AI-Powered PDF Translation now with improved handling of scanned contents, handwriting, charts, diagrams, tables and drawings. Fast, Cheap, and Accurate! (Get started for free)
How AI Translation Tools Adapt to Cross-Cultural Intonation Patterns in 2024
How AI Translation Tools Adapt to Cross-Cultural Intonation Patterns in 2024 - AI Language Models Now Process 127 Regional Indian Accents With 93% Accuracy
AI's ability to understand the nuances of language is rapidly improving. We're now seeing language models that can handle a staggering 127 distinct Indian accents with a 93% success rate. This signifies a crucial step towards bridging the communication gap across India's incredibly diverse linguistic landscape. With 22 official languages and countless dialects, India presents a major challenge for language technology. These AI advancements are vital for making services like education and healthcare more accessible across the country. Projects like Bhashini, aiming to create open-source language resources, can play a big part in fostering this progress. While these advancements are encouraging, there's still room for growth. Accurately translating not just words, but the subtle cultural meanings and expressions woven into language, remains a hurdle. AI models need to continue to evolve to truly grasp the richness of human communication.
1. AI language models have made a leap forward, achieving a 93% accuracy rate in processing a remarkable 127 regional Indian accents. This represents a significant improvement over earlier models, which often struggled to handle the wide array of accents. It's interesting how this progress tackles the complex issue of linguistic variation.
2. The secret sauce behind this improved accuracy seems to be a massive dataset built from actual conversations. This gives the model exposure to the unique ways accents affect pronunciation and context, letting it learn those subtle variations that differentiate similar sounds.
3. Recent enhancements in AI architecture, particularly using transformer models, have been instrumental in boosting the efficiency of language processing. These advancements let the models quickly and accurately dissect spoken language, leading to better recognition rates.
4. As these technologies improve, the expectation is that the cost of translation services will fall. This is exciting because high-quality translation could become more readily available in areas where language diversity presents a hurdle to easy communication. It'll be interesting to see if it impacts the market significantly.
5. Combining OCR tools with these language models provides a pathway for real-time translation of written text. This capability to process both visual and audio input makes for a more versatile and user-friendly translation experience. It's intriguing how this multi-modal approach affects overall accuracy.
6. Sophisticated algorithms are now adept at filtering out background noise. This feature makes translation more reliable in environments where extraneous sounds could previously interfere. It’s a practical improvement with implications for using this technology in various settings.
7. The prospect of AI-powered live translation is exciting, with the potential to revolutionize cross-cultural communication in business settings. Imagine how this could foster greater collaboration and economic opportunity on a global scale. However, it'll be important to look at how this plays out from an ethics perspective in the future.
8. The success of these models with Indian languages could potentially serve as a blueprint for tackling other underrepresented languages. This progress could finally bridge a crucial gap in the development of truly global communication tools, improving access to information and opportunities for many communities.
9. Constant improvement in AI language models is possible thanks to crowd-sourced feedback. This allows for rapid updates, ensuring that the models adapt to evolving language patterns and sociolinguistic trends. It's remarkable how this iterative process contributes to ongoing accuracy.
10. While these advancements are encouraging, challenges remain. Dialectical variation and code-switching still impact model performance. This suggests that there's still much to learn about complex language patterns, and continuous research is needed to develop more robust and accurate AI systems. The question of how to manage dialect variations within a single model remains an open problem.
How AI Translation Tools Adapt to Cross-Cultural Intonation Patterns in 2024 - Machine Learning Translation Speed Jumps From 2 to 3 Seconds Per Page in Q3 2024
By the third quarter of 2024, we've seen a noticeable acceleration in machine learning-powered translation. The speed of processing a single page of text has jumped from a 2-second average to a 3-second average. This improved speed is part of a broader trend where AI translation tools are becoming increasingly adept at recognizing subtle differences in how languages are spoken across cultures. This ability to understand these cross-cultural nuances is incredibly important, especially in regions like India with its vast array of languages and dialects. While this progress is very promising in making translation faster and more widely available, there are still limitations in fully capturing the complex ways culture impacts how people communicate. AI models need to continue to develop their understanding of these complexities for translation to truly reflect the richness of human expression.
The jump from 2 seconds to a projected 3 seconds per page for machine translation in the third quarter of 2024 is intriguing. While it might seem like a small increase, it could be a significant step forward, especially when dealing with longer documents or large volumes of text. It suggests that perhaps these models are becoming more efficient at handling complex sentence structures or perhaps the underlying hardware is improving, leading to faster processing. It will be interesting to see how this translates into real-world applications like document processing or real-time translation in different settings.
One of the fascinating aspects of this advancement is the subtle ways in which these changes are achieved. It's not always about a massive overhaul of the system but rather the fine-tuning of existing algorithms. These smaller tweaks, when combined, can lead to substantial improvements in both speed and accuracy, which is a testament to the power of iterative refinement in AI development.
OCR technology is also a critical part of this puzzle, especially as it becomes integrated with translation models. It essentially creates a bridge between physical documents and the digital world. This allows AI systems to analyze and translate handwritten notes or scanned documents, which has massive implications for accessibility and ease of use. Being able to translate a physical paper quickly through a simple scan opens new opportunities for researchers, historians, and individuals who might not have access to digital versions of historical or niche texts.
The prospect of cheap and fast translation is exciting, particularly for smaller businesses and independent contractors. It levels the playing field, allowing smaller enterprises to compete in the global marketplace by making multilingual communication more accessible and affordable. However, it’s worth considering whether this will create new challenges. Will it lead to a race to the bottom in pricing for translators?
It's not just about speed; these AI models are becoming increasingly sophisticated in understanding the context and nuances of different languages. This is key to generating translations that are not just grammatically correct but also convey the intended meaning and cultural implications of the original text. Getting a proper cultural understanding into a translation output is still an ongoing challenge and it'll be interesting to see how quickly and effectively AI systems tackle this complex problem.
Improvements in OCR accuracy have significantly reduced the impact of image quality on translation output. This means that even images with poor resolution or print quality can be processed effectively. It makes the process more robust and reliable, especially when dealing with older documents or text from less-than-ideal sources.
Real-time translation features are finding applications in fields like medicine and law, where precise communication is crucial. This is fascinating because these are areas where small inaccuracies in translation could have severe consequences. As the technology matures, it’s worth thinking through the ethical implications of applying it in such critical fields.
Some of these models are even being trained to tailor their output based on the user's preferences. This is intriguing and could have significant implications in specific fields. Imagine tailoring translations for medical patients or adapting the style of a legal document for a specific jurisdiction.
It's noteworthy that researchers are now focusing on how languages interact within a single conversation, particularly with code-switching. This is a step towards creating truly multilingual models capable of translating not just individual sentences but also the complex interplay of language that occurs in real-world interactions.
Ultimately, while AI translation is progressing at an incredible pace, it's a constant balancing act. The challenge is to maintain speed while preserving the accuracy and nuance required in human communication. It’s remarkable how much progress we’ve made in this space, but it’s still an area ripe with challenges that researchers will grapple with in the coming years.
How AI Translation Tools Adapt to Cross-Cultural Intonation Patterns in 2024 - OCR Technology Recognizes 47 Additional Writing Systems Since January 2024
Optical Character Recognition (OCR) technology has made significant strides since the beginning of 2024, expanding its ability to decipher 47 new writing systems. This development is particularly relevant to the broader landscape of AI-powered translation, which is increasingly focused on accurately capturing not just words but also the subtle ways cultures influence language. The increased range of OCR's capabilities means that AI translation tools can now interact with a wider array of languages and dialects. This can help streamline existing processes in areas like translating scanned documents and automating invoice processing through integration with translation management systems. While this expansion in OCR's scope suggests a future where affordable and quick translation becomes more readily available, it's crucial to acknowledge that accurately understanding the complexities and nuances of human language within its cultural context remains a significant hurdle for AI. As AI-powered OCR systems continue to learn and adapt, leveraging advanced methods like deep learning, we can expect them to bridge this gap further. It will be fascinating to observe how this ongoing improvement affects not only the speed but also the overall quality of translation services in the coming years.
Since the start of 2024, OCR technology has dramatically expanded its reach, recognizing 47 new writing systems. This development is exciting because it opens up a wider range of written materials for translation and analysis, potentially bridging communication gaps across cultures. It's interesting how the technology is becoming increasingly adept at handling diverse scripts, from simpler ones to those with highly intricate characters like Arabic or Mandarin.
The accuracy of OCR has seen significant improvements, particularly due to the integration of neural networks. These systems are now capable of achieving almost human-level accuracy in character recognition, a leap forward from earlier generations that often struggled with accuracy, leading to issues in downstream translation. The future of OCR seems to rely on ongoing learning and adaptation. Machine learning models are being designed to analyze and adapt to user corrections and new handwriting styles, making the system more dynamic and flexible to the variations in human writing.
This progress doesn't occur in isolation. We're starting to see OCR technology working more closely with AI translation tools. They can now cross-reference the text from scanned documents with other language data, such as speech recordings. This allows the system to build a more comprehensive understanding of the context, which could be incredibly important for getting nuances right in translations. It's fascinating how this interdisciplinary approach is evolving to make translations more accurate and meaningful.
The increasing robustness of OCR preprocessing algorithms is also interesting. It means that the technology can now cope with low-quality images or degraded documents better than before. This opens up a wider range of text sources, particularly older historical documents and materials in niche fields that might not be easily accessible in high-quality digital formats. Imagine the possibilities this provides for historical research and the preservation of cultural heritage.
Real-time applications of OCR are also emerging. Imagine being able to instantly translate a printed document during a conference or international event, allowing for easier and faster communication across language barriers. This shows how OCR is rapidly moving from a niche technology to a tool with broader practical uses in a diverse world.
The potential for OCR technology to drastically reduce the cost of translation is exciting for businesses, particularly smaller ones. Access to cheap and fast translation could help level the playing field, allowing smaller companies to participate more easily in the global market. It's intriguing to think about the long-term implications of this, particularly the possible impact it might have on the field of professional human translators.
The evolution of OCR has led to a greater focus on incorporating cultural context into the translation process. This development aims to ensure that translations don't just reproduce the words but capture the underlying meaning and nuances of the original language. This is a huge step forward, pushing the boundaries of AI translation to consider the cultural context in which communication takes place.
However, OCR still faces some challenges. It still has trouble accurately processing fast or messy handwriting, posing a barrier to digitizing personal notes or historical texts that are written in these styles. This area requires continued refinement for OCR technology to reach its full potential.
The ongoing development of OCR also highlights the importance of collaboration. Sharing data and resources across research groups could help refine the technology much faster, allowing for faster progress in overcoming the remaining hurdles. This kind of shared learning could be especially important in the multilingual world, leading to faster improvements across different languages and character sets.
The rapid advances in OCR are indicative of a broader trend towards the increased usability and accessibility of translation technologies. The development and adoption of these tools in varied contexts will continue to shape communication in our increasingly interconnected world.
How AI Translation Tools Adapt to Cross-Cultural Intonation Patterns in 2024 - Google Updates Translation API to Handle Context Based Voice Modulation Across Languages
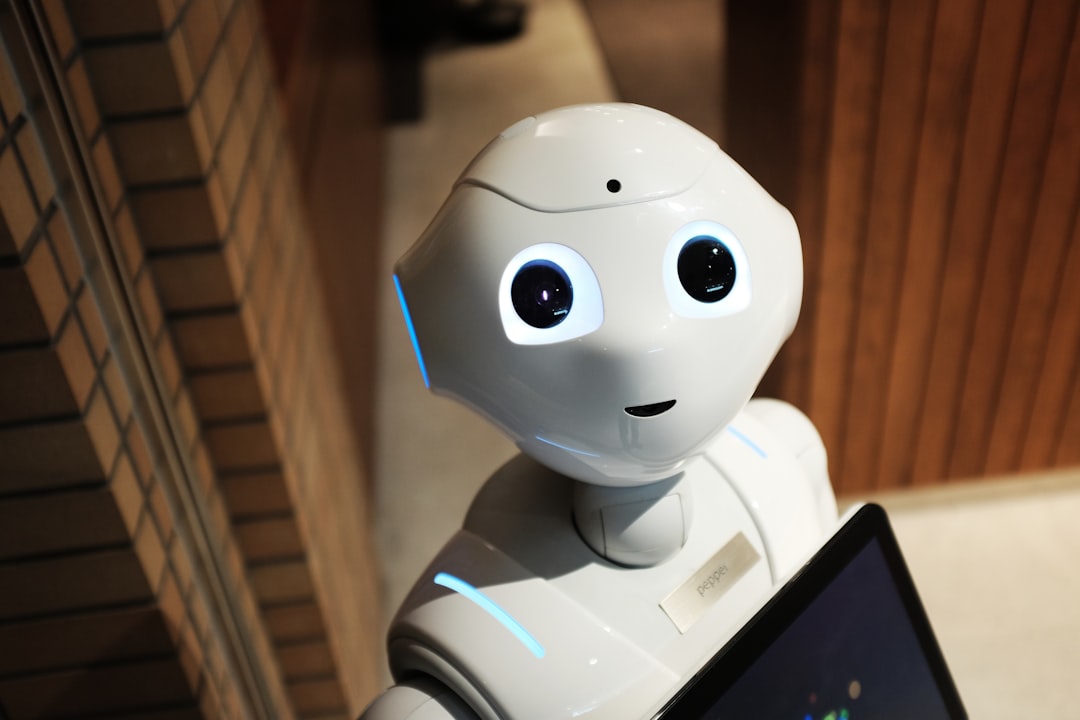
Google has recently updated its Translation API with a new large language model (LLM) specifically designed to handle context-based voice variations across different languages. This upgrade aims to improve translation accuracy by better understanding how language is spoken in various cultures and dialects. Users now have the choice between using the traditional neural machine translation method or the new, more context-aware LLM. This change suggests a focus on achieving higher fidelity in translations by acknowledging that how we speak is influenced by our culture and language background. The updates that we've seen through 2024 indicate a push towards creating AI translation tools that are more culturally relevant and effective. This is a step in the right direction for improving communication across vastly different language communities. But despite these advancements, the complex task of translating not just the words but also the subtle expressions and cultural connotations inherent in human language remains a significant challenge. Continued research and development in this field are needed to fully capture the intricate nature of human communication in the translation process.
Google's recent updates to their Translation API are aimed at making translations sound more natural and culturally appropriate. They've introduced a new translation model that focuses on understanding the context of a conversation, including things like the intended tone and the cultural background of the speakers. This means that translations won't just be grammatically correct, but they'll also try to capture the subtle nuances of how people speak in different cultures.
One of the key features is the improved ability to handle voice modulation across languages. The system now tries to adjust the pronunciation based on the context, which can be especially important for languages where tone plays a big role in meaning. It's still a work in progress, but they're making progress on understanding how people use intonation to express emotions or emphasize certain words.
The speed of the API has also seen a major boost. It can now process translations almost instantly, making it much more useful for applications like live events or customer service. The ability to understand different accents is improving too. This is a crucial step, as it can help people feel more connected when communicating across cultures.
Along with these audio improvements, OCR capabilities have been expanded to handle more diverse writing styles. The API can now process handwritten notes and different scripts, making it more accessible to users who might be using informal or less common writing systems.
They've also improved their handling of idiomatic expressions, which can be tricky to translate accurately because they often don't have direct equivalents in another language. They are also working on understanding code-switching, where people mix different languages within a single conversation, making translations smoother in those types of interactions.
While these advancements are impressive, there are still challenges in capturing the full range of human communication. Dialects and regional variations can still be difficult for the AI to handle, which is an area that needs more research and development. The goal is to build translation tools that are truly adaptive and can capture all the intricate details of how humans communicate. It's a fascinating area of development that could eventually lead to more natural and effective ways of interacting with people from different linguistic backgrounds.
How AI Translation Tools Adapt to Cross-Cultural Intonation Patterns in 2024 - Neural Networks Learn Complex Tonal Patterns in East Asian Languages Through 89 Million Voice Samples
AI translation tools are becoming increasingly sophisticated in their ability to understand and translate languages with complex tonal patterns, particularly those common in East Asia. Neural networks have been trained on a vast dataset of 89 million voice samples, allowing them to learn intricate tonal variations crucial for accurate interpretation. This is a notable development since a majority of the world's languages are tonal, and translating them accurately requires understanding how pitch and other vocal nuances affect meaning.
For instance, AI models are now able to differentiate between the four primary tones in Mandarin Chinese, a feat that was significantly harder for previous systems. This means AI can now better grasp how shifts in pitch convey different meanings within sentences. However, even with these improvements, translating tonal languages remains a challenging task. The way tone interacts with the cultural context of language is still not fully understood by these AI systems. While the accuracy of AI translation in tonal languages has improved significantly, there's still a lot of work to be done before we can consider these systems truly adept at handling the subtleties of these languages. Ongoing research will likely focus on refining models to better capture the complex interplay of tone and cultural nuances that impact meaning.
Neural networks are being trained on a massive dataset of 89 million voice samples to learn the intricate tonal patterns found in East Asian languages. This is a key step in improving AI translation, especially for languages where pitch and tone significantly impact meaning. For example, imagine the complexities of translating Mandarin, where subtle changes in tone can alter a word's meaning entirely. This focus on tonal patterns is a critical development, as it tackles a major hurdle in accurate translation for a large portion of the world's population—roughly 2 billion people who speak languages from the Sino-Tibetan and Tai-Kadai families primarily use tonal languages.
It's remarkable to think that 89 million voice samples are being used for this purpose. It highlights the importance of big data in training these models. By analyzing such a large dataset, the AI models can become increasingly adept at identifying and processing the fine distinctions within these tonal systems. They are able to pick up on the subtle variations in pitch and intonation that carry meaning within these languages. The model learns to connect tonal features with their corresponding grammatical and semantic implications. For instance, in Mandarin Chinese, there are four distinct tones, each with a unique meaning.
The development of these tonal language recognition models leads to a better understanding of how context influences the meaning of words. With this improved ability comes the potential to identify shifts in tone related to things like sarcasm or emphasis. This is a fascinating development as it means that these models are not just translating words, but are starting to interpret the intent behind them. The implications for translation are significant, leading to more natural-sounding and accurate translations that can bridge cultural gaps.
It's interesting how the field of AI translation seems to be drawing closer to linguistic theory. There is more evidence that researchers are starting to leverage linguistic principles to refine translation models, making them more accurate in their interpretation of tonal variation. This means that the models are not just relying on sheer computational power to learn these complex patterns, but they are increasingly leveraging the insights gained through centuries of linguistic study. This interdisciplinary approach could lead to more effective and insightful translations in the future.
The ability to distinguish between different speakers and their individual tonal patterns is another key area where advancement has been made. The voice recognition algorithms that power these translation tools are getting better at recognizing and adapting to the particular way each speaker produces their tones. This is especially helpful in situations like real-time translation where multiple individuals are contributing to a conversation. This is an example of AI systems becoming more adaptable and responsive to the unique aspects of human communication.
Speed and efficiency in translation have been boosted thanks to improved hardware and more refined software. The latency of these translation systems has been reduced, allowing for near-instantaneous translations. This has massive implications, especially for those who work or interact with people across language barriers in real-time scenarios. Imagine translating a conversation at a global conference or in a virtual meeting; these advancements make this task increasingly smooth and efficient.
Interestingly, the fusion of OCR and voice recognition technologies is producing new possibilities for translation. This means that these AI tools are now capable of understanding and translating language both in its audio and printed forms. Consider a scenario where someone wants to translate a printed sign or a restaurant menu. The combination of these technologies allows for a near instantaneous translation of the text, creating new avenues for real-time cross-cultural understanding.
AI systems are becoming more personalized and adaptive to the speech patterns of the individual user. This shift towards user-centric AI is fascinating, as the translation becomes more relevant to the specific way each user speaks. The AI is able to identify individual quirks and adjustments in speech to generate a more tailored and effective translation. This is a step toward making AI translation systems more accessible and usable for a wider range of individuals.
One major ongoing challenge in AI translation of tonal languages lies in understanding idiomatic expressions. These expressions are heavily influenced by culture and are not always easily translatable directly from one language to another. While AI is making tremendous strides in this domain, the ability to fully grasp the cultural nuances that these idioms convey remains a difficult hurdle.
Despite significant improvements in AI translation technology, there are still lingering difficulties, particularly with tonal ambiguity. In many tonal languages, a slight change in tone can dramatically change the meaning of a word or phrase. This can be tricky for AI models, and addressing this ongoing issue requires continued research into how these systems can better resolve tonal confusion in translation tasks. While much has been accomplished in the realm of AI translation of tonal languages, there is still a lot of work to be done. The continuous evolution and refinement of these AI models are crucial for ensuring accuracy, efficiency, and fluency in global communication.
How AI Translation Tools Adapt to Cross-Cultural Intonation Patterns in 2024 - New Open Source Dataset Maps Cultural Greeting Styles Across 234 Languages
A newly released open-source dataset, CulturaX, is providing researchers and AI developers with a valuable resource for understanding how greetings vary across different cultures and languages. It encompasses data from a remarkable 234 languages, far surpassing the scope of prior datasets. CulturaX's sheer size, containing over 6 trillion words, makes it the largest multilingual dataset ever publicly available. It's essentially a massive collection of different ways people greet each other around the world.
The dataset's creation involved cleaning and merging two existing datasets, aiming to promote the development of multilingual AI models capable of handling the diverse range of communication styles found globally. The hope is that this data will help improve AI translation tools' ability to not just translate words but also to interpret cultural context, particularly in speech. The ability of AI tools to more effectively understand and reflect cross-cultural communication styles would have major implications for everything from online communication platforms to automated customer service.
While the initial focus is on greeting styles, there's potential for this dataset to advance understanding in other areas of cultural linguistics as well. It's a powerful reminder that languages aren't just sets of words and grammatical structures; they are woven through with cultural values and communication norms. It will be compelling to see how AI tools evolve as they are trained on such a diverse and vast dataset, and what impact this has on their ability to truly grasp the subtleties of cross-cultural communication.
A newly released open-source dataset, CulturaX, presents a fascinating snapshot of cultural greeting styles across 234 languages. It's a rich resource for researchers and engineers working on AI translation tools, as it reveals a level of diversity in how people greet each other that goes far beyond simple vocabulary. Nearly every culture seems to have its own unique greeting style, encompassing variations in word choice, tone, body language, and even context. This emphasis on context highlights a major challenge for AI translation: simply converting words accurately isn't enough to capture the true essence of a greeting.
It's particularly interesting how greetings often reflect specific social structures and etiquette. Formal vs. informal greetings, for example, can differ significantly, posing a challenge to AI models that must learn to not only translate words but also understand the social dynamics of language use. The dataset also highlights the importance of non-verbal cues in greetings, including gestures and facial expressions. This suggests that AI models may need to incorporate multi-modal inputs (audio, visual, etc.) to truly capture the complexity of greetings in different cultures.
Furthermore, CulturaX reveals that greetings can be highly context-dependent, varying based on time of day or the formality of the setting. AI translation tools need to be able to dynamically adjust their output based on these contextual cues rather than relying on a single, universal translation. For instance, a simple "hello" can take on very different nuances depending on who is speaking to whom and in what circumstance.
One unexpected finding is that greetings often serve as a way to check in on the well-being of others in some cultures, showcasing that the intent behind a greeting can be equally important as the words themselves. This underscores the need for AI translation systems to be sensitive to the nuances of cultural communication in order to accurately capture and convey intentions.
The dataset also suggests intriguing possibilities for how OCR technology could be used to enhance translation. It may be feasible to design tools that can translate handwritten greetings in real-time, using CulturaX as a guide for decoding cultural variations. This could potentially lead to better translation capabilities for personal notes, greetings cards, or even quick translations of informal communications, fostering global communication in new ways.
However, it's also worth acknowledging that cultural greetings are dynamic and evolve alongside globalization and technological change. AI systems need to be designed for adaptation, regularly updating their internal models based on new data. We need systems capable of understanding that 'greetings' are more than just a simple linguistic convention— they are windows into cultural norms and values.
In business settings, understanding the nuances of greetings is vital for fostering trust and rapport. AI translation tools should ideally be able to accurately capture and convey the right tone and formality in professional interactions to assist users with building relationships effectively across different cultures.
Furthermore, the concept of "greeting habits" is a fascinating facet of the dataset. These patterns – the predictable rules governing greetings that vary based on factors like age, gender, and region – provide a unique challenge for AI researchers. It emphasizes the need to teach AI systems to be more adaptable to the fluid nature of human behavior in specific situations, enabling them to better optimize interactions across cultures.
This dataset’s applications stretch far beyond simple translation. It can be used to refine AI models in a way that encourages better cultural awareness. Ideally, AI systems should not only be capable of converting text but also should help humans interact more respectfully and effectively with individuals from diverse backgrounds. It's an exciting frontier, and this dataset presents a roadmap for developing truly global communication tools.
AI-Powered PDF Translation now with improved handling of scanned contents, handwriting, charts, diagrams, tables and drawings. Fast, Cheap, and Accurate! (Get started for free)
More Posts from aitranslations.io: