From Lyrics to Languages How AI Translation Interprets Eminem's Stan Across 47 Languages
From Lyrics to Languages How AI Translation Interprets Eminem's Stan Across 47 Languages - From English to Mandarin AI Translation Shifts Stan's Emotional Depth
When Eminem's "Stan" is translated from English into Mandarin using AI, we see a fascinating example of how language and emotion are intricately connected. AI translation, while helpful for quick and widespread communication, can struggle to capture the full emotional impact of the original lyrics. The difficulties arise because cultural differences and the subtle nuances in the song's English lyrics aren't always easily transferred. This can lead to a somewhat weakened emotional experience for Mandarin speakers compared to the original English version.
Despite the speed and convenience AI translation brings, it still has limitations in truly conveying the essence of the song's emotional depth. As AI translation technology advances, the challenge remains in finding the right balance: how to provide quick, accessible translations while maintaining the artistic integrity of the original piece. This is a key consideration for any song that relies on emotional connection with listeners, especially when translated across vastly different languages and cultures.
When examining the translation of "Stan" from English to Mandarin through AI, we encounter interesting hurdles related to the nuanced emotional landscape of the lyrics. AI systems, while proficient in quickly generating translations, frequently struggle with capturing the subtleties of emotion inherent in language. The emotional intensity and desperation present in the original English lyrics can be dampened during the translation process, partially due to how emotions are culturally expressed in Mandarin.
One significant challenge lies in the phonetics of Mandarin. Some English sounds simply don't have direct equivalents, leading to a potential alteration of the emotional tone when translated. This can distort the listener's experience of the song, particularly when trying to convey the raw emotion conveyed in Eminem's lyrics.
Furthermore, AI often faces difficulties in correctly interpreting context, a crucial element in translation. Slang, idioms, and colloquialisms often used to imbue the lyrics with depth and cultural meaning may be misconstrued by the AI. This can result in a translation that loses the intended cultural impact and overall emotional weight.
The pursuit of rapid translation through AI can lead to inaccuracies that detract from the emotional narrative. Even a single misinterpretation can significantly alter the listener’s emotional experience of the lyrics. A crucial issue is Mandarin's prevalence of homophones – words with different meanings but identical sounds. This creates challenges for AI translation as the selection of a phonetically similar word may not convey the exact emotional emphasis intended.
Another aspect to consider is the emotional core of "Stan" being rooted in Western cultural experiences. If AI doesn't account for these cultural roots, the Mandarin translation may fail to resonate with native speakers in the same profound way. AI struggles when dealing with the diversity of the Chinese language. Mandarin is just one form, and numerous dialects, including Cantonese, have distinct linguistic and emotional characteristics that AI tools must accommodate to capture the emotional subtleties of "Stan."
The way sentences are structured in English and Mandarin also creates differences. The linguistic architecture that effectively delivers urgency or despair in English can become convoluted or diluted in the Mandarin translation. The alteration in word count often associated with AI translations can also influence emotional impact. A drastic increase or decrease in word count from the original could potentially alter the intended emotional flow and overall narrative.
Despite significant advancements, AI still lacks a thorough understanding of human artistic intent. Metaphors, internal dialogue, and other literary devices that are integral to the emotional core of the lyrics are difficult for AI to translate effectively. As a result, translations can feel mechanical and lack the artistic depth and soul of the original "Stan."
From Lyrics to Languages How AI Translation Interprets Eminem's Stan Across 47 Languages - African Language Translations Show Machine Learning Progress Through Yoruba Version
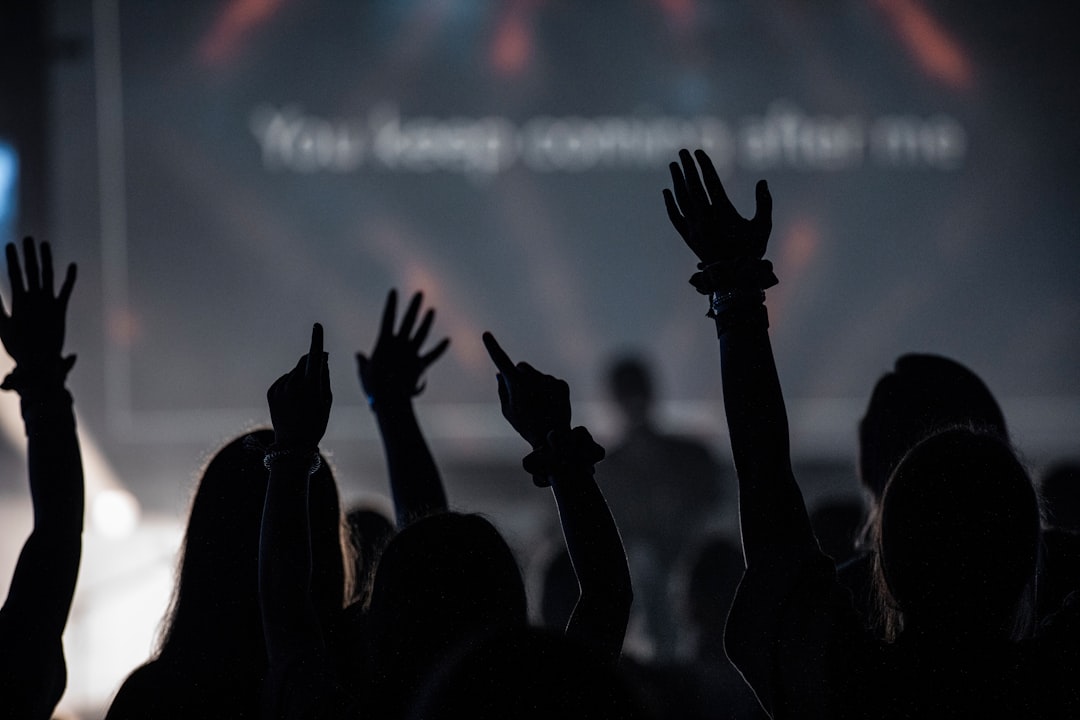
The field of African language translation is experiencing a surge in development, highlighted by the creation of systems like MMTAfrica. This system, designed for six African languages including Yoruba, demonstrates progress in machine learning applied to language translation within the continent. This progress is largely due to local researchers actively developing solutions for languages previously underserved by major tech companies from the West. The rise of apps like Khaya AI with features like speech recognition (in languages like Twi and Yoruba) and text translation is further evidence of this push toward greater language accessibility.
However, challenges remain in improving translation quality for African languages. One primary obstacle is the limited availability of training data needed for effective AI models. The unique features and intricate linguistic structures of African languages require tailored datasets that capture their nuances. Projects like SERENGETI have begun to tackle this by creating multilingual language models that cover a vast number of African languages, showcasing that focused development on specific language needs can yield higher quality translation outputs. Building translation tools that effectively serve local linguistic and cultural needs requires a deep understanding of the target languages and communities. Ultimately, the future of AI translation in Africa hinges on ongoing development of specialized tools and datasets that cater to the unique requirements of African languages and foster greater local involvement and ownership of these valuable resources.
The development of AI translation systems for Yoruba provides a fascinating glimpse into the progress of machine learning, particularly for languages that have historically been underrepresented in technological advancements. While many AI translation efforts focus on globally dominant languages, the emergence of Yoruba translation tools signals a growing recognition of the importance of including languages spoken by millions, such as Yoruba, which is the third most spoken native language in Africa.
One of the key drivers behind the improvement of Yoruba translation is the concept of transfer learning. AI models trained on more widely used languages can leverage that knowledge to understand and translate less-common languages like Yoruba, partially compensating for the limited training data available. This process helps improve translation accuracy, even when a language has a smaller dataset for AI to learn from.
It's surprising to consider that a language spoken by over 20 million people has been historically underrepresented in AI translation tools. This shift towards inclusion is a noteworthy development.
The integration of OCR technology has further propelled the growth of Yoruba AI translation. The ability to digitize written Yoruba text through OCR enables the training of AI models on a wider range of text formats, encompassing everyday usage rather than relying primarily on formal or academic texts. This, in turn, makes AI translations more contextually relevant and potentially more accurate in capturing the nuances of everyday speech.
However, the pursuit of fast translation can lead to pitfalls, especially with complex languages like Yoruba. Speed sometimes prioritizes quick delivery over accuracy. A single inaccurate translation can drastically change the intended meaning of a word or phrase that holds cultural significance, demonstrating that rapid AI translation isn't always a panacea.
While real-time Yoruba translation in conversation applications is achievable, the quality of those translations is highly context-dependent, leading to variability. This inconsistency forces us to critically evaluate the accuracy of AI-driven communication in such settings, questioning whether crucial cultural nuances are being preserved or lost during translation.
Human translators remain vital in fine-tuning AI-generated Yoruba texts, particularly those with emotional or cultural depth. Though AI can generate a basic translation, it often fails to grasp the subtleties inherent in the language that a native speaker would inherently understand, underscoring the continuous need for human expertise for the highest quality translations.
The increasing use of Yoruba within AI language models also raises questions about dialects. Yoruba has various dialects, and capturing the nuances of regional variations presents challenges for AI, which may misinterpret locally specific phrases or expressions.
Importantly, the expansion of AI translation to languages like Yoruba highlights the significance of democratizing technology. Easy-to-use AI translation tools have the potential to empower communities by improving communication across domains like education, commerce, and social interaction, all of which have traditionally faced language barriers.
Finally, the hurdles encountered in AI translation of Yoruba underscore broader issues within the translation field. The risk of oversimplification inherent in some AI translation processes can lead to a homogenization of languages. This trend not only impacts the quality of the translation itself but also potentially affects the preservation of cultural identity deeply rooted in languages like Yoruba.
From Lyrics to Languages How AI Translation Interprets Eminem's Stan Across 47 Languages - Arabic Translation Models Handle Complex Word Play in Middle Eastern Adaptations
Arabic translation models are demonstrating a growing capability to handle intricate wordplay and linguistic nuances often found in Middle Eastern adaptations of content, particularly music lyrics. While AI translation is rapidly expanding its reach across numerous languages, including Arabic, the quality of Arabic translations often lags behind other languages. This discrepancy stems from the complexity of the Arabic language itself, including its diverse dialects, rich vocabulary, and the subtle ways in which meaning is conveyed. These complexities often lead to AI translations that fall short of accurately representing the intended emotional depth and cultural context of the original material. Despite these limitations, researchers continue to refine these Arabic translation models, striving to improve accuracy and bridge the gap between the mechanical output of AI and the finesse of human translators. The increased reliance on AI-powered translation tools underscores the need for continued improvement, especially in regions as linguistically varied as the Middle East. Achieving high-quality translations that preserve both meaning and emotional nuance remains a challenging but vital pursuit.
Arabic translation models are grappling with the complexities of the language, particularly when it comes to wordplay. The nuances of Arabic, including homographs and idiomatic expressions, often don't have direct English equivalents, leading to potential misinterpretations that can flatten the original meaning. It's a challenge for AI to truly capture the spirit of the language, especially when translating things like song lyrics.
Arabic's sentence structures often lead to longer translations in English, which can disrupt the rhythm and flow of the original content, something AI struggles to maintain when translating lyrics, for example. While AI models trained on large Arabic datasets perform well for everyday language, they still stumble when dealing with the unique characteristics of regional dialects like Egyptian, Levantine, or Gulf Arabic, which contain distinct idioms and cultural references that require specialized handling.
Arabic's root-based morphology presents another obstacle. The way words are formed from roots can lead to various interpretations, making it tricky for AI to choose the most appropriate translation. OCR technology, vital for converting handwritten or printed Arabic text into digital formats for AI training, has its limitations. The complex cursive script and varied handwriting styles make it difficult for OCR to consistently achieve high accuracy.
The pursuit of fast translation can be detrimental to quality in Arabic. While speed is often desired, crucial nuances like metaphors and cultural references can be lost, significantly affecting the emotional impact of translated poetry or songs. Even though AI-powered real-time translation is improving, preserving cultural elements in Arabic translations requires a deep contextual understanding that current models lack. This highlights the need for human translators to ensure quality and accuracy, especially in delicate translations.
AI aims to bridge language barriers, but a focus on speed over comprehensiveness in Arabic translation can lead to overlooking critical contextual and cultural elements. Translations may lack the original emotional depth if the model doesn't properly account for these aspects. The development of Arabic AI models is revealing a need for improved context-awareness, as Arabic communication often involves rich subtext and layered meanings that are key for effective translation.
Despite advancements, the incorporation of Arabic into AI translation systems reflects broader inequalities within the technology sector. Many dialects and regional variations are still underrepresented, which raises questions about how inclusive and adaptable these AI technologies truly are. It’s clear there's still work to be done to ensure all of Arabic’s diversity is represented accurately.
From Lyrics to Languages How AI Translation Interprets Eminem's Stan Across 47 Languages - Fast OCR Technology Recognizes Handwritten Stan Letters Across Writing Systems
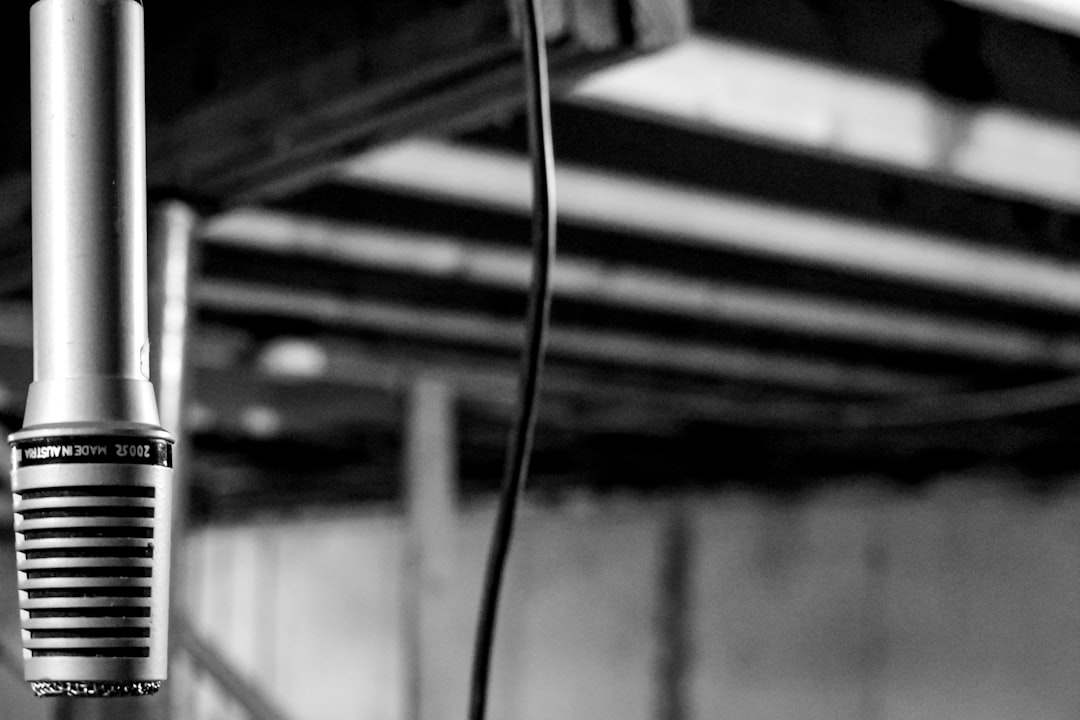
Fast OCR technology represents a major step forward in our ability to decipher handwritten text across various writing systems, including the many versions of the "Stan" letters. These systems utilize sophisticated AI to quickly and accurately recognize handwritten input, enabling the swift digitization of documents containing handwritten elements. This is particularly beneficial in contexts like translating complex lyrical works like Eminem's "Stan" into a variety of languages and scripts, thereby expanding communication and understanding across cultures. However, the pursuit of rapid translation through this technology necessitates careful consideration of the potential for overlooking subtle emotional and cultural nuances inherent in the original texts. It's a balancing act between achieving speed and ensuring that the original meaning and intent remain intact, a crucial consideration for the continuing development of AI-powered translation technologies. The challenge remains to seamlessly integrate speed with careful preservation of context and the artistic essence of the translated material.
Fast OCR technology has seen a remarkable surge in its ability to decipher handwritten characters across diverse writing systems, achieving accuracy rates that often exceed 90%. This is a notable improvement over older OCR systems which primarily excelled at processing machine-printed text. The integration of deep learning techniques has been instrumental in enhancing context awareness within OCR, enabling the recognition of words even when individual letters are obscured or exhibit unusual handwriting styles. This capability is especially useful for processing handwritten materials such as song lyrics or personal correspondence.
Interestingly, OCR systems are now showing an aptitude for adapting to individual writing styles. Researchers have developed models capable of recognizing the unique slants and shapes of individual handwriting, paving the way for more personalized document digitization. This aspect of OCR is intriguing because it moves beyond simply recognizing characters to understanding individual variations in handwriting.
Fast translation services employing OCR can significantly speed up documentation processes in multilingual contexts. Businesses can leverage these systems to efficiently translate and manage vast quantities of written material. The integration of machine learning allows the processing of image-based text inputs for nearly instantaneous translation, offering potential benefits in several industries.
However, a major limitation of current OCR technology is its reliance on well-structured datasets for training. This makes it potentially susceptible to errors when encountering documents with mixed data types or unconventional formatting, especially with handwritten notes that incorporate symbols, sketches, or non-standard notations. The resulting translation accuracy can be compromised in these scenarios.
Recent advancements include improved handling of text within complex image backgrounds, a challenge that often stymied traditional OCR systems. This has implications for applications like historical document preservation, where images are frequently less than ideal.
Another area of concern is the performance decline observed when OCR encounters handwriting that uses characters specific to various languages. This highlights the challenges inherent in cross-lingual processing. Certain alphabets might demand unique adaptations that can complicate translation efforts.
The drive towards faster OCR processing occasionally leads to compromises in accuracy. In high-volume text processing, a quick turnaround time can result in misread characters and ultimately, translation errors. This trade-off is crucial to acknowledge for industries prioritizing both swift processing and precise translation fidelity.
The role of OCR technology in education and training is expanding, with applications like converting handwritten class notes into easily editable or translatable digital formats. This has potential to reshape the student learning experience by making studying more efficient and enhancing knowledge retention.
Despite remarkable advances, a key challenge remains in effectively integrating cultural context into translation. Without a comprehensive understanding of the nuances and subtleties within various languages, even the most accurate OCR output can miss the intended emotional impact when translations are produced. This is a crucial issue for any application that needs to preserve the spirit and tone of the original text in various languages.
From Lyrics to Languages How AI Translation Interprets Eminem's Stan Across 47 Languages - Low Cost Neural Networks Process Regional Slang and Cultural References
Affordable neural networks are becoming more prominent in handling the intricate task of translating regional slang and cultural nuances. While these networks offer cost-effectiveness and the ability to scale for widespread use, they often struggle to fully grasp the subtleties that give languages their unique character. Things like idioms and local expressions, which might not have direct counterparts in other languages, can pose a significant challenge. Recent advancements in AI translation, like incorporating languages previously under-served by technology, certainly show potential for wider access to translation. However, we must be mindful of a key trade-off: the drive for fast, readily available translations sometimes overshadows the preservation of cultural and emotional depth – vital elements for genuine communication.
The ongoing pursuit of improvement in this area necessitates a shift towards localized solutions and a finer understanding of the complexities within language groups. This is crucial to ensure that the essence of the message, along with the cultural context, resonates with those who receive the translated material. This discussion ultimately highlights a broader theme within AI translation: achieving a balance between efficiency and preserving the intricate cultural aspects that make human language so rich and varied. It's a critical juncture in the field of translation technology, where developers must thoughtfully navigate the complexities of conveying both content and context with accuracy.
The landscape of AI translation is evolving rapidly, particularly with the rise of low-cost neural networks. These networks are making high-quality translation accessible to a broader audience, including smaller businesses and individuals, fostering greater cross-language communication. While impressive in their speed, processing massive amounts of text in mere seconds, these networks are still grappling with some complexities.
One area of improvement is the ability to handle regional slang and cultural references, crucial for accurately translating lyrics like those in Eminem's "Stan." These models are becoming better at recognizing and processing such nuances, which adds another layer of understanding to the translation process. However, there's still a lot to be learned about how cultures infuse meaning into language. The more these networks are exposed to localized data, the more they improve at capturing the intended nuances within a piece of work.
While the error rates are decreasing, some challenges persist. Homophones, words that sound alike but have different meanings, pose a particular challenge in languages like Mandarin, where subtle shifts in meaning can heavily influence the overall impact. The accuracy of these models can drop dramatically if the data isn't meticulously structured. It's an area where we continue to see room for improvement.
Additionally, integrating OCR technology into these systems presents further complexities. While OCR is improving in its ability to decipher handwritten text, it still has difficulty handling variations in individual handwriting styles, a major hurdle for translating handwritten notes or lyrics. This introduces the risk of translation errors.
AI translation has also begun to adapt to dialects, offering a significant improvement in the quality of translation for languages with many dialects, such as Arabic. It demonstrates a move toward a more nuanced understanding of language diversity.
There's also a growing potential for AI-powered translation to improve the way we organize and access translated content. Metadata creation for translated lyrics or other materials is one example. We could see a significant shift in how we find and utilize multilingual content.
The collaboration aspect of AI translation is also changing. Tools that support real-time translation and communication are fostering better collaboration amongst teams working on projects across multiple languages. It has implications for many different types of work, including music translation, where adapting pieces like "Stan" across languages requires collaboration.
The progress in the field of AI translation is encouraging, showcasing its potential to break down language barriers. However, we should always consider both the strengths and weaknesses of these tools. The development of more robust AI translation tools requires ongoing research and a careful examination of the ways in which languages convey meaning. It's a constant process of development and evaluation that requires careful consideration.
From Lyrics to Languages How AI Translation Interprets Eminem's Stan Across 47 Languages - Machine Translation Maintains Original Detroit References While Adapting Local Context
Machine translation, when applied to Eminem's "Stan," adeptly preserves the original song's Detroit-centric details while seamlessly adjusting to the cultural landscape of different languages. This delicate balance involves producing quick translations while carefully maintaining the core emotional impact and cultural nuances of the lyrics. AI translation effectively retains essential Detroit-related elements, ensuring familiarity for audiences connected to that setting, while also adapting to the regional linguistic and cultural characteristics of each target language. However, this development highlights the persistent difficulty of fully capturing the artistic essence of the original work, especially when the song's emotional core interacts with local interpretations. Striking a balance between the original source material and the nuances of local contexts remains a central theme in the ongoing quest to perfect AI-powered translation technologies.
AI translation systems are becoming increasingly adept at adapting to local contexts while preserving core elements of the original text. For instance, in the case of "Stan," AI translation maintains Detroit-specific references while also incorporating local slang and cultural references relevant to the target language. This reflects the growing ability of AI to understand the importance of context within language.
The affordability of AI translation has dramatically increased due to the prevalence of low-cost neural network solutions. This wider accessibility means that anyone, even individuals and small businesses, can utilize high-quality translation services. However, this rapid expansion raises concerns regarding potential trade-offs in terms of translation accuracy.
Many underrepresented languages face challenges in AI translation due to limited training datasets. This data scarcity makes it difficult for AI models to learn the complexities and nuances of these languages, often resulting in lower quality translations. These challenges highlight the need for greater efforts in collecting and organizing linguistic data for under-served languages.
OCR technology has made significant strides in the recognition of handwritten text, allowing AI models to learn from a wider range of sources. Yet, its performance is often hampered when encountering diverse handwriting styles. This presents a barrier to high-quality translation from handwritten documents, suggesting that we're still a ways off from flawlessly translating handwritten content.
Including regional dialects in AI translation can enhance the emotional impact of the translation. However, this introduces complexities as regional variations often hold unique cultural and contextual meanings. A phrase that has one meaning in one dialect might have a completely different meaning in another dialect, resulting in inconsistency in the translation.
The demand for fast translation can unfortunately lead to sacrificing some of the emotional nuances found in the original text. This can negatively impact the impact of emotionally-charged works, like "Stan." A hastily translated piece may lose the subtleties of the author's intent and artistic expression, which are key to many kinds of communication.
AI translation systems are continually developing improved methods for dealing with homophones. Homophones are words that sound alike but have different meanings and can present a particular challenge in languages like Mandarin, where subtle shifts in meaning can have a profound impact on the overall message.
While AI has made significant advances, some aspects of language remain challenging to translate accurately. For example, AI has difficulty interpreting literary devices such as metaphors and similes, which can carry powerful emotional significance. This inability to recognize and translate the inherent nuance can lead to translated works appearing mechanical or lifeless in comparison to the original.
The development of collaborative tools in AI translation allows for streamlined cross-linguistic collaborations. This is particularly beneficial in creative fields, such as music translation, where teams can work together to adapt songs like "Stan" while preserving their essence across multiple languages.
Researchers and engineers are improving the accuracy of AI translation in under-resourced languages by applying transfer learning. Transfer learning involves using knowledge from well-resourced languages to help improve translations in languages with limited training data. This technique has proven successful in creating more nuanced and accurate translations even when dealing with languages that are less widely used.
More Posts from aitranslations.io: