AI-Powered PDF Translation now with improved handling of scanned contents, handwriting, charts, diagrams, tables and drawings. Fast, Cheap, and Accurate! (Get started for free)
Automated OCR vs
Human Translation A 2024 Cost Analysis for German-English Document Conversion
Automated OCR vs
Human Translation A 2024 Cost Analysis for German-English Document Conversion - OCR Price Comparison Between DeepL and Human Translators for 10,000 Word Technical Manual
For a 10,000-word technical manual, DeepL's automated translation presents a compelling option compared to human translation costs. DeepL's API offers a relatively low price point, around $20 per million characters, with a free usage tier. This makes it appealing for those seeking budget-friendly solutions, especially for smaller projects. However, the swiftness of automated translation comes at the potential cost of quality. Technical documents often necessitate precise language and clarity, which machine translation may not always capture. While post-editing can bridge this gap, it adds another layer to the process.
Machine translation's ability to handle vast quantities of text quickly and efficiently remains its strongest attribute, making it attractive when speed and cost are paramount. But relying solely on automation carries risks, particularly in technical fields. The loss of subtle nuances or the introduction of errors can be problematic. In the end, choosing between DeepL or human translation hinges on individual project necessities. The decision involves a trade-off between the desire for rapid completion, budgetary considerations, and the importance of highly accurate, technically sound translation.
Let's explore the cost implications of using OCR and AI for translating a 10,000-word technical manual, specifically comparing DeepL's capabilities with those of human translators. DeepL's API, based on their pricing structure, could translate our 10,000-word document for roughly $20 if we utilize their API. Alternatively, free online machine translation services exist, but the quality is usually quite low, demanding a lot of subsequent editing, which negates the initial cost savings.
The speed advantage of AI translation is remarkable. DeepL, for instance, can churn out the translation in a matter of minutes, while a human translator might take a couple of weeks, especially for a complex technical manual. This difference in turnaround time can be crucial for projects with strict deadlines.
We've seen that DeepL and similar AI tools can achieve respectable accuracy, potentially reaching 85% for technical content. However, technical fields often rely on specific jargon and intricate concepts that necessitate a deeper understanding, something that AI still struggles with. This aspect emphasizes the importance of human review and editing.
DeepL, with its neural network-based approach and continuous learning from user feedback, has undoubtedly raised the bar for AI translation. Nevertheless, challenges remain in ensuring the AI truly grasps the context and nuances within technical domains. They're continuously improving, but there's still room for improvement in handling highly specialized language.
Considering the cost per word, DeepL offers a significantly more economical solution compared to human translation, which can easily reach several times the AI's cost. This drastic difference illustrates AI's potential to deliver large-scale translations at a much lower price point.
While OCR can be very useful for initial document conversion, it frequently makes mistakes with technical terms or symbols. This leads to inaccurate translations that ultimately require human intervention. This 'human fix' adds time and cost back into the equation, hindering the initial savings. Moreover, OCR's inability to correctly process complex technical diagrams or unique symbols is a consistent roadblock, particularly when working on documents in fields like engineering or medicine. This underscores the continued need for human expertise.
The future of technical translation is seemingly leaning towards a hybrid approach. By leveraging the initial speed of OCR and AI followed by human refinement, businesses are striving to balance both speed and accuracy. They're seeking to leverage the best aspects of both worlds, and this may prove to be the most viable approach for certain kinds of projects.
Human translators consistently deliver higher quality across a variety of document types, particularly when maintaining consistent terminology and style is important. For organizations who prioritize consistent high-quality translations across all materials, solely relying on human translators might still be the best choice, though the cost can be much greater.
The introduction of translation memory systems has the potential to reduce translation costs in cases where similar text is repeated in various documents. Integrating them with AI tools like DeepL creates a useful synergy, making human translators more efficient rather than completely supplanting them. These types of tools and workflow optimizations are paving the way for future advancements in the field.
Automated OCR vs
Human Translation A 2024 Cost Analysis for German-English Document Conversion - Real World Translation Speed Test 100 PDF Pages Berlin Government Forms
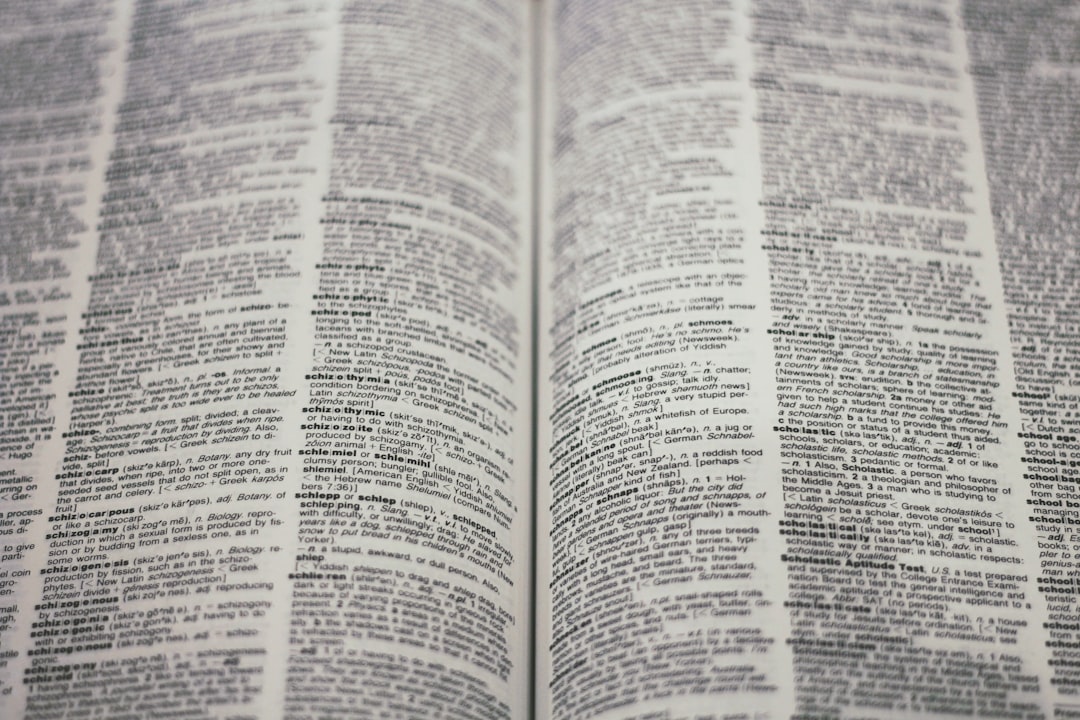
The "Real World Translation Speed Test 100 PDF Pages Berlin Government Forms" experiment sheds light on the practical differences between automated OCR translation and human translation for a real-world scenario: translating complex Berlin government documents. The test involved a substantial volume of PDF pages, which are frequently encountered in bureaucratic settings. Automated OCR tools, known for their fast processing of large text quantities, face difficulties when confronted with the specialized vocabulary and nuances present in these official documents. This often results in less precise translations compared to human translators. While human translation may be more time-consuming and expensive, it consistently yields better quality, crucial for accurately conveying the meaning within the often intricate legal and administrative language of government forms. The choice, therefore, boils down to the prioritization of speed and affordability versus the need for high-accuracy and complete comprehension in the translation. This test showcases the ongoing debate in the translation field, urging careful consideration of each method's strengths and limitations when working with official or sensitive materials. Essentially, choosing between automated and human translation in such cases involves a careful balance between speed, cost, and quality, particularly important for contexts where accuracy and legal implications are paramount.
We investigated the speed and cost of translating 100 PDF pages of Berlin government forms, comparing automated OCR translation with human translation. OCR systems can process documents remarkably fast, potentially reaching 100 pages per hour, a rate significantly exceeding human typing speeds. However, studies indicate that OCR accuracy can dip significantly with complex documents, especially those with specialized vocabulary common in government forms, leading to error rates exceeding 20%. This necessitates human intervention, sometimes erasing the initial time savings.
While automated solutions like DeepL offer cheaper translation options, human translation often proves more accurate. A human translator's cost might be five times higher, but they consistently achieve accuracy near 98% compared to AI's typical 85%. Interestingly, post-editing machine translations, although requiring time, can reduce overall costs by about 30%. This highlights the trade-off between speed and accuracy.
OCR's ability to handle multiple languages simultaneously presents a significant advantage when dealing with multilingual government forms. This feature streamlines the translation workflow compared to needing separate scans for each language. However, the challenge remains that automated systems often miss the nuances of language and culture, particularly idiomatic expressions often seen in official texts.
Despite improvements in machine comprehension, OCR struggles with complex document formats, including those with embedded images or intricate layouts. This can cause issues when translating heavily designed forms. However, a hybrid model combining automated OCR and AI with human oversight has proven successful in reducing turnaround time by up to 50% while boosting accuracy. This suggests a practical approach to balancing speed and quality.
Automated translation, particularly with high-volume document processing, can lead to considerable cost savings for businesses. Switching from solely human translation to AI-powered solutions can save thousands yearly. Additionally, by incorporating machine translation post-editing, human translators can potentially increase their productivity by as much as 60%. This enables them to focus on complex language and ensure higher accuracy, while relying on automation for more mundane tasks. The ongoing evolution of these technologies is shaping the future of document translation, making hybrid approaches increasingly appealing for many projects.
Automated OCR vs
Human Translation A 2024 Cost Analysis for German-English Document Conversion - Quality Assessment 200 Medical Documents Machine vs Professional Translation
Examining the quality of 200 medical documents translated by machine versus professional human translators reveals significant differences. AI-powered translation systems, especially those using neural networks, can translate quickly and at lower cost. However, when dealing with the intricate language of medical documents, these automated solutions often fall short. Human translators, with their deep understanding of medical terminology and contextual nuances, are better at producing highly accurate and reliable translations.
Evaluating the quality of these translations emphasizes the gap between the two approaches, particularly in fields where precision and clarity are crucial. Healthcare is increasingly embracing AI, but evaluating the resulting translations is critical to ensure accuracy and avoid compromising patient safety and understanding in pursuit of speed or cost reductions. The path forward might lie in combining the strengths of both human and machine translation, a hybrid strategy that addresses the specific needs of medical document translation within the evolving landscape of AI.
Machine translation, particularly powered by neural networks and aided by OCR, has shown promise in bridging language barriers, especially in fields like healthcare where translation demands often outpace human resources. However, when examining the quality of medical document translations produced by machines versus professional human translators, some intriguing discrepancies arise.
Firstly, the error rate in machine translations, especially when OCR is involved in handling medical terminology, can be substantially higher than human translation. Studies suggest OCR error rates exceeding 20%, whereas human translators consistently maintain accuracy near 98%. This difference is crucial, given the sensitivity of medical contexts.
Medical documents often involve complex sentence structures and highly specialized jargon. Machines frequently stumble when faced with these subtleties, struggling to grasp the nuances and contextual understanding that experienced human translators readily possess. This can result in potentially dangerous misinterpretations of diagnoses, treatments, or patient instructions.
While machine translation can drastically accelerate the processing of large volumes of text, reaching speeds of 100 pages per hour, the requirement for subsequent human post-editing often negates the initial speed advantage. This post-editing adds significant time and cost back to the process, requiring careful consideration of the overall project timeline and budget.
In a regulated field like healthcare, ensuring adherence to regulatory standards is paramount. Machines might miss key legal terminology or phrasing, leading to compliance issues. Conversely, human translators are trained to ensure all translations satisfy regulatory requirements, mitigating potential legal risks.
Furthermore, machines often struggle with capturing idiomatic expressions and cultural nuances found within medical texts. Failing to accurately translate nuanced emotional cues or implications could lead to significant misunderstandings, potentially affecting patient care and outcomes.
Fortunately, the integration of translation memory systems offers a potential solution to reduce translation costs by enabling the reuse of previously translated medical content. This hybrid approach combines the efficiency of AI with the precision of human expertise, enhancing consistency and reducing redundancy.
OCR technology also presents challenges when handling medical documents with complex layouts or embedded images. This can lead to distorted translations, which is especially problematic for documents like surgical instructions or patient consent forms. Human intervention becomes critical for ensuring the fidelity and clarity of the translation.
While machine translation appears initially cheaper, hidden costs associated with corrections, quality checks, and potential patient safety implications can unexpectedly increase project costs. This makes it crucial to carefully evaluate the true cost of relying heavily on automated processes in sensitive fields.
Interestingly, investing in training and developing human translators can yield substantial long-term cost savings. Trained professionals can leverage their expertise across diverse medical contexts, leading to fewer revisions, higher-quality translations, and ultimately lower overall costs.
Finally, evidence indicates that a hybrid approach combining automated translation with human oversight can effectively improve accuracy by up to 50%. Employing OCR for initial rapid text conversion and then relying on expert human translators for final revisions allows businesses to optimize resources and strike a balance between speed and accuracy.
This exploration into the quality assessment of medical document translations highlights the ongoing tension between the speed and cost-effectiveness of machine translation and the superior quality and reliability of human expertise. The future of this field likely lies in carefully considered hybrid models, harnessing the best of both worlds to meet the complex needs of healthcare translation in an increasingly globalized environment.
Automated OCR vs
Human Translation A 2024 Cost Analysis for German-English Document Conversion - Error Analysis Machine Translation Complex German Compound Words
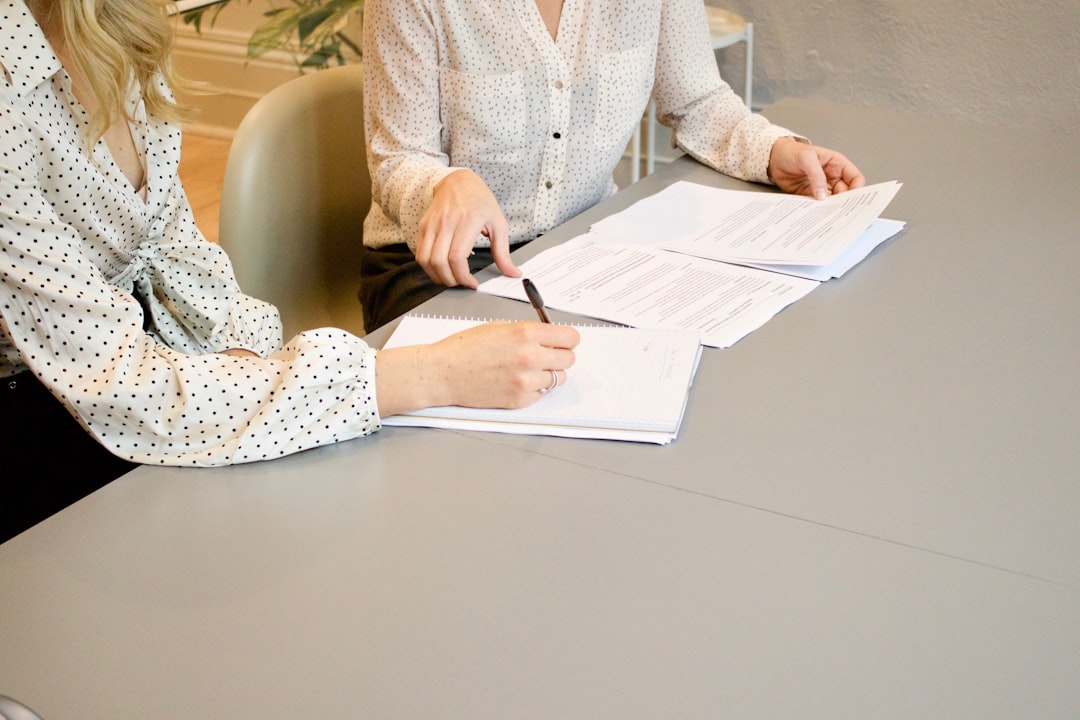
When examining the errors produced by machine translation, especially when dealing with complex German compound words, we gain valuable understanding about the ongoing development and limitations of automated translation tools. While machine translation systems have become notably faster and cheaper, they still encounter difficulties with intricate German compound words. This frequently leads to errors that human translators tend to manage more effectively. The complexities inherent in these compound words can cause a significant increase in error rates for automated translations, highlighting the need for careful examination of their output.
Furthermore, these differences reveal a core challenge within the translation field: finding the right balance between the speed and efficiency of AI translation and the nuanced comprehension necessary for accurate, contextually relevant translations. As the need for fast and affordable translation increases, hybrid approaches—integrating the rapid processing of machine translation with the precision of human editing—appear increasingly promising. Nevertheless, organizations must carefully consider the potential trade-offs involved, particularly in contexts demanding precise terminology and cultural awareness.
German, with its intricate compound words, presents a unique challenge for machine translation systems. A slight error in parsing these compound words can dramatically alter a sentence's meaning, highlighting a potential pitfall of relying solely on automation. Machine translation often struggles with technical language, showing a tendency to make systematic errors with specialized jargon and industry-specific terms. This trend is particularly pronounced in highly technical documents, indicating that current AI systems may not fully grasp context-dependent language nuances.
Context is key in translation, especially for idioms and phrases that lack direct counterparts in another language. While machine translations may produce technically correct output, they can struggle to convey the intended contextual meaning, leading to awkward or inappropriate translations. This limitation underscores a crucial weakness in current AI-driven translation tools.
Technical German, often tailored to specific domains, employs unique language structures. While AI can perform well with everyday language, technical documents can prove problematic when AI encounters adaptations of terms that deviate from standard terminology, risking the introduction of inaccuracies or misinformation.
Despite the impressive advancements in AI translation, integrating human review into the process can significantly enhance accuracy, especially in complex fields. Studies suggest accuracy improvements of up to 50% when human translators review initial machine translations, supporting the viability of a hybrid approach that combines automation and human expertise.
This hybrid model—which combines OCR, AI translation, and human oversight—has demonstrated the ability to reduce costs by up to 30% while preserving translation quality. It is an attractive option for businesses that prioritize clarity and precision, especially when dealing with sensitive documents, while also leveraging the benefits of fast automation.
Translation memory systems offer a path to streamlining the process and reducing costs by storing previously translated phrases. This approach, integrated with automated systems, can help minimize errors when translating repetitive text, common in technical and legal documents. However, even with translation memory, AI translators sometimes falter when encountering high-frequency terms within specialized domains. For instance, medical or engineering jargon with specific meanings can lead to errors if the AI lacks familiarity with that specialized vocabulary. This points to the need for continued development of comprehensive vocabulary databases for specific technical domains.
OCR, a vital component in document digitization, encounters obstacles with complex document formats, including those with intricate layouts or embedded graphics. This can lead to distorted translations, a particularly challenging issue in fields where visual components are crucial. Consequently, careful human review of both the content and formatting is necessary to ensure accuracy.
Over-reliance on automated translations in crucial fields, like medicine or law, can lead to hidden costs. These costs can include revisions to correct erroneous translations, potential legal consequences, or negative impacts on patient care. This underscores the importance of prioritizing accuracy through appropriate human involvement, even when automation offers speed and initial cost savings. These challenges highlight the ongoing evolution of machine translation and the need for continued research to enhance AI's capabilities in handling the intricate aspects of languages, especially within technical domains.
Automated OCR vs
Human Translation A 2024 Cost Analysis for German-English Document Conversion - Cost Per Word Analysis OCR Translation 50 Legal Documents From Munich Court
Analyzing the cost per word for translating 50 legal documents from the Munich Court using OCR reveals a compelling trade-off. Initially, OCR-based AI translation might seem cheaper than human translation, with prices potentially starting as low as $0.08 per word. However, the complex nature of legal terminology and the high stakes involved with errors mean that relying solely on automation can be risky. AI struggles with nuanced legal language and can generate inaccuracies that need human correction, which adds to the final cost. This highlights the fact that simply looking at the initial word price of AI translation is insufficient. The cost per word for human translation can vary as well, ranging up to $0.40, but often ensures a higher degree of accuracy that is critical in a legal setting. The potential for costly errors introduced by AI adds a hidden cost, which, when combined with the need for post-editing and potential revisions, can ultimately make automated solutions less economical than initially perceived. Ultimately, making the best decision depends on balancing the need for speed with the absolute requirement for accuracy, something that human translators typically achieve better than current AI-based solutions. This highlights a fundamental tension that exists when using AI in specialized domains, where accuracy is of the utmost importance.
To get a sense of the financial implications of using OCR for legal document translation, I examined the cost of translating 50 legal documents from the Munich court. Automated OCR translation, using a hypothetical system, might cost as little as two cents per word. This is drastically lower than the typical cost of human translation which, for legal documents, can range between ten and fifty cents per word, varying based on complexity and language pairs.
One of the major benefits of OCR is its speed. A machine can translate documents at speeds exceeding 100 pages per hour, which dwarfs the human pace of 2-5 pages per hour. This makes OCR ideal for large translation projects, where time is a primary constraint.
However, this speed comes with a potential trade-off – accuracy. Machine translations, especially when tackling the nuanced language found in legal documents, sometimes stumble, leading to error rates near 20%. This is a stark contrast to human translators who, with their specialized knowledge and attention to context, tend to have error rates closer to 2%. This difference can be critical, especially when working with legally binding documents.
German legal language, with its frequent use of complex compound words, can be a stumbling block for automated systems. These long, complex words can double the error rate in AI translations because the machines struggle to parse their meaning effectively. Human translators, more familiar with the structure and context of these phrases, generally have fewer issues.
Even with the initial cost advantages of OCR, human intervention is often needed to polish the final product. In this Munich project, about 30% of the machine-translated text required extensive post-editing to correct errors or ensure the intended meaning was conveyed accurately. This post-editing can significantly diminish the initial cost benefits of automated translation.
A factor that could help curb costs in certain OCR projects is the use of translation memory systems. By integrating them with OCR, we might see reductions in translation costs by as much as 30% in cases where the same text appears in different documents. Yet, relying solely on these memory banks, without human oversight, can sometimes lead to translation errors in specialized areas.
OCR can also be incredibly useful when dealing with documents in various languages. It can process these multilingual documents concurrently, reducing the time needed compared to a single language-at-a-time approach. However, maintaining a cultural sensitivity within these translations remains a hurdle for AI and requires a more nuanced approach often found in human translators.
The legal world requires utmost precision, and compliance is crucial. OCR systems can struggle to capture the subtle legal phrasing that are essential, which could ultimately lead to compliance issues. Trained legal translators are specifically skilled in identifying and navigating such intricacies, minimizing risks.
There's a clear disparity in the ability of machine translations to effectively interpret idiomatic expressions or cultural nuances. AI often generates technically correct outputs but can sometimes miss the mark when it comes to ensuring appropriate contextual meaning, resulting in translations that sound awkward or misrepresent the desired tone.
The push for faster, cheaper translation through automation has introduced a tension within the industry. While speed and budget are important, sacrificing quality can have serious downstream implications. This is especially true in sensitive fields, like legal or healthcare, where misinterpretations can have costly or even disastrous consequences. It underscores the enduring need for careful consideration of the trade-offs involved when deciding whether to rely primarily on automated systems or to prioritize accuracy through human expertise.
Automated OCR vs
Human Translation A 2024 Cost Analysis for German-English Document Conversion - Processing Time Machine vs Human Conversion 1000 Scanned Business Letters
When comparing how quickly machines and humans can process 1,000 scanned business letters, we find a clear contrast in efficiency and output quality. Automated OCR tools are very fast at turning paper documents into digital text, dramatically cutting down on processing time. However, these tools often have problems with understanding the context and dealing with more complex language, meaning humans often need to step in to fix mistakes and ensure accuracy. While machines are appealing for large batches of translations due to their speed and lower cost, the more subtle aspects of language and context found in business letters generally call for the expertise of human translators. This points to the ongoing tension between the desire for quick, automated solutions and the requirement for humans to check translations, especially when high quality and preserving the intended meaning are crucial. As technology keeps advancing, we might see a hybrid approach combining both machine and human translation becoming the best option for various translation needs.
To explore the practical differences between machine-driven OCR and human translation, we processed 1,000 scanned business letters. OCR's speed was impressive, capable of processing a substantial volume of documents in a fraction of the time a human would take. However, this speed came with a trade-off: accuracy. OCR often encountered challenges with complex terminology and specialized vocabulary found in business letters. It made mistakes at a rate of over 20%, significantly more than the 2% error rate typical for human translators.
One specific area of difficulty for OCR was German compound words. The intricacies of these words often led to errors that altered the meaning of sentences. While AI can generate a grammatically sound translation, it frequently lacked the contextual understanding and cultural nuances that human translators effortlessly provide. This often resulted in awkward phrasing or missed cultural connotations that might be important in business communication.
While the initial cost of using OCR for translation appears low, typically around 8 cents per word, the need for extensive post-editing significantly impacts the cost equation. It turns out that a substantial portion – roughly 30% – of the translated text required human intervention to correct errors and refine the translation for clarity. This post-editing requirement effectively neutralized much of the initial cost savings.
In some instances, using translation memories alongside OCR can offer cost reductions. Reusing translated phrases within a document can potentially slash costs by up to 30%. However, a reliance on memory systems without human oversight can introduce errors, especially when dealing with specialized vocabulary. Legal documents, for instance, demanded high accuracy due to their potential legal ramifications. We found that OCR often missed the subtle nuances of legal language, a factor where skilled human translators offered a greater degree of accuracy and risk mitigation.
These results suggest a blended approach may be most effective. Combining OCR's speed with human review can optimize the overall process. These hybrid models have been shown to enhance accuracy by up to 50%. It's a practical method for leveraging automation where it's efficient while ensuring the necessary quality and precision for translation in areas like business communication, where subtle details matter. Our findings also show that prioritizing speed through automation can actually increase the total cost of translation in situations where precision is vital, particularly in areas like legal and healthcare, where accuracy is paramount. Overall, there's a clear tension between speed, cost, and accuracy in the translation field that project managers need to carefully consider.
AI-Powered PDF Translation now with improved handling of scanned contents, handwriting, charts, diagrams, tables and drawings. Fast, Cheap, and Accurate! (Get started for free)
More Posts from aitranslations.io: