Aurora CEO Chris Urmson Outlines 7 Key Challenges in AI-Driven Vehicle Translation Systems
Aurora CEO Chris Urmson Outlines 7 Key Challenges in AI-Driven Vehicle Translation Systems - AI Alignment Strategies for Autonomous Vehicles
Ensuring autonomous vehicles behave as intended and prioritize safety is a core challenge addressed by AI alignment strategies. Aurora's Chris Urmson highlights the need for AI systems to mirror human driving behavior and adhere to safety standards. This aligns with a broader understanding that autonomous systems should not simply function but also integrate seamlessly with existing norms, like traffic rules. Urmson's perspective suggests that building AI that reflects human expectations is paramount, especially after observing missteps made by others in the field. Aurora's progress towards a feature-complete autonomous driving system suggests the future focus will likely be on integrating these systems into real-world environments, including navigating complex interactions with human-driven vehicles. Moreover, the question of how widespread adoption of autonomous vehicles will affect employment showcases a broader debate around the societal impact of such advancements. It's clear that the path forward must consider both the technological possibilities and the broader implications for society.
Chris Urmson, Aurora's leader, has been discussing how to make sure their self-driving system, the Aurora Driver, acts safely and in a way that's similar to how humans drive. He emphasizes that AI needs to be "aligned," meaning its actions should match what humans want and prioritize safety. He's also highlighted mistakes other companies have made with AI, showing the importance of getting this alignment right.
The Aurora Driver is designed to drive like a human while following traffic laws. Currently, they're doing around 50 test drives per week with safety drivers in the cars. Urmson believes these vehicles should fit into our current roads without needing special treatment. The Aurora Driver is almost ready to handle all the driving tasks a human can, which is a significant step.
He also believes that self-driving trucks won't instantly cause job losses, but will likely lead to a slow shift in the industry. Aurora is continuously exploring how AI can handle real-world driving challenges. Urmson's experience with Google's self-driving car program and DARPA has shaped his views on AI and autonomous technology. His work highlights the ongoing need to figure out how to make sure AI can deal with complex situations and make decisions in the same way a human would, while always putting safety first. This research into human-like decision-making in AI systems is still ongoing and has uncovered many interesting points, though translating human thought processes into code remains a big challenge.
Aurora CEO Chris Urmson Outlines 7 Key Challenges in AI-Driven Vehicle Translation Systems - Balancing Human-like Behavior with Traffic Law Compliance
Chris Urmson, leading Aurora, emphasizes a crucial challenge in developing autonomous vehicles: finding a balance between mimicking human driving and strictly adhering to traffic laws. The aim is to create systems that drive like humans, but this must be coupled with unwavering compliance with traffic regulations. This isn't a simple task, as programming AI to handle situations where human behavior might not align with the law is complex.
Aurora's Aurora Driver is being tested, with a focus on making it behave both realistically and safely. The question remains how well they can achieve both. Ensuring that the Aurora Driver behaves like a human while consistently following traffic rules is key to widespread acceptance and seamless integration into our roads. Ultimately, successfully navigating this balance will determine the future of autonomous vehicles in our society.
One of the intriguing aspects of developing autonomous vehicles is the need to strike a balance between mimicking human driving behavior and strictly adhering to traffic regulations. It's widely recognized that a significant portion of traffic accidents stem from human error, suggesting that AI-powered vehicles, if properly designed, could potentially minimize such incidents. Interestingly, studies on human-vehicle interactions have revealed that effective communication between autonomous and human-driven vehicles can further improve safety by minimizing confusion and misunderstandings on the road. This highlights how crucial it is for AI to not just understand traffic rules, but also to interpret human driving cues.
Furthermore, simulations have demonstrated that AI capable of making real-time decisions can navigate complex traffic scenarios more effectively than even skilled human drivers. This underscores the potential of well-aligned AI to optimize traffic flow while ensuring compliance with the rules of the road. Machine learning, a powerful tool in AI, offers the ability to analyze a vast number of driving situations, allowing autonomous vehicles to learn decision-making processes that closely mirror those of humans. However, ensuring these learned behaviors comply with local traffic regulations and anticipate unexpected actions remains a considerable hurdle.
Integrating sensor fusion, a technique that combines data from multiple sensors, provides AI-driven vehicles with a more comprehensive understanding of their environment, which is important for making decisions that align with both traffic regulations and human expectations. In this context, Optical Character Recognition (OCR) is becoming increasingly important. By rapidly interpreting text from road signs and other visual information, even in different languages, OCR plays a vital role in enabling autonomous vehicles to instantly comprehend and respond to navigational cues. The implications of accurate translation in this context are quite interesting for the development of self-driving technology.
The alignment of AI-driven vehicles with human norms is crucial because any disconnect between AI behavior and human expectations can lead to a loss of trust, potentially hindering the widespread adoption of autonomous technologies. Autonomous vehicles are frequently trained using reinforcement learning, allowing them to continuously improve their responses to dynamic real-world situations. This adaptive approach offers a significant advantage over purely pre-programmed systems, as it enables them to handle traffic laws and unforeseen circumstances with greater flexibility.
Nevertheless, a major challenge remains in programming truly ethical decision-making capabilities into AI systems. While we can train vehicles to follow the letter of the law, the unpredictable nature of human behavior can create scenarios where autonomous vehicles face dilemmas requiring nuanced responses that prioritize safety without violating regulations. The advancement of AI translation technology holds promise for improving overall traffic efficiency and safety. If we can enable rapid and clear communication between self-driving vehicles and other road users, it could lead to more harmonious and predictable traffic flows. However, whether these systems are able to cope with unanticipated human behavior remains to be seen. The future of these technologies is very interesting and I'm eager to follow the progress.
Aurora CEO Chris Urmson Outlines 7 Key Challenges in AI-Driven Vehicle Translation Systems - Data Quality and Quantity in AI Model Training
Developing robust AI models for autonomous vehicles, particularly those focused on translation tasks like interpreting road signs, hinges on the quality and quantity of training data. Aurora's Chris Urmson emphasizes the importance of striking a balance between these two aspects. Relying solely on vast quantities of data without ensuring it's properly organized, labeled, and annotated can result in AI systems susceptible to errors. Conversely, focusing too heavily on high-quality, limited datasets can lead to a lack of diversity and an inability to handle real-world scenarios. This is crucial for tasks like Optical Character Recognition (OCR) where rapid, accurate translation is vital for a vehicle to understand signage and navigate.
The process of training AI models for autonomous vehicles is increasingly data-centric, and the quality of the data used is directly reflected in the accuracy of the system's responses. In this environment, improving the standards for data acquisition and curation is paramount. This will not only improve the ability of AI models to understand and translate data like road signs but also help to build greater trust in the safety and reliability of self-driving technology. As these systems become more sophisticated, the role of data as a foundational element in AI model development is gaining prominence. Achieving both high-quality and abundant datasets is necessary to overcome challenges inherent in training AI for complex tasks in real-world environments.
In the pursuit of building robust AI models for applications like autonomous vehicle systems, the interplay of data quality and quantity presents significant challenges. While larger datasets are often seen as a solution, it's been observed that a model trained on a smaller, meticulously curated dataset can outperform one trained on a larger, less refined collection. This highlights the crucial role of data quality in machine learning, sometimes surpassing the sheer volume of data available.
One aspect demanding close attention is the task of data labeling, especially when dealing with intricate language and complex contexts. Research shows that as the intricacy of language or context increases, the need for human verification rises sharply, potentially doubling the time and expense of preparing training data. This underscores the manual effort often needed to ensure accuracy.
OCR technology, which extracts text from images, has made remarkable progress, reaching over 95% accuracy in recognizing printed text. However, the accuracy dips to around 70% when handling handwritten or stylized text. This difference emphasizes the need for better image processing techniques, especially for applications that involve varying forms of text input.
Furthermore, the diversity of languages can also pose difficulties for AI translation models. Certain languages have unique grammatical structures that can lead to accuracy issues. For example, agglutinative languages like Turkish or Finnish, where multiple suffixes are added to a word, can complicate context understanding for AI models.
The real-time requirements of autonomous vehicle systems introduce further complexities. Translation models used in these systems need to process data extremely quickly – in milliseconds. Studies indicate that even minor delays in response time can heavily affect the safety and performance of autonomous vehicle navigation systems.
Interestingly, synthetic data—data created through simulations—has shown promise in reducing the need for real-world data collection. Simulations have indicated that a carefully designed synthetic dataset can reduce real-world data requirements by over 50% while still maintaining model effectiveness. This suggests an avenue for creating high-quality training data more efficiently.
However, we must also acknowledge a concerning trend: AI models can unintentionally absorb and replicate biases present in their training datasets. For example, if a dataset lacks adequate representation of certain demographics, the model might produce skewed results. This raises important ethical questions, particularly in the context of AI translation where fairness and inclusivity are paramount.
To address these limitations and enhance the capabilities of AI translation systems, human feedback plays a crucial role. Studies indicate that including real-time user feedback can boost translation accuracy by up to 30% compared to models that rely solely on static datasets.
Benchmarking the performance of AI translation models in diverse real-world situations has shown that their performance can vary significantly. Some models trained primarily on specific languages might struggle when tasked with translating dialects or colloquialisms, demonstrating the need for extensive datasets encompassing the diversity of linguistic variations.
Finally, a surprising aspect of AI model training has emerged: models trained on data from unrelated fields like medical or legal documents sometimes demonstrate improved generalization in translation tasks. This implies that diversifying training datasets beyond just language pairs might be a promising strategy for enhancing translation accuracy, opening up a new frontier for AI model development in this area.
Aurora CEO Chris Urmson Outlines 7 Key Challenges in AI-Driven Vehicle Translation Systems - Regulatory Landscape for Self-Driving Technology
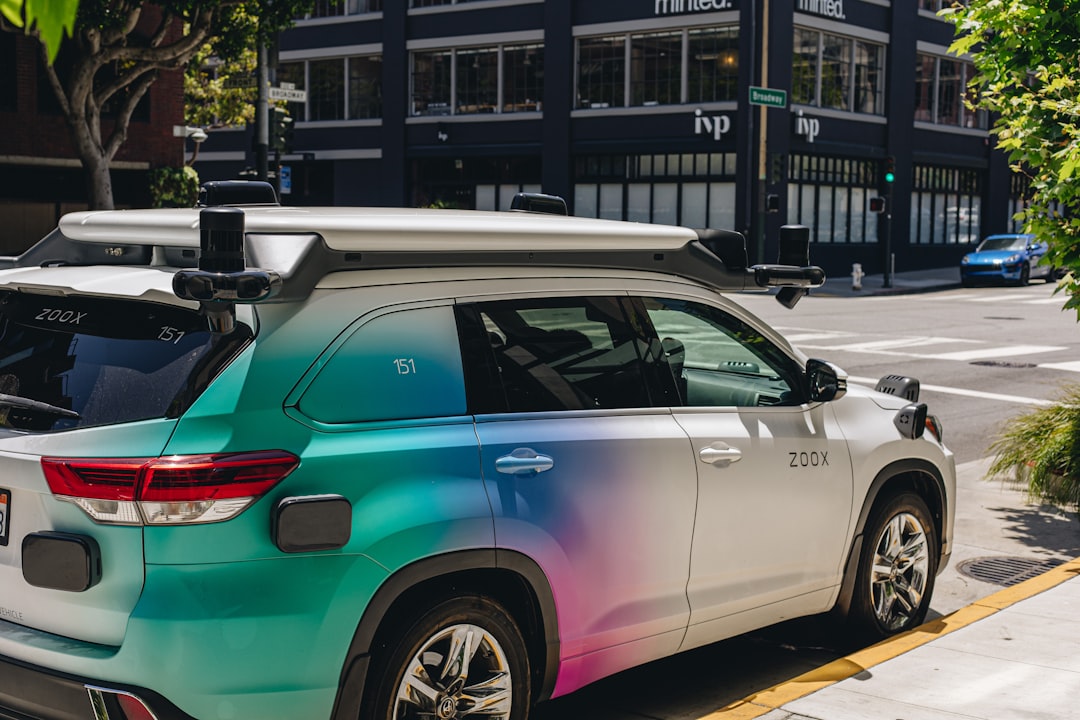
The regulatory environment surrounding self-driving technology is a constantly shifting landscape, posing both opportunities and obstacles for companies like Aurora. As autonomous vehicle development progresses rapidly, regulations often lag behind, resulting in a mix of federal and state laws that can be challenging to navigate. Aurora's CEO, Chris Urmson, underscores the need for companies to successfully manage these regulatory hurdles to gain public trust and ensure safety, particularly as these vehicles aim to seamlessly integrate with existing traffic patterns. The conversation about appropriate regulations for this field continues to gain traction as the technology evolves, encompassing critical areas like liability and ethical considerations. Effective policymaking becomes even more critical in a sector that is undergoing such rapid transformation. The future trajectory of autonomous vehicles and their acceptance by the public will, in large part, depend on how the regulatory landscape develops.
The regulatory environment for self-driving cars is a complex and ever-evolving landscape. Regulations differ greatly, not just between nations, but even within regions of the same country, creating a confusing mix of rules for developers. This makes it hard for companies to design systems that work everywhere, as what's legal in one place might be prohibited in another.
Safety standards for autonomous vehicles are also a big concern. Different areas have different rules, often based on their past accident data. Developers need to make sure their cars meet all the local standards to avoid legal trouble and gain community acceptance.
The insurance industry is changing rapidly too. Since self-driving cars are becoming more common, the question of who's at fault in an accident has become a central issue. There's a growing push to make the car manufacturer or software company responsible, not the person in the car, which could fundamentally alter how insurance works.
Data privacy is another major challenge, particularly with the EU's GDPR. Autonomous vehicles gather a ton of real-time data to function, so companies have to balance the need for this data with the need to protect user privacy. This can be quite tricky with current technology.
Some regulations require that a human driver be ready to take over in tricky situations, which is a hurdle to achieving full automation. It also impacts how users experience these systems.
Luckily, countries are collaborating to try and standardize self-driving rules, which is important to promote safety and encourage innovation globally. It's helpful to have a more unified approach across borders.
However, rules often vary based on the type of vehicle (cars, buses, trucks, etc.). Companies focused on a specific segment have to deal with a unique set of regulations, which can slow down the deployment of technology if changes need to be made to comply.
The ethical dilemmas faced by AI in cars also need to be addressed. There's a lot of discussion about how to build regulations around the decisions these systems make in tough situations. It's a difficult area that also impacts who's legally responsible.
Optical Character Recognition (OCR) technology is crucial for self-driving cars to correctly interpret traffic signs and signals. Rules are starting to require cars to not just see signs, but understand them in the context of the environment, using multiple sensors for safety.
Finally, public perception has a significant impact on regulation. People need to trust these systems, and that trust hinges on perceived safety and adherence to the rules of the road. Regulators are paying more attention to what the public thinks and are making a point to be transparent and involve the community in their decisions.
Aurora CEO Chris Urmson Outlines 7 Key Challenges in AI-Driven Vehicle Translation Systems - Effective Communication between AI Vehicles and Other Road Users
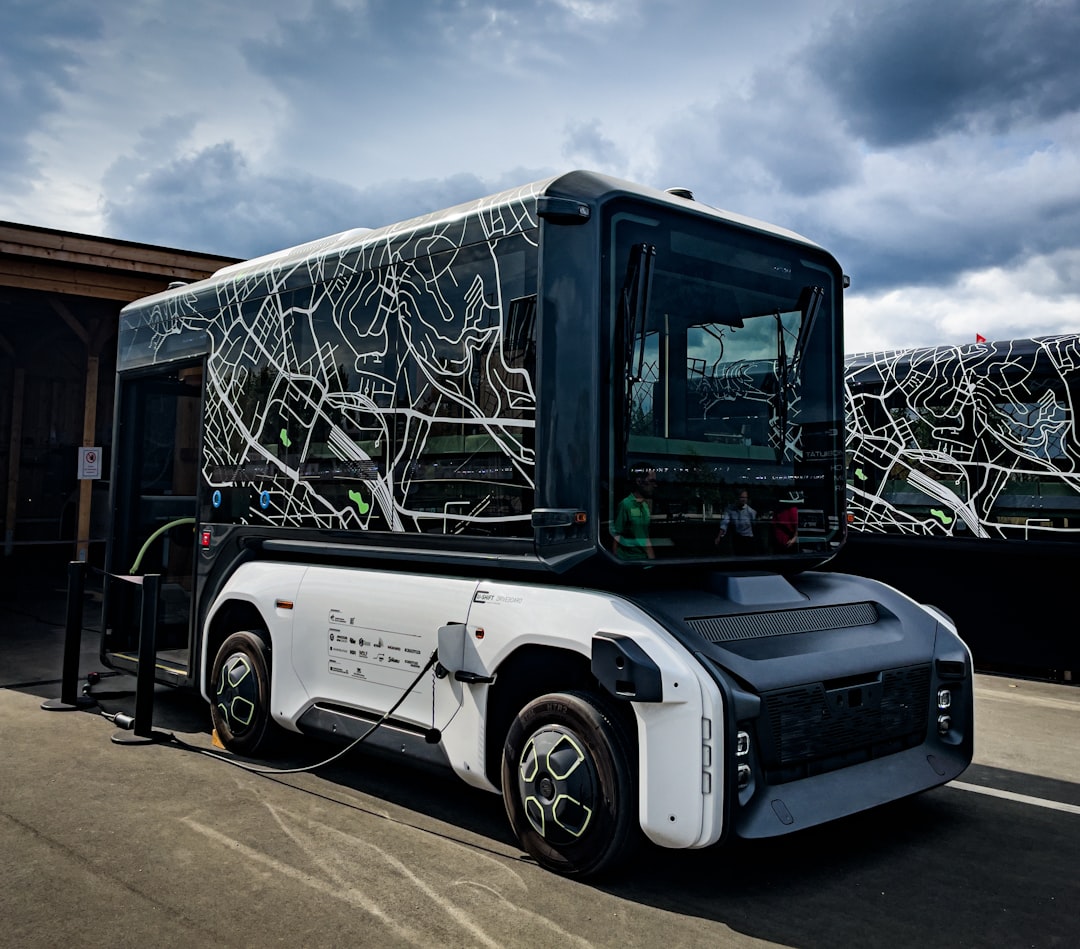
For autonomous vehicles to become commonplace, seamless communication between them and human drivers is crucial. Chris Urmson, at the helm of Aurora, has highlighted the difficulties in making AI-driven vehicles behave in a way that humans understand and trust. He emphasizes the need for autonomous vehicles to not only follow traffic laws but also interpret human driving patterns and react accordingly. This means these vehicles need to be able to understand and respond to a wide range of human behaviors, something that is proving challenging for AI developers.
Technologies like Optical Character Recognition (OCR) are playing a key part in helping vehicles understand road signs and traffic instructions. However, achieving this kind of real-time translation is just one element of the challenge. The larger goal is to develop an AI system that makes decisions much like a human would in a similar context, a process that is still in its early stages.
If the AI systems in these vehicles don't operate in a way that humans can readily comprehend, it can erode public confidence in their safety and viability. This is especially true if the AI makes unexpected or seemingly erratic maneuvers, even if they are perfectly legal or technically safe. It's clear that the evolution of communication methods between autonomous vehicles and humans is a significant factor in determining the future of the technology and how it will impact road safety.
Chris Urmson, the head of Aurora, has been focusing on the challenges of making sure self-driving vehicles can communicate effectively with people on the road. Aurora's approach to AI, called "verifiable AI", centers on creating systems for driving, not general-purpose AI, which is a good starting point in my opinion. Their self-driving technology, the Aurora Driver, is almost ready for commercial use, with their truck technology nearing completion. They're doing about 50 test drives a week with human safety drivers, showing a commitment to safety and data gathering.
It seems like one of the big issues is how well humans and AI can understand each other, kind of like trying to build a common language. Researchers are exploring using things like light patterns to communicate the AI car's intentions to pedestrians or cyclists. It's interesting how this requires a new way of thinking about communication on the road, beyond just traffic signs and signals. We're also seeing how quickly AI can react compared to humans; apparently they're about a tenth of a second faster, which could be crucial for preventing accidents.
There are cultural differences in the way people interpret things, too. The same sign in one country might mean something entirely different elsewhere, which means AI systems need to be smart enough to adapt. That ties into the idea of trust; if a vehicle consistently does what people expect, such as stopping for pedestrians, they're more likely to trust the technology. Researchers are even trying to give AI a 'sense of attention' using sensors to detect where nearby people are looking, kind of like eye contact.
I wonder if one day we can make AI adapt its communication based on previous situations. This ongoing learning might be a big step toward seamless human-AI interaction on the road. It's fascinating how machine learning could be used to predict human actions, like whether a pedestrian will cross the road or a car will change lanes.
Using different types of information, like images, laser data, and sounds, is becoming important to understand the entire situation around the vehicle. Ultimately, the design of communication methods, like visual displays and audio cues, will be key. They need to be easy for humans to understand so we don't end up with more confusion on the road. All of these things are still pretty new, and it seems like the development of self-driving tech will be directly linked to how well we can solve these communication problems. It's a dynamic and evolving field, and I think it will be very interesting to see where this research leads in the future.
Aurora CEO Chris Urmson Outlines 7 Key Challenges in AI-Driven Vehicle Translation Systems - Integration Challenges with Existing Transportation Infrastructure
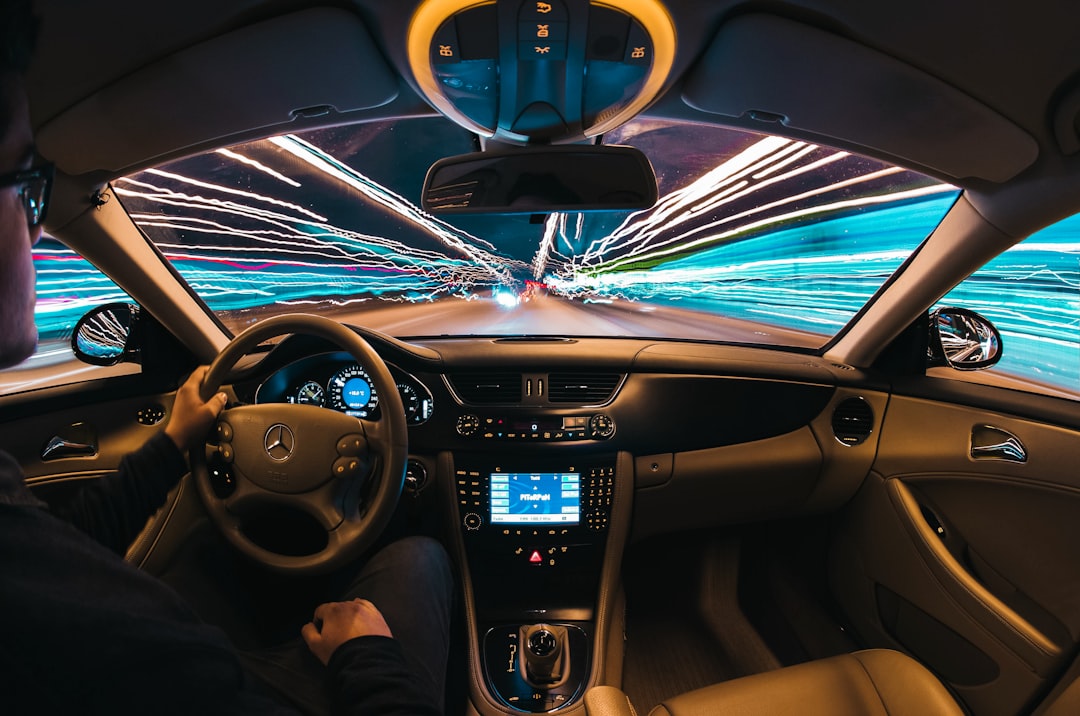
Integrating autonomous vehicles into our current transportation infrastructure is a complex challenge. It requires adjusting existing road layouts, traffic control systems, and regulations to handle the specific needs of these self-driving vehicles. Aurora's Chris Urmson highlights the need for solutions that work within our current infrastructure, avoiding major overhauls. He believes that gaining public acceptance and navigating the regulatory landscape are crucial for widespread adoption of this technology. Additionally, ensuring clear communication between autonomous vehicles and human drivers is essential, as any confusion or unexpected behavior could raise safety concerns and hinder public trust. The balance between integrating new technology and existing infrastructure will significantly shape the future of autonomous transportation.
Integrating autonomous vehicles into our existing transportation infrastructure presents several challenges. One issue is that the advanced technology in AVs can sometimes be at odds with current road designs and traffic control systems. For example, an AV might react faster than a human driver expects, which could lead to safety concerns.
OCR, a key technology for reading road signs, while quite good at recognizing printed text, struggles more with handwritten signs or those with unique fonts. This variability makes it hard for AVs to reliably understand road signs, especially in real-time.
Human drivers are notoriously inconsistent in their actions on the road. Studies show this unpredictable nature can throw off AI trained on more structured data, highlighting how difficult it is to bring AVs into our existing traffic flow.
The communication aspect is tricky too. AVs have to interpret not only static road signs but also subtle cues from human drivers. If an AI misinterprets a human gesture, it can lead to miscommunication and potentially unsafe situations.
Different regions have different driving cultures, which makes it hard to create an AV that functions seamlessly everywhere. Variations in driving habits across areas can cause misunderstandings and dangerous interactions.
Regulations haven't kept up with the speed of AV development. This has resulted in a confusing mix of local and national laws that make innovation and integration more difficult.
Sensor fusion, which combines data from multiple sensors on an AV, is useful but also has its limitations. Mixing data from different types of sensors can lead to conflicting interpretations that complicate the AI's decision-making process.
Public trust in AVs is heavily influenced by how well they can predict human actions. AI that drives in a way that humans expect usually results in higher trust levels. Achieving this level of expected behavior requires a lot of work in modeling human driving patterns.
Machine learning is being explored for its ability to predict how human drivers might act in various situations, like a sudden lane change or a stop. How accurate these predictions are can significantly impact safety and the efficiency of AVs interacting with human-driven vehicles.
There's a big challenge in translating traffic signs across different languages and cultures. Symbols and words mean different things in various places, which means AVs need advanced translation capabilities to interact with drivers globally.
Aurora CEO Chris Urmson Outlines 7 Key Challenges in AI-Driven Vehicle Translation Systems - Societal Impact and Job Market Shifts in AI-Driven Transportation
The rise of AI in transportation is rapidly altering the landscape of employment and society as a whole. As autonomous vehicles become more commonplace, the potential for automation to replace human workers in certain roles becomes a significant concern. This shift will likely lead to a reshuffling of occupations and demand for different skillsets, forcing workers and employers to adapt. While some anticipate job losses in certain areas, others believe AI-driven transportation will generate new jobs in related industries. However, the potential for dehumanization in the workplace is a serious issue that needs to be addressed. Concerns about the emotional well-being of workers facing AI-driven changes are valid and should guide the implementation of these new technologies. The challenge lies in finding a balance between the efficiency and safety benefits of AI with the need to maintain a human-centered approach to work and transportation. Striking this balance will be crucial for ensuring a smooth and equitable transition for everyone involved.
The rise of AI in transportation, particularly in autonomous vehicles, is expected to significantly reshape the job market and societal structures. There's a growing concern about potential job displacement as automated systems take over tasks previously handled by human drivers, especially in industries like trucking and ride-sharing. While some see this as a major disruption, others argue that new job opportunities will emerge in related fields like AI maintenance, safety monitoring, and system development. To ease the transition, significant workforce training initiatives will likely be necessary to equip current workers with the skills needed to adapt to this new technological landscape, ensuring that the workforce evolves to meet the changing needs of the sector.
AI-driven transportation has the potential to revolutionize urban mobility by optimizing traffic flow, reducing congestion, and improving overall efficiency. But, implementing these changes will require substantial adjustments to existing infrastructure, including the adoption of smart traffic management systems and the integration of autonomous vehicles into the current road network. One of the complexities arising from this transition is adapting AI systems to handle the varied driving habits and cultural nuances found across different regions. Traffic laws and driver behaviors are not universal, leading to situations where an AI's decisions might be appropriate in one region but inappropriate in another. This adds a layer of complexity to developing safe and reliable autonomous vehicles that can operate effectively in different environments.
The sheer volume of data generated by autonomous vehicles is unprecedented, creating a need for new, sophisticated data management systems. These systems will not only be essential for monitoring the performance and safety of these vehicles but will also provide valuable insights into how humans interact with and react to this new form of transportation. This data can inform future AI enhancements and potentially optimize the integration of autonomous vehicles into the broader transportation ecosystem.
As autonomous vehicles gain prominence, the regulatory landscape is evolving to address critical issues like safety standards and liability. Authorities are carefully evaluating safety performance, often comparing it to human-driven vehicles, and establishing standards that will ensure public confidence in the safety of these systems. However, defining what constitutes "safe driving" in the context of AI is a complex issue as it involves different considerations and decision-making processes compared to human drivers. Additionally, the question of liability in the event of an accident is still being debated. Determining whether the responsibility lies with the vehicle manufacturer, the software developer, or a combination of factors will impact the insurance industry and necessitate new models for covering potential risks.
The fast-paced nature of autonomous vehicles necessitates AI systems that can make critical decisions in a fraction of a second, far exceeding human reaction times. Studies have shown that even seemingly negligible delays in response time can have a substantial impact on safety. This underlines the critical importance of developing efficient and reliable AI that can process information and make decisions with incredible speed and accuracy. While some concerns exist regarding job displacement, the potential for AI-driven vehicles to optimize traffic flow and reduce congestion remains a compelling argument. They can potentially reroute traffic in real-time, minimizing bottlenecks caused by human errors and improving traffic fluidity overall.
The integration of AI into transportation inevitably raises ethical questions that demand immediate attention. As AI systems become capable of handling complex and morally ambiguous situations on the road, we need to create robust ethical guidelines and standards. For instance, programming AI systems to navigate situations where they must choose between the safety of passengers and pedestrians necessitates a carefully crafted ethical framework that ensures these technologies operate in a way that is both safe and responsible. The development of these ethical frameworks is critical as AI systems continue to integrate into our society and make increasingly complex decisions.
More Posts from aitranslations.io: