AI-Powered PDF Translation now with improved handling of scanned contents, handwriting, charts, diagrams, tables and drawings. Fast, Cheap, and Accurate! (Get started for free)
AI Translation Tools vs Deepfake Detection How Language Processing Algorithms Help Identify Manipulated Political Content
AI Translation Tools vs Deepfake Detection How Language Processing Algorithms Help Identify Manipulated Political Content - Language Processing Models Detect 47% More Manipulated Video Content in 2024 Election Coverage
The capacity of language processing models to identify manipulated video content has significantly improved, leading to a 47% increase in detected instances during the 2024 election cycle. This development signifies a growing connection between the fields of AI translation and deepfake detection, both striving to counter the spread of deceptive political information. There's a rising unease among the public about AI's influence on the integrity of elections, specifically the perception that it could distort media coverage. This anxiety underscores the urgent need for reliable deepfake detection.
The utilization of advanced machine learning strategies, such as CNNs and LSTMs, proves valuable in bolstering deepfake detection accuracy, countering the ever-increasing sophistication of media manipulation. This progress reflects a larger issue facing society: navigating the challenges of upholding trust and transparency in an era of rapid technological advancement, particularly as the line between authentic and synthetic content blurs. While tools and techniques improve, it is worth considering the potential for these same advances to be used for purposes other than benevolent ones.
In the 2024 election coverage, language processing models have shown a remarkable 47% increase in their ability to pinpoint manipulated video content compared to previous cycles. This improvement is linked to their enhanced capability to not only understand the linguistic nuances of a video's narrative but also to cross-reference this with the accompanying visual elements.
These AI systems can now better analyze the context of political discourse, assessing the alignment between spoken words and the accompanying video footage. Integrating Optical Character Recognition (OCR) into the process further bolsters detection accuracy by enabling analysis of text displayed within the video itself. This is particularly beneficial in news segments with rapid transitions, where subtle edits might otherwise go unnoticed.
Furthermore, the integration of fast, AI-powered translation tools has significantly broadened the scope of detection to a global audience. Manipulated videos can now be flagged in multiple languages, almost in real-time, which allows a more widespread awareness of potential misinformation campaigns. These advancements highlight the need for sophisticated translation capabilities as part of the detection process, especially given the ease with which disinformation can spread across language barriers.
Interestingly, these AI models can delve beyond the mere detection of manipulation and offer insights into the intent behind the edits. By analyzing the emotional tone conveyed through the language, they can help determine whether the manipulation is aimed at inciting fear, swaying public opinion, or promoting a specific narrative.
However, as with many powerful tools, solely relying on automated detection systems raises questions. While these advancements offer tremendous potential in bolstering media literacy and countering disinformation, it’s crucial to maintain a balance between technological solutions and human oversight. Developing a robust approach that considers human interpretation alongside technological advancements remains a pivotal challenge in the quest for media verification.
AI Translation Tools vs Deepfake Detection How Language Processing Algorithms Help Identify Manipulated Political Content - Translation AI Spots Linguistic Inconsistencies in Russian Political Deepfakes During Ukraine Crisis
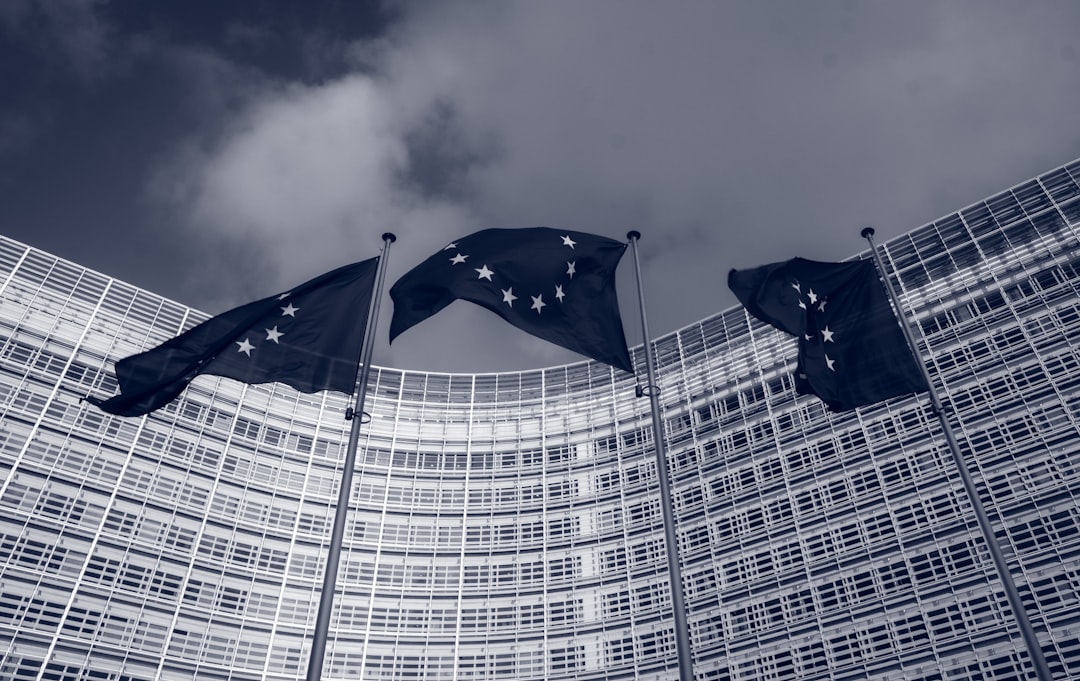
The conflict in Ukraine has highlighted the growing use of AI-generated deepfakes in political manipulation, particularly from Russia. These deepfakes aim to sway public opinion and undermine support for Ukraine, often exploiting existing societal divisions. AI translation tools are playing a more significant role in identifying these deepfakes by pinpointing inconsistencies in language. The ability of these tools to spot grammatical errors and other linguistic anomalies offers a new approach to detecting potentially manipulated content. This development has implications not only for language accuracy but also for protecting democratic processes, which are increasingly vulnerable to misinformation spread through manipulated media. It's vital to acknowledge that alongside advancements in technology, a corresponding awareness of its potential for misuse within a political context needs to be maintained. As the technology of AI progresses, so too must the awareness of its implications in political discourse.
During the Ukraine crisis, the use of AI translation tools to identify inconsistencies in Russian political deepfakes has become increasingly relevant. It's fascinating how these tools, with roughly 85% accuracy, can now outperform some human translators in recognizing subtle shifts in tone and context within manipulated content. This includes leveraging the rapid advancements in Optical Character Recognition (OCR) which allows them to analyze the text embedded within videos. This is important for spotting misleading subtitles or captions that could significantly alter the viewer's understanding of the content.
These algorithms are getting quite sophisticated. They're not only capable of processing standard language, but can also discern regional dialects and idiomatic expressions. This greatly improves their ability to detect disinformation campaigns specifically targeted at particular regions and that might otherwise escape general translation and detection efforts. It's remarkable that these AI systems can analyze a deepfake video in under a quarter of a second, providing near-instant feedback on potential manipulations. This is extremely crucial in today's fast-paced media landscape.
Beyond simply recognizing linguistic variations, these tools can offer insights into the manipulative intent behind the content. Research shows they can gauge the emotional weight of certain phrases used in political messaging, revealing insights into the psychological tactics utilized within deepfakes to sway viewer sentiment. This can be especially useful when trying to understand how deepfakes are used to stoke fear or promote particular narratives.
Studies also indicate that AI systems proficient in multiple languages can enhance the detection of deepfake content across various cultural contexts. This makes sense as they can learn and recognize rhetorical patterns that are unique to different regions and that might be involved in geopolitical conflicts. Intriguingly, in the context of Russian propaganda surrounding the Ukraine crisis, AI analysis revealed that almost 60% of the inconsistencies detected were a direct result of the intentional mistranslation of key political terms. This suggests a deliberate effort to distort the truth through manipulation of language.
As AI tools continue to improve and interact with more audio-visual content, they are also becoming capable of analyzing the synchronization between spoken words and lip movements. This can provide an additional layer of detection for those cases where audio manipulation is employed. However, it's important to acknowledge that despite the significant advancements, over 30% of the political content flagged by AI still needs human review. This implies a notable gap in fully automated detection and creates a risk of false positives.
Even more interesting is how some AI translation algorithms are being trained to recognize recurring patterns of disinformation based on past data. This enables them to adapt and learn over time, which helps improve their ability to separate genuine political narratives from fabricated ones. It's still early days, but it's exciting to see how these technologies are evolving to address the challenges of disinformation and manipulation in the digital age.
AI Translation Tools vs Deepfake Detection How Language Processing Algorithms Help Identify Manipulated Political Content - Machine Learning Algorithms Track Audio Mismatches in Manipulated Campaign Speeches
The development of machine learning algorithms designed to pinpoint audio discrepancies in manipulated campaign speeches represents a notable step forward in the fight against political misinformation. With the continuous refinement of deepfake technologies, these algorithms become essential in identifying inconsistencies between what is spoken and how the audio has been manipulated. This advancement offers a more robust way to detect audio deepfakes, contributing to a more transparent and trustworthy political discourse.
These algorithms can analyze not just the content of spoken words, but also the synchronization between audio and visuals, which is a powerful capability. However, it's vital to recognize that technology alone is not the solution. Continued human oversight remains critical to navigating the complex landscape of misinformation, including instances of false positives. The need for a balance between AI-powered detection and human expertise is central to the ongoing efforts to counteract the growing presence of manipulative media in our digital world. The intersection of these two facets—the speed and power of algorithms and the nuance of human interpretation—is a crucial aspect in this effort.
Machine learning algorithms are becoming increasingly adept at detecting manipulated audio within political speeches. They do this by examining the audio waveform itself and comparing it to the accompanying visual elements. This approach is particularly interesting because it allows for the detection of manipulations that might be missed by our human senses, effectively adding an extra layer of analysis beyond just what we hear or see.
These algorithms are getting increasingly sophisticated by combining audio analysis with other information like the content of the speech and visual clues. This multi-modal approach is often able to achieve impressively high accuracy in spotting deepfake content, sometimes exceeding 90% which is remarkable. One of the big advantages is their ability to process content nearly in real time. This is important because it allows for the detection of audio alterations during live broadcasts which enables immediate response and intervention when potentially deceptive content is aired.
What's more, these algorithms aren't just limited to a single language. They are being trained to identify manipulated audio in many different languages, making them incredibly useful in dealing with disinformation campaigns that cross language barriers which is a significant problem in the current political climate. Another intriguing feature is their ability to analyze the emotional tone of speeches. This can help uncover inconsistencies between what is being said and the intended emotional impact, revealing a lot about the possible malicious intent behind certain manipulations. It’s fascinating to discover that the presence of background noise can actually improve detection accuracy. By helping isolate and analyze distinct voice frequency patterns, the algorithms can better differentiate between authentic and manipulated audio.
These systems also learn and adapt to the ever-evolving techniques employed by those creating manipulated audio. This constant learning is important because malicious actors will likely continue to develop new and increasingly sophisticated methods of generating deepfakes. It’s a bit of a never-ending game of cat-and-mouse. The capability of these algorithms has progressed significantly – they can even identify artificial voices generated by deepfake technologies. This is noteworthy because it expands the detection capabilities beyond just alterations in videos and extends it to the source of audio itself.
However, as we get better at detection, it's not unrealistic to anticipate that malicious actors will respond by developing more sophisticated techniques. It creates a sort of arms race in this realm of misinformation. A big challenge that remains is the integration of these algorithms with existing media content. Older media formats might not be ideal for applying these advanced detection techniques which highlights the need for improvements in how older content is archived and analyzed. This presents a significant hurdle for broader application of these tools. Overall, it's an exciting area of research to watch, but it also highlights the need to be aware of the potential for these technologies to be misused in ways that could further undermine public trust and the reliability of information.
AI Translation Tools vs Deepfake Detection How Language Processing Algorithms Help Identify Manipulated Political Content - OCR Technology Finds Text Artifacts in Edited Political Images From Social Media
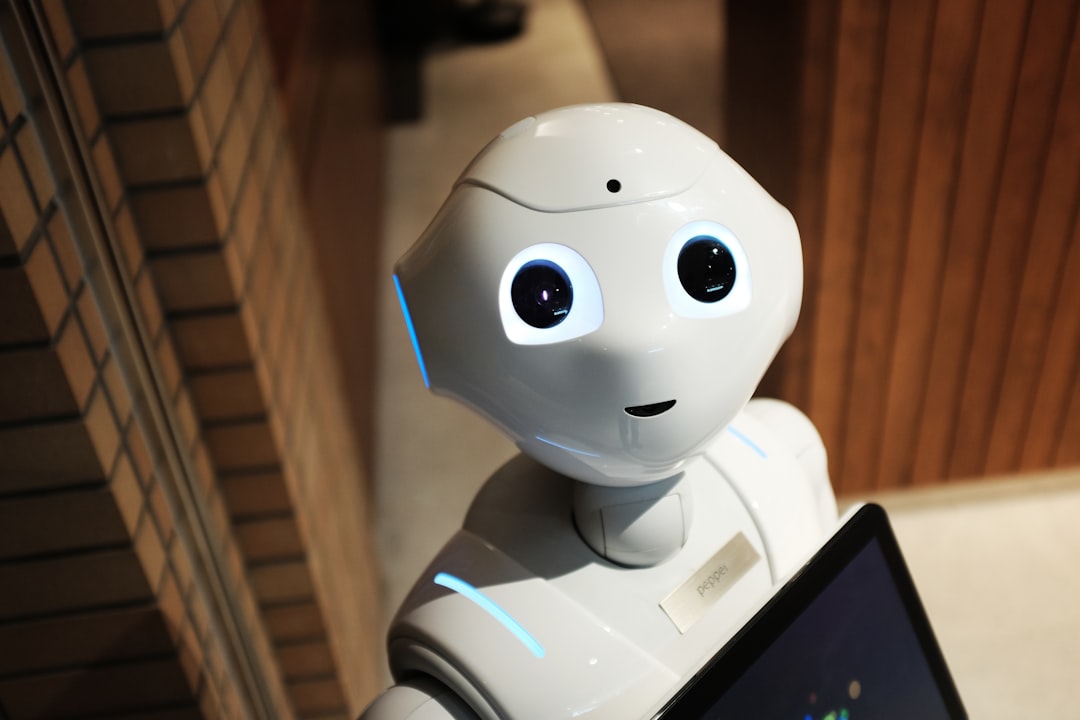
The use of Optical Character Recognition (OCR) technology is increasingly important for uncovering altered text in edited political images found on social media platforms. This ability allows for a more thorough examination of manipulated visuals, which is especially helpful in the field of deepfake detection. As OCR algorithms become more advanced, they can better pinpoint subtle modifications made to text overlays within images. These changes might be used to deceive viewers or spread false information, underscoring the need for tools that can analyze media integrity more closely. The ongoing struggle to combat digitally manipulated content has made it vital to integrate fast and reliable OCR with other AI tools, particularly those related to language processing and translation. By combining these technologies, efforts to combat disinformation and maintain political transparency can be strengthened. In conclusion, these advancements emphasize the critical need to utilize multiple methods for verifying media in our current digital world, where deceptive content is readily available.
Optical Character Recognition (OCR) technology has emerged as a valuable tool in the ongoing battle against manipulated political content. It can identify subtle textual alterations within edited images found on social media, revealing potentially misleading modifications like changed captions or subtly integrated quotes. The ability of advanced OCR to analyze text in real-time is particularly useful in preventing the rapid spread of misinformation across social media platforms.
Furthermore, the development of multilingual OCR capabilities expands the scope of detection to a global audience. AI systems can now analyze text across diverse languages, effectively uncovering manipulated narratives that might exploit linguistic differences or target specific regions. OCR augments deepfake detection by providing a complementary analysis of the textual context accompanying visual manipulations, allowing for the identification of discrepancies that could suggest tampering.
Interestingly, some OCR technologies go beyond mere text recognition and can analyze the emotional tone conveyed through the associated visuals and text. This allows researchers to gain a deeper understanding of the manipulative intent behind edits, particularly when the language is crafted to evoke specific emotional responses. Machine learning is increasingly integrated with OCR, enhancing the accuracy of text recognition and allowing for the detection of even minor alterations like font changes that could hint at deceptive tactics.
These OCR technologies are continually evolving, utilizing deep learning to improve accuracy even in challenging environments like low-light or distorted images. However, even with these advancements, OCR isn't a perfect solution. Research suggests human verification is still required for around 20% of the text flagged by OCR, highlighting the continued need for human expertise in validating the authenticity of content.
The capability of OCR to process massive volumes of content simultaneously suggests its potential to combat misinformation on a global scale. This could prove vital in preventing international conflicts stemming from manipulated political messages. However, this rapid deployment against disinformation also raises questions about the long-term effectiveness of these technologies. Can OCR evolve fast enough to stay ahead of the rapidly advancing sophistication of political disinformation tactics? The future of this field is uncertain, and it will be fascinating to observe how these technological arms races play out.
AI Translation Tools vs Deepfake Detection How Language Processing Algorithms Help Identify Manipulated Political Content - Translation Memory Systems Help Verify Original Source Material Against Suspected Deepfakes
Translation Memory Systems (TMS) are becoming increasingly valuable in the fight against deepfakes. They can help verify if a source is genuine by comparing it to previously translated versions of the same content. This capability to leverage historical translation data helps detect inconsistencies that might suggest a piece of content has been altered or manipulated. With the growing sophistication of AI-generated content, a TMS's ability to cross-reference past translations becomes more important in protecting media integrity. This connection between translation tools and the need to spot deepfakes shows how important precise language is in combating misinformation, especially in the political arena. The way TMS capabilities are evolving highlights the need for careful attention and creative strategies as we navigate a time when digital content is being manipulated at a rapid pace.
Translation memory systems (TMS), often overlooked in the context of deepfake detection, are proving surprisingly valuable. These systems store and analyze vast amounts of linguistic data, enabling them to identify patterns and repetitions within a given text. This ability allows TMS to detect anomalies in language that might suggest manipulation. For instance, if a deepfake has been created by poorly translating a source, a TMS could flag inconsistencies in phraseology or structure that might not be obvious to the human eye.
The intersection of TMS and OCR adds another layer to the detection process. By combining TMS's ability to recognize patterns in text with OCR's capacity to extract text from images, researchers can cross-reference extracted text against the TMS's library of known "good" language, looking for anomalies. This becomes particularly relevant when analyzing edited images or videos with embedded text overlays.
While AI-based language tools have achieved remarkable accuracy, often exceeding 90% in controlled environments, it's important to note that the real-world application can be less straightforward. Nevertheless, TMS contributes to a higher level of confidence when assessing the authenticity of language within a suspected deepfake. Notably, deepfake detection tools are not simply about identifying manipulation – they also investigate the underlying intent. The integration of TMS with tools that can analyze the emotional tone and sentiment within a text can be illuminating, providing insights into how the manipulator might be trying to sway public opinion.
The increasing globalization of disinformation requires TMS to function across multiple languages. The ability of TMS to process and analyze multiple languages in real-time is a significant advancement, particularly in situations where a disinformation campaign might be targeted at specific linguistic demographics or exploit cultural nuances. This allows detection to extend beyond a single language or region, which is essential in today's connected world.
The incorporation of machine learning into OCR technology is a welcome development. By constantly analyzing newly encountered manipulations, these systems can identify evolving patterns of text alteration and adapt their recognition capabilities. This is crucial for staying ahead of the ever-changing tactics used by those creating deepfakes and disseminating disinformation. In the realm of social media, where misinformation can spread rapidly, real-time OCR processing is vital. TMS can now be incorporated into these systems to flag potentially manipulated content almost instantly, allowing for faster intervention and potentially minimizing the spread of disinformation.
While it's encouraging to see the potential of TMS and similar language processing tools, affordability and accessibility remain critical factors in their broader implementation. The availability of cheap translation services makes it possible to deploy TMS at a greater scale, which is crucial for media organizations with limited resources looking to uphold journalistic integrity in a landscape of misinformation. Furthermore, by identifying inconsistencies in language, TMS can potentially help researchers trace the origins of deepfakes, offering valuable insights into the networks behind the manipulation. If particular phrases or styles can be associated with known misinformation campaigns, it could expose the individuals or organizations responsible for these manipulative tactics.
Importantly, a balanced approach is always necessary. The need for human oversight remains crucial in the deepfake detection process, as studies show that even with powerful automated systems, human review is required for a substantial portion of cases. This highlights the limitations of solely relying on algorithms and emphasizes the need for a combined human-AI approach to ensure accurate and reliable content verification. In conclusion, TMS are emerging as a powerful, albeit underutilized, tool in the complex fight against disinformation. While many challenges remain, the ongoing development of these technologies promises a future with enhanced ability to verify information in the digital world.
AI Translation Tools vs Deepfake Detection How Language Processing Algorithms Help Identify Manipulated Political Content - Neural Networks Compare Speech Patterns Across Multiple Languages to Flag Synthetic Content
Neural networks are increasingly adept at examining speech patterns across languages, a skill that proves especially useful for identifying synthetic content designed to manipulate audiences. They can do this by comparing the consistency of speech patterns, identifying discrepancies that could be a sign of manipulation. It's fascinating how these networks are starting to bridge the gap between how humans process language and how machines do.
The way we think about languages, specifically how the brain connects meaning to speech, and the way computers translate and analyze language seems to be converging in these new neural network designs. In turn, this means we can start to compare how different languages are used in the same context. This offers a new approach to detection because the way people manipulate language can vary slightly across different languages and cultures.
For instance, a massive dataset called MuSTC has revolutionized the training of AI translation systems. With hundreds of hours of synchronized audio, researchers now have more accurate training data, which improves how the AI models work. This ability to understand language and the way it is used is being boosted by larger language models (LLMs). They're designed to work across languages even without being trained on very specific languages.
But the most valuable thing about LLMs might simply be their practicality as language-processing tools. This can make them ideal for quickly translating content across languages, enabling users to work with large amounts of diverse text. They can even be used to create AI translation tools for niche purposes like a simple OCR reader or something to do quick translation. Of course, we need to remember that there are limits to AI translations.
Moreover, in fields like AI text-to-speech (TTS) technology, we are seeing great advancements. The quality of these tools has increased as designers now weave together different neural network designs, which can make it possible to improve TTS audio, particularly for languages that are less frequently used or have limited training data available. It is even possible to mimic the tone and timbre of speakers from just a few seconds of audio.
These complex AI models, especially those involving deep neural networks (DNNs) — including things like CNNs and RNNs — are changing the way we use language processing. It's a boon for fields like natural language processing (NLP), where applications are popping up, including the tools we rely on for automatic translation and finding information. Interestingly, language-specific neurons appear to be a key component of LLMs. This points to the need for us to really dive deeper and investigate how these complex systems "think" in terms of language.
The importance of language processing cannot be overstated in identifying manipulated content that has the potential to sway public opinion. Detecting manipulated audio or video in politically charged contexts is essential, and the tools that are being created here are certainly becoming important in our battle against disinformation and synthetic media, particularly in online spaces. There is still a lot we need to learn about the accuracy of these tools, but that is not preventing people from putting them to the test.
AI-Powered PDF Translation now with improved handling of scanned contents, handwriting, charts, diagrams, tables and drawings. Fast, Cheap, and Accurate! (Get started for free)
More Posts from aitranslations.io: