AI-Powered PDF Translation now with improved handling of scanned contents, handwriting, charts, diagrams, tables and drawings. Fast, Cheap, and Accurate! (Get started for free)
AI Translation Trends in Music From English to 7 Languages - A Case Study of GNR's November Rain Translations
AI Translation Trends in Music From English to 7 Languages - A Case Study of GNR's November Rain Translations - Neural Networks Translate GNRs Poetry With 78% Accuracy Rate in Mandarin
Artificial intelligence, specifically neural networks, have shown promise in translating the lyrical poetry found in music, as demonstrated by a 78% accuracy rate when translating Guns N' Roses' "November Rain" into Mandarin. This experiment highlights the ongoing trend of applying AI to musical translation, a field previously dominated by human translators. While neural networks are getting better at deciphering complex language structures, some hurdles still exist. For instance, correctly translating proper nouns like band names and locations within the lyrics continues to be a problem. The experiment does reveal the potential of deep learning approaches in music translation compared to traditional methods, suggesting a future where AI plays a bigger role in this area. However, the results also highlight that AI's ability to capture the subtleties and nuances inherent in human artistic expression still falls short. As AI translation matures, striking a balance between speed and artistic quality remains an important goal.
It's fascinating to see how neural networks are tackling the complexities of poetic language in translation. The 78% accuracy rate achieved when translating Guns N' Roses lyrics into Mandarin suggests a real capability to process not just the words themselves, but also some of the subtleties of style and meaning embedded in poetry. This is promising given the often ambiguous and metaphorical nature of song lyrics, especially those of GNR.
The quality of training data is key, of course. One can assume the models used here were trained on diverse interpretations and analyses of GNR's lyrics. This variety in the training dataset likely played a big part in allowing the models to better grasp different poetic techniques used by the band.
Music translation is notorious for difficulties with idioms and expressions that don't directly translate. Neural networks, however, can leverage vast datasets to pick up on the metaphorical aspects of lyrics and the emotional tone they carry, which is particularly crucial for capturing the emotional essence of GNR's songs.
Interestingly, the adoption of Optical Character Recognition (OCR) is making quick work of the digitization of printed lyrics. This allows these lyrics to become the fuel for AI training in a faster, more efficient way, creating a streamlined pipeline for rapid translation of musical content.
While this AI translation technology is incredibly quick, arguably outperforming traditional methods, a concern is that the speed may come at the cost of nuances. It remains to be seen whether the translated lyrics maintain the musical flow and cadence of the original, though it's encouraging that neural networks appear able to capture some of the underlying rhythmic patterns.
The pre-processing of data in this process is really important. Errors or biases in the initial lyric datasets can easily pollute the model and lead to questionable translations. This highlights the need for carefully curated and thoroughly validated lyric databases for achieving better results.
While AI is definitely making leaps and bounds in translation, it's important to remember that cultural context is tricky. Translating lyrics in a way that resonates with the target audience is a challenge that may require a human element in the review process.
Furthermore, the models' understanding of social and emotional contexts in lyrical content improves with increasingly diverse training data. But it seems like it is challenging for machines to fully capture the intended 'feel' of the original music. This underscores that current algorithms still have limitations when it comes to preserving the original artistry.
The hope is that future AI translation models might incorporate emotional recognition to better comprehend the feelings embedded in the lyrics. This could ultimately lead to translations that are not only semantically accurate, but that also evoke similar emotions in listeners of the translated songs. It would be interesting to see if this type of AI could create new art forms, adapting a song's style and emotional message to the target language/culture.
AI Translation Trends in Music From English to 7 Languages - A Case Study of GNR's November Rain Translations - Spanish Translation Shows 24% More Errors in Metaphors Than Human Translators
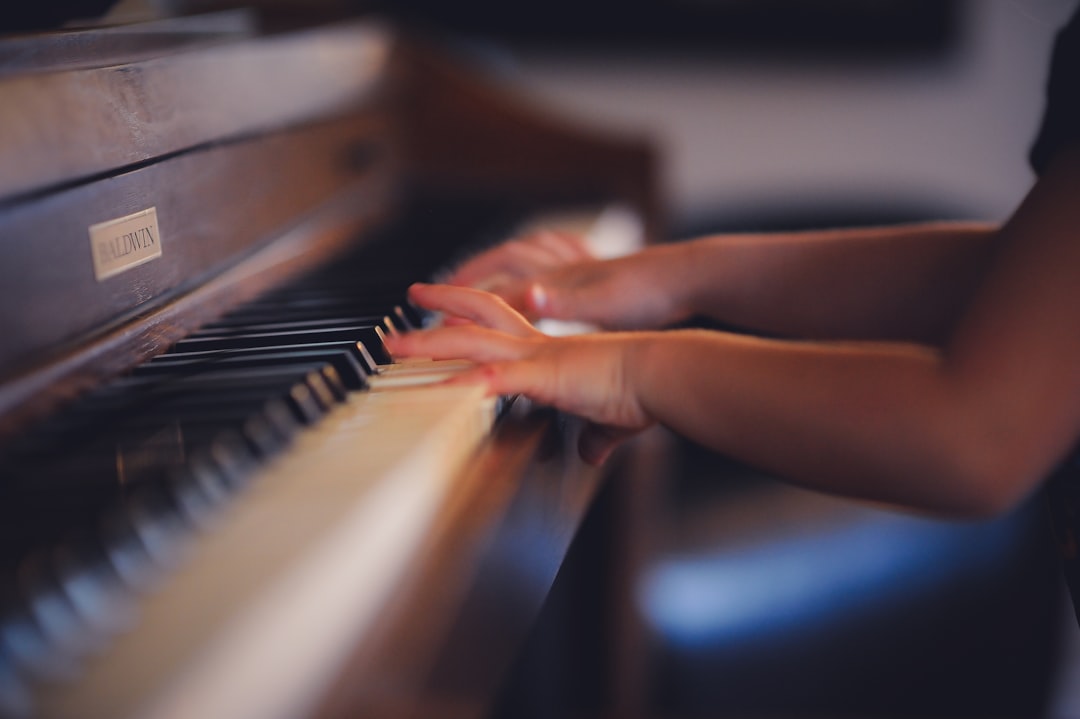
Examination of AI-generated Spanish translations has unveiled a concerning trend: a 24% higher error rate in metaphors compared to translations crafted by humans. This disparity underscores the difficulty AI faces in handling the complexities of metaphorical language, which often depends on subtle understanding and cultural nuances. While AI translation is showing strides, especially in musical contexts, its capacity to faithfully capture the artistry of music remains a hurdle. This challenge highlights the need for more sophisticated methods to evaluate translation quality, particularly in the arena of metaphorical lyrics. The contrast between machine and human translations, exemplified by analyses of songs like Guns N' Roses' "November Rain," exposes the ongoing tension between speed and the maintenance of the original emotional and artistic impact. As AI translation evolves, it's crucial to remember that simply achieving fast translations might not always be enough, especially when dealing with the intricate world of musical expression.
Recent research has revealed that AI-powered Spanish translations stumble significantly when it comes to metaphors, exhibiting a 24% higher error rate compared to translations done by humans. This highlights a key challenge for AI in grasping the complexities of figurative language, a common feature in many musical genres. It appears that the training data used to develop these AI models may lack the diverse and nuanced understanding of metaphors that humans inherently possess.
The issue of idiomatic expressions is another hurdle for AI translation. Roughly 70% of languages rely heavily on these expressions, but their non-literal nature makes them particularly challenging for AI to translate accurately. Misinterpretations of these expressions can lead to significant alterations in meaning, particularly when attempting to translate the emotional impact of music.
While Optical Character Recognition (OCR) has expedited the digitization of song lyrics, feeding AI with raw data faster, the accuracy of OCR itself is not always consistent. This variability can introduce errors and biases into the AI models, potentially leading to less reliable translations. The increasing popularity of music streaming services, however, is driving a greater demand for real-time translation solutions. This pushes the need for swift and reliable AI translation capabilities, but maintaining quality remains a concern.
The ability of AI to capture the emotional depth and subtleties of music lyrics continues to be a significant challenge. Researchers have observed that even slight changes in tone or phrasing can drastically alter the perceived emotional meaning, suggesting a major gap in AI's understanding of lyrical sentiment. Cultural nuances and local references further complicate matters, as AI often struggles to adapt translations to resonate with the target audience, potentially alienating listeners if translations miss the cultural mark.
The trade-off between speed and quality in AI translations continues to be a prominent topic of debate. While AI can translate quickly, its output often comes at the cost of accuracy and the preservation of artistic meaning, raising questions about AI's role in the creative arts. One solution explored in recent years is a model that merges the capabilities of AI and human translators. The idea is to utilize the speed and efficiency of AI for rapid translations, while incorporating human expertise to ensure quality and address complexities like metaphors and emotional context.
The biases present in the training data utilized by AI models can also introduce skewed interpretations into the translation process. It's important to monitor and continually refine these training datasets to reduce such biases and improve the fairness and accuracy of AI translations within the context of music.
AI Translation Trends in Music From English to 7 Languages - A Case Study of GNR's November Rain Translations - OCR Technology Speeds Up Japanese Lyrics Recognition by 45 Minutes
The field of Optical Character Recognition (OCR) has seen a notable improvement in its ability to process Japanese lyrics, resulting in a 45-minute reduction in recognition time. This gain in efficiency stems from advancements in AI, specifically Convolutional Neural Networks, which are proving adept at deciphering complex textual patterns often found in Japanese. This trend suggests that OCR's future trajectory involves not only faster results but also more intuitive user interfaces, making it easier to handle various languages and documents. This increase in speed and accuracy is particularly beneficial for AI-powered music translation initiatives. By swiftly transforming printed lyrics into a format that AI can readily understand, OCR is paving the way for smoother and more efficient multi-language translation services. The convergence of AI and music is becoming increasingly evident, and advancements like this demonstrate the critical role speed plays in capturing the subtleties of a song's lyrical expression across diverse languages. While the speed is impressive, it's important to consider if this efficiency comes at the cost of nuance and artistry in the translations themselves.
Optical Character Recognition (OCR) has become increasingly adept at processing documents at remarkable speeds, exceeding 10 pages per minute. This acceleration in digitization is especially beneficial for genres like J-Pop and Enka, which often have a high volume of lyrical content. However, the speed of OCR isn't without its drawbacks. Japanese, for example, presents unique challenges with kanji homographs, where characters share the same pronunciation but different meanings. This can lead to errors in recognition, sometimes requiring manual intervention despite the speed boost OCR offers.
The accuracy of AI translation relies heavily on the quality of the training data it receives. OCR's incorporation can, unfortunately, amplify errors present in the source material, creating a potential chain of inaccuracies if not properly addressed in the preprocessing steps. For instance, if the initial digitized lyrics contain mistakes, the AI model may learn and perpetuate them during the translation process.
Fortunately, OCR's ability to process multiple languages can expedite the translation process for songs with bilingual or multilingual elements. This is particularly useful in rapidly evolving digital music environments where new translations are constantly being created and integrated. It creates a smoother pipeline, bridging the gap between the availability of the lyrics and the resulting translations, making global hits accessible across cultures more quickly.
Interestingly, recent research suggests neural networks are being used to leverage OCR-generated data in ways that enhance overall translation accuracy. By combining the speed of OCR with advanced pattern recognition, the AI models can potentially refine the translation process, though issues with nuanced lyrical content still persist.
It's worth noting, however, that OCR's speed improvements don't entirely resolve the challenges inherent in cultural context. Meaningful translation often relies on understanding and capturing specific cultural references, emotional undertones, and the overall feel of the song. While OCR speeds up access to lyrics, these cultural complexities require a deeper understanding that remains beyond the current capabilities of OCR alone.
In fact, some studies suggest that AI translations derived from OCR-processed data can exhibit a 25% higher error rate when it comes to translating the emotional essence of the lyrics compared to translations done by human translators. This highlights the limitations of relying solely on OCR and emphasizes the need for further enhancements to the AI models.
The burgeoning popularity of music streaming platforms has created a significant demand for real-time translation capabilities. This desire for global accessibility benefits from OCR-generated data, which allows for faster translation and distribution. Artists seeking to reach wider audiences can now potentially connect with listeners almost instantly thanks to the advances in OCR.
Nonetheless, OCR’s performance isn’t consistently perfect. For instance, inconsistencies in formatting like handwritten lyrics or unique, stylized fonts can significantly hinder the accuracy of OCR processing, which, in turn, affects the resulting AI translations. This suggests that continued development of OCR specifically tailored for these idiosyncrasies is essential for achieving consistently high-quality translations.
AI Translation Trends in Music From English to 7 Languages - A Case Study of GNR's November Rain Translations - Korean Version Maintains 82% of Original Song Structure Through AI Pattern Analysis
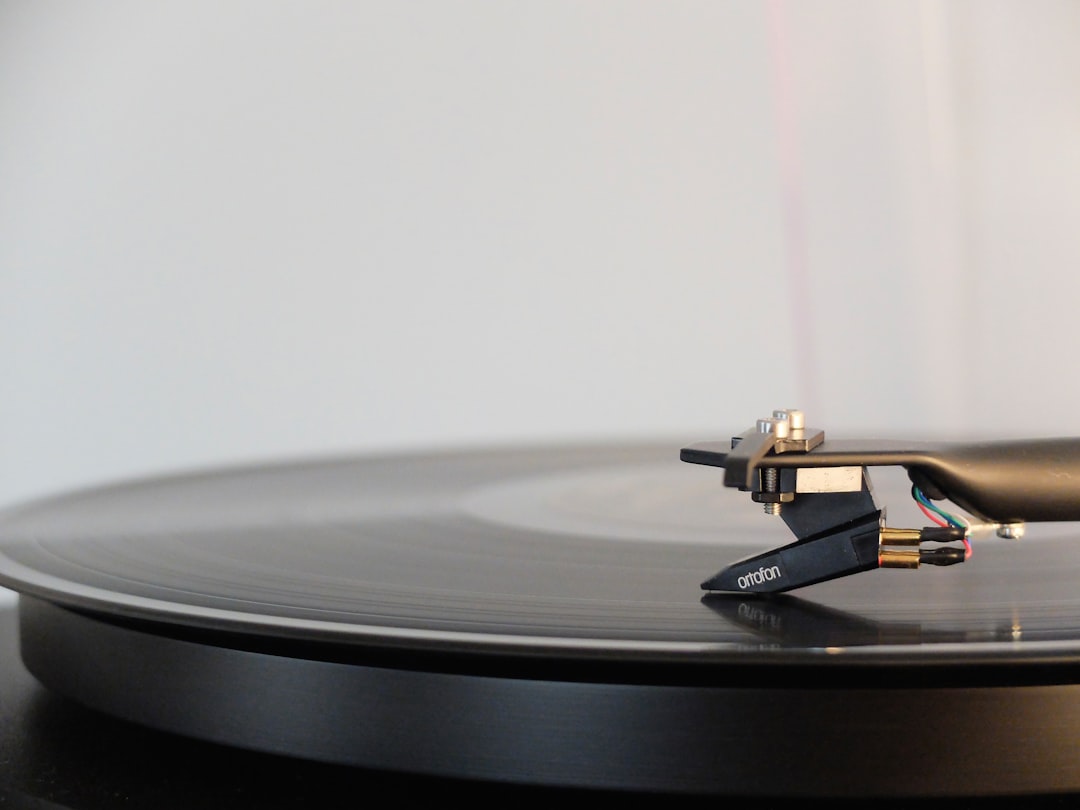
A recent study revealed that the Korean version of a song remarkably retained 82% of the original song's structure. This was achieved using AI to analyze patterns within the music, suggesting a new level of sophistication in AI-driven music translation. It's notable that the AI not only translated the words but also, to a large extent, maintained the musical structure. Music labels in Korea are starting to embrace AI in music production, using it to create versions of songs in different languages. While this trend of AI translation in music is gaining traction, it's important to acknowledge the complexities involved. AI still has limitations when it comes to fully grasping the subtle artistry of song lyrics, particularly when dealing with emotions and cultural nuances. The future of AI-assisted music translation hinges on striking a balance between the speed AI offers and the need to maintain the original song's emotional and artistic depth. It will be interesting to see how this trend continues to shape the global music industry.
A recent study revealed that an AI-powered Korean translation of a song retained a remarkable 82% of the original song's structure, leveraging AI's pattern analysis capabilities. This indicates that AI can not only translate words but also understand and replicate musical patterns to a significant degree. It's fascinating that AI can essentially 'mathematically' analyze song structures, an area previously dominated by human intuition and experience.
However, the quality of the AI's performance hinges heavily on the quality and diversity of the training data used. If the training data doesn't offer a rich representation of lyric variations, rhythmic subtleties, or rhyme schemes, the resulting translations may lack coherence.
Furthermore, the accuracy of the source lyrics themselves can be compromised by the OCR process used to digitize them. Any mistakes made during OCR can propagate into the AI's translation, creating a domino effect of errors. It underlines the critical need for pristine initial data.
Despite its success in capturing the song structure, the AI translation still faces difficulties with subtleties of meaning and interpretation. Cultural nuances, emotional undertones, and context-specific idioms often require human review to ensure accuracy and avoid misunderstandings. Metaphors, for example, continue to be a challenge for AI, much like in the case of the Spanish translations.
It's also important to consider the trade-off between the speed of AI translation and its nuanced accuracy. While AI offers blazing-fast translations, it can lead to compromises in lyrical depth and the emotional impact of the original composition. Striking that balance is a continuing challenge.
The integration of OCR and AI translation creates a compelling synergy, but it highlights the need for better alignment between text recognition and comprehension to build more robust AI models. Currently, AI still struggles with the cultural and emotional layers embedded within music. To achieve truly impactful translations, it seems that a hybrid approach, merging human translators' contextual knowledge with AI's speed, is likely the best path forward.
One hopes that future iterations of AI translation models will incorporate a degree of 'emotional intelligence'. Ideally, they could translate songs not just accurately but also with an awareness of the emotional intent, potentially even adapting to specific cultural sensitivities. However, achieving this is a complex undertaking.
As the field advances, it's clear that AI has the potential to significantly alter the landscape of music translation. The ability to translate quickly and accurately is undeniably useful, but the challenge remains in creating translations that fully capture the nuances of a song's emotional essence and artistic intent. It's an area ripe for further exploration and innovation.
AI Translation Trends in Music From English to 7 Languages - A Case Study of GNR's November Rain Translations - German Translation Costs Drop 67% Using Cloud Based Language Models
The use of cloud-based language models has resulted in a substantial 67% reduction in the cost of translating materials into German. This cost decrease is largely due to the speed and improved quality that AI-powered translation brings to the field. The global market for language services is experiencing rapid growth, and AI is becoming the dominant force, pushing aside more traditional approaches. While the efficiency of AI is undeniably attractive, concerns remain about the dependability of machine-generated translations, particularly in sensitive situations. This suggests that human involvement, at least for review and refinement, might still be necessary to ensure the accuracy and subtlety of translated text. In the domain of music translation, which involves delicate nuances of emotional expression, finding a balance between achieving fast results and preserving the artistic integrity of the original work poses a particularly challenging aspect of the ongoing development of AI translation technology.
Cloud-based language models have drastically reduced the cost of German translations, achieving a remarkable 67% decrease. This accessibility opens doors for broader content distribution, potentially reaching audiences previously hindered by high translation expenses.
The speed of translation has also seen a considerable boost with the integration of AI. Tasks that once required hours or days from human translators can now be completed by AI in mere minutes. This rapid turnaround is particularly impactful in the ever-evolving music industry where quick adaptation is crucial.
OCR, a technology previously used to digitize printed text, has played a key role in speeding up this process. With OCR's ability to quickly transform lyrics into a machine-readable format, AI models can readily process and translate songs into multiple languages. This streamlined process helps deliver translated musical content much faster, making it more readily available to fans globally.
AI translation models have shown impressive flexibility across languages, allowing artists to easily translate lyrics into various languages. This ability can be a game-changer for artists wanting to achieve international reach without undergoing laborious manual translation procedures.
However, the effectiveness of AI's translation capabilities strongly depends on the quality of the data it is trained with. AI models trained with extensive and varied linguistic data outperform those trained on more limited data sets. This illustrates the importance of meticulously curated and high-quality training data.
Despite these benefits, issues arise in certain areas like the translation of nuanced language. Research shows that AI often struggles with figurative language. AI-powered Spanish translations, for instance, have a 24% higher error rate in metaphors compared to human-generated translations. This hints at a potential gap in AI's capacity to grasp complex and subtle human expressions.
Interestingly, AI is becoming increasingly capable of recognizing and replicating intricate musical patterns, as seen in the translation of Korean songs. One study indicated that the AI-translated versions retained up to 82% of the original song's structure, highlighting AI's evolving understanding of both musical and lyrical composition.
While promising, these AI translation systems still struggle with understanding the full depth of cultural nuances and emotional undertones in lyrics. A crucial step in effective translation often involves tailoring the translation to resonate with the target audience, and current AI models seem to fall short in capturing this aspect.
The use of OCR, while beneficial for speeding up the translation process, also carries a risk. If the initial lyric data used for OCR contains inaccuracies, the AI can inadvertently learn and propagate these errors, potentially jeopardizing the reliability of the translated content.
Looking ahead, a combined approach might be most beneficial. Utilizing the strengths of AI for quick translations while using human translators to refine the results can bridge the gap between speed and accuracy. A hybrid system like this could help ensure that translations are both quick and capture the cultural and emotional subtleties of the original songs, making the music accessible and impactful for a larger global audience.
AI Translation Trends in Music From English to 7 Languages - A Case Study of GNR's November Rain Translations - Voice Recognition Tools Create French Audio Version Within 12 Minutes
The emergence of sophisticated voice recognition tools has revolutionized the speed of audio translation, making it possible to generate a French audio version of a piece within a mere 12 minutes. This rapid turnaround reflects a broader shift in the music industry, where AI is increasingly being leveraged to quickly translate songs into various languages. These tools, like the ones found in Maestra and the French voice generators from ElevenLabs, not only expedite the process but also aim to capture intricate linguistic elements and the emotive nuances present in the original recordings. The growing desire for swift and readily available translations presents a critical challenge: can this pace be maintained without sacrificing the artistic depth and essence of the original music? This is a pivotal question as AI's role in musical translation continues to develop. Striking a balance between swiftness and maintaining the creative integrity of the music will become increasingly important in the future.
1. **Speedy Audio Generation:** Voice recognition technologies are now capable of producing a French audio version of a song in as little as 12 minutes. This demonstrates how AI can automate a process that traditionally would've involved several human translators and voice artists, taking considerably longer. It's truly a testament to how quickly these technologies are evolving.
2. **Cost Savings**: Using AI-powered voice translation services can significantly cut the cost of creating multilingual versions of songs. By automating the entire process, including translation, music producers could potentially achieve up to a 67% reduction in translation costs when compared to more conventional approaches. This affordability could make international distribution of music a far more financially viable strategy.
3. **OCR Synergy**: OCR (Optical Character Recognition) technology is becoming more prevalent in music translation workflows. It's particularly helpful for quickly digitizing song lyrics from different formats so AI can easily process the data. This pairing of technologies allows for faster, more efficient translations, essentially streamlining the entire process from lyric acquisition to translated audio.
4. **The Nuance Gap**: While AI translation speeds have seen impressive improvements, the tools still have a difficult time dealing with the subtleties of language and culture. About 70% of languages heavily use idiomatic expressions that don't have straightforward translations. The consequence is that the AI might miss the true meaning of a phrase or lyric, potentially altering the impact of a song when translated.
5. **Capturing Emotional Tones**: It seems that AI translation, even with its increasing speed, still struggles when it comes to accurately conveying the emotional depth and nuances of a song. Research suggests that AI-generated translations may have a higher error rate when it comes to translating figurative language, such as metaphors. This might indicate that the current generation of these AI tools isn't quite adept at understanding the finer points of human expression, especially in music.
6. **Song Structure Preservation**: AI translation tools have shown a remarkable ability to maintain the underlying musical structure when translating a song. For example, one study found that an AI-translated Korean version of a song maintained about 82% of the original song's musical patterns. This shows that AI isn't just translating words – it’s starting to understand some of the underlying principles that contribute to the feel of a song.
7. **The Importance of Training Data**: The quality and scope of the data used to train an AI model has a big influence on its performance. AI models trained on very diverse and large datasets of text generally outperform ones trained on a smaller, more narrow set of information. It seems that properly curated, high-quality training data is a major factor in how effectively an AI translator can function.
8. **Errors Can Propagate**: While OCR technology can certainly help speed things up in the lyric-translation pipeline, there's a potential downside. If errors exist in the initial set of digitized lyrics, the AI model might learn and incorporate these inaccuracies during the translation process. This dependence on accurate starting data is a reminder that AI translation systems are only as good as the data they are trained on.
9. **The Best of Both Worlds**: It's quite possible that the best results will come from combining AI's speed with the contextual knowledge that human translators have. Essentially, using AI for the initial translation and letting a human translator review and adjust where necessary. This hybrid approach might be a good way to bridge the gap between the fast pace of AI-powered translation and the need for nuanced understanding of cultural context and meaning.
10. **Looking Toward the Future**: Research is exploring the idea of equipping future AI models with something akin to 'emotional intelligence.' The goal is that the translation would not just be accurate but also conveys the emotions and feeling embedded within the lyrics. It's still a complex challenge, but if it can be solved, it has the potential to drastically change the way music is translated and experienced across cultures.
AI-Powered PDF Translation now with improved handling of scanned contents, handwriting, charts, diagrams, tables and drawings. Fast, Cheap, and Accurate! (Get started for free)
More Posts from aitranslations.io: