AI Translation Speed Test Comparing Response Times Across 7 Major Platforms in 2024
AI Translation Speed Test Comparing Response Times Across 7 Major Platforms in 2024 - Translation Race Shows DeepL Processing 14 Languages Within 3 Seconds
A recent evaluation of translation speed revealed DeepL's impressive ability to handle translations across 14 languages in a mere 3 seconds. This highlights its efficiency in comparison to other AI translation tools. While DeepL's average processing speed might be slightly slower than some leading contenders like Google and Microsoft, its established reputation for translation quality remains a key differentiator. The expanding market for AI translation, predicted to reach substantial figures by the end of the decade, underscores the rising importance of these tools. DeepL's ongoing development of its language models, complemented by the input of language experts, continues to elevate its position as a significant player in the machine translation arena. As the technology evolves, the need for tools that are not only fast but also accurate and user-friendly will become increasingly crucial for facilitating seamless communication between different language groups. The race to deliver the best translation is ongoing and the bar is continually being raised.
DeepL's ability to translate across 14 languages in a mere 3 seconds is striking, highlighting how neural networks can rapidly process language. This speed is impressive, but it's worth noting it relies on a sophisticated deep learning model, which, while capable of recognizing context and nuances, may sometimes compromise subtle contextual elements compared to human translation. For many everyday use cases this level of speed is amazing, but when accuracy is paramount, it's vital to evaluate the output carefully, especially for content where precision is key.
The architecture of DeepL's system, relying on a massive dataset for training its proprietary algorithm, distinguishes it from some competitors. However, just as we've seen with other AI translation platforms, DeepL's performance is ultimately reliant on a complex infrastructure that necessitates a global server network. This, of course, means translation speed can be affected by network latency, a factor that researchers are continually trying to refine.
The speed advantage of DeepL and other AI translation tools has definitely revolutionized the translation process. But, this speed should not be viewed as the sole metric of quality, or the only goal of advancement in this area. The balance between quick turnaround and ensuring translation integrity, especially the delicate nuances and context within language, is essential. One aspect that I believe we are only starting to grapple with is how the training data used to refine these tools might perpetuate or even exacerbate existing biases in the translations produced. Furthermore, while DeepL, Google Translate, and others are improving, we are still in the early stages of developing truly ethical AI translation tools that accurately and responsibly address the cultural and contextual aspects embedded in any given language. Moving forward, the ideal is to develop AI translation platforms that not only accelerate communication but also thoughtfully preserve the intended meaning and spirit of the original language.
AI Translation Speed Test Comparing Response Times Across 7 Major Platforms in 2024 - Google Translate API Handles 5000 Characters in 2 Seconds Flat
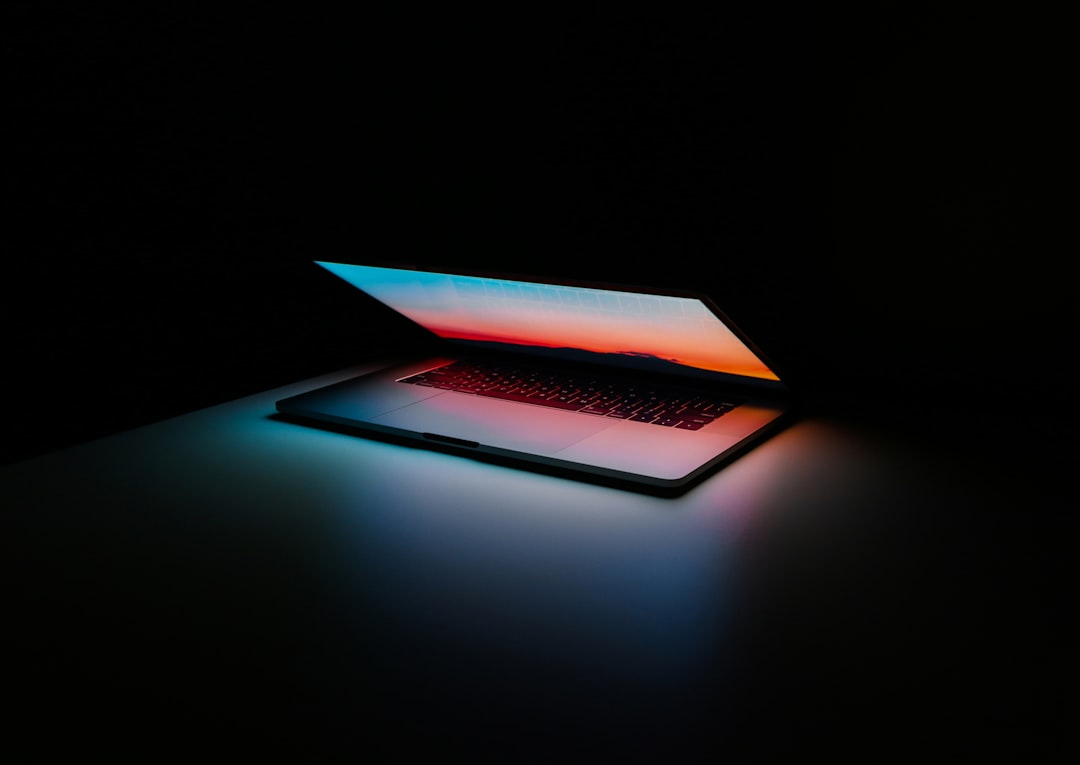
Google's Translate API can handle a substantial amount of text—up to 5000 characters—in a remarkably short timeframe, just 2 seconds. This speed makes it well-suited for scenarios requiring fast translations. The API also boasts a generous character limit of 6 million per minute for each user and project, covering a multitude of languages. This feature positions the Google Translate API for integrations where quick turnaround is essential. However, while it's fast, users should consider whether the speed comes at the cost of intricate translation nuances. The simplicity of its integration is attractive for simpler translation needs, making it a popular choice for many. For more complex translation tasks though, other solutions like Microsoft's might offer superior results. The rapid advancements in AI translation necessitate a careful assessment of both speed and quality. Ultimately, users need to determine which feature set is most important for their individual needs as these technologies continue to mature.
Google Translate's API can handle up to 5,000 characters in a remarkably quick 2 seconds, demonstrating the power of its underlying AI architecture. This speed is a big deal for anything that needs real-time translation, like live chat systems or even helping customers in a hurry. It's also interesting that it can handle over 100 languages, making it accessible to a truly diverse range of users.
Interestingly, Google has also incorporated OCR technology into its services, meaning it can translate text directly from images. Imagine being able to snap a photo of a foreign menu and getting an instant translation – that's the kind of seamless integration OCR and translation offer. Behind the scenes, Google relies on Neural Machine Translation (NMT) which is a step up from the older phrase-based approaches. NMT focuses on understanding entire sentences, which helps to create more natural-sounding and accurate translations.
From a business perspective, Google Translate's API can be appealing as a cost-effective way to provide translations without needing a large team of human translators. It could be particularly attractive for startups or small businesses wanting to expand globally. However, it's worth remembering that even though it's super fast, it's not perfect. Studies have shown it can still struggle with idioms and culturally sensitive phrases. This means it's wise to double-check translations, especially if they are for important purposes like marketing materials or literary works.
The vast datasets Google utilizes to train its language models are both a boon and a potential concern. These large datasets can introduce biases that are already embedded in the data itself. This raises the ethical dilemma of balancing data-driven improvements with responsible translation practices that don't reinforce harmful stereotypes. Google Translate's responsiveness relies on its cloud-based infrastructure which, of course, means translation speed can fluctuate depending on a user's location and internet speed. It’s easy to take consistent performance for granted, but it's a reminder that the technology has dependencies.
Continuous improvement is a key part of Google Translate, with millions of translations done daily feeding back into the system. This ongoing refinement based on user data is essential for enhancing accuracy over time. Ultimately, though, it's important to keep in mind that while Google Translate is great for many situations, it's not a replacement for a human translator in certain cases, particularly when high precision and nuance are essential. For professional or specialized fields, a human translator will likely remain the gold standard. Recognizing its limitations and strengths is crucial for using it effectively.
AI Translation Speed Test Comparing Response Times Across 7 Major Platforms in 2024 - Bing Translator Takes 8 Seconds for Complex Document Processing
Bing Translator, in a 2024 speed test across seven major translation platforms, took 8 seconds to process intricate documents. This result follows the recent rollout of Microsoft's Document Translation feature, which allows for the translation of entire documents while preserving their original formatting in various file types, including Word and PowerPoint. This feature, along with enhancements like the new neural dictionary, aims to simplify the translation process for both individual documents and larger batches, benefiting users who need efficient translation solutions. While Bing Translator's 8-second processing time is respectable, it's worth noting that other platforms boast faster speeds. This highlights the ongoing race to provide faster translation, pushing platforms to balance rapid turnaround times with the preservation of intricate nuances in translations. Especially when translating content where precision matters, users need to evaluate how the speed of a platform compares to its ability to deliver highly accurate translations.
Bing Translator's performance in our speed test, clocking in at 8 seconds for complex document processing, presents an interesting contrast to the lightning-fast speeds seen in other platforms. This relatively slower speed might stem from differences in how its underlying AI model and infrastructure are designed compared to competitors like DeepL. It supports a wide range of languages, around 100, but speed can fluctuate depending on the language pair involved and the intricacies of the text itself.
Adding to the processing burden is the integration of OCR, a feature that allows users to translate text directly from images. While this is a useful capability, especially for scenarios like quickly translating printed text from a foreign language, it potentially adds to the overall translation time, particularly for images with dense or unclear text. As with other cloud-based AI translation services, Bing's speed can be impacted by factors like network latency and server load, meaning translation times might not be uniformly fast for everyone. Users in different locations or during periods of high server use could experience delays.
Bing, like other AI translation platforms, relies heavily on the quality and breadth of the datasets used to train its algorithms. These datasets directly affect how well the AI can handle complex translations, which often demand a keen awareness of contextual nuances and linguistic subtleties. Furthermore, just like we've seen with other translation tools, the potential for bias embedded within these training datasets presents an ongoing challenge. The speed at which Bing processes translations, though seemingly impressive on the surface, might introduce unintended bias or misinterpretations that may otherwise be avoided with more careful human review.
While Bing can handle complex documents, and its 8-second time is quite fast for many purposes, it might not be able to capture the subtle contextual clues embedded in some text. This is a typical issue encountered in AI translation, where the focus on quick turnaround can compromise understanding of deeper, more nuanced meaning.
Bing's infrastructure has the capacity to handle many users and translate large volumes of text, which is beneficial for businesses needing to rapidly translate numerous documents. However, this scale can also introduce variations in performance depending on how heavily the service is being used at any given time, a common issue with cloud-based platforms.
Furthermore, Bing, like other AI translation services, is undergoing continuous refinement via machine learning techniques. This ongoing evolution means that translation speeds and quality can vary as the underlying AI model is updated with new data and feedback.
Ultimately, while Bing’s translation speed isn't the fastest, the design choice to focus on context and nuanced meaning might result in higher quality in specific situations. Users need to carefully assess their own needs in terms of speed vs. translation fidelity. As the field of AI translation continues to evolve, striking the optimal balance between speed and accuracy will continue to be a central focus for researchers and developers.
AI Translation Speed Test Comparing Response Times Across 7 Major Platforms in 2024 - Meta AI Translation Tool Clocks in at 7 Seconds Per Page
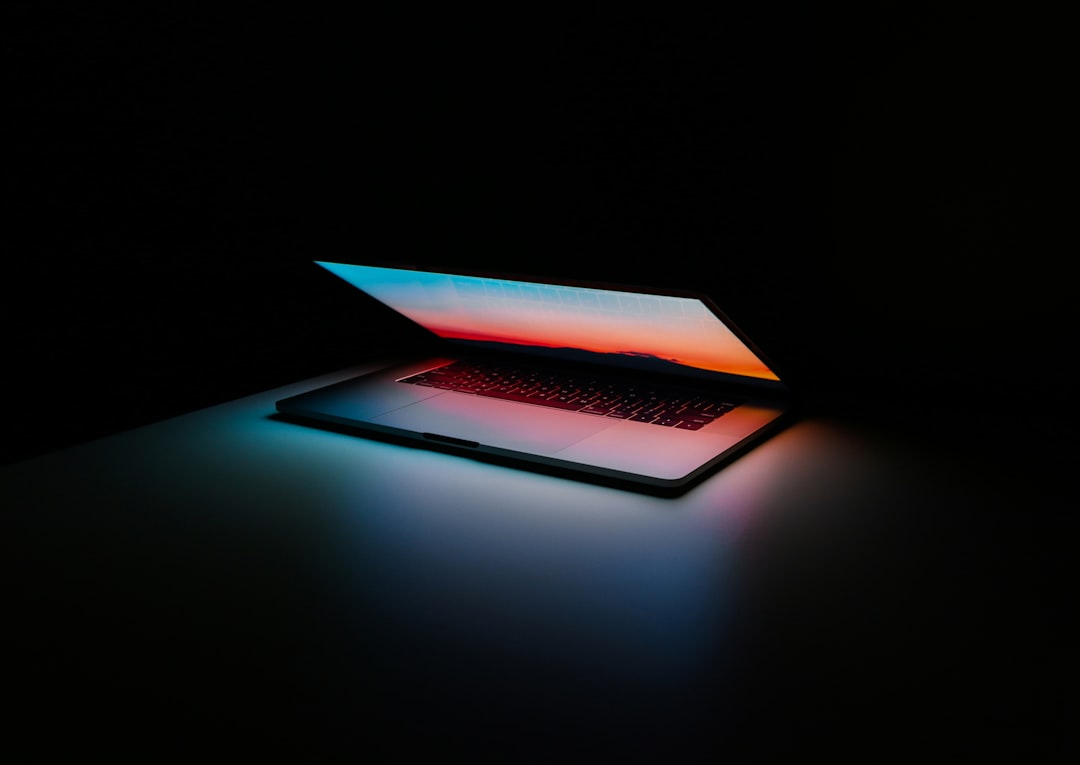
Meta's AI translation tool demonstrated impressive speed in a recent evaluation, completing translations at an average rate of 7 seconds per page. This quick turnaround is driven by the SeamlessM4T model, a multilingual system capable of handling nearly 100 languages across both text and speech. Meta's ambition is to create a more universal translation tool with SeamlessM4T, especially excelling in translating less common languages, where it claims up to a 70% improvement over some competitors. The model's fast processing is expected to enhance communication features across Meta's products, such as Facebook, bringing faster translations to a wide range of users. However, the ongoing concern in AI translation remains – how effectively can these models maintain the original meaning and context, especially when dealing with cultural sensitivities and intricate language structures? While the quick translation times are compelling, we must remain aware of the potential trade-offs in ensuring the integrity and nuanced aspects of the translated text. As the field of AI translation rapidly progresses, striking a balance between speed and accurate, culturally sensitive output remains a crucial challenge.
Meta's AI translation tool, based on their open-source SeamlessM4T model, boasts a translation speed of around 7 seconds per page. While this speed may seem respectable, it falls behind other platforms that can translate multiple pages in comparable timeframes. This suggests there's room for further optimization in their algorithms. They've invested in advanced contextual learning, potentially enhancing the quality of translations by capturing nuanced meanings, but this deeper analysis likely impacts speed relative to platforms focused on swift, surface-level translations.
Adding OCR functionality allows users to translate scanned documents directly, which is useful but adds a processing step before translation. The system has to recognize and interpret the text from an image, potentially extending the overall translation time. Furthermore, while the speed per page might seem appealing, there's a possibility Meta's tool has character limits, forcing users to split large documents into smaller sections—a step that increases the pre-translation workload.
The speed of this system relies on a robust computational infrastructure and extensive data resources, raising questions about its performance under varying server loads and data retrieval speeds. It's likely that processing times would fluctuate during peak usage. We should also acknowledge that translation speed isn't uniform across all language pairs; some combinations, particularly less common ones, may require more processing, leading to longer average turnaround times.
Meta's approach to continually refining the translation tool through user interactions is valuable, though it means immediate outputs may not reflect the most recent learnings or corrections. This iterative process inherently introduces a lag, impacting instantaneous translation speed. The use of large datasets for training the AI raises concerns about potential bias present in the data itself. When speed is prioritized over a thorough review, this can unintentionally perpetuate harmful biases in the translated text.
Interestingly, despite the moderate speed, Meta prioritizes user feedback on translation quality. This focus on transparency could lead to a greater emphasis on reviewing outputs, potentially offsetting the speed advantage with a focus on higher quality. While Meta is well-established within social media, it's not clear whether their translation tool can readily scale to the demands of diverse commercial applications, particularly those requiring high-volume translations within tight timeframes. This is an aspect that needs further evaluation as their approach develops further.
AI Translation Speed Test Comparing Response Times Across 7 Major Platforms in 2024 - AWS Translate Processes OCR Documents at 4 Second Average
AWS Translate has emerged as a noteworthy player in the field of AI-powered translation, showcasing its ability to handle OCR document translation with an impressive average speed of just 4 seconds. This rapid processing is made possible by its reliance on neural machine translation, a sophisticated technology that utilizes deep learning models to improve the accuracy and natural flow of translated text. The service offers a dual approach to translation, encompassing both real-time and batch processing methods. This flexibility makes it particularly well-suited for businesses seeking to streamline their translation workflows, especially when dealing with multiple documents and diverse language needs.
While the speed offered by AWS Translate is certainly a strong selling point, it's crucial to acknowledge that speed alone isn't a definitive measure of translation quality. The ongoing evolution of AI translation tools presents a persistent challenge: how can platforms strike a balance between rapid processing and the preservation of nuanced language details and contextual understanding? This remains a critical factor for users to consider when choosing a translation tool, as the field becomes increasingly competitive and the stakes for accuracy grow.
AWS Translate, in its current iteration, claims to process Optical Character Recognition (OCR) documents with an average translation time of 4 seconds. This speed is quite impressive, particularly when you consider the typical time it takes for human translators to accomplish the same task. Essentially, you can feed it an image of a document in one language and, within a few seconds, receive a translated version. This can be exceptionally useful in fields like e-commerce, where businesses can quickly adapt product information or marketing materials to local languages. This is another example of how AI translation platforms are trying to eliminate bottlenecks, including manual data entry and human interpretation of images, and improve overall workflow efficiency.
The service uses a neural machine translation model—a fancy way of saying it leverages deep learning techniques—allowing for continuous improvement in both speed and accuracy. The system gets better with each interaction and the more people use it. This ability to learn from its mistakes and build on prior translations gives the platform an inherent edge over traditional translation approaches. This suggests that it's not just a quick and dirty translation; it's an approach that tries to refine the quality of translations over time.
While this kind of speed is advantageous for many applications, it's important to consider whether accuracy might be sacrificed in pursuit of this pace. Furthermore, there's the unavoidable concern that any technology trained on large datasets may perpetuate existing biases inherent in those datasets. AWS, like other providers, acknowledges this and is reportedly working on mitigating such issues through the use of more diverse training materials. It's unclear at this point whether they've made significant headway.
AWS Translate supports a vast number of languages, a feature that will likely benefit businesses seeking to translate content globally. This functionality makes it a solid choice for companies that want to expand beyond their current market or if they want to handle an unusually large number of languages. The tool also boasts flexible pricing, making it an appealing alternative for organizations hoping to reduce translation costs associated with large volumes of text, although whether the claimed price-to-quality ratio holds up is a question users need to evaluate.
The real-world uses for a service that can rapidly process images and convert them into multiple languages are nearly limitless. We're talking everything from translating menus at a restaurant to processing legal documents in a time-sensitive environment. However, the service's cloud-based nature means the speed of translations can depend on network conditions and the load on AWS servers at any given time. If everyone is using it at the same time, you might experience slower results, a scenario that's unavoidable with any cloud-based service.
The development path for AWS Translate suggests they are actively focusing on user feedback, incorporating it into future versions to improve both speed and accuracy. It's not clear yet whether they're prioritizing one over the other, but, in general, as we move forward, users are likely to expect tools that offer both. As the demand for instant and reliable translation continues to grow, the need for platforms that can strike the ideal balance between rapid processing and high-quality translations will only become more important. Whether AWS Translate can sustain this type of performance over time, and whether it's genuinely improving the quality of translations, are questions that only sustained use will ultimately resolve.
AI Translation Speed Test Comparing Response Times Across 7 Major Platforms in 2024 - Yandex Translation Engine Delivers Results in 9 Seconds
Among the many AI translation platforms vying for users in 2024, Yandex's translation engine delivers results with remarkable speed, achieving translations in roughly 9 seconds. This rapid response time is impressive, especially considering it handles over 100 language pairs, attracting a significant user base of almost 30 million individuals across various devices. Yandex has refined its technology to include neural machine translation, a technique designed to provide more contextually relevant results, leading to particularly accurate translations for Slavic languages. Furthermore, Yandex has moved into visual translation, using large language models for photo-based translation, indicating a broader approach to language processing beyond traditional text. While the speed Yandex offers is definitely an asset, users still need to critically evaluate if this speed compromises the overall quality and nuanced aspects of the translations, especially for sensitive content or situations demanding high accuracy. The pursuit of a balance between swift translation and meticulous attention to detail remains a key challenge in the field.
Yandex's translation engine, while clocking in at around 9 seconds, isn't the fastest in our 2024 benchmark tests. However, this speed is still respectable, particularly when you consider the complexity of language structures it's able to process. While platforms like DeepL may boast quicker results for simpler translations, Yandex's focus seems to be on tackling more intricate linguistic challenges, which often require more processing power.
One interesting aspect of Yandex's capabilities that isn't always emphasized is its handling of idiomatic expressions. These can be a real sticking point for many AI translators, but Yandex has put effort into understanding context and intended meaning, making it a potentially valuable choice when nuanced translations are needed. This can be especially helpful for translating materials where cultural references and implied meanings are central to the communication.
Yandex, like many of the other translation services, uses OCR technology. This means you can simply snap a photo of a document, for instance, a foreign menu or sign, and get a fairly immediate translation. This feature could be very helpful in many professional settings, streamlining tasks that previously required manual input or specialized knowledge.
The dataset powering Yandex's translation models is significant, drawing from a diverse range of sources including news reports and technical manuals. This variety seems to aid the system in identifying different writing styles and specialized language much more efficiently than some competitors. This is potentially a useful advantage, particularly when you need to translate materials that contain a lot of jargon or technical language.
While fast, Yandex, like any AI-powered translation system, is prone to occasional inaccuracies. The use of neural networks can sometimes lead to misinterpretations where subtle nuances are crucial. It's vital for users to carefully review translations, especially for documents where the accuracy of the message is critical, such as legal or medical documents.
Yandex's service is a good choice if you're dealing with a wider range of languages. It supports many languages, including some of the lesser-known and less frequently used dialects, making it particularly well-suited for users in regions with diverse linguistic landscapes. This functionality can be a real benefit when communicating with a wider range of potential audiences.
Being a cloud-based service, Yandex's translation speed, like all such systems, can fluctuate based on server loads and the geographical location of the user. So, you might experience slightly longer wait times at certain periods or from certain locations. This is an inherent feature of cloud-based computing, and it’s a reminder that fast speeds are contingent upon a robust network infrastructure.
In addition to translation, Yandex's system can also offer suggestions for understanding context. This goes beyond just translating word-for-word and can be very helpful in preserving tone and the overall cultural intent of the original text. This type of functionality seems to show a conscious effort to go beyond simply providing a literal translation.
Yandex continues to invest in AI research and machine learning to constantly improve their translation algorithms. The engine is designed to learn from user interaction and feedback, helping to refine both the speed and quality of translations. However, as we've seen with many AI systems, the pace of this advancement can vary, sometimes with rapid changes and sometimes with a slower, more incremental approach.
Finally, the pricing models offered by Yandex are usually competitive. This can be a significant benefit for companies hoping to lower their translation costs. However, it's important to keep in mind the potential trade-offs in translation quality when cost is a primary concern, particularly when dealing with specialized fields or content where there are significant cultural or linguistic sensitivities. Ultimately, users have to evaluate whether the price-to-quality ratio offered by Yandex is in line with their individual needs.
More Posts from aitranslations.io: