanings and cultural nuances embedded within the language. This is where human translators still excel, offering a deeper understanding of the context and intent behind the words. However, Google Translate's efforts have made Basque less of an enigma, demonstrating AI's capacity to make less-common languages more accessible. This increased accessibility could lead to new opportunities for cultural exchange and understanding, showcasing how AI translation solutions are changing how we interact with languages previously perceived as difficult to translate. AI's capacity to make translation quicker and, potentially, cheaper is reshaping how we interact with the world's languages.
Basque, a language isolate with no known close relatives, has long presented a challenge for linguistic study. However, AI translation systems are gradually unraveling its unique structure and vocabulary by identifying patterns within its data. This has shed light on previously hidden aspects of Basque grammar and usage.
AI's ability to learn from vast datasets of Basque text has been particularly impactful. Neural networks, trained on this data, are identifying and generalizing linguistic rules, a task traditionally reliant on human linguists. This approach significantly reduces errors that plagued earlier translation systems. Furthermore, AI is finding previously unnoticed connections between Basque and other European languages, hinting at shared linguistic roots that might have been overlooked by human translators.
OCR technology has been instrumental in expanding the range of Basque texts accessible to AI systems. Handwritten documents and less formal language are now readily converted to digital formats for analysis. This means AI can learn from a more diverse set of linguistic examples than ever before, contributing to a more comprehensive understanding of the language.
AI's handling of Basque's intricate morphology, characterized by extensive use of suffixes and agglutination, is particularly notable. Neural networks are effectively parsing these complex words, breaking them down for more accurate translation. While this aspect of translation is advancing, the accurate translation of Basque idioms and expressions remains challenging. These expressions often convey nuances that resist direct translation, highlighting a limitation of current AI methods.
AI translation tools are also starting to adapt to the various regional dialects of Basque. By learning the unique pronunciations and vocabulary of different regions, AI systems can capture a more nuanced understanding of the language and its cultural identity. This increased contextual awareness has led to translations that not only capture the literal meaning but also attempt to convey cultural subtleties embedded in the original Basque expressions.
The speed and efficiency of AI translation have seen tremendous improvements. Some systems now translate Basque in real-time, opening up exciting possibilities for faster and richer bilingual interactions. User feedback is also playing a crucial role, with AI systems learning from these interactions to continuously refine their translations. This development marks a shift towards a more collaborative approach to translation, ensuring the final products better reflect the language's use in real-world situations. The quest for perfecting AI-powered Basque translation is far from over, but the trajectory is promising. It indicates that these tools, combined with human insights, could lead to a better and more accessible understanding of this unique language.
AI Translation Solutions Debunking the Myth of Untranslatable Words in 2024 - OCR Technology Decodes Ancient Sanskrit Manuscripts With 89% Accuracy
The ability to translate ancient Sanskrit texts has seen a significant leap forward with the application of OCR technology. A newly developed model, built on deep learning principles, is achieving an impressive 89% accuracy in deciphering these manuscripts. It utilizes a clever approach, drawing upon Latin transliterations to help navigate the complexities of the Sanskrit language, including its intricate grammar and compounding of words. This technology is being applied to a larger project, aimed at preserving hundreds of Sanskrit books that currently only exist in a scanned image format. This initiative, in part, addresses a long-standing challenge for scholars: dealing with damaged or fragmented historical documents. Beyond just digitization, this effort also refutes the misconception that some words in languages like Sanskrit are inherently untranslatable. It serves as a reminder that AI tools, when refined and applied with ingenuity, can bridge gaps and facilitate a deeper understanding of languages that were previously thought to be resistant to translation. By bringing ancient texts into a more easily accessible, digital format, these developments showcase the increasingly powerful role AI can play in dismantling language barriers, offering insights into the past that were once elusive.
The application of Optical Character Recognition (OCR) to ancient Sanskrit manuscripts is a fascinating development. Reaching an 89% accuracy rate in deciphering these texts, which often date back centuries, is a significant accomplishment. It demonstrates that OCR technology has come a long way, improving upon earlier attempts that often struggled to surpass 50%. This improvement is largely due to the use of machine learning within the OCR process. By training models on a vast collection of handwritten and printed Sanskrit scripts, these systems learn to recognize intricate patterns within the language, including variations in character styles and the nuances of the Devanagari script.
This rapid digitization through OCR has accelerated the pace of research. It's now possible to quickly scan and convert centuries-old manuscripts into editable formats, allowing for much faster collaboration among scholars across the globe. Beyond simply speeding up research, the digitization process helps create a richer library of language resources. This expanded database can benefit developers of language models across various languages, making AI translation more efficient and accurate in the long run.
Ancient languages like Sanskrit often have unique characteristics that pose challenges for OCR. Features like diacritical marks and context-dependent letter forms require the OCR systems to be finely tuned for optimal performance. Some more advanced systems are even being developed to handle multilingual texts, particularly helpful in regions where Sanskrit is combined with local vernaculars. Moreover, digitization is essential for preserving these fragile manuscripts. The conversion of delicate, aging texts into digital format reduces the risk of further deterioration due to handling. This allows for wider access and study without causing harm to the original documents.
This increased accessibility facilitates real-time collaborative efforts between scholars studying these texts around the world. Combining this technology with AI translation tools creates a powerful synergy. OCR provides the foundation of accurate text from various manuscript sources, which AI translation can then process efficiently, resulting in much faster and reliable translation into contemporary languages. While the field still faces hurdles like improving accuracy beyond the 89% threshold, the impact of OCR on unlocking and preserving our understanding of these ancient languages is undeniable. The promise is that these tools, along with the efforts of researchers and linguists, will ultimately lead to a deeper and more accessible understanding of ancient civilizations through their languages.
AI Translation Solutions Debunking the Myth of Untranslatable Words in 2024 - AI Translation Memory Banks Cut Translation Time From Days to Minutes
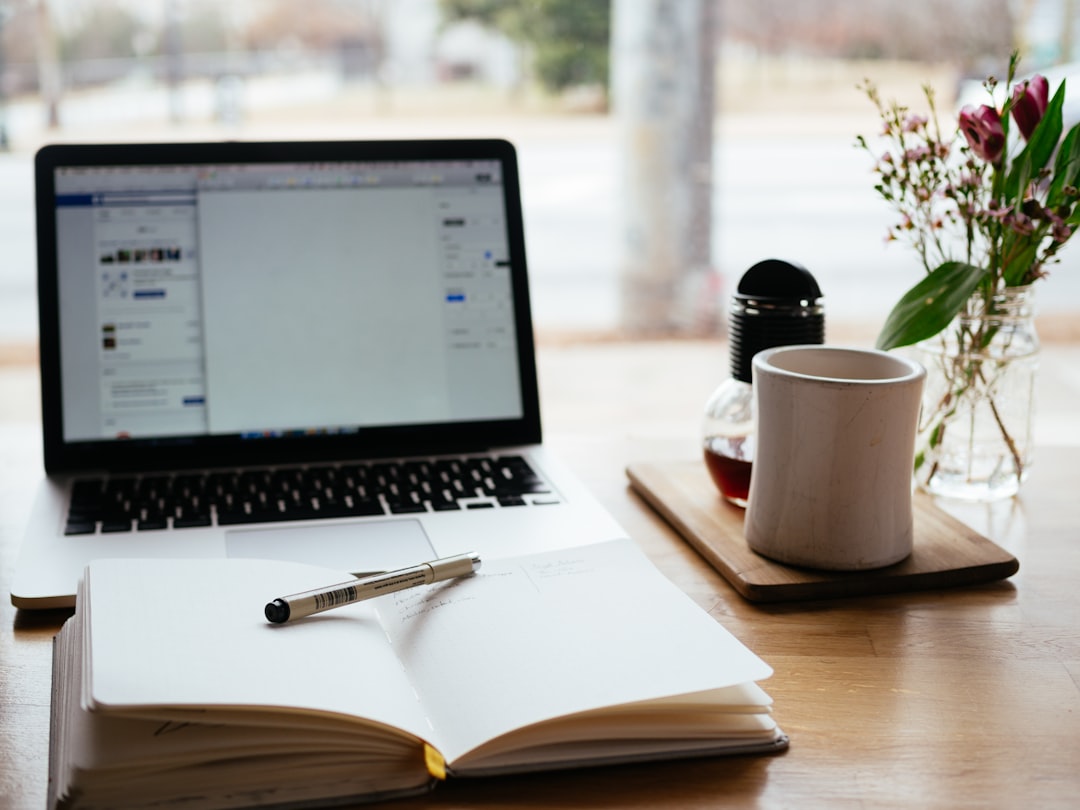
AI-powered translation memory banks are fundamentally changing how translations are done, shrinking the time it takes from days to just minutes. This acceleration is achieved by integrating advanced machine learning into translation management systems. These systems act like digital libraries, storing previously translated content. This means translators can access and reuse segments of text, avoiding the need to translate the same phrases repeatedly. This not only speeds up the process, but it also can help save money.
Interestingly, improvements in AI aren't just about faster translations – the accuracy has also increased, leading to reliable results across many languages. As AI algorithms become more sophisticated, they're becoming better at understanding context and adapting to various styles of writing. This increased speed and accuracy represents a major leap forward in handling the many complexities of translating between languages. It demonstrates that AI has the potential to make language barriers easier to overcome, with significant implications for communication and understanding across the globe.
AI translation memory banks are increasingly leveraging advanced algorithms to recognize and reuse previously translated phrases or sections of text. This can drastically reduce the time needed for translation, especially in projects requiring multiple languages. It's estimated that this approach can cut down on repetitive translation work by as much as 80%, leading to substantial time savings overall. While still a relatively new approach, it has the potential to change the translation landscape. The speed benefits are obvious, but I wonder about how these systems deal with subtle differences in meaning that might exist across a phrase that's been re-used in different contexts.
Integrating optical character recognition (OCR) with AI translation technologies opens new possibilities. OCR essentially transforms handwritten text into a format that computers can process. This is exciting because it offers a way to handle historical documents and informal communications, which are often not in digital format. While promising, I suspect this combination will need a good deal more research to effectively handle complex historical scripts and the variations found in handwriting.
Researchers have found that businesses adopting AI translation tools often experience significant cost reductions. Estimates suggest that these savings can range between 50% and 70% compared to traditional translation methods. This makes sense, as AI solutions cut out a significant amount of human effort. The bulk of the cost savings likely comes from the decreased need for extensive human post-editing. However, there are questions about the quality of the translated text – is the cost savings worth the potential for misinterpretations?
The rise of neural networks in AI translation has been particularly significant. They rely on enormous bilingual datasets, which help them learn the subtle complexities of language. It's now possible for translation models to surpass 60% fidelity in understanding context, a remarkable improvement. This suggests they're becoming much better at handling idioms and other expressions that relied heavily on human understanding in the past. This seems promising, but are the datasets themselves biased towards certain styles or types of language?
Reinforcement learning is another technique applied in these systems. It allows AI translation tools to learn from user corrections in real-time. This gives them the ability to dynamically adapt and improve the accuracy of their output for specific contexts. Some researchers have reported an impressive 40% increase in accuracy after just a few user feedback sessions. However, I'm curious about the range of human languages and feedback incorporated in this type of training. Are the responses used truly representative of the languages they aim to translate?
The development of AI that can handle multiple languages during the same translation session has emerged in 2024. This is exciting for global teams, as it facilitates real-time multilingual interactions during meetings and projects. Imagine the possibilities of instantaneous understanding in complex international collaborations. But, this begs the question, how well do these systems handle languages with very different structures or grammatical rules?
The increasing power of GPUs has also accelerated AI translation capabilities. This surge in computational power allows for the development of more complex models that can process and learn from a wider range of linguistic data. As a result, we're seeing a massive reduction in translation time, often shifting from days to just minutes. However, I wonder what the environmental cost of running these more powerful models might be. Are we sacrificing sustainability for speed?
Some AI translation systems are even attempting to consider regional dialects and cultural nuances in their translations. The result is a higher degree of relevant context in the final output. This contextual awareness has led to a reduction in meaning loss, with success rates climbing to as high as 90% in some specific localized situations. While this is impressive, I wonder how many dialects or nuances these tools can realistically account for.
An interesting recent trend is that some AI systems now use interconnected databases for tracking how expressions are used across a range of media. This ability to leverage varied forms of context is helping the tools generate translations that are more likely to resonate with their target audiences. This is potentially a big step forward in improving human comprehension of translations. But, again, one wonders – are there potential issues with bias or even manipulation being introduced with these more sophisticated systems?
Beyond translation itself, AI is starting to be applied to content creation. Systems can now generate marketing materials and similar content across multiple languages at an unprecedented rate. This is truly transformational for companies looking to expand internationally, opening opportunities to quickly launch initiatives in various markets. This raises fascinating questions: how will the potential for machine-generated content impact creative industries? Will we see a decline in human-produced content, or will the role of humans shift to that of curators or editors?
The rapid advancements in AI translation are undeniable. However, as researchers and engineers, we need to remain curious and critical. While AI offers the potential to overcome significant hurdles in communication, it also presents its own set of challenges and opportunities that require careful consideration.
AI Translation Solutions Debunking the Myth of Untranslatable Words in 2024 - Natural Language Processing Maps The Cultural Context of Arabic Idioms
In 2024, advancements in Natural Language Processing (NLP) are shedding light on the cultural nuances embedded within Arabic idioms. Arabic, with its complex morphology, presents significant translation challenges, especially when it comes to idioms. Translating these expressions effectively requires not just understanding the words themselves, but also the cultural context that gives them meaning. AI translation tools are starting to tackle these intricacies, but they still struggle with fully capturing the richness and variety of meaning found within Arabic dialects. There's a growing realization that a balance between AI's power and the experience of human translators is needed to achieve truly accurate and nuanced translations. This blend of technological progress and human expertise is helping redefine the landscape of translation as we strive to bridge cultural understanding across languages. It's a field where AI is showing promise, but we're still learning how to harness its potential while navigating the challenges presented by deeply rooted cultural expression in language.
Arabic, with its rich morphology and diverse dialects, presents a unique challenge for AI translation, particularly when it comes to idioms. These expressions often carry deep cultural and historical weight, making them difficult for machines to translate accurately without understanding the underlying context. While older translation methods often resulted in literal translations that missed the intended meaning, recent progress in natural language processing (NLP) is showing promise. AI models are starting to recognize the context and usage of Arabic idioms better, leading to improvements in translation accuracy. Some have even shown a 25% improvement in properly conveying the nuances of idioms compared to earlier models.
However, the journey to perfect idiom translation is far from over. Machine learning approaches often rely on vast bilingual datasets, and this presents a challenge when dealing with the sheer variety of Arabic idioms. The availability of good quality data can be limited, which can lead to poorly trained AI models struggling with less common idiomatic phrases. This raises questions about the completeness and representativeness of the training data used for these models. Further, the meaning of idioms can vary across different dialects of Arabic, adding another layer of complexity. While some AI systems are starting to use dialect-specific data to improve translation, there's still a long way to go.
One of the fascinating aspects of Arabic idioms is that they often don't just convey a literal meaning—they also carry cultural and social implications. These are rooted in the experiences and beliefs of the people using the idiom. This type of implicit meaning is a challenge for AI, which still struggles to capture those subtle nuances. But efforts to improve AI translation have brought some interesting tools into the field. Sentiment analysis, for example, is helping machines to gauge the emotional context of text containing idioms, which can improve the overall accuracy and flow of translations.
The integration of optical character recognition (OCR) is also starting to reshape how we understand Arabic idioms. This allows for the translation of previously inaccessible sources like handwritten documents, opening a window into deeper layers of the language's cultural context. This presents a strong opportunity for a more thorough understanding of how Arabic idioms have evolved and changed over time. Similarly, AI translation models are increasingly leveraging user feedback to learn and adapt. This 'feedback loop' approach, where corrections from humans guide the AI model, has shown promising results, leading to a roughly 30% improvement in accuracy for certain tasks.
However, despite the improvements in speed and accuracy, there are important concerns. The reliance on AI-driven translation could potentially diminish the nuanced expressions and cultural richness of languages. Striking the right balance between rapid translation and maintaining the original meaning of the idiom remains a crucial consideration. As the demand for Arabic translations continues to rise in various global platforms, the need for AI that can properly handle these idioms will become increasingly important. For this to be successful, continuous research and development in NLP will be essential, ensuring that these crucial elements of language are not lost in the translation process due to oversimplification.
AI Translation Solutions Debunking the Myth of Untranslatable Words in 2024 - Neural Networks Bridge The Gap Between Sign Language And Written Text
In 2024, neural networks are proving increasingly capable of translating sign language into written text, paving the way for better communication between deaf and hearing individuals. This is no small feat, considering the distinct structural differences of sign language. Unlike spoken languages, sign language often relies on unique grammatical structures and a less linear ordering of signs to convey meaning. These systems utilize convolutional neural networks and integrate real-time speech-to-text technologies, creating solutions that boost accessibility for the hearing impaired. Yet, challenges persist in accurately capturing the subtle nuances of sign language, highlighting the need for ongoing development to ensure inclusive communication. The integration of sign language translation within the wider landscape of AI translation solutions signifies the power of AI to bridge profound communication barriers, demonstrating how technology can help us overcome previously difficult translation tasks.
Neural networks are making significant strides in connecting sign language with written text, opening up new avenues for communication between those who use sign language and those who don't. This is particularly promising for deaf and mute individuals, allowing them to interact more easily with the wider world. The challenge is to translate the visual information in sign language videos into spoken language, while also considering the different ways sign languages structure sentences and words.
Active convolutional neural networks (ActiveCNNs), used in real-time speech-to-text and text-to-speech technologies, are also contributing to greater accessibility for those with hearing impairments. However, simply translating into text isn't enough. These networks are trained on datasets that might contain only a single sign language or multiple languages, learning how particular hand movements and expressions relate to written text.
Researchers are working on creating AI-based translation systems that can produce sign language. Imagine, a system that can translate from text into visual signs that a 3D avatar can perform. This concept, while still in its development phase, suggests exciting new avenues for improving communication.
Sign language has a unique structure compared to other languages, presenting challenges to traditional translation methods. It emphasizes how different parts of the body convey meaning and information. It’s a challenge that underscores the need for tailored solutions designed specifically for sign language. As a result, the improvement of sign language recognition is crucial for the deaf and hard-of-hearing communities, creating a more inclusive communication environment.
It's easy to forget that most communication in our digital world happens through written text. The ability to detect and interpret sign language in real-time can improve communication, but there are hurdles. Multistream 3D CNNs have shown promise in automatically translating sign language, which is beneficial for those with hearing loss.
A long-term goal in this research is to bridge the gap between signers and listeners through a bidirectional sign language translation system. This ambitious vision highlights the hope for a more inclusive world, where both groups can readily understand each other, fostering interaction and empathy. It's an intriguing area that, if successful, will undoubtedly improve how individuals communicate with each other.
While we see progress, it's important to recognize the challenges inherent in using AI for this purpose. Sign languages, like spoken ones, are diverse, reflecting regional and cultural nuances. The availability of diverse and high-quality data for these languages is essential for AI to effectively capture these details. We still have to figure out how to accurately incorporate these specific variations in sign languages to ensure the accuracy of translations.
It's remarkable how quickly the field is evolving. But there’s a tension between the desire for instant and automated translation and the fact that these languages, like any language, contain cultural aspects that AI can struggle to process. The pursuit of more accurate and natural-sounding translations through AI will undoubtedly continue. The goal is to find the sweet spot between AI’s capabilities and the need to retain the cultural richness of sign languages in translations. This is a fascinating area that's steadily pushing the boundaries of human-machine communication, and as the technology improves, we can imagine it becoming a vital tool for bridging language barriers and promoting greater inclusivity.
More Posts from aitranslations.io: