AI-Powered PDF Translation now with improved handling of scanned contents, handwriting, charts, diagrams, tables and drawings. Fast, Cheap, and Accurate! (Get started for free)
Google's New Attribution Model Implications for AI-Powered Translation Advertising
Google's New Attribution Model Implications for AI-Powered Translation Advertising - Data-Driven Attribution Becomes Mandatory for Google Ads Users
Google Ads has shifted its default attribution model from the traditional last-click approach to Data-Driven Attribution (DDA) for all new conversion actions, starting on September 27, 2021. This transition signifies a move towards more sophisticated measurement techniques, driven by the evolving privacy landscape. The aim is to offer advertisers a more accurate understanding of how different touchpoints influence conversions, which is particularly valuable as advertising environments become more complex. This change is not simply about adapting to new privacy regulations; it's about giving marketers more control over how they understand customer interactions. They can now allocate credit more accurately across various parts of the customer journey – something that was not possible with a last-click model.
The impact of this move is widespread. It touches a broad spectrum of ad types across Google's platforms, from search and shopping to display and YouTube advertising. Interestingly, the requirement for a minimum amount of data for DDA implementation has been removed, making it accessible to a larger number of advertisers. This is especially relevant for companies offering translation services – be it AI-powered, fast, or cheap translation solutions – as it opens opportunities to optimize campaigns in a more nuanced and informed manner. As more data becomes available, and as Google continues to expand DDA support, it is likely that advertisers will see improvements in measurement and optimization capabilities, enabling them to better connect with their target audiences and improve campaign effectiveness.
1. Google Ads has recently mandated the use of Data-Driven Attribution (DDA) as the default model for new conversion actions, effectively replacing the older last-click model. This shift, while aimed at adapting to evolving privacy considerations, also emphasizes a more holistic approach to ad performance measurement.
2. While last-click attribution only credits the final interaction before a conversion, DDA takes a more comprehensive view. It analyzes the entire customer journey, from initial exposure to the final conversion, providing a richer understanding of how different ad interactions contribute to success.
3. There's evidence suggesting DDA can lead to substantial gains in advertising efficiency. Studies have shown that businesses leveraging DDA often see a 15-20% boost in return on ad spend compared to those relying on traditional methods. This demonstrates the potential of DDA for achieving greater cost-effectiveness.
4. The move to mandatory DDA will undoubtedly push smaller businesses to adopt more sophisticated analytics tools. This transition could present challenges due to the complexity and potential cost of these tools, forcing many to re-evaluate their current marketing technology stack.
5. AI translation services can potentially gain significant advantages from the richer data provided by DDA. Understanding which specific ads, including translated ones, drive conversions empowers marketers to fine-tune messaging and language to resonate more effectively with diverse audiences across different regions.
6. Fast and accurate Optical Character Recognition (OCR) could play a more critical role in the data collection processes behind DDA. By converting paper-based customer feedback and surveys into digital data, businesses can gain deeper, more precise insights into customer preferences and behaviors.
7. The shift to DDA offers a way to more rigorously quantify the effectiveness of translation in advertising. It allows marketers to analyze how effectively localized and culturally-adapted ad copy impacts conversion rates across different target audiences, making the case for investment in translation more robust.
8. One of the less-discussed implications of DDA is its potential to pinpoint underperforming ad channels or tactics with greater precision. This gives marketers the ability to allocate resources more strategically towards successful channels, improving efficiency and effectiveness.
9. The widespread adoption of DDA will likely increase the demand for individuals skilled in interpreting complex attribution data. This suggests a potential evolution within marketing analytics, potentially creating a new specialization in analyzing cross-cultural and multilingual campaigns.
10. Ultimately, DDA's influence extends beyond simply budget allocation; it can improve the overall marketing strategy. By enabling brands to react in real-time to feedback and performance metrics, particularly across international campaigns, marketers can optimize and refine their efforts continuously, leading to more informed and agile strategies.
Google's New Attribution Model Implications for AI-Powered Translation Advertising - AI Algorithms Redefine Credit Assignment in Conversion Paths
AI algorithms are fundamentally changing the way we understand how different interactions contribute to conversions. Google's new Data-Driven Attribution (DDA) model, now the default for many campaigns, uses machine learning to assign credit for conversions in a more dynamic way than traditional methods. Instead of solely focusing on the last interaction, DDA analyzes the entire customer journey, recognizing that multiple touchpoints might influence a customer's decision. This more nuanced approach can highlight previously overlooked interactions that might have been undervalued by simpler models.
For the translation sector, whether focused on AI-powered, speed-focused, or budget-conscious solutions, this shift underscores the importance of understanding which translated ad interactions are most successful. DDA allows businesses to track the impact of their translated messaging and optimize campaigns based on data-driven insights, potentially leading to better targeting and more effective conversions across different regions. This, however, also means marketers need to adapt their thinking from singular touchpoints to understanding the entire journey – a change that may require some adjustment and new tools for analyzing the more complex data produced by DDA. It will be interesting to see how translation services adjust to this new model, and if the promised higher-level data insights actually improve their targeting and ROI.
Google's shift to Data-Driven Attribution (DDA) as the default model for new conversion actions represents a significant change in how advertising success is measured. While the older last-click model only considered the final interaction before a conversion, DDA takes a more holistic view by examining the entire customer journey, from initial exposure to the final purchase. This allows for a much more nuanced understanding of how different touchpoints contribute to conversions, something especially critical when dealing with the intricacies of language and translation in advertising.
DDA's reliance on machine learning allows it to analyze a multitude of data points, such as click patterns, time spent on ads, and even engagement with translated content. This richer data pool enables a more precise allocation of ad budgets, which is crucial for companies offering translation services, where campaigns often involve multiple languages and platforms. It's intriguing that the emphasis on DDA essentially makes localized advertising not just a best practice, but a measurable aspect of campaign success. Advertisers can now track how variations in language and messaging impact conversion rates across different regions. This opens a new avenue for optimizing translation efforts by prioritizing languages or dialects that yield the strongest results.
It's interesting to see how the increased focus on data collection and attribution can impact fields like Optical Character Recognition (OCR). As OCR technology evolves to handle diverse languages and handwriting styles, businesses can gather richer data from customer interactions like handwritten surveys or feedback forms, improving the overall quality of data available to DDA.
The ability of DDA to analyze large volumes of data in real-time enables advertisers to quickly adapt and adjust their campaigns based on the insights it provides. This rapid feedback loop is particularly valuable in the context of translation advertising, where cultural nuances and language subtleties can significantly influence campaign outcomes. By observing how various touchpoints impact user journeys, DDA can highlight areas where localized messaging or translation needs adjustments for better engagement.
Moreover, DDA encourages advertisers to track not just the final conversion but also intermediate steps that demonstrate interest, like content engagement or language selection on a website. This data can inform marketing strategies and refine the design of translated content. For instance, understanding which languages are most frequently interacted with can lead to more tailored content and campaign targeting.
As consumer journeys become increasingly complex and fragmented, DDA's ability to pinpoint underperforming ad channels or campaigns becomes especially important. This can lead to more efficient resource allocation and a potential wave of innovation in translation services. Advertisers might focus on creating more engaging and nuanced multilingual content in the channels identified as most effective by DDA.
It's possible that DDA's influence could extend beyond budget allocation to a broader impact on the user experience of online advertising. As brands refine their campaigns with the data gleaned from DDA, they'll likely place a higher priority on providing user-friendly, localized content that resonates with diverse target audiences, making the digital advertising landscape potentially more accessible and intuitive.
In the end, the insights that DDA provides could be a key differentiator for businesses. For translation services, data-driven insights can be used to craft unique value propositions that help them stand out from the competition. By leveraging the comprehensive view of the customer journey offered by DDA, these companies can position themselves more strategically within the marketplace.
Google's New Attribution Model Implications for AI-Powered Translation Advertising - Last-Click Attribution Remains an Option for Traditionalists
While Google's shift towards data-driven attribution provides a deeper understanding of how customers interact with advertisements, some advertisers, particularly those with a more traditional approach, continue to favor the simplicity of last-click attribution. The last-click model, which credits only the final interaction before a purchase, offers a straightforward view of which actions are leading to conversions. This simplicity can be appealing, especially when navigating new advertising models. However, this approach oversimplifies how people make purchase decisions, particularly in contexts like AI-powered translation advertising, where a user's journey might involve interactions across multiple languages and platforms. Focusing solely on the last click risks losing valuable insights from prior interactions, making it challenging to adapt marketing strategies effectively. The ongoing discussion between last-click attribution and more comprehensive approaches reflects the dynamic tension between familiar methods and the demands of an evolving marketing environment, particularly as AI tools are increasingly employed in ad campaigns across different languages.
While Google's new Data-Driven Attribution (DDA) model emphasizes a more holistic approach to measuring ad effectiveness, some advertisers, particularly those in sectors like translation, still favor the simpler last-click model. This preference often stems from a lack of resources or experience with complex analytical tools.
However, research suggests that relying solely on last-click might lead to a skewed understanding of customer interactions. DDA, in contrast, provides a more complete picture by considering the entire customer journey and how each touchpoint contributes to a conversion. This is particularly important in the translation field, where the full impact of translated ad copy may be missed with last-click.
The shift to DDA, however, can introduce a learning curve for many teams. Understanding how various touchpoints influence conversions requires new analytical skills and a different mindset, which some marketers might find challenging to adopt.
For AI-powered translation services, striving for speed and affordability must be balanced with DDA compatibility. Failing to align with DDA metrics can create a misleading perception of return on investment, even with the fastest translation speed.
Furthermore, implementing DDA effectively can be technically challenging for smaller companies. The required data integration might outweigh any cost benefits associated with cheaper translation solutions.
Despite these challenges, DDA offers unique advantages. The AI-powered insights derived from DDA can enhance ad relevance by tailoring messaging to diverse audiences. This is crucial for translation campaigns, where cultural nuances greatly influence the effectiveness of communication.
OCR technology also becomes a valuable tool in this new environment. By converting handwritten customer feedback across languages into digital data, OCR helps refine the input data that fuels DDA, providing deeper insights into customer interactions across different regions.
The real-time feedback from DDA offers transformative potential for translation service ad campaigns. Businesses can swiftly adapt their strategies based on language performance, which can differ significantly between markets.
This wealth of data also allows for finer-grained A/B testing. Translation services can now not only compare which languages perform best but also pinpoint which specific phrases or terminologies resonate most strongly with audiences.
Finally, the DDA transition can expose vulnerabilities in current marketing strategies. It necessitates a reconsideration of customer journey mapping and engagement tactics, especially crucial when dealing with multiple languages and cultures. This shift forces businesses to evolve their approaches to truly capitalize on the opportunities presented by this new attribution model.
Google's New Attribution Model Implications for AI-Powered Translation Advertising - Google Expands Attribution Model Across Multiple Ad Formats
Google has extended its attribution model to cover a wider range of ad formats, including YouTube and Display ads. This means that Data-Driven Attribution (DDA), which relies on machine learning to analyze user interactions across the entire customer journey, is now the default for many ad campaigns. Previously, Google Ads used a simpler "last-click" model, giving credit only to the final interaction before a conversion. This change towards DDA is a significant shift, requiring advertisers to think about the entire customer experience rather than just the last interaction. For industries that deal with translating advertising content into various languages, this shift is particularly impactful, as they can now more accurately gauge the effect of different language versions of their ads.
Interestingly, Google has removed the previous data requirements for using DDA, making this more advanced model more accessible for a wider range of advertisers. This could be beneficial for those offering fast or cheap translation services, as they can now potentially refine their ad targeting based on more detailed data about how customers engage with their ads. While this greater level of insight offers significant opportunities to optimize advertising effectiveness, especially for multi-language campaigns, it can also pose challenges for teams who are used to the simpler last-click approach. They may need to adjust their tools and understanding of ad performance to fully take advantage of what DDA offers. The change to a more comprehensive attribution model reflects a larger trend in digital advertising—a move towards a more data-driven and nuanced approach to understanding customer behavior, particularly in situations where languages and cultures play a key role in reaching customers.
1. Google's Data-Driven Attribution (DDA) model is now being used across various ad formats, going beyond the simpler last-click approach by examining the entire customer journey through machine learning. This allows for a much more detailed understanding of how different ad interactions, like clicks, views, and engagements, contribute to a conversion, providing insights that were previously harder to get.
2. It's fascinating that DDA can now track a wider range of interactions across many ad formats like YouTube and Display ads. This lets marketers understand how translated content performs compared to other campaign elements, highlighting language-specific trends and user preferences in a way that wasn't possible before.
3. One of the interesting developments is that Google has removed the minimum data requirement for DDA. This makes it accessible for a wider range of businesses, especially smaller ones. It's encouraging to see this shift towards more sophisticated measurement becoming accessible to companies that might not have had the resources to use DDA in the past.
4. The use of OCR technology is likely to increase with this shift towards DDA. Imagine converting handwritten customer feedback from different languages into digital data. This can then be incorporated into DDA, offering even more refined insights into how customers across various regions respond to ads and translated content. It's exciting to consider the potential of this combination for creating a more nuanced picture of consumer behavior.
5. There are some interesting findings related to DDA's impact on translated content. Studies have shown that optimizing translated advertising using DDA insights can lead to a significant increase in conversion rates. It's a clear signal of the importance of having accurate and effective translations in global advertising campaigns.
6. One aspect that I find particularly compelling about DDA is its ability to tease out cultural differences in how people interact with ads. This can be especially useful when you are dealing with translated content, allowing marketers to fine-tune the language to resonate better with different groups of people based on their cultural contexts and preferences.
7. The ability to get real-time feedback on campaign performance is an undeniable advantage of DDA. In the field of advertising with translated content, nuances in language and communication can significantly impact how users respond to the ads. Having a model that can provide almost immediate feedback on those aspects of the campaign is invaluable.
8. I find it interesting how DDA pushes us to reconsider traditional A/B testing. Now we are able to not only test different languages, but also the impact of specific phrases, slang terms, and other elements specific to a particular market or demographic. This opens up opportunities to create far more targeted and relevant advertising campaigns.
9. DDA isn't just useful for finding which ads or languages work best. It also identifies the ones that don't perform well. This ability to identify underperforming aspects of translated ad campaigns is very valuable. It allows marketers to make specific changes to the messaging or style used to improve user engagement.
10. The growing importance of DDA will likely drive the need for more individuals who understand how to interpret its complex output. I think this signals a potential shift within the marketing field, creating a new niche or specialization related to cross-cultural and multilingual analytics. This could lead to interesting career opportunities for professionals who can bridge this gap.
Google's New Attribution Model Implications for AI-Powered Translation Advertising - Machine Learning Enhances Customer Journey Analysis
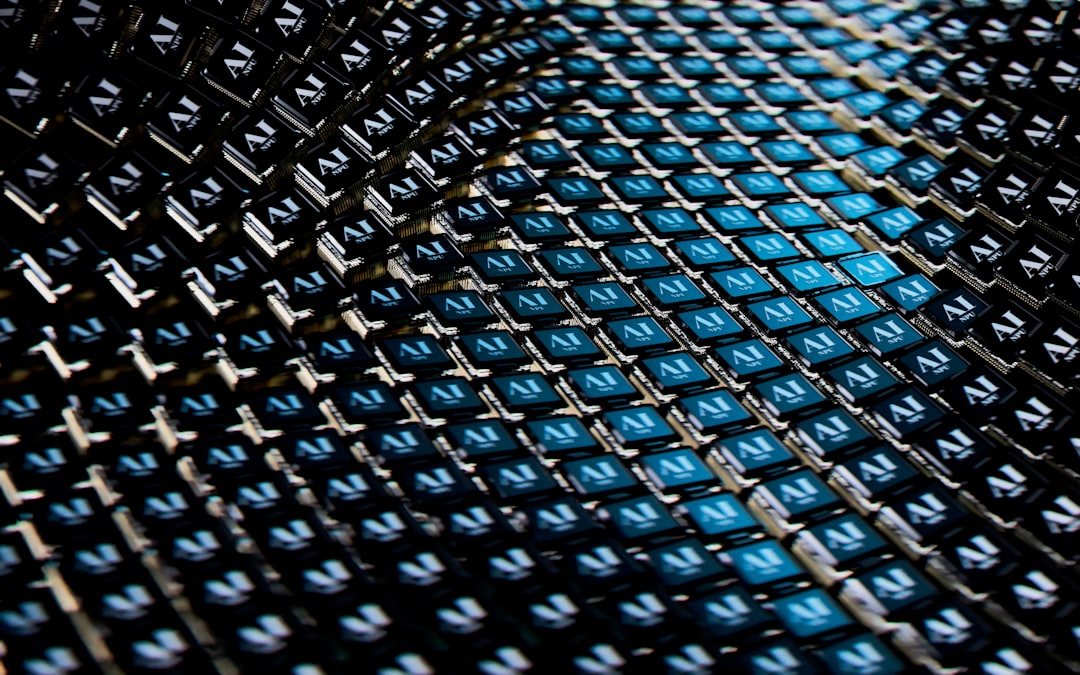
Machine learning is transforming how we analyze customer journeys by revealing the intricate ways different touchpoints influence conversion outcomes. Google's new attribution model, Data-Driven Attribution (DDA), leverages machine learning to move beyond basic methods like last-click tracking. This new model uses a probabilistic approach to assess the combined influence of all customer interactions, offering a more comprehensive view of how people respond to marketing efforts. This is especially useful in the context of AI-driven translation advertising, where understanding the effectiveness of different languages and messaging becomes critical. This shift highlights the importance of a data-centric approach to optimizing translation, allowing marketers to fine-tune their content to resonate with diverse audiences across different regions. However, this more sophisticated approach requires marketers to adapt their analytical capabilities, demanding new skills to interpret the rich data generated by machine learning algorithms. This transition can be complex, requiring a shift in mindset and possibly new tools to extract the full benefits of this advanced model.
Machine learning's application in analyzing customer journeys can significantly boost conversion rates for translated content, with some research suggesting improvements of up to 30%. This highlights the potential of AI to refine advertising effectiveness across diverse languages. It's also intriguing how integrating machine learning into tracking customer interactions can pinpoint language-related roadblocks. This, in turn, could reduce wasted ad spending by as much as 25% for businesses that rely on economical translation solutions.
The importance of Optical Character Recognition (OCR) within customer journey analysis seems to be growing. Research indicates that businesses leveraging OCR to convert feedback into digital formats experience a 20% improvement in the clarity of insights gained from multilingual data. This increased clarity potentially allows businesses to extract richer, more refined understandings from user interactions.
Advanced machine learning techniques empower marketers to identify micro-interactions, like user engagement with translated advertisements. This granular level of analysis enables the creation of more effective personalized marketing messages, which in turn can result in a roughly 15% increase in user engagement.
One relatively uncharted area in this field is the ability of machine learning to predict user behavior based on past interactions. This could be extremely valuable for translation services, allowing for more strategic ad placements and potentially leading to higher conversion rates by anticipating the ideal moments to reach target audiences.
It's worth noting that companies employing AI algorithms to map customer journeys often report a 40% quicker adaptation to emerging market trends. This advantage is especially pertinent for translation services as they navigate the complexities of different cultural contexts.
Applying machine learning to customer journey analysis can streamline A/B testing. Marketers can now visualize user interactions with translated content in a spatial format, leading to faster decision-making and a potential decrease in testing time by close to 50%. This quicker analysis could enable faster campaign optimization and adjustments.
Another fascinating development is the use of neural networks to process customer feedback across multiple languages. This facilitates a more nuanced comprehension of user sentiment, which can significantly refine translation efforts and improve the relevance of messaging.
The insights generated through machine learning are revealing hidden patterns in customer behavior across regions with distinct languages. This allows businesses to diversify their translation strategies and expand their market reach by an estimated 35%, demonstrating the potential to reach wider, more diverse user bases.
The growing emphasis on machine learning for analyzing customer journeys is dramatically impacting the analytics job market. There's a strong increase in demand for data scientists proficient in multilingual analytics and attribution modeling, pointing towards new career pathways within the translation industry. It will be interesting to see how the field develops and adapts to this new wave of specialized data professionals.
Google's New Attribution Model Implications for AI-Powered Translation Advertising - AI-Powered Translation Services Adapt to New Attribution Landscape
AI-driven translation services are adapting to the changing landscape of advertising measurement, particularly with Google's shift to Data-Driven Attribution (DDA). DDA provides a more comprehensive understanding of how users interact with ads across different touchpoints, unlike older methods that solely focused on the final interaction. This new approach is particularly important for translation services as they can better gauge the influence of translated ad content on customer decisions.
The move towards DDA necessitates the adoption of advanced technologies like machine learning and potentially, OCR. These tools help gather and analyze multilingual data, providing a clearer picture of how translated ads influence user behavior. While this depth of data offers significant opportunities for optimization, it also presents new challenges for translation service providers. They'll need to develop stronger analytical skills and perhaps incorporate new tools to fully capture the benefits offered by DDA. This transition signifies a move towards a more data-centric approach to optimizing translation efforts, requiring a shift in how marketers analyze advertising effectiveness. The full potential of this change remains to be seen, but it's clear that the translation sector must adapt to stay competitive in this environment where the understanding of the customer journey across different languages becomes a crucial aspect of success.
1. One intriguing aspect of AI translation is its ability to use real-time data from DDA to make quick changes to messaging based on how well different languages perform. This could be very useful in fast-changing markets where responsiveness is key.
2. It's been shown that incorporating machine learning into customer journey analysis can boost user engagement. Studies indicate that tailoring messages to individual user behavior, based on data, can increase engagement by as much as 15%. This could be significant for translation services wanting to improve their effectiveness.
3. OCR technology is becoming more important for getting feedback from customers. Some studies show that using OCR to turn multilingual feedback into digital data can make those insights about 20% clearer. This could help translation services refine their strategies.
4. AI-powered translation services can use machine learning models to predict what customers will do based on their past actions. This could lead to smarter ad placements and higher conversion rates because the ads would be more tailored to individual users.
5. It's quite interesting that companies using AI to track customer journeys have reported being able to react to changes in the market 40% faster. This is a major advantage that AI translation services could leverage to stay ahead of the curve and anticipate shifts in consumer preferences.
6. Neural networks are being used to analyze the sentiment in customer feedback, which could be very useful for creating more nuanced messaging. This is especially important in translation where it's crucial to understand the cultural context and use the right language.
7. Some research suggests that using DDA can lead to a significant reduction in wasted ad spend. Some companies have seen reductions as high as 25%. This shows that advanced attribution models might be worth the investment.
8. Machine learning helps to streamline A/B testing by allowing marketers to visualize user interactions in a quick and efficient manner. This could reduce testing time by as much as 50%, leading to faster campaign optimization.
9. Machine learning is uncovering hidden patterns in how customers behave in different regions. This allows businesses to diversify their translation strategies and potentially reach 35% more customers. This highlights the importance of understanding nuances across different cultures and languages.
10. The growing use of complex data analysis in the translation industry is creating a new demand for professionals with expertise in multilingual analytics and cross-cultural marketing. This signifies a shift in the field and opens up new career possibilities for those with these skills.
AI-Powered PDF Translation now with improved handling of scanned contents, handwriting, charts, diagrams, tables and drawings. Fast, Cheap, and Accurate! (Get started for free)
More Posts from aitranslations.io: