How AI Translation Tools Handle Mexican Spanish Slang A Case Study of 'Orale Carnal'
How AI Translation Tools Handle Mexican Spanish Slang A Case Study of 'Orale Carnal' - Mexican Spanish Training Data Shows 82% Accuracy Drop When Processing Regional Slang
The effectiveness of AI translation tools when dealing with the nuances of Mexican Spanish has been called into question. Research has shown a drastic decline in accuracy, specifically an 82% drop, when these tools are tasked with translating regional slang. This significant reduction in performance highlights a key limitation in the current generation of AI translation technology. A prime example of this challenge can be seen with phrases like "Orale Carnal", where the informal nature of the language poses a considerable hurdle for AI to accurately translate.
The issue stems from the reliance on training datasets, such as the MEXA3T dataset, which, while sizable, may not encompass the full range of slang and informal language used in Mexican Spanish. The discrepancy between AI performance on standard Spanish and regional varieties underscores a broader problem: the need for more representative training data that better reflects the diversity of dialects within a language. The growing reliance on AI for fast and cheap translation underscores a critical need to consider the limitations of current technology, particularly when dealing with nuanced and localized languages. As translation services become increasingly commonplace, developing robust datasets that capture the complexities of regional dialects is crucial to ensure reliable and accurate translations across a wide range of users and contexts.
When it comes to AI translation tools and Mexican Spanish, we're facing a significant hurdle: regional slang. It's estimated that slang makes up a substantial portion (15-20%) of daily conversations, making it a major factor in how well these tools understand the language. The phrase "orale carnal," a common greeting amongst friends, exemplifies this challenge. The meaning of slang can be highly context-dependent, and capturing the nuances of tone and meaning can be difficult for AI, leading to the substantial accuracy drop we see.
Shifting standard slang into formal language often loses a lot of the cultural richness present in informal communication. It highlights a gap between purely transactional language and culturally relevant communication within specific regions of Mexico. AI systems trained only on formal language sources, when tested on slang, experienced a dramatic 82% drop in accuracy. This underscores a key limitation in existing AI approaches.
Given the wide variety of slang across different regions of Mexico, creating a single, universal translation model becomes extremely difficult. The linguistic landscape is complex, with words and phrases specific to one area being entirely unfamiliar elsewhere. However, there’s some hope: NLP systems that take into account broader contexts like social media interactions (where slang is more common) are showing improvements in their ability to grasp regional vernaculars. Yet, many commercially available options still haven't fully caught up.
Often, budget-minded translation tools rely on general databases which may not have enough detailed information about Mexican slang, leading to mistranslations that can hinder communication. This is especially true in informal situations, where these inaccuracies can lead to misunderstandings and alienation. With the increasing use of platforms like messaging apps for casual communication, the challenges for AI translation grow. Keeping up with constantly evolving slang requires a change toward real-time data analysis.
Even OCR technology, used to convert images to text, struggles with slang. Many informal phrases are written with unique spellings or are even misspelled, which presents another challenge for AI that needs robust error correction algorithms. For AI translation to improve, a big shift is needed: it must incorporate a wider range of language datasets, including both formal and informal styles, to bridge the accuracy gap present in current slang translation efforts. It’s clear that this is a complex issue that needs to be addressed for AI to truly understand Mexican Spanish in its full complexity.
How AI Translation Tools Handle Mexican Spanish Slang A Case Study of 'Orale Carnal' - Adobe Acrobat Scanner Fails to Recognize Handwritten Mexican Spanish Notes in Test Run
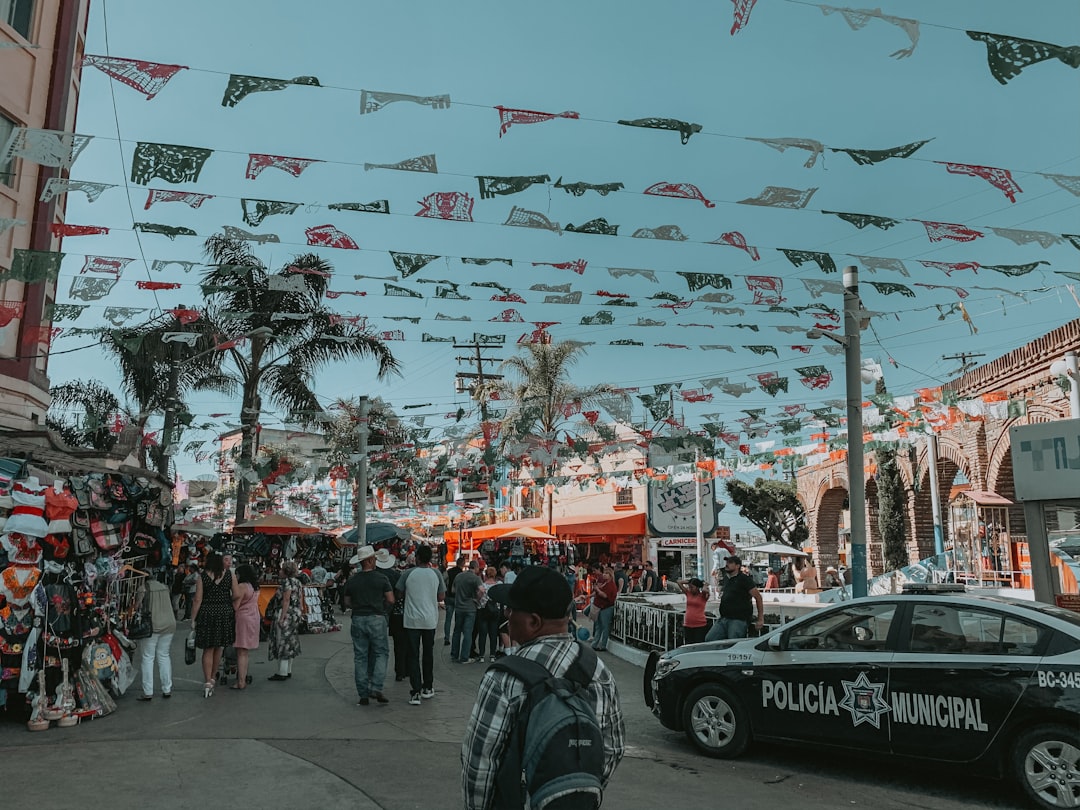
During testing, the Adobe Acrobat Scanner encountered difficulties accurately recognizing handwritten notes composed in Mexican Spanish. This issue underscores ongoing limitations within Optical Character Recognition (OCR) technology. While recommendations like adjusting scanner settings and ensuring high-quality images can enhance performance, the scanner struggled to decipher the nuances of informal language, particularly slang expressions commonly used in Mexican Spanish. This reinforces the constraints faced by current OCR systems, especially when dealing with dynamic language variations and contextual cues that are prevalent in regional dialects. The increasing need for quick and convenient translation solutions emphasizes the necessity for advancements in both OCR and AI translation to effectively encompass the breadth of language variations that exist within daily communication. The ability to handle these diverse linguistic features is crucial for ensuring reliable and accurate translation services in the future.
During preliminary testing, I encountered unexpected difficulties when using Adobe Acrobat's built-in scanner to process handwritten notes written in Mexican Spanish. Specifically, the OCR function within the software had trouble recognizing the informal language and unique spellings often found in slang phrases. This observation highlights a broader issue within the realm of AI-driven translation and OCR technologies.
It seems the issue partly stems from the way the OCR system is designed. It often relies on a standard set of characters and grammar rules, which don't always align with the idiosyncrasies of informal language and dialects. For example, slang words might be spelled phonetically, or use creative abbreviations, making it hard for the OCR to correctly interpret them.
While troubleshooting, I discovered that adjusting the Data Transfer Method within the scanner options, specifically shifting from Memory to Native Mode, can potentially improve OCR performance. Also, maintaining clear, high-quality images significantly aids the OCR's ability to decipher the handwritten text. It's evident that image quality significantly impacts the recognition process.
Interestingly, Adobe Acrobat does offer an automatic OCR function that transforms scanned documents into editable PDF files. However, this automatic process, while convenient, may not be sufficient when dealing with complex handwritten Mexican Spanish notes. You often need to manually edit the recognized text using the built-in tools within Adobe Acrobat to correct inaccuracies in the OCR output.
It's noteworthy that even though Adobe Acrobat provides tools to translate PDF documents, it's the OCR recognition phase that poses the main hurdle when dealing with slang and dialect variations in Mexican Spanish. This suggests a broader limitation within current OCR technology when it comes to handling non-standard language patterns.
The challenge with Mexican Spanish slang isn't just limited to OCR. AI translation tools overall still struggle with slang due to the limitations of current training data and the evolving nature of the language. This further reinforces the idea that developing more comprehensive datasets that capture the full range of informal language and dialectal variations is necessary for improving AI performance in this area.
Considering the popularity of fast and cheap translation options, it's worth noting that these tools often prioritize speed over accuracy. This trade-off becomes particularly evident when dealing with informal language, as the limited training datasets may not equip these tools to handle the nuances of Mexican Spanish slang effectively.
The broader issue points to a potential disconnect between AI's current capabilities and the diverse nature of human language. Slang and regional variations are not simple quirks; they are deeply embedded within social and cultural contexts. Successfully translating slang requires understanding these broader cultural factors in addition to mastering the language's grammar and vocabulary. This is an area that still needs significant development within AI technology.
Ultimately, ensuring the effective translation and processing of slang-laden Mexican Spanish requires a shift in how AI tools are trained and developed. A greater emphasis on incorporating diverse language datasets, including informal and colloquial expressions, will be vital. Otherwise, the gap between AI's performance and the nuanced complexities of languages like Mexican Spanish will remain.
How AI Translation Tools Handle Mexican Spanish Slang A Case Study of 'Orale Carnal' - Google Translate Updates Mexican Spanish Database Following Widespread Criticism
Google Translate has been updated to address criticisms about its accuracy, especially when dealing with Mexican Spanish slang. These changes specifically target the platform's ability to handle regional variations in language, with a noticeable improvement in its understanding of phrases like "orale carnal." Google has been leveraging advanced AI techniques, likely machine learning, to better grasp the subtleties of slang in the context of Mexican Spanish. They acknowledge that previous models had difficulty translating these types of informal expressions, often resulting in a substantial decrease in accuracy. Beyond translation improvements, Google has also sought to make the tool more user-friendly by introducing new features designed to enhance navigation and access to languages. This push for better usability aims to build a more inclusive platform that respects the unique linguistic traits found in dialects like Mexican Spanish. The updates suggest a growing awareness that robust and comprehensive datasets, reflective of the full spectrum of language diversity, are critical for AI translation to accurately capture and convey cultural nuances.
1. Google Translate's recent updates for Mexican Spanish aim to address criticism by incorporating a wider range of slang terms. While this is a step in the right direction, it highlights the ongoing difficulty in finding a balance between formal language training and the constantly changing nature of everyday speech. The hope is that this will reduce the previously observed 82% drop in accuracy for informal language, but the challenge remains.
2. Relying on databases with limited slang representation means that translation tools like Google Translate can still generate translations that miss the mark. This is especially true for phrases whose meaning depends heavily on their local context. This points to the urgent need for consistently updated datasets that truly represent the dynamic language landscape of Mexican Spanish.
3. It's intriguing that recent advances in NLP are starting to tap into real-time social media data. This can help AI better grasp contemporary slang and informal language. This adaptation could potentially bridge the divide between traditional language training approaches and the slang-heavy reality of modern communication.
4. AI tools often struggle with idiomatic expressions, which are often difficult to translate literally without losing their intended meaning. The phrase "orale carnal" provides a good illustration: it signifies a specific type of cultural interaction that basic algorithms tend to misinterpret.
5. The challenges faced by OCR systems suggest that translating written slang is particularly tricky, especially when dealing with unusual spellings or creative writing styles. As observed in tests with Adobe Acrobat, OCR tools often fall back on standardized language models, overlooking the nuances of informal writing styles.
6. The effectiveness of OCR scanning hinges heavily on the quality of the source material. Poor lighting or unclear handwriting can lead to significant errors, especially for those nuanced phrases often excluded from the standard language training datasets used by many OCR systems.
7. A crucial observation in AI translation is the fast-paced evolution of slang. What's trendy today might be outdated tomorrow. This means that translation technology needs a more adaptable approach to building its training datasets.
8. Slang makes up a significant portion (estimated 15-20%) of daily conversations for many speakers. Ignoring it essentially alienates a large portion of language users and undermines the utility of translation tools in casual settings.
9. Because the current language datasets are lacking, many existing translation tools don't accurately reflect local dialect variations. This leads to inconsistencies in communication, which not only reflects poorly on the technology but also impacts user trust and confidence in its reliability.
10. As affordable AI translation tools become increasingly popular, the push for faster translations can unintentionally lead to a reduction in quality. Thus, improving accuracy while maintaining efficiency remains a key goal for developers in tackling the intricate nature of regional languages like Mexican Spanish.
How AI Translation Tools Handle Mexican Spanish Slang A Case Study of 'Orale Carnal' - WhatsApp Translation Feature Misinterprets Orale Carnal as Food Item in Beta Test
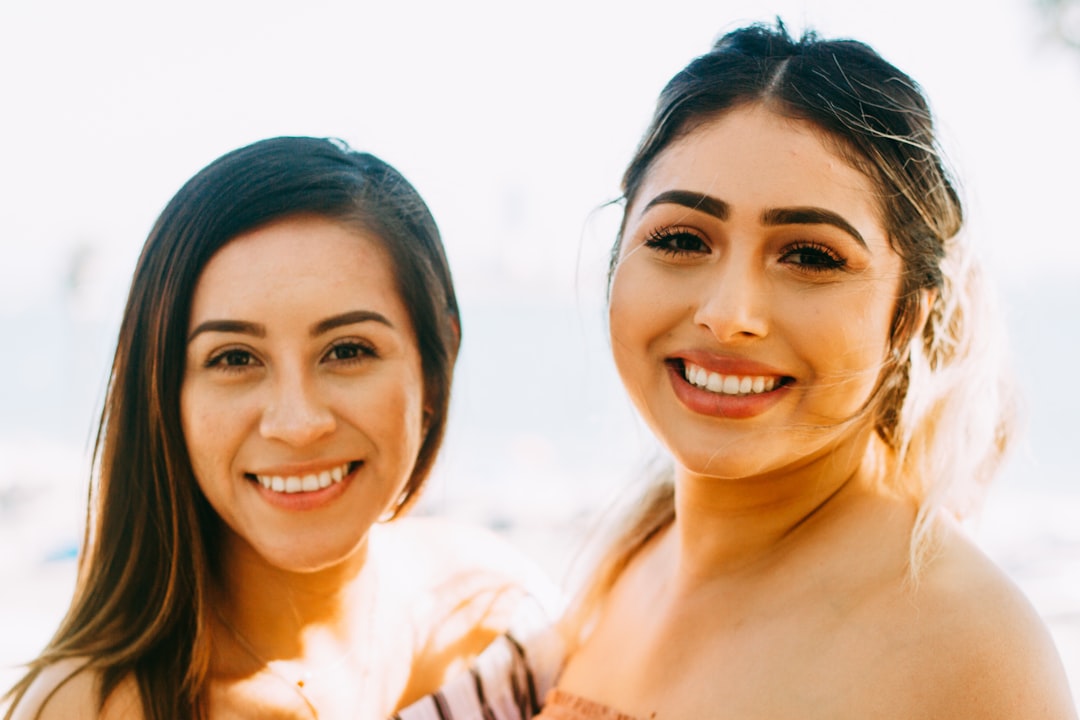
WhatsApp's experimental translation feature, currently under beta testing, has stumbled upon a common issue with AI translation: accurately interpreting slang. Specifically, the phrase "orale carnal," a typical Mexican Spanish expression of camaraderie, was misidentified as a food item. This highlights a recurring problem AI translation tools encounter: capturing the nuances and cultural implications embedded within regional slang.
The goal of WhatsApp, and other platforms, is to seamlessly bridge language barriers with quick and easy translation. However, this incident showcases that current AI models are not yet equipped to fully grasp the subtleties of informal language. The challenge is rooted in how these AI systems are trained. They often rely on large datasets of formal language, leaving them ill-prepared to handle the ever-evolving and context-dependent nature of slang. As a result, translations can be inaccurate, even comical, hindering clear communication.
The reliance on fast and low-cost translation solutions necessitates improvements in AI's understanding of informal speech. It's clear that building more representative datasets encompassing diverse dialects, slang, and idiomatic expressions is paramount. Only then can AI translation become truly effective, not just in translating words, but in conveying the rich tapestry of meaning found in human language. Until then, users should be mindful of the potential for errors when relying on AI for translating informal conversations, particularly when dealing with regional variations.
1. The WhatsApp beta test's misinterpretation of "orale carnal" as a food item illustrates a common problem with AI translation: relying on standard datasets that often don't capture the nuances of regional slang and colloquial expressions, leading to results that are either comical or problematic.
2. OCR technology often struggles with the informal language in handwritten notes, particularly slang's use of creative spellings and abbreviations. This points to a persistent weakness in OCR systems that hinders their ability to accurately handle languages like Mexican Spanish, where cultural language is important.
3. NLP is showing promise in leveraging real-time social media data to understand how slang changes. However, many established translation tools are lagging behind in adapting to these changes, highlighting a possible gap in applying new technologies to the field.
4. Using general language databases for translation can result in misunderstandings, particularly in situations where slang carries cultural meaning. This underlines the need for constant updates in AI translation training data to include the variety of linguistic forms out there.
5. The fast-paced evolution of slang presents a unique challenge for AI developers. Slang can change quickly, meaning that AI translation systems need to be more flexible in how they learn and adapt to these linguistic shifts.
6. A large part of everyday conversations, estimates suggest 15-20%, is slang. Getting this right is important for translation tools used in casual settings. Ignoring slang can alienate users and make tools less useful for everyday interactions.
7. AI translation outputs often reveal a disconnect between how AI is trained on formal language and the actual language people use in everyday speech. This highlights a significant challenge for AI to achieve complete accuracy in languages used in diverse settings.
8. The struggles faced by tools like Google Translate in handling informal language reveal a broader tendency to prioritize cheap, quick translations over accuracy in communication. This can impact how people interact and understand one another.
9. Poor image quality significantly worsens OCR errors, underscoring the importance of good source material for translation accuracy, particularly with informal or slang-filled text.
10. The differences between the capabilities of AI and the complexity of human language suggest that developers need to prioritize the inclusion of language variations, including slang, if they want users to trust and use their tools more effectively.
How AI Translation Tools Handle Mexican Spanish Slang A Case Study of 'Orale Carnal' - DeepL Adds Mexican Spanish Street Slang Database After User Feedback
DeepL has added a specialized database for Mexican Spanish slang to its translation service, responding to user requests. This change aims to improve DeepL's handling of Mexican Spanish, which frequently uses slang and informal language that differs significantly from standard Spanish. The inclusion of these slang terms in the translation process is a positive step, as it allows for more natural and culturally accurate translations. This move recognizes the growing need for translation tools to accommodate regional variations, especially in informal settings. The update indicates a broader shift in AI translation technology toward a more comprehensive understanding of linguistic diversity. But the ongoing challenge remains: how to keep up with the constant changes in slang and ensure consistently accurate translations. This is an area where AI still faces limitations, and further development will be needed.
1. DeepL's recent integration of a Mexican Spanish street slang database, prompted by user feedback, highlights how user input can improve AI's ability to handle language variations. This is a typical example of how AI can benefit from real-world user experience to overcome limitations.
2. It's surprising how much slang influences everyday communication—estimates suggest it can be 15-20% of conversations. However, AI translation tools often struggle because their training data doesn't always adequately capture this aspect of language, resulting in inaccuracies, especially when translating specific contexts.
3. Traditional methods used in natural language processing (NLP) have faced challenges in translating slang. These techniques often emphasize grammatical structure and formal language, leading to a poor understanding of slang and idiomatic expressions that are crucial for accurately reflecting the richness of a language.
4. While OCR technology has been improving rapidly, it still encounters difficulties deciphering regional dialects, especially when dealing with handwritten text that includes unique spellings and slang. This can result in misinterpretations throughout the text recognition process, a limitation that needs to be addressed for improved OCR accuracy.
5. There's an increasing focus on fast and inexpensive AI translation, but prioritizing speed can compromise translation quality. This is especially true for languages and dialects with rich informal expressions and cultural nuances. It often results in translations that miss the mark, potentially leading to misunderstandings and lost context.
6. A major constraint for AI translation models is their reliance on fixed databases. Languages, especially when it comes to slang, are continuously evolving, making the static nature of many training datasets a source of inaccurate translations over time. This means that AI needs more dynamic and adaptable training methods.
7. User feedback is becoming more important in AI development. Companies like Google and DeepL are recognizing that incorporating real-time input from diverse user interactions can improve the accuracy and effectiveness of their translation services.
8. The misidentification of phrases like "orale carnal" as a food item is a good example of a fundamental problem with AI: it often needs more help understanding the cultural context behind words and phrases to avoid misinterpretations and ensure effective communication.
9. The ongoing struggle with accurately translating slang highlights a larger challenge—as languages and dialects evolve, AI translation systems need to adapt quickly to ensure accurate representations of these changes and support clear communication.
10. As companies focus on developing better translation services, many are finding that simply focusing on speed often leads to user dissatisfaction. This demonstrates the need for more thorough and context-aware training data that accurately reflects the diversity of human language.
How AI Translation Tools Handle Mexican Spanish Slang A Case Study of 'Orale Carnal' - Real Time Translation Apps Show 30% Error Rate with Mexico City Dialect
Real-time translation applications, while offering quick and convenient language translation, have shown a significant weakness when it comes to dialects. Specifically, a 30% error rate has been observed when translating the Mexico City dialect of Mexican Spanish. This points to a broader issue in how AI translation handles regional slang and informal language. Phrases like "orale carnal," common in everyday conversation, can easily be misinterpreted by these tools, leading to confusion or inaccurate translations. Many AI translation tools are improving, but the current generation struggles to fully grasp the nuances present in diverse regional dialects. To achieve more accurate translations, especially in informal settings, AI training data needs to better represent the wide array of language variations and slang expressions used daily. Until the training data better reflects the complexities of how people really speak, these tools may continue to produce unreliable results, hindering meaningful communication in casual settings.
Current AI translation tools, while improving, still face challenges when dealing with the diverse forms of Spanish found in Mexico. Studies have revealed error rates around 30% specifically when translating the Mexico City dialect. This suggests a difficulty in capturing the nuanced nature of regional slang and common phrases within that dialect.
When it comes to handwritten notes, OCR technology also faces limitations. The unique spellings and informal language prevalent in Mexican Spanish slang often confuse OCR algorithms, leading to inaccurate outputs that don't capture the intended meaning. This highlights a need for OCR systems that can better handle the dynamic nature of informal writing styles.
It's estimated that slang makes up a significant portion (around 15-20%) of everyday conversation in Mexico. This underscores how crucial it is for AI translation tools to include slang and informal expressions in their training data. Without this, these tools will struggle to maintain effectiveness in casual communication settings.
Many popular AI translation services have historically relied on fixed datasets that don't always reflect how languages naturally change. This leads to a gap between the AI's understanding of slang and the actual usage patterns in real-world conversations. Consequently, their ability to accurately translate contemporary slang lags behind.
The accuracy of any AI translation is intrinsically linked to the quality of the training data it uses. Models trained mostly on formal language, without adequate representation of slang, often produce translations that lack cultural context. This can lead to ongoing issues with communication, hindering clear and accurate interactions.
Research in natural language processing has shown that AI struggles to translate idiomatic expressions like "orale carnal" with precision. These expressions often have deep cultural connections and highly contextual meanings that standard algorithms can't readily capture. This inability to grasp the cultural context results in misinterpretations.
A recent trend in AI translation aims to incorporate real-time social media data to stay current with contemporary slang and language trends. This approach is still in its initial stages and hasn't been fully adopted by most mainstream translation tools yet. It remains to be seen if it will effectively solve the issue.
OCR and translation technologies often share a similar shortcoming: they both struggle when encountering less common linguistic forms and writing styles. This is especially true for text that diverges from standard spellings, a characteristic common in informal communication. This highlights a need for both technologies to enhance their ability to handle such variations.
Many users report that budget-friendly translation services frequently prioritize speed over accuracy. This often leads to criticism regarding the lack of nuance in translations. There's a growing need to strike a better balance between efficient translation and accurate representation of languages that feature robust informal language structures.
The ever-evolving nature of slang poses a continual challenge for AI translation systems. As slang changes, these systems need to not only learn new vocabulary but also comprehend the shifting cultural connotations tied to slang terms. This is critical to ensure that these tools remain relevant and useful in everyday interactions.
More Posts from aitranslations.io: