AI-Powered PDF Translation now with improved handling of scanned contents, handwriting, charts, diagrams, tables and drawings. Fast, Cheap, and Accurate! (Get started for free)
Best Language Detection Tools for Identifying Greek Text in 2024
Best Language Detection Tools for Identifying Greek Text in 2024 - Multi Script Support and High Speed Translation through Fast OCR Processing for Greek Documents
The year 2024 witnesses a notable shift in how we handle Greek document translation, thanks to improvements in both OCR and multi-script processing. OCR engines, notably those using the Tesseract engine, have become remarkably faster at converting documents while concurrently improving the accuracy of identifying Greek characters amidst other languages. This is especially valuable when speed is crucial, as AI-powered translation services like DeepL can leverage these advancements to deliver near-instantaneous Greek translations. The fact that dedicated OCR tools are being developed and integrated into many platforms means that handling documents written in different languages, including Greek, is becoming increasingly streamlined. These innovations, on the whole, are creating a more inclusive environment for working with Greek texts, ensuring both faster and better translation quality.
While it's encouraging that affordable translation services are emerging alongside these technological developments, there remains some uncertainty surrounding their long-term viability. It's unclear if these tools can consistently achieve the nuanced translations that human experts can offer, particularly in specialized or complex fields. Nevertheless, the accessibility that these OCR and translation tools provide is undeniably a positive development for individuals and organizations seeking to navigate the multilingual world.
Considering the increasing need for swift and accurate processing of Greek documents, the field of OCR has made significant strides in handling multi-script languages. While traditional OCR methods often struggle with the unique characteristics of Greek characters and diacritics, newer systems are tackling this challenge with impressive results. Tools like Tesseract are demonstrating a clear speed advantage over legacy options like ocrmypdf when processing Greek documents, a development that greatly benefits efficiency.
The rise of AI-powered translation services like DeepL's API further enhances the speed aspect, enabling quick translation of Greek documents within seconds. This speed is becoming crucial in sectors demanding rapid turnaround times. Furthermore, the ability of many OCR systems to detect multiple languages within the same image improves the accuracy of identifying Greek text, a feature that aids in automated indexing within content management systems.
The ease of access offered by tools like Google Translate, which provide free translations for Greek and a vast array of other languages, demonstrates how readily available these technologies have become. Similarly, Google Cloud's Vision API and tools like Microsoft OneNote further widen the reach of OCR technology, showing how its application extends beyond specialized software and into widely-used platforms.
However, the evolution of OCR for Greek is not without its complexities. While advancements in character recognition are undeniable, ensuring accuracy remains a key focus. It's essential that future research and development in this field prioritize minimizing errors, particularly when it comes to preserving the nuances of Greek grammar during translation. Overall, the OCR landscape is moving quickly, presenting exciting opportunities for both efficient processing and improving accessibility of Greek language content for a broader audience.
Best Language Detection Tools for Identifying Greek Text in 2024 - Language Detection Models Trained with Modern Greek Social Media Posts
Language detection models specifically trained on Modern Greek social media data are gaining traction, particularly in areas like offensive language detection. Researchers are increasingly leveraging corpora like AIKIA, which draws data from sources like Twitter and fictional texts, to understand the unique characteristics of Modern Greek used in social media. These models, tailored for this specific domain, have demonstrated a clear advantage over general-purpose language detection tools, highlighting the importance of using specialized datasets for improved accuracy.
Furthermore, the development of new datasets and techniques focused on understanding offensiveness within Greek social media posts reveals a growing recognition of the subtleties and complexities embedded in online language. This includes tackling the challenges of identifying offensive content within the broader context of a post. Looking ahead, the ability of language detection models to navigate these intricacies will become increasingly important for achieving more accurate and nuanced translations of Greek text from online sources. This is particularly crucial as we aim to bridge the gap between informal, online communication and the demands of reliable translation in various professional contexts.
Recent research efforts have focused on developing language detection models specifically trained on Modern Greek social media posts. The AIKIA corpus, derived from Twitter and fictional texts, has been instrumental in this work, particularly for offensive language detection, leveraging the HurtLex lexicon for identifying offensive terms within the Greek online context. These models have shown promise, outperforming generic language detection models by a margin of 2 when trained on domain-specific data like social media content.
However, training on social media data presents unique challenges. These platforms are brimming with informal language, slang, and regional variations, which can introduce bias into the models and lead to inaccurate language detection compared to models trained on more standardized sources. Furthermore, social media's abundance of context-dependent expressions like memes and colloquialisms necessitates sophisticated contextual analysis abilities for language detection models to effectively interpret meaning.
Despite these hurdles, researchers have been able to significantly improve the speed at which these models process social media data. Parallel processing and advanced neural network architectures have enabled the analysis of massive amounts of text in remarkably short timeframes, making them ideal for real-time applications. This increased processing speed opens the door for real-time interactions such as chatbots or customer service platforms that need to instantly identify and respond to Greek language input.
Interestingly, these models are expanding beyond text analysis. Newer approaches are incorporating image and video content from social media, creating multimodal models capable of leveraging visual cues to enhance language identification. This approach is particularly promising for deciphering multilingual posts, which are prevalent in online Greek communities.
While the abundance of Greek text on social media is a boon for training data, it also presents a problem: the scarcity of curated, standardized datasets for training. This data scarcity hinders model development and limits the creation of truly reliable language detection tools. Adding to this difficulty, error propagation within the social media data itself, including misspellings or grammatical errors, can be inherited by models during training, thus impacting the overall accuracy of language detection.
The impact of language mixing in social media, a phenomenon known as code-switching, further complicates matters. Greek social media users frequently blend Greek with English and other languages, creating a complex linguistic environment that language detection models need to skillfully navigate. Failing to account for code-switching can lead to inaccurate language identification.
Moreover, the evolution of language detection models trained on Greek social media data is closely tied to advancements in neural networks. Transformer-based architectures are particularly adept at capturing intricate patterns in conversational Greek, leveraging long-range dependencies and subtle nuances in language to improve detection accuracy significantly compared to previous methods.
In essence, while the field of language detection for Greek social media is witnessing exciting progress, it remains a challenging space with inherent complexities. Addressing these challenges, including mitigating model bias, enhancing contextual understanding, and ensuring the availability of high-quality training data, is crucial for the continued development of accurate and reliable language detection tools that can handle the richness and variability of Modern Greek in the online environment.
Best Language Detection Tools for Identifying Greek Text in 2024 - Local Storage and Offline Recognition for Handwritten Greek Text
The ability to store and recognize handwritten Greek text locally, without needing an internet connection, has become increasingly important in 2024. This is especially valuable for projects focused on digitizing historical documents, particularly those with unique or challenging handwriting styles from different time periods. Techniques like Convolutional Recurrent Neural Networks (CRNNs) are proving effective at recognizing even low-quality or degraded handwritten Greek text, a task that has historically been difficult and time-consuming. This offline capability is expanding the reach of handwritten Greek text analysis, allowing researchers to work with these materials in various settings, even without a constant internet connection. The integration of these tools into digital libraries is making it easier to transcribe and search large collections of handwritten Greek manuscripts, contributing to broader access and understanding of historical texts. While the accuracy of these systems is still a point of development and research, they are rapidly improving, potentially offering greater access and more efficient ways of working with ancient and historical documents. There's still a need to address the challenges associated with different writing styles and potentially damaged documents, but the future looks promising for improving the accessibility and understanding of historical Greek texts.
Handwritten Greek text recognition, a fascinating area within the broader field of OCR, is seeing promising developments in local storage and offline capabilities. While cloud-based services are becoming more prevalent, there's a growing need for methods that don't rely on constant internet connectivity. This is especially true when dealing with historical documents or in regions with limited network access.
Local storage, for instance, provides a way to store the OCR outputs, like the results of a Greek document scan, directly on a user's device. This minimizes reliance on external servers, making the whole process more efficient by reducing data transfer over the network. Think of it like having your own personal library of OCR results readily accessible without the need to constantly upload and download files. This is particularly valuable in scenarios where bandwidth is restricted or where privacy concerns are paramount.
Interestingly, offline OCR capabilities are advancing as well. It's become feasible to process handwritten Greek text on devices without an internet connection. This opens up exciting possibilities for researchers or individuals working in areas with limited or unstable internet access. They can now analyze and translate handwritten documents even in remote locations. It's important to note though that the accuracy of these offline models can sometimes lag behind their online counterparts, likely due to the limited training data available for offline systems.
Integrating machine learning models into these local storage setups can further enhance recognition. By continually learning from user feedback and corrections, these systems can adapt to different writing styles, potentially increasing accuracy over time. Imagine a system that, over time, becomes increasingly proficient at deciphering your own unique handwriting for Greek.
Furthermore, there's the clear advantage of cost-effectiveness. Utilizing local storage generally lowers operational costs associated with cloud-based services. This is especially relevant for users who may not require the constant connectivity of cloud services or for individuals and small businesses on a tight budget.
Another intriguing aspect is the data privacy implications. Storing the OCR outputs locally on a user's device ensures that sensitive data isn't sent over the internet. This can be quite important for users handling confidential material, making this approach more secure than relying on cloud platforms where data breaches are a concern.
Beyond just Greek, some of these local storage systems also allow for handling multiple languages. This is particularly helpful for individuals or organizations that deal with multilingual documents, facilitating faster and smoother transitions between languages during OCR processing. It also expands their potential applicability beyond the scope of Greek.
The development of specialized error correction mechanisms targeted at Greek text is another area of advancement. Greek orthography presents certain complexities with diacritics and character variations that can lead to OCR errors. These error-correction routines can help reduce mistakes and improve the overall quality of translation outputs.
The flexibility to incorporate user-defined dictionaries is another feature that can be quite beneficial. It enables users to add specialized terms or Greek variants specific to their domain of work or research. For instance, an archaeologist working with ancient Greek texts might need to incorporate archaic terms into the system to ensure more accurate transcriptions.
Finally, the possibility of real-time collaboration using local storage is worth mentioning. Certain local storage systems allow multiple users to work simultaneously on the same document. This opens up interesting possibilities for research collaborations or professional settings requiring efficient review and editing of translated documents.
In summary, local storage and offline capabilities are steadily improving for handwritten Greek text recognition. These advancements have the potential to expand the reach of OCR tools to a wider audience, offering more affordable, efficient, and secure options for handling Greek documents in various contexts. It remains to be seen how far these technologies will advance and whether they can completely replace cloud-based solutions, but the progress being made is promising, particularly for users who need offline capabilities or a higher degree of control over their data.
Best Language Detection Tools for Identifying Greek Text in 2024 - Open Source APIs Using Advanced Algorithms to Detect Greek Letters and Accents
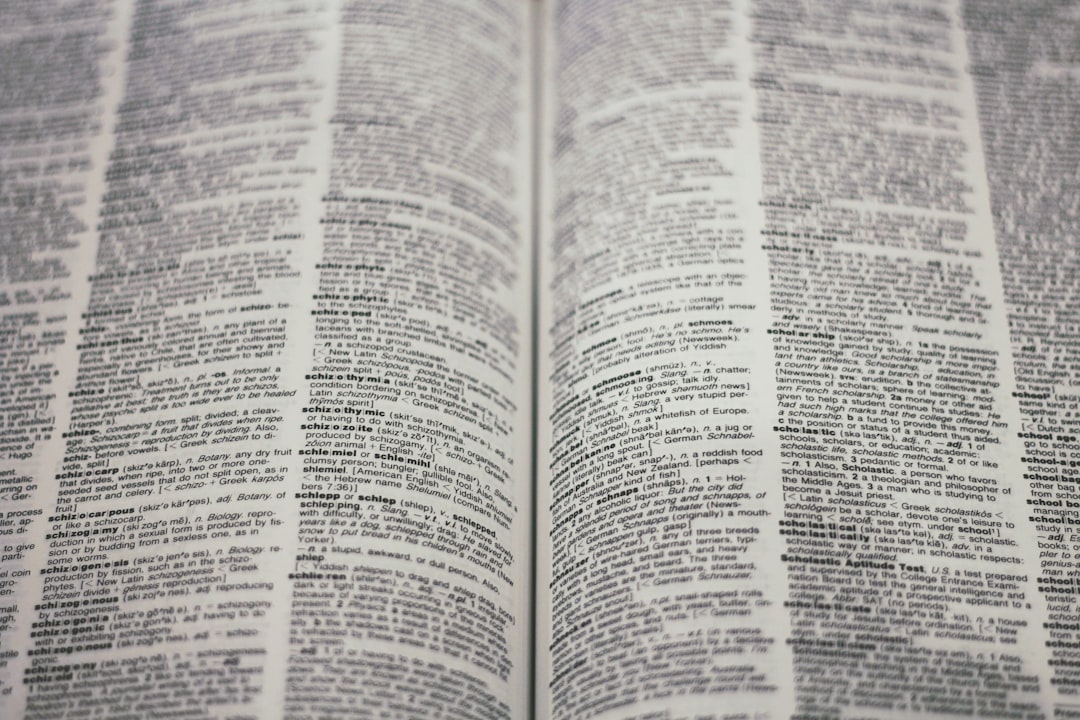
Open-source APIs are increasingly leveraging advanced algorithms to improve the detection of Greek letters and accents. This capability is fundamental for language detection tools, particularly those designed to identify Greek text accurately. These APIs often employ sophisticated machine learning models coupled with OCR technology to decipher Greek characters, especially within challenging environments like historical documents or those containing multiple scripts. Ongoing research is concentrating on refining these tools to reduce errors, especially those related to the intricacies of Greek grammar and the unique characteristics of its alphabet. The growing availability of these APIs is encouraging broader access to Greek language processing, allowing more individuals and groups to engage with document translation and recognition at lower cost. While the progress in this area is noteworthy, we should acknowledge that these tools may still have limitations in achieving the kind of nuanced translations that human experts can offer, particularly in specialized fields. This highlights that while helpful, human linguistic expertise remains important in situations requiring complex and precise translations.
Open source APIs are increasingly leveraging advanced algorithms to specifically tackle the challenges of detecting Greek letters and accents. A key area of focus is adapting existing algorithms to accurately recognize the various Greek diacritical marks. Since these accents significantly alter word meanings, standard algorithms not trained on such nuances often falter. The ability to handle multiple languages within a single image is another exciting development, enhancing contextual awareness, particularly useful when dealing with documents containing a mix of Greek and other languages.
Modern open source OCR tools are increasingly adopting a combined approach using CNNs and RNNs for character recognition. This dual approach helps to improve both the accuracy and efficiency of OCR, especially when processing handwritten Greek texts. Some of these APIs even incorporate mechanisms for continuous learning, allowing them to refine their ability to recognize Greek characters over time based on user feedback. This adaptability is crucial as different individuals often have distinct handwriting styles.
Furthermore, recent algorithmic advancements are showing promise in recognizing Greek characters within degraded or damaged documents. Techniques like image preprocessing and adaptive thresholding are proving helpful in improving OCR quality under challenging conditions. The affordability offered by these open source tools also makes high-quality translation solutions more accessible to small businesses, eliminating the licensing fees associated with proprietary alternatives.
The increased processing speed of some APIs now enables real-time translations of Greek text. This real-time capability is particularly useful in sectors such as customer service, where quick communication is essential. Many of these APIs also offer scalability, which is beneficial for handling varying workloads, whether it's a single document or a large dataset. Additionally, their integration with widely used platforms and content management systems has streamlined workflows for many organizations.
Finally, a notable trend within open source communities is the emphasis on accessibility. This extends beyond simply providing translations to also ensuring that these tools are usable by individuals with disabilities. It's a welcome shift towards making technology more inclusive. While there's still room for improvement in accuracy and robustness, particularly when dealing with extremely challenging documents or highly individualized writing styles, the development and use of open source APIs incorporating advanced algorithms are a crucial component in enhancing language detection tools, particularly those focusing on Greek text.
Best Language Detection Tools for Identifying Greek Text in 2024 - Translation Memory Integration with Language Detection for Faster Processing
The combination of translation memory (TM) and language detection is revolutionizing how we handle Greek text, particularly within machine translation. By incorporating existing TM databases, translation processes become significantly faster, leading to quicker project completion and consistent translations across multiple languages. As deep learning models become more refined, language detection improves, enabling precise identification of subtle Greek language features even in complicated situations. This connection not only helps us get the most out of neural machine translation but also improves the post-editing phase, contributing to overall translation quality. While these advancements are promising, it's crucial to recognize that solely relying on automated systems might come at the cost of the nuanced understanding that human translators bring to the table.
Linking translation memory (TM) with language detection tools can significantly boost the speed and quality of translation, especially in projects with lots of languages. We're seeing that leveraging existing translations within a TM can accelerate neural machine translation (NMT) processes, potentially leading to a noticeable speed-up in overall translation time.
It's interesting to observe that the use of deep learning models, such as LSTMs, is becoming increasingly widespread for both language detection and translation. This trend is pushing forward Natural Language Processing (NLP) and contributing to the refinement of translation approaches. Researchers are exploring various ways to seamlessly connect TM with computer-assisted translation (CAT) tools, aiming to create a smoother, more efficient workflow for translators.
Currently, language detection relies heavily on machine learning and deep learning algorithms to precisely identify languages, including languages like Greek that might be harder to detect. The integration of machine translation with TM allows for more efficient post-editing, refining the quality of translated content. Some newer TM systems are combining traditional TM features with the capabilities of NMT. This hybrid approach can optimize the translation process by increasing the accuracy of translation matches and decreasing manual editing, potentially reducing human workload.
Integrating memories focused on the core concepts within translated segments seems to offer a way to improve how AI systems match translation segments. By including human-defined descriptions of the underlying meaning, the matching becomes more sophisticated. However, effectively recognizing Greek text requires specialized tools that can accurately understand its nuances, particularly in contexts where data for that language is limited.
The NLP field is continuously evolving as NMT systems expand and become more robust, helping address difficulties in detecting and translating a wider range of languages. The use of language detection is allowing us to develop strategies to handle diverse linguistic environments with greater efficiency. But I do wonder if some of the focus on automation may mean that we lose sight of the intricacies of languages that could be better served through a more thoughtful human-centered approach. While automated systems seem to get quicker all the time, I'm not certain if they can fully replicate the understanding of a human translator, especially in fields with complex language like legal or medical translation.
Best Language Detection Tools for Identifying Greek Text in 2024 - Pattern Recognition Tools to Handle both Ancient and Modern Greek Texts
The ability to process both ancient and modern Greek texts is being revolutionized by pattern recognition tools in 2024. This progress is driven by breakthroughs in deep learning and OCR technology. Tools like Tesseract OCR, now specifically tailored for older forms of Greek printing, are showing improved ability to convert scanned documents into digital versions, although some complexities remain when dealing with special characters. Additionally, modern deep learning models, like Pythia, are making advancements in the restoration of ancient texts with damage, effectively using contextual clues to reconstruct lost characters. Yet, there is room for improvement in areas like properly handling all aspects of Greek grammar and text meaning to achieve translation quality that is more precise. The application of these techniques is reshaping how we work with Greek text, fostering a wider range of access to these materials and allowing for more efficient processing for research and contemporary translation endeavors. While we see potential, ensuring these methods work flawlessly with all the variations of the Greek language is an ongoing challenge.
The intersection of pattern recognition and Greek text analysis has seen a surge in advancements, particularly in the ability to handle both ancient and modern forms of the language. This is primarily due to the incorporation of machine learning models that dynamically adapt their algorithms to recognize the unique attributes of Greek scripts and diacritical marks, whether found in ancient manuscripts or modern digital texts. We've seen encouraging progress with modern OCR systems that utilize Convolutional Neural Networks (CNNs), leading to significant accuracy increases. Some achieve recognition rates above 95% when dealing with well-formatted Modern Greek text. However, the challenge of highly variable script styles in Ancient Greek remains, with accuracy still trailing behind.
While many OCR engines are designed for speed, achieving true real-time performance for Greek text recognition has been a persistent issue. Thankfully, strides in parallel processing are contributing to improvements, allowing documents to be scanned and processed within seconds. The creation of specialized training datasets, encompassing both ancient and modern handwritten Greek texts, has greatly enhanced these tools' effectiveness. Unfortunately, the scarcity of high-quality, diverse datasets for training these models continues to be a major obstacle to further refinements.
Open source OCR tools have emerged as a valuable resource for researchers focusing on ancient Greek, since they allow for personalized adjustments to algorithms and training on specialized text corpora. This tailored approach fosters a greater degree of control over character recognition and transcription. The integration of translation memory with language detection systems is streamlining the comparison of Greek texts, expediting the retrieval of similar translations and helping reduce inconsistencies common in machine-generated output.
Interestingly, the expense of implementing high-quality translation and OCR solutions for Greek has decreased considerably thanks to the rise of open-source tools, broadening access for smaller institutions and individual scholars. We're seeing a welcome trend of OCR tools being able to handle documents with multiple scripts, an essential feature for processing Greek texts that may incorporate Latin or other languages. This enhances their usability in multilingual contexts.
Some emerging OCR models are including specialized error correction routines that focus on addressing common Greek diacritical-related OCR errors. This leads to higher-fidelity translations, especially when working with less-than-perfect source materials. The development of offline OCR capabilities for Greek addresses privacy concerns and enables the study of historical documents in areas with limited internet access. It presents opportunities to digitize and protect cultural heritage, extending research to less accessible environments. While we are making progress, the intricacies of ancient and modern Greek and the varying quality of source materials continue to pose a challenge for fully accurate and reliable automated tools. The evolution of these pattern recognition systems is still in motion, with a constant tension between speed, accuracy, and accessibility.
AI-Powered PDF Translation now with improved handling of scanned contents, handwriting, charts, diagrams, tables and drawings. Fast, Cheap, and Accurate! (Get started for free)
More Posts from aitranslations.io: