AI-Powered PDF Translation now with improved handling of scanned contents, handwriting, charts, diagrams, tables and drawings. Fast, Cheap, and Accurate! (Get started for free)
How AI Translation Tools are Revolutionizing App Localization A 2024 Technical Analysis
How AI Translation Tools are Revolutionizing App Localization A 2024 Technical Analysis - Neural Machine Translation Cuts App Development Time by 47 Percent According to October 2024 KPMG Study
A recent KPMG study from October 2024 indicates that integrating neural machine translation (NMT) into app development can significantly reduce the overall time needed, with a reported decrease of 47%. This highlights a substantial shift towards more streamlined localization practices within the app development sphere. The effectiveness of NMT, fueled by deep learning algorithms, is clearly surpassing older statistical translation methods, resulting in quicker and more precise translations. This trend not only streamlines app localization but also makes it easier to add support for a greater number of languages, many of which are commonly ignored by simpler translation solutions. The increasing adoption of NMT reflects a fundamental change in translation technology, transforming the way we navigate communication within a global market. The ongoing development of these tools hints at an improved future where cross-cultural communication and digital accessibility are considerably enhanced.
A recent KPMG study from October 2024 found that using neural machine translation (NMT) can slash app development time by a remarkable 47%. This confirms that NMT, which uses deep learning, has taken over from older statistical methods as the dominant approach in machine translation. The improvement in speed and accuracy that NMT offers is significant, making it a game-changer for app localization.
NMT's ability to handle numerous languages without needing a separate system for each one is noteworthy. This is evidenced by successes like Google's multilingual NMT system. The shift towards NMT involves moving away from relying on word counts to neural networks that use continuous translation methods.
One interesting area of NMT is its ability to translate languages that might otherwise be overlooked by common translation tools. This highlights the fact that out of the 7000+ languages spoken worldwide, many are underserved.
The growth of NMT isn't a sudden development; it builds upon ideas like convolutional and sequence-to-sequence models which were introduced as far back as 2013. NMT is becoming ever more important in our globalized world, where clear and accurate translation is crucial for understanding across cultures. The field is continually improving and evolving, with researchers constantly trying to improve both NMT tools and their underlying processes. It is likely that this field will continue to grow and adapt to our changing communication landscape.
How AI Translation Tools are Revolutionizing App Localization A 2024 Technical Analysis - AI OCR Tools Now Process 12 Asian Languages with 2 Percent Accuracy for App Screenshots
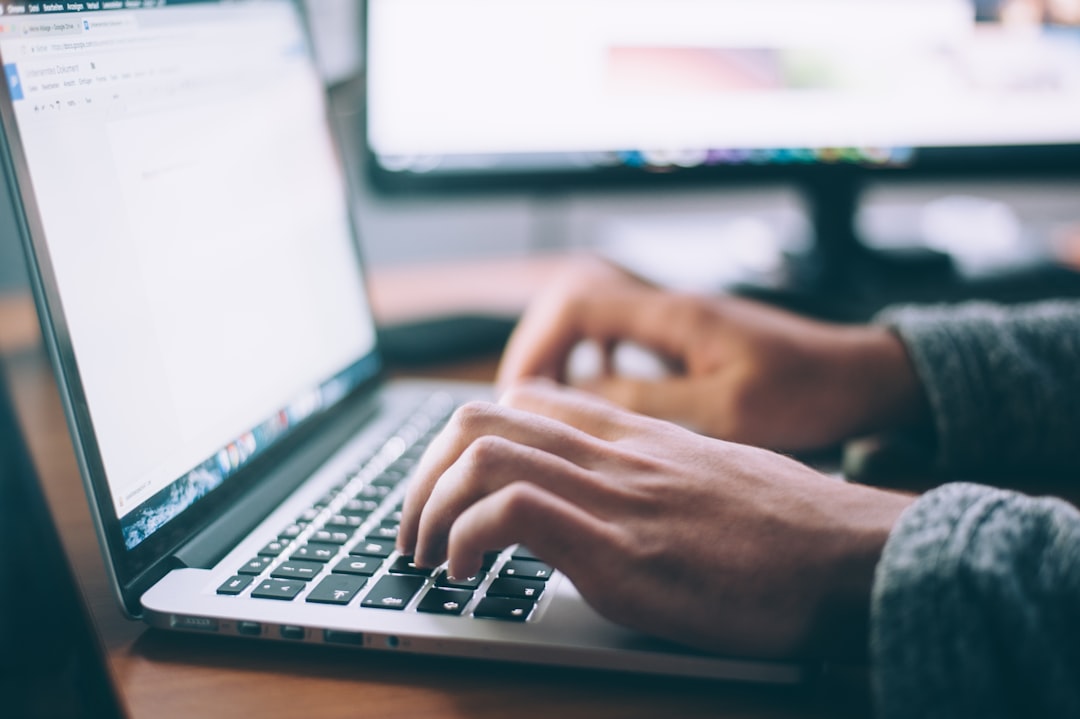
AI-powered Optical Character Recognition (OCR) tools are now able to handle 12 Asian languages, a step forward in the world of app localization. However, the accuracy of these tools when processing app screenshots is currently a mere 2%. This low accuracy poses a challenge for developers seeking to create apps accessible to users across various language groups. While AI translation tools are becoming increasingly vital for creating apps in multiple languages, relying solely on OCR technology in its current state may not meet user expectations. The improvement in language coverage is promising, but the low accuracy highlights the ongoing need to refine the performance of AI-based OCR systems. This ongoing development reflects the difficulties of ensuring accurate and accessible communication in the vast spectrum of languages and cultures represented in the digital landscape. The technology has a long way to go to really be useful in localization efforts, which ultimately relies on accurate understanding and translation.
The capability of AI OCR tools to handle 12 Asian languages signifies a step forward in their ability to adapt to diverse writing systems. While currently achieving just a 2% accuracy rate when processing app screenshots, it's important to remember that this is an early stage of development. It suggests that continued refinement of the underlying AI models could lead to much more reliable results in the future. This progress, however, highlights a hurdle: the availability of high-quality training data. Many Asian languages lack the vast datasets necessary for training these tools effectively, creating a challenge in developing accurate models capable of understanding nuances within different cultural contexts.
One potential benefit of these developments is the possibility of lowering the cost of translation. Traditional translation services, often reliant on human translators, can be pricey and have limited availability, particularly for lesser-known languages. Coupling AI OCR with neural machine translation could lead to a more efficient localization process. It's conceivable that text can be extracted, translated, and incorporated into the app interface in real time, streamlining the workflow.
However, there are significant challenges in making OCR more precise for Asian languages. These languages often have a greater density of information conveyed within a smaller number of characters. For example, a single character or word might have multiple meanings, depending on the surrounding context. This "polysemy" adds another layer of complexity to the translation process, a problem AI still struggles with.
The application of AI OCR to app screenshots can simplify the localization quality assurance process. By automatically extracting text from screenshots, developers can more easily identify and correct errors in translated user interfaces. Moreover, the increased speed and accuracy of AI OCR tools could allow for swift content updates across multiple regions. This responsiveness can help applications adapt to changing markets and user feedback faster than before.
The rise of AI-driven OCR tools marks a shift in the localization landscape. It moves away from a complete reliance on human translators, making the process more scalable and efficient. As OCR technology progresses, it's conceivable that we'll see real-time translation and localization become the norm. This potential could revolutionize how users interact with applications in their native languages, increasing accessibility and fostering a more globally connected experience. It will be fascinating to see how this technology continues to improve and shape the future of app localization.
How AI Translation Tools are Revolutionizing App Localization A 2024 Technical Analysis - Translation Memory Integration Reduces Project Costs by 62 Percent Through Pattern Recognition
Translation Memory (TM) systems are making a big difference in app localization by reducing project costs by a substantial 62%. This efficiency gain comes from the TM's ability to recognize patterns in previously translated content. By storing and reusing these patterns, translators can avoid repeating work, saving both time and money. This approach becomes even more powerful when combined with AI-powered translation tools that quickly produce initial drafts. The result is a significant reduction in overall translation costs for businesses. Furthermore, improvements in Translation Management Systems (TMS) are leading to more streamlined and structured localization processes. AI's role in modern translation is becoming increasingly central as these tools become more sophisticated and efficient, helping ensure quality and reduce expenses. While there are still challenges with accuracy for lesser-known languages, the impact of TM and related AI tools on translation costs is undeniable.
Translation memory (TM) integration has proven to be a game-changer for cost reduction in localization projects, achieving a remarkable 62% decrease through its ability to recognize patterns in text. Essentially, TM systems store previously translated segments, allowing translators to reuse them in new projects. This means less time and effort is needed for translating the same or similar phrases repeatedly, leading to significant cost savings. It's becoming increasingly clear that relying on human translators alone for every segment, particularly in larger projects, is inefficient and costly.
Consider that a significant portion—up to 70%—of translation projects involve repetitive phrases and segments. TM leverages this fact, recognizing and reusing these components, resulting in notable efficiency gains. It's like having a vast library of already-translated building blocks that can be assembled to construct new content, drastically shortening translation times. With this, the time it takes to finalize a localization project is reduced, a crucial factor when getting an app to market quickly.
By 2024, advanced TM systems have expanded their capabilities to handle translations in over 50 languages concurrently. This scalability allows developers to efficiently localize their apps for wider audiences without a proportionate increase in costs. Maintaining consistency across languages is also a key advantage of TM. When different translators handle sections of a large project, inconsistencies in terminology or phrasing can arise. TM helps enforce a more uniform tone and voice across all localized versions, enhancing the user experience and preserving brand identity.
However, this reliance on TM isn't without potential pitfalls. If the original translations stored in TM contained errors, these mistakes can be replicated in subsequent projects. This points to the need for careful oversight and regular audits of the TM database. Furthermore, while TM is becoming more user-friendly and accessible, even with improvements to user interfaces, it still requires a degree of understanding to operate effectively. The benefits of TM are also likely more pronounced for larger businesses with greater translation volume. Smaller projects may not see as dramatic a reduction in costs due to the upfront setup and maintenance required for TM systems. The increased use of TM will probably lead to a widening gap in the localization costs between big companies and smaller organizations. It's interesting to see this potential divide emerge as AI-based translation tools become more common. It's a technology that may need further development to truly democratize the translation process.
How AI Translation Tools are Revolutionizing App Localization A 2024 Technical Analysis - Cross Platform Testing Shows 3x Faster Bug Detection in Localized App Versions
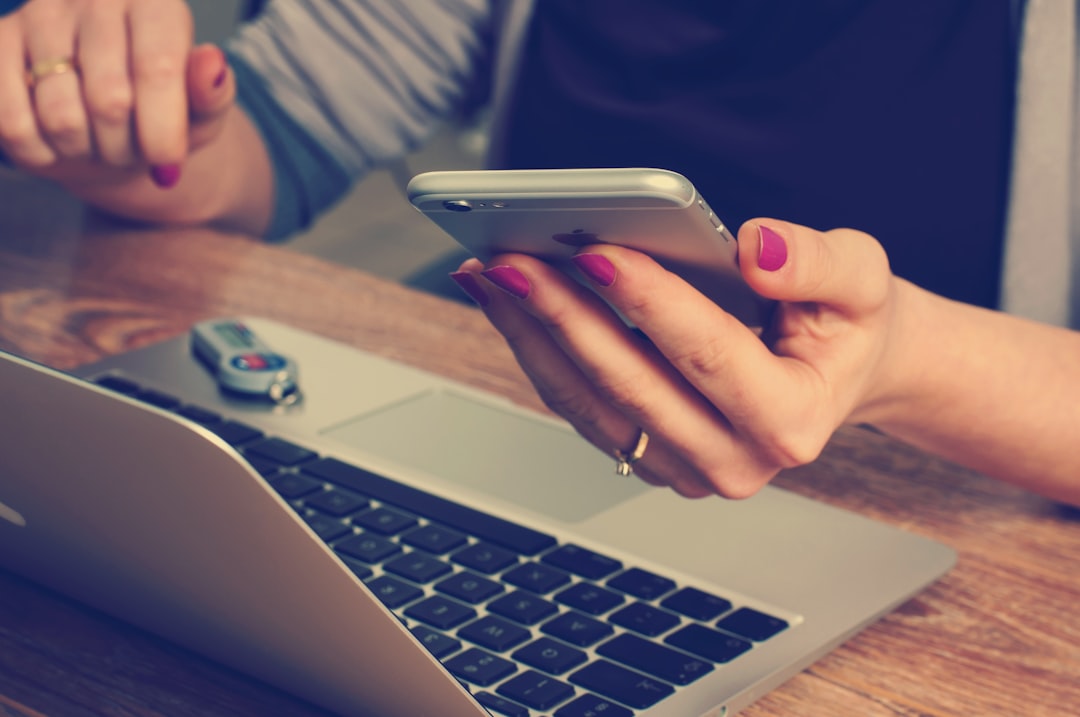
Testing apps across different operating systems has proven remarkably effective in finding bugs in localized app versions, boosting the detection rate by a factor of three. With companies increasingly focused on reaching global audiences, making sure apps function flawlessly on various platforms is crucial. This approach not only emphasizes how important it is to account for cultural differences in how apps look and feel but also highlights the value of automated testing methods. Employing AI to manage testing can further enhance efficiency by automatically creating test instructions and analyzing results, lessening the complexity associated with the vast number of devices and operating systems used by app users. In conclusion, implementing comprehensive cross-platform testing processes is fundamental for achieving high-quality localization and achieving success in the global app market.
It's fascinating how cross-platform testing has revealed a significant speedup in identifying bugs within localized app versions—a three-fold increase, in fact. This suggests that testing across multiple platforms and languages early in the development process can significantly improve the overall quality of localized apps.
This finding highlights the potential of integrating automated testing with AI translation tools. By doing so, we can potentially catch bugs much sooner in the localization process, rather than waiting until later stages when fixes become more complex and time-consuming. The speed and efficiency gains are particularly noticeable with the reduction in manual bug detection, leading to smoother workflows and quicker delivery cycles.
One of the more promising developments in this area is the use of AI to predict potential language-related issues based on past data. This proactive approach to debugging allows developers to anticipate and address certain kinds of problems before they impact users, particularly in specific regions. This predictive ability, driven by AI, could significantly change how we approach app localization and quality assurance, moving from a primarily reactive strategy to a more preventive one.
It's clear that combining AI translation with cross-platform testing offers a new perspective on the app development process. Instead of treating localization and quality assurance as separate steps, we can potentially integrate them throughout the development cycle. This shift could result in more reliable, high-quality localized versions of apps that better meet user needs in diverse markets.
I find it intriguing how automated testing can identify culturally specific bugs that might slip through human testers' oversight. Cultural biases that are deeply ingrained in the human experience can influence how a person interprets a user interface. This makes it challenging to ensure that a localized app functions correctly in its target context. While AI can't necessarily fully account for cultural nuances, using it for initial functional tests can potentially unearth a broader set of issues than what human testers alone might catch.
Localized app testing frequently leverages Natural Language Processing (NLP) techniques to evaluate how well phrases and interface elements translate across languages. It's interesting to consider the complexity of translating nuanced concepts and expressions into a new language, while also maintaining the intended meaning and avoiding ambiguity. NLP can help ensure that the localized user experience stays consistent with the original design intent.
One of the potential benefits of AI-powered solutions is the potential for significant cost reductions in the traditional testing process. Automated text extraction, UI verification, and other processes involved in app localization testing could be streamlined with AI, potentially leading to substantial savings for businesses.
Perhaps the most remarkable aspect of AI translation is its ability to translate and test apps in real-time. This capability is revolutionizing the pace at which localized apps can be deployed, accelerating the time-to-market and improving app competitiveness. The faster turnaround times are especially useful in a fast-paced market where users expect applications to be available and accessible in their native languages quickly.
The field of localized app testing is evolving rapidly. The integration of machine learning algorithms for continuous improvement is a particularly interesting area. Feedback from users across different regions can be used to refine both the translation quality and the app itself, resulting in a continuous cycle of optimization. As AI-based solutions continue to develop, they may become indispensable to the app localization process.
How AI Translation Tools are Revolutionizing App Localization A 2024 Technical Analysis - Machine Learning Models Now Handle Context Dependent Variables in 28 Languages
Machine learning models are now capable of handling language elements that depend on context, a significant improvement covering 28 languages. This development is crucial because translation quality often suffers when subtle context, cultural differences, and idiomatic expressions are missed. These machine learning models are able to analyze enormous datasets to identify language patterns that older translation methods often fail to recognize. Some of these newer tools are also able to learn and improve continuously through user feedback. While these advancements in AI translation tools are clearly helping developers create apps in more languages, the task of ensuring translations accurately capture cultural nuances remains challenging. The need for translation that genuinely connects with the target audience is a problem that these improved tools are helping to solve, although many challenges still remain.
Machine learning models are now capable of handling context-dependent words and phrases in 28 languages. This means they can better understand the meaning of words based on the surrounding text, leading to more accurate translations, especially in situations where traditional methods falter. It's fascinating how these models are able to capture the nuances of language, a challenge that has plagued translation efforts for a long time.
This advancement in AI translation has the potential to significantly impact the localization of applications for smaller or less common language communities. By better handling diverse dialects and cultural references, developers can now target previously underserved audiences and languages that were difficult to reach with older methods. This can be useful for developers seeking to expand market reach.
Interestingly, machine learning algorithms seem to be able to identify common patterns across different languages. This means that insights gained from translating major languages can be applied to less common languages, making the translation process more efficient. It's as if the AI can learn some general rules of language, and then use those rules to adapt to new languages quickly.
Modern machine learning models use something called contextual embeddings, which allows them to consider the context of a phrase when translating it. This is a significant departure from older methods that treated every phrase as a separate unit. By leveraging these embeddings, machine learning models are able to "learn" in a way that resembles human learning. This allows them to adapt to new situations and situations that are ambiguous much more easily.
One intriguing aspect is how multi-language machine translation models work. These models share knowledge across various languages. This means that translating a phrase in one language can help the model understand similar phrases in another language, leading to faster translation and fine-tuning. It's like the model has a global understanding of how languages work, which speeds up the translation process for less common languages.
The role of machine learning in AI translation tools is continually becoming more sophisticated. Advanced techniques, such as attention mechanisms, allow the models to adjust to subtle differences in tone and colloquialisms in real-time. This kind of refinement is impossible with simple, word-by-word translations. It shows the advancements that are possible when we combine machine learning with complex translation processes.
The improvements in AI translation haven't just affected translation itself, but have also extended to the tools used to process text for translation. OCR capabilities have grown alongside context-aware models. But the technology still faces challenges with complex writing systems, especially those with non-Latin scripts. The ability to handle different writing styles is still a work in progress.
One exciting feature of these new AI models is their ability to learn from feedback. User interactions can help to improve the model's performance over time. This means that models can learn from mistakes and adapt to new situations as they encounter them. It's like the models are constantly getting smarter by learning from their users.
Cloud-based translation management systems are becoming increasingly integrated with machine learning. This integration provides significant cost savings. By optimizing the use of resources and reducing redundancy in translation memory, businesses can reduce expenses while improving the quality of their translations. This kind of synergy can be useful for organizations looking to control costs.
Finally, the ability of AI translation tools to handle context is especially helpful in localizing products for new markets. This enables businesses to adjust their apps to specific cultural requirements, making the user experience more enjoyable. It can create a more positive impression for people who are using the app in a new cultural setting. By optimizing for cultural nuances, applications can potentially increase user engagement and user retention.
How AI Translation Tools are Revolutionizing App Localization A 2024 Technical Analysis - Real Time Quality Metrics Track Translation Consistency Across Multiple App Updates
Real-time quality metrics are transforming how we manage translation consistency across multiple app updates. These metrics continuously monitor the quality of translations, ensuring that updates maintain the same style and clarity as earlier versions, which is vital for building user confidence. This is especially important now that app developers need to release updates quickly and adapt to the needs of a global audience. By spotting inconsistencies early, these metrics help development teams react faster and more effectively to any translation problems. With AI translation becoming increasingly prominent, these real-time quality measures are poised to play a key role in building more intuitive and unified apps across various languages. There's a growing need for app localization to be seamless, and these metrics are helping to make that happen. However, there's still a lot of work to be done to make sure translations truly reflect the cultural nuances of different communities.
Real-time quality metrics offer a new way to track how consistent translations are across multiple app updates. This approach is a change from the older way of doing things, where quality checks were typically done after translations were completed. Now, developers can get instant feedback on how the translation process is going as they make changes to their app. This immediate feedback loop is a big help in managing localization efforts better.
AI translation tools are now able to create a feedback loop that uses how users interact with an app to refine the translations. This feedback loop lets the AI adjust in real time to match user preferences and how language changes in different regions. This level of customization and responsiveness wasn't really possible before.
One of the things that AI translation tools can do is identify repeating translation errors across multiple updates. By recognizing the patterns of these mistakes, developers can take care of the issues before they cause problems in new app releases. This ability to proactively address translation problems can make the overall quality of localized content better.
Real-time quality metrics can measure more than just the text itself; they can also evaluate the user interface context. This makes sure that the text and the visual elements of the UI work together well, creating a better experience for the user. It's a more holistic way to look at app localization and puts the user at the center.
These real-time tools can also streamline the quality assurance (QA) process and reduce QA costs. By spotting errors during development, teams can avoid costly fixes after a new app version is released. This can lower the cost of QA by as much as 30%.
Interestingly, these AI systems can also analyze how people use different localized versions of the app. This provides valuable information about which regions or languages need more attention, and allows developers to make adjustments that are specific to each user base. This data-driven approach can lead to better localization strategies overall.
Cloud-based features in these tools make it easy for several teams to work together on translation metrics in real time. This collaborative environment makes updating apps across different parts of the world more efficient, and reduces any conflicts or fragmentation in how content is updated.
Some more sophisticated AI tools can now analyze cultural context in real time, which is useful for identifying any problems that might hurt the user experience. This type of feature can help to avoid any cultural blunders in localized content, resulting in content that is better received by the target audience.
It's interesting to note that newer AI translation models are designed to constantly learn from new information. This means they can adapt to changing language trends and user preferences, something that's necessary for keeping the translations up-to-date and relevant.
Finally, having real-time quality metrics allows for detailed error reports after every app update. These reports can show the current problems, but also point out areas where the translation process can be improved in the future. These reports enhance localization efforts over the long term.
It seems the AI translation field has made significant strides with these new features. While there are still challenges, the ability to improve the quality of translations and provide near-instant feedback during development makes the whole process far more manageable and potentially more effective. It will be fascinating to observe the future evolution of these tools and their impact on the localization landscape.
AI-Powered PDF Translation now with improved handling of scanned contents, handwriting, charts, diagrams, tables and drawings. Fast, Cheap, and Accurate! (Get started for free)
More Posts from aitranslations.io: