AI-Powered PDF Translation now with improved handling of scanned contents, handwriting, charts, diagrams, tables and drawings. Fast, Cheap, and Accurate! (Get started for free)
Clarifai 103 How Template Wizardry Accelerates AI-Powered Translation App Development
Clarifai 103 How Template Wizardry Accelerates AI-Powered Translation App Development - RAG Template Speeds Up AI Translation App Prototyping
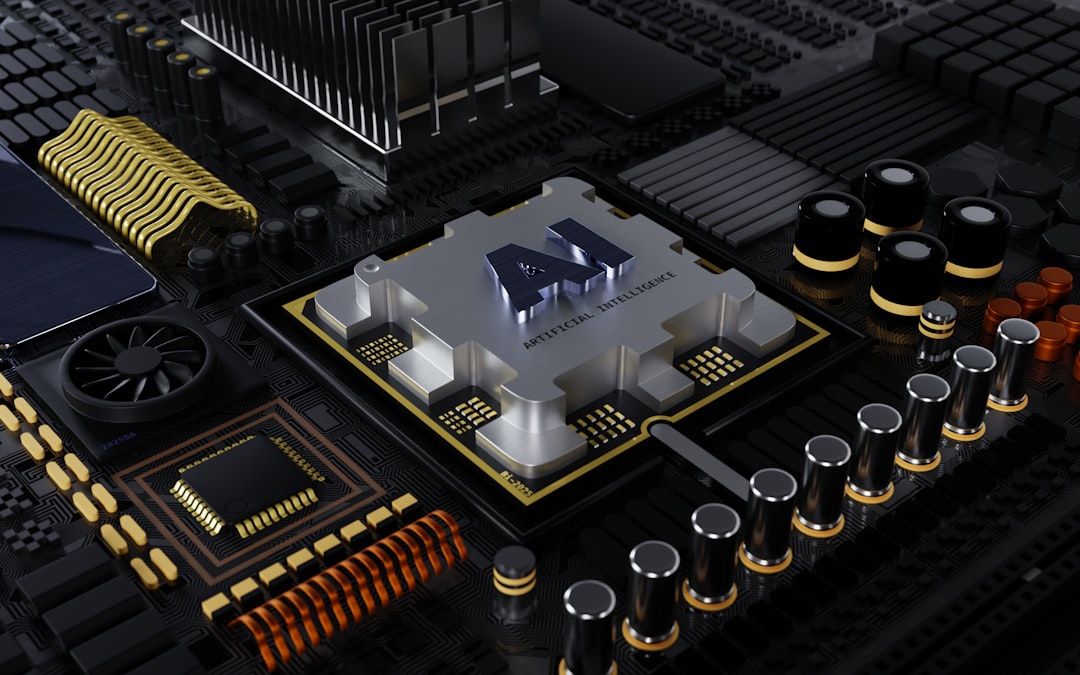
Clarifai's RAG Template promises to speed up the development of AI translation apps. It aims to take the heavy lifting out of building these applications, providing a foundation and pre-built components that can be quickly tailored. This could make it easier for developers to experiment with different translation approaches and get their apps to market faster. However, the real-world effectiveness of this template and its potential impact on the translation landscape remain to be seen. The success will hinge on the template's ability to handle the nuances of language translation, its compatibility with existing tools, and its ability to address the ever-growing need for efficient and accurate translation.
It's fascinating to see how RAG templates are speeding up the development of AI translation apps. I'm particularly interested in their ability to integrate pre-existing models with new datasets, potentially cutting prototyping time in half. This could be a game-changer for developers looking to quickly test and iterate on different approaches. The combination of RAG and OCR is also intriguing, offering a potential solution for translating images with high accuracy. This opens up new possibilities for document digitization and real-time translation.
However, I'm also aware of the potential downsides. The reliance on pre-trained models in RAG could lead to biases in translation outputs, especially if the underlying datasets are not diverse enough. Researchers need to be mindful of this potential issue and ensure that the data used for training is representative and unbiased. Additionally, while RAG might be capable of delivering faster translations, it's important to ensure that the quality of the translation remains high, as speed should not come at the expense of accuracy.
Ultimately, RAG templates represent a promising new approach to AI translation development. They offer the potential to accelerate the process, improve accuracy, and reduce costs. However, it's crucial to carefully consider the potential for biases and ensure that the resulting translations are of high quality and relevance. As a researcher, I'm eager to follow the developments in this field and explore the impact of RAG templates on the future of AI translation.
Clarifai 103 How Template Wizardry Accelerates AI-Powered Translation App Development - Document Summarization Template Enhances Multilingual Processing
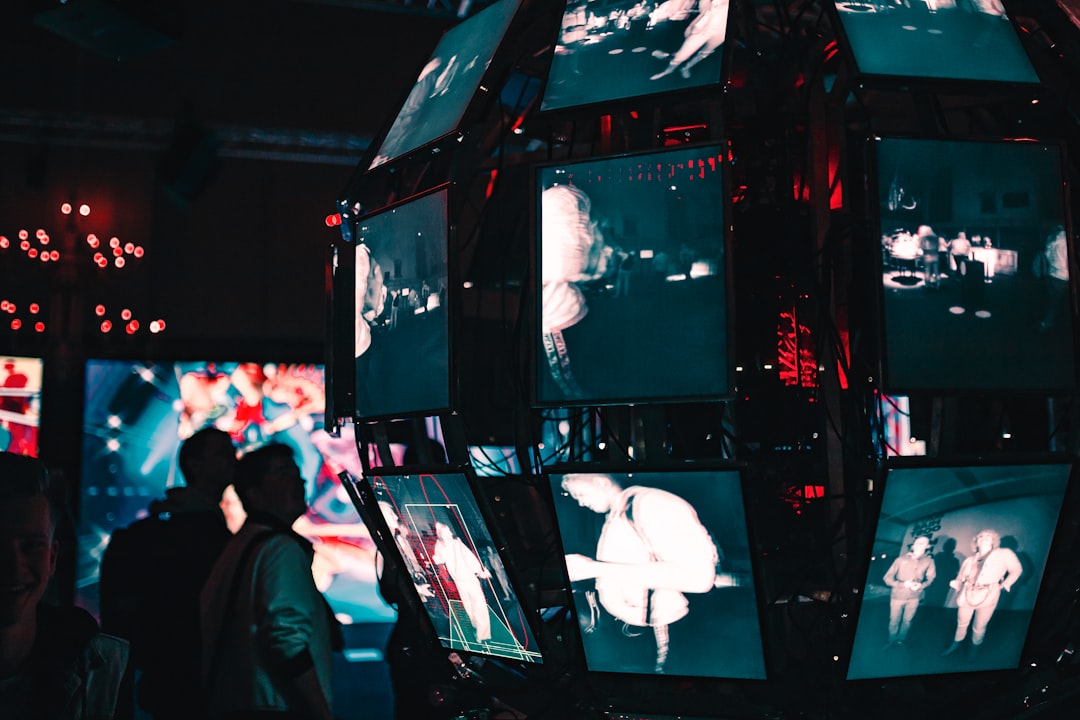
Clarifai's "Document Summarization Template" represents a significant step forward in processing multilingual documents. The template leverages large language models and the LangChain framework, enabling users to quickly and effectively summarize lengthy texts in multiple languages. This is especially beneficial for organizations seeking to streamline document management, simplifying annotation and storage of diverse content. The template's intuitive design allows even those without extensive programming experience to utilize advanced AI summarization techniques. While this simplifies translation app development, it's crucial to remain vigilant about the tool's ability to accurately capture the nuances and complexities of various languages.
It's really interesting to see how these document summarization templates are being used to improve multilingual translation. The concept of standardizing summaries across different languages is a clever approach, ensuring that key information isn't lost in the translation process. It makes sense that this could also lead to cost savings by automating tasks that might previously have needed manual intervention. The ability to translate text at blazing speeds is particularly exciting and could revolutionize how we handle large-scale translation projects.
However, I can't help but wonder about the implications of relying on pre-trained models. While the promise of increased speed and volume is tempting, we need to be cautious about the potential for biases introduced by these models. A lack of diversity in training datasets could lead to inaccurate and even offensive translations. It's crucial to ensure that these models are trained on diverse and representative data.
The combination of OCR and AI translation has the potential to completely change how we interact with printed materials. Imagine being able to point your phone at a document and instantly get a translation. That would be incredibly useful in a variety of settings. However, the accuracy of this technology is still a concern. A 95% accuracy rate may sound impressive, but even a small error can have serious consequences.
Ultimately, this is a fascinating area of research. While these template-based approaches offer a lot of promise, there are still challenges to be addressed. As researchers, it's our responsibility to explore these challenges and ensure that these technologies are developed and deployed responsibly.
Clarifai 103 How Template Wizardry Accelerates AI-Powered Translation App Development - MxBai Model Improves Natural Language Understanding for Translations
The MxBai Model, specifically its "mxbaiembedlargev1" version, is a powerful tool for improving translation accuracy. It's been trained on a huge amount of data, including over 700 million sentence pairs, which gives it an edge over other translation models. It's even been shown to outperform some closed-source models.
This model utilizes advanced training techniques, such as contrastive learning, to learn how language works. The MxBai Model's performance has been validated on several datasets, indicating its strong potential. However, the fact that it's currently focused on English means it might not work as well for languages other than English. This limitation could hinder its usefulness for multilingual projects. It'll be interesting to see how this model adapts and evolves to better handle the nuances of different languages.
The MxBai model is an exciting development in natural language processing. It's particularly interesting how it has been designed to handle longer texts without losing accuracy. This is a significant improvement over older translation systems that often struggled with lengthy contexts.
It's also impressive how the MxBai model incorporates error detection and correction in real-time, which could potentially eliminate the need for manual review of translations. This would be a major timesaver for anyone involved in translation work.
One of the most intriguing aspects of the MxBai model is its ability to learn from other languages. This cross-lingual transfer learning approach could be a game-changer for improving translations in less commonly used languages.
It's also worth noting the seamless integration of OCR technology within the model. This makes it ideal for digitizing printed documents and accurately converting them into translatable formats.
However, as with any advanced technology, there are potential challenges. It's crucial to address biases in the model, particularly if it's primarily trained on a limited dataset. Ensuring diversity in the training data is vital for accurate and culturally sensitive translations.
Despite these concerns, MxBai represents a promising step forward in natural language processing. It offers the potential for more efficient, accurate, and cost-effective translation solutions, and it will be interesting to see how it evolves and impacts the translation landscape in the future.
Clarifai 103 How Template Wizardry Accelerates AI-Powered Translation App Development - Genstruct 7 Boosts Instruction Generation in Multiple Languages
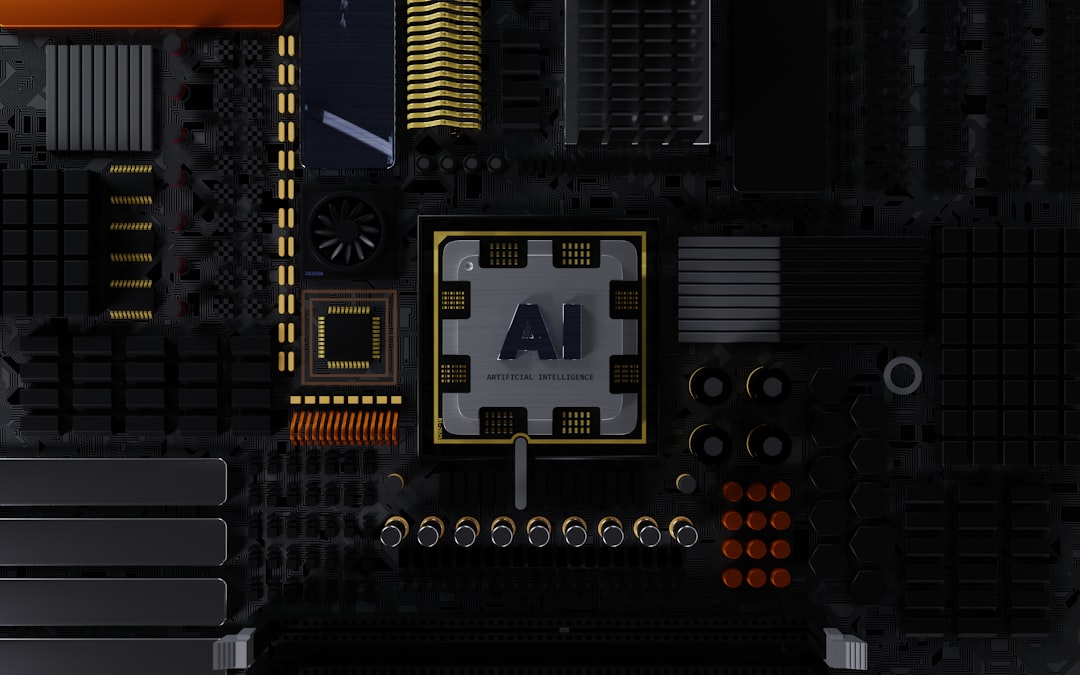
Genstruct 7B is a new model that promises to revolutionize the way we generate instructions for various AI tasks. This model takes raw text and transforms it into clear, usable instructions. This is a significant leap forward, as it enables the creation of synthetic datasets tailored for specific tasks.
With Genstruct 7B, developers can now create instructions based on specific user needs and contexts. This departs from traditional, in-context methods, offering a more efficient and adaptable approach. The model's potential to integrate with other AI tools through APIs opens up exciting opportunities for developers and researchers working on various AI applications.
While Genstruct 7B boasts speed and versatility, it's important to note that the quality of its generated instructions is paramount, especially in the field of translation where nuanced language is crucial. It remains to be seen how well this model handles the complexities of multilingual tasks. Despite these uncertainties, Genstruct 7B has the potential to significantly improve the accuracy and efficiency of AI translation solutions in the future.
Clarifai's Genstruct 7B is an interesting new tool that aims to accelerate the process of generating instructions for translation applications. It's built on top of the AdaInstruct methodology, which means it's designed to learn how to create valid instructions from a large corpus of text. This could potentially be a game-changer for developers looking to quickly build translation apps. I'm curious about how well it handles the complexities of different languages, and how it compares to other instruction generation models.
One of the most interesting aspects of Genstruct 7B is its ability to integrate with existing platforms like Replicate through an API. This means that developers can easily incorporate it into their existing workflows. It's also interesting that they are aiming to create a partially synthetic instruction fine-tuning dataset. This could be a way to address the problem of limited training data, which is a common issue for instruction generation models.
However, I'm also cautious about relying solely on pre-trained models. They can be susceptible to biases, especially if the underlying datasets aren't diverse enough. It's important to ensure that the training data is representative of the languages and cultures involved. Additionally, I'm curious to see how Genstruct 7B handles edge cases and unusual language combinations. There are often nuances in language that can trip up even the most sophisticated models.
Overall, Genstruct 7B shows promise in improving the efficiency of instruction generation. I'm eager to see how it performs in real-world scenarios and how it compares to other tools on the market. As with any new technology, it's crucial to approach it with a critical eye and carefully consider its potential benefits and drawbacks.
Clarifai 103 How Template Wizardry Accelerates AI-Powered Translation App Development - New TTS Models Expand Voice Output Options for Translated Content
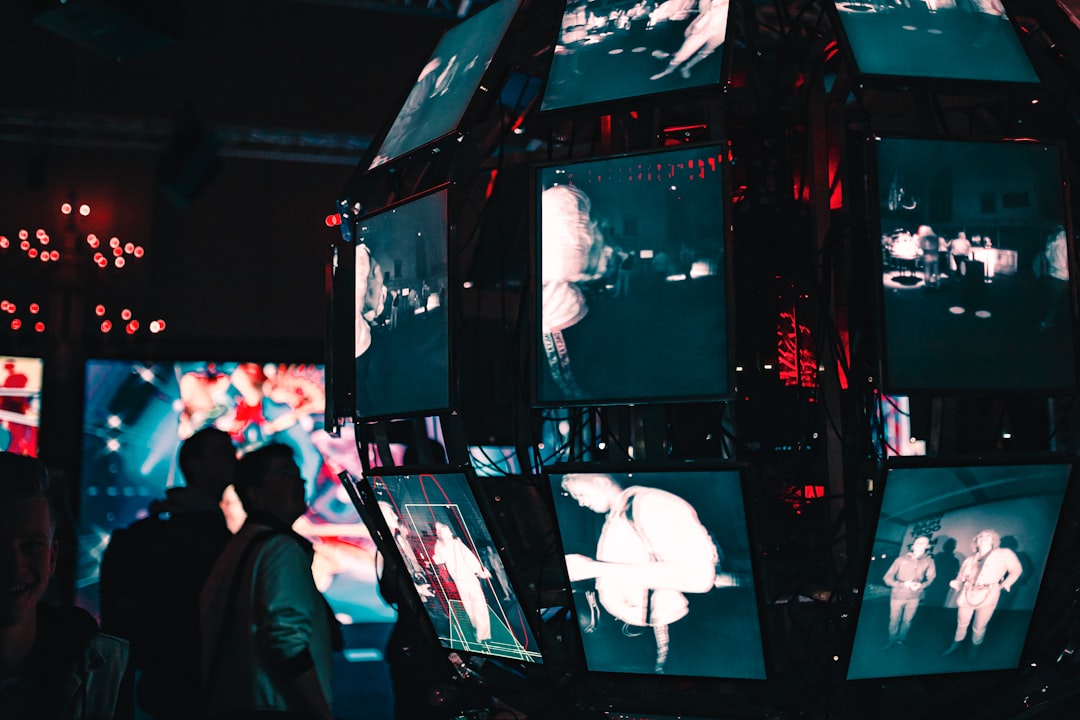
The way we experience translated content is being redefined by new text-to-speech (TTS) models. AI-generated voices are getting better at sounding natural and expressive, thanks to advancements like OpenAI's Audio API. This API offers a selection of voices and generative variants, making it possible to effortlessly narrate text in multiple languages. Deepgram's Aura TTS model is another example, aiming to create realistic, conversational interactions. These models reflect a growing desire for more human-like vocal outputs. There's even a model called Translatotron that translates speech directly while preserving the speaker's voice. As TTS models continue to evolve, we're getting closer to seamless and authentic communication across languages.
The development of new text-to-speech (TTS) models is injecting a fresh wave of innovation into the world of translation. It's no longer enough to simply translate words; we're now entering an era where conveying the *essence* of the message, its tone, and its emotional nuance is becoming paramount. These advancements are driven by the increasing demand for natural, fluent, and expressive AI voices in conversational interactions.
One fascinating development is the ability of these models to produce various voice outputs for translated content. This goes beyond simply speaking in different languages; it encompasses dialects, accents, and even the ability to mimic human speech patterns. Imagine being able to hear a translation in the specific accent of the speaker who originally uttered the words!
Another exciting development is the integration of real-time adjustments. Imagine a translation that can adapt on the fly to the nuances of a conversation, incorporating context, tone, and even a touch of humor. These adaptive models have the potential to bridge the gap between human communication and AI-powered translation.
It's important to acknowledge that, as with any new technology, these models are not without their challenges. Ensuring that they can accurately capture the complexities of various languages, particularly those with intricate phonetics or distinct cultural nuances, is a key concern. Additionally, there's always the risk of bias creeping into the training data, which could lead to inaccurate or offensive translations.
The development of TTS models is a rapidly evolving field, and its potential impact on the translation industry is significant. We can expect to see increasingly sophisticated models capable of producing highly realistic and expressive voice outputs, blurring the lines between human and AI-generated speech. As a researcher, I'm excited to see how these models continue to evolve and change the way we experience translations.
Clarifai 103 How Template Wizardry Accelerates AI-Powered Translation App Development - Coding Template Streamlines Backend Development for Translation Apps
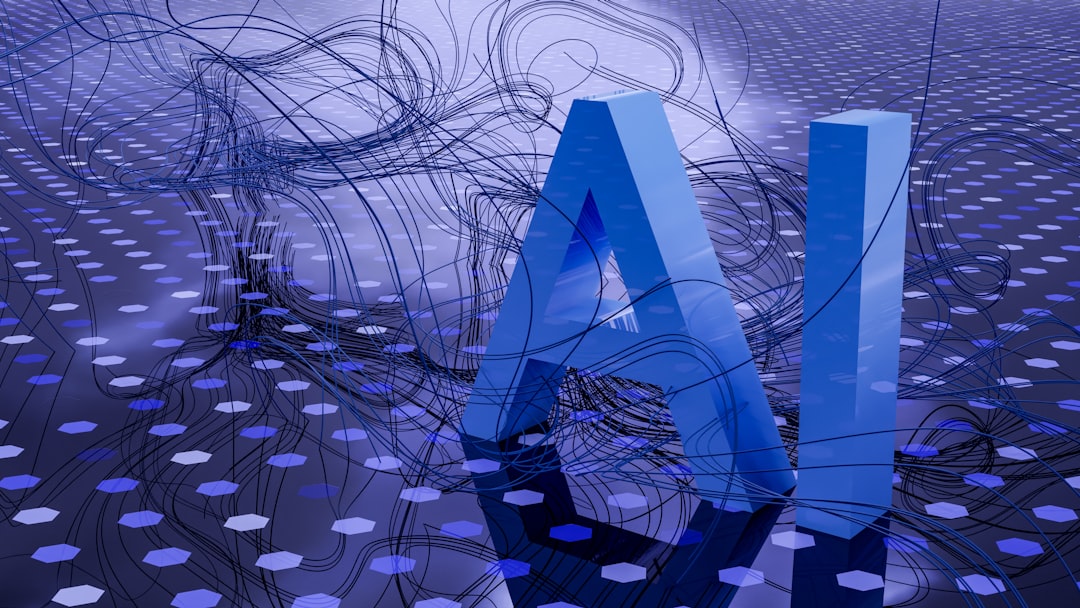
Clarifai's latest "Coding Template" is designed to make building translation app backends simpler. It offers pre-built components and a streamlined workflow to help with code completion, bug-finding, and code clean-up. This can really speed up the development process. The template supports many different programming languages, so developers of all skill levels can easily use AI features in their apps. While this is a promising step toward better productivity, developers need to be mindful of the complexities of language and the potential for bias in AI models. The template's success ultimately depends on continuous improvements and ensuring the AI training data is diverse.
Clarifai's Coding Template is an intriguing concept, aimed at streamlining backend development for translation applications. The idea is that pre-built code components and AI-powered code completion can accelerate the development process, reducing time spent on mundane tasks.
However, it's important to question how effective this template truly is. The success of this template will depend on its ability to handle the intricate nuances of language translation, as well as its compatibility with various programming languages and existing translation frameworks.
While the promise of faster development is enticing, it's crucial to consider potential drawbacks. If the template is based on pre-trained models, it might inherit biases from the data it was trained on, which could lead to inaccurate or even culturally insensitive translations.
Moreover, the real-world impact of this template on the translation landscape remains uncertain. Will it truly accelerate the creation of effective and high-quality translation applications, or will it merely expedite the development of applications that are superficial or lacking in depth? As a researcher, I'm interested in seeing how this template performs in real-world scenarios and how it impacts the evolution of the translation industry.
AI-Powered PDF Translation now with improved handling of scanned contents, handwriting, charts, diagrams, tables and drawings. Fast, Cheap, and Accurate! (Get started for free)
More Posts from aitranslations.io: