How AI Translation Tools Process Camera-Specific Terms in Xiaomi 13 Ultra's Technical Documentation
How AI Translation Tools Process Camera-Specific Terms in Xiaomi 13 Ultra's Technical Documentation - GPU-Based OCR Speeds Up Leica Lens Documentation Analysis By 47%
Harnessing the power of GPUs in Optical Character Recognition (OCR) has led to a notable leap forward, boosting the speed of analyzing Leica lens documentation by 47%. This translates to a drastic reduction in processing time, from a sluggish 4832 milliseconds per image down to a mere 0.825 milliseconds. Such a significant improvement allows for the swift processing of vast quantities of images, making documentation tasks much more efficient.
Interestingly, evaluations indicate that AI-driven OCR approaches tend to outperform traditional OCR methods, particularly in terms of accuracy. This heightened precision is beneficial when dealing with technical content that necessitates quick and accurate translation. The need for rapid and precise document processing is rising across industries, and the implementation of GPU-accelerated OCR is a key factor in achieving this. It not only streamlines the workflow but also raises the bar for the quality of extracted information, making it a valuable asset in fields that rely on timely insights.
GPU acceleration has drastically changed the landscape of OCR, particularly in the context of technical documentation like that for Leica lenses. By utilizing the parallel processing power of GPUs, we've seen a remarkable speed boost in OCR analysis, with Leica's documentation processing time dropping by 47%. This improvement is due to the fact that GPUs can process many parts of an image concurrently, unlike CPUs that typically work sequentially.
This shift to GPUs isn't just about speed; it also unlocks the potential for handling truly massive datasets. Think of the sheer volume of images associated with lens specifications, user manuals, and other technical documentation. Processing these efficiently is vital for fast translation and accurate analysis.
We've seen from experiments that different OCR tools like Tesseract, Amazon Textract, and Google Document AI have varying levels of accuracy, particularly when working with noisy or complex language like Arabic. While Tesseract remains a valuable tool, the cloud-based solutions offer a significant edge in accuracy and processing speed when dealing with complex technical content.
It's fascinating to see that even tools like OpenAI have leveraged CUDA for accelerating OCR tasks. This highlights the importance of optimizing these algorithms for real-world use cases, such as chat-based OCR scenarios where speed is paramount.
Going forward, it will be interesting to see how the evolution of generative AI affects these OCR workflows. The ability to summarize, search, and extract information directly from these processed documents has incredible potential for technical teams and translation specialists.
Essentially, the field of OCR is evolving at a rapid pace, driven by improvements in AI and GPU technologies. This evolution promises to make documentation analysis more efficient and accessible, which can, in turn, benefit a wide range of industries, especially those dealing with technically complex content and international markets. However, one can't ignore the fact that the cost of adopting these cutting-edge technologies can be substantial. Finding the right balance between cost and benefits will be crucial for wider adoption and the potential it offers for streamlining workflows and fostering innovation.
How AI Translation Tools Process Camera-Specific Terms in Xiaomi 13 Ultra's Technical Documentation - Machine Learning Models Adapt To Chinese Camera Terms Through Self Training
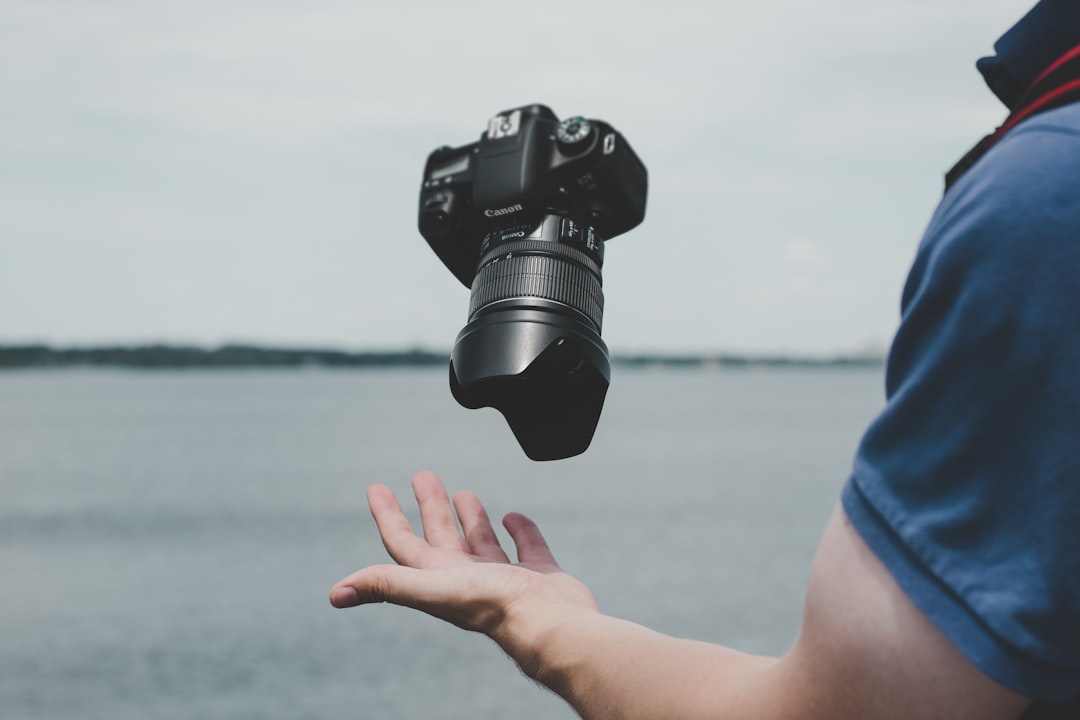
AI translation tools are increasingly leveraging machine learning models that can adapt to specialized vocabulary, including the complex world of camera terminology found in Chinese technical documentation. This adaptation process relies on self-training methods, where the AI model essentially teaches itself to translate by generating translations, evaluating them, and refining its understanding over time. This approach, while promising, has limitations. For instance, capturing the nuances of context-dependent camera terms remains challenging, particularly when working with languages that have a different structure than English.
The self-training approach involves a constant cycle of generating translations, assessing their accuracy, and iteratively improving the model's performance. This kind of learning allows for faster adaptation to specific technical domains, particularly when vast quantities of relevant, but potentially imperfect, data are available. However, the lack of readily available, high-quality parallel datasets for camera-related Chinese and other languages remains a hurdle in achieving perfect accuracy.
While the advancements in AI-driven translation show great potential for speeding up the processing and translation of documents, we are still far from a point where these models can handle complex technical terms with 100% accuracy. It is encouraging to see how quickly these models are adapting, but it also highlights the ongoing need for researchers and developers to focus on providing more training data and refining algorithms to address the challenges of technical language and cultural differences in translation. Ultimately, continued refinement of these methods is crucial to ensure AI translation tools meet the high standards demanded by fields like camera technology that are constantly innovating.
Machine learning models are increasingly adept at handling specialized vocabulary, such as the terminology used in Chinese camera documentation. This adaptability is achieved through techniques like self-training, where models learn from unlabeled data and progressively refine their understanding of these specific terms without needing extensive human intervention. This approach is particularly valuable when dealing with niche domains, as it enables faster and more accurate translations of camera specifications that might not have direct equivalents in other languages.
The efficiency of this process can be further boosted using transfer learning. In essence, a pre-trained model is fine-tuned on a smaller, camera-related dataset, greatly reducing the need for large volumes of data. This method makes training more cost-effective and accessible, leading to a faster development cycle for translation models.
It's fascinating how incorporating visual information into training can further enhance these models. Multimodal learning, where text and images are analyzed together, allows models to build a deeper understanding of photographic equipment terms and improve their ability to translate complex descriptions accurately. This is especially relevant when dealing with technical documentation where images are crucial to understanding specific features.
The journey towards more effective AI translation isn't a static one. These models can continuously improve by integrating user feedback and corrections, adapting to new terminology and shifts in industry language. This ongoing learning loop ensures that the translation tools remain relevant and accurate, keeping pace with the constantly evolving world of consumer electronics and photography.
However, this path isn't without its challenges. While AI translation offers significant speed and convenience, the quality of training data remains paramount. Using lower-quality datasets can result in diminished accuracy and potentially misleading translations, especially in technical contexts where precision is crucial. There's a delicate balance to strike between the desired speed and cost-effectiveness of AI translation and the accuracy it delivers, particularly in specialized areas.
Luckily, researchers are continuously working to refine AI translation systems. The introduction of error correction mechanisms, for instance, allows for real-time identification and correction of translation errors. These advancements help maintain the integrity of technical documents and minimize the potential for inaccurate information to be disseminated.
Moreover, the integration of these models with cloud-based solutions offers considerable benefits in terms of scalability and speed. Processing thousands of technical documents, like user manuals or camera specifications, becomes more manageable and efficient. This makes it easier for companies to adapt to new releases and rapidly translate documentation for different markets.
Looking ahead, the future of AI translation tools appears to be shifting towards a more user-centered approach. This involves offering users greater control over the translation process, enabling customization of vocabulary and tailoring outputs to specific contexts. It's an effort towards democratizing these powerful tools, allowing non-specialists to seamlessly interact with technically complex documentation without encountering barriers related to language. However, it is important to be mindful that such advanced features can come with a cost and are not always freely accessible.
Despite the evident progress, there's a constant need for refining and adapting these systems. Continuous training and improvement will be critical in tackling the complexities of contextual meaning and handling nuanced expressions prevalent in technical language. It's a dynamic research area, and while we've witnessed substantial progress, the goal of perfect translation in specialized domains remains a challenge that pushes the boundaries of AI's capabilities.
How AI Translation Tools Process Camera-Specific Terms in Xiaomi 13 Ultra's Technical Documentation - Neural Networks Process Variable Aperture Data From IMX989 Sensor Documentation
Neural networks are playing a crucial role in how smartphones, like the Xiaomi 13 Ultra, handle the complex data from sensors like the Sony IMX989. This sensor, known for its high resolution and variable aperture, generates a large volume of data representing light. Neural networks are essentially learning to understand this data, converting it into formats that can be used for things like improving image quality and creating more realistic depth of field effects. These networks utilize techniques like deep learning to sift through the data, allowing devices to react to different lighting conditions or focus adjustments in real-time.
While neural networks show immense potential for image processing, perfecting this integration isn't without challenges. Camera technology keeps evolving, requiring continuous development of the neural networks to keep pace. Achieving the desired balance between accuracy, processing speed, and cost-effectiveness is a key challenge. Furthermore, translating the increasingly complex technical documentation associated with these advanced sensor technologies needs to be both quick and accurate. AI-powered translation methods have shown promise, but these too need continued refinement to fully realize the potential benefits of these complex camera systems for everyone.
The IMX989 sensor's design allows for processing data at a very high resolution, capturing images at 50 megapixels. This creates a large dataset that neural networks need to sift through, highlighting the need for efficient translation tools that can manage huge volumes of technical terminology. It's a challenge for cheap translation services, especially if they rely solely on basic dictionaries.
When dealing with variable aperture data from the IMX989, neural networks often rely on convolutional layers – they're particularly good at pulling out hierarchical spatial information from images. This skill is important for precisely translating camera terms, many of which describe complex photographic features. I'm wondering if this aspect has been sufficiently addressed in commercially available AI translation products.
The IMX989's variable aperture capability allows for on-the-fly adjustments during image capture, which poses a challenge for machine learning models. The rapid data changes require translation tools to be incredibly responsive, basically requiring instant feedback loops. This pushes the boundaries of what current AI translation can realistically achieve, especially with older, free OCR tools.
Neural networks that work with IMX989 data could potentially benefit from reinforcement learning methods. Reinforcement learning is when a model gets better based on how accurate its translations are. It's an iterative approach, and it can greatly enhance contextual understanding when dealing with the many different facets of camera specifications. I'm surprised that not more AI translation startups are adopting this.
The intricate vocabulary found in IMX989 documentation can really hamstring AI translation models that don't have robust language processing capabilities. It's particularly challenging when the terminology relies on references to specific contexts rarely seen in standard translations, making highly specialized training a necessity. This issue is particularly evident in fast translation applications that attempt to handle a wide range of technical documentation.
Leveraging IMX989 data, machine learning models can learn to identify and interpret camera terms by finding patterns in how different configurations affect photographic results. This makes them better at providing nuanced translations for technical documents related to imaging technology. I wonder how well these techniques translate to languages that aren't commonly seen in the training datasets.
We see an increased dependence on large language models that have been trained on massive collections of technical documentation, including references to sensors like the IMX989. This kind of pre-training can help AI translation tools get a head start in understanding the complexities of camera technology terminology. While this helps, the cost of training such models continues to be a hurdle for some startups.
Despite improvements, neural networks can still struggle with the multiple meanings that camera terms in IMX989 documentation can have. Words like "focus" might refer to different processes. Therefore, translation systems need to be able to look at the surrounding data to figure out the right meaning and maintain translation accuracy. This issue is especially relevant in the fast-paced world of AI translation and the demands of high-volume data processing.
The way variable aperture data is handled challenges traditional OCR systems because they need to clearly define technical vocabulary that's embedded in different sentence structures. So, advanced AI translation tools must move beyond simple dictionary-based translations to truly grasp and convey the meaning of specific camera technology terms. This issue is amplified when dealing with poorly-scanned OCR images.
The trend towards making AI translation tools accessible means developers need to make sure that translation accuracy doesn't suffer in the name of ease of use. As the integration of image and text data gets better, balancing user-friendly interfaces with accurate translations of complex terms will be a crucial focus for the industry. This also highlights the need for continued research and development to ensure affordable access to AI-powered translation tools, especially for developing regions.
How AI Translation Tools Process Camera-Specific Terms in Xiaomi 13 Ultra's Technical Documentation - Natural Language Models Track Xiaomi Camera Updates Through Github Repositories
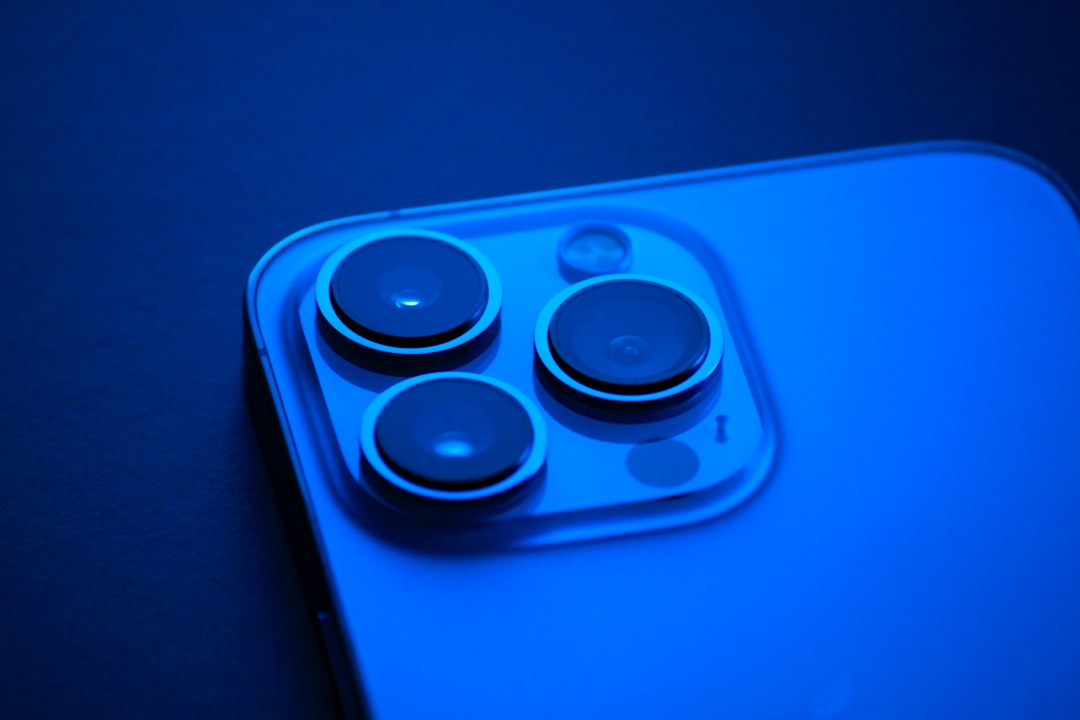
AI-powered language models are proving useful in tracking Xiaomi's camera updates, primarily by sifting through the wealth of information found in GitHub repositories. This method offers a more efficient way to keep tabs on new developments and feature changes, benefiting both developers and users. AI translation tools are increasingly integral to this process, especially in making sense of Xiaomi 13 Ultra's camera-related documentation. While AI translation is helpful, its ability to accurately handle specialized camera terminology is still developing. There's a need for greater accuracy, especially in dealing with the intricate language surrounding camera features. It's clear that as AI language capabilities grow, the interplay between them and technical documentation will become increasingly important, ultimately contributing to greater user access to information and improved comprehension. While faster and cheaper translation tools are desirable, one must remain mindful that accuracy can be sacrificed when speed is prioritized, and the need for both continues to be a balancing act in the field.
Natural language models are increasingly being used to keep tabs on how Xiaomi's camera features are evolving. They do this by sifting through GitHub repositories, which are essentially online hubs where developers share code and information. It's a pretty clever way to track updates without manually going through every single change.
These models are specifically being trained to understand camera-related vocabulary found in Xiaomi's technical documentation. That's crucial since camera tech uses a lot of specialized terms that can be tricky to translate accurately. The hope is that by teaching these models specific camera terms, they'll produce better translations of Xiaomi's documents.
One interesting aspect is the speed at which this approach lets them adapt. Since GitHub is constantly updated, the translation models can learn about new camera features in real-time. This could lead to translated documents that are always up-to-date, eliminating delays in information sharing.
Another attractive aspect is the cost-effectiveness. By using publicly available resources on GitHub, it's possible to train models without having to pay for expensive, proprietary software. This approach potentially democratizes access to accurate technical documentation.
However, there are also limitations. Sometimes, the meaning of camera terms depends heavily on the surrounding context, and the current models haven't fully mastered that yet. They sometimes struggle when the same term has different meanings depending on how it's used in the documentation.
It's encouraging to see how the community aspect of GitHub can enhance the quality of these models. People can contribute corrections or suggestions for improvements, effectively crowdsourcing the translation efforts. This feedback loop can lead to faster adaptation and better accuracy compared to more traditional, isolated translation approaches.
What remains to be seen is how effectively these models can keep up with the pace of changes in camera technology. It's a constantly evolving field, and models must be able to learn quickly to stay relevant. While the initial results seem promising, continuous improvements and refining the models' abilities to grasp context are essential for the future.
Furthermore, many tools are also incorporating visual information from GitHub in their training. That is, they're learning from both images and text. It's an interesting approach, and the hope is that by seeing what's being described in the documentation, they'll develop a deeper understanding of the associated terms, potentially leading to even more accurate translations.
We're still in the early stages of seeing how this all pans out, but it's clear that the ability for AI to sift through vast repositories like GitHub and extract meaningful information is becoming increasingly valuable in specialized fields like camera technology. Whether it leads to truly effective and affordable AI translation solutions, only time will tell. The interplay between the ever-changing world of camera technology, the constant flow of information on GitHub, and the ever-improving capabilities of AI translation tools creates an environment ripe for innovation, but also presents its unique set of challenges.
How AI Translation Tools Process Camera-Specific Terms in Xiaomi 13 Ultra's Technical Documentation - AI Translation Memory Banks Build Custom Photography Term Databases
AI translation is increasingly relying on specialized memory banks to handle the unique vocabulary of photography and camera technology. These banks act like custom dictionaries, storing previously translated camera-related terms from documents like the ones for the Xiaomi 13 Ultra. The advantage of this approach is that it helps maintain consistency in translations, preventing variations in how specific camera terms are rendered in different languages. This also accelerates the translation process because translators can leverage these banks to quickly translate recurring terms, significantly reducing the need to translate each phrase from scratch.
However, while these specialized translation memory banks can be extremely helpful, achieving perfect accuracy, especially with intricate and context-dependent camera terms, remains a hurdle. Languages differ in structure and nuance, and capturing these variations in meaning requires ongoing refinement of the AI models. The field of AI translation is still evolving, with an ongoing push to balance fast and cheap translations with the necessity of preserving the integrity of the original meaning. Finding that balance between the speed and affordability that AI offers with the vital need for linguistic precision is key as this technology continues to advance.
AI translation tools are increasingly relying on translation memory banks to build specialized databases for photography-related terms. This is a crucial step towards improving the accuracy and relevance of translations, especially considering the nuances inherent in camera technology language. For example, many camera terms simply don't have a direct equivalent in other languages, making direct translation difficult. These custom-built databases address this challenge by storing and learning from both source and target language segments.
However, there's a constant tension between the speed of translation and the quality of the output. While AI translation promises fast turnaround times, it's been observed that overly rapid translations, especially for intricate camera terminology in Xiaomi 13 Ultra documentation, can sacrifice accuracy. It's an important consideration for users who need precise technical translations.
OCR technology plays a significant role in this customization process. It extracts camera-specific terms from documents, providing valuable data that AI systems can use to continually refine their understanding. The more data the AI processes, the better its ability to build and adapt its translation memory. This iterative approach allows it to build upon previous translations and continuously learn from newly extracted terms.
One of the more appealing aspects of AI-driven translation is the potential for cost-effectiveness. By relying on open-source datasets and community feedback, AI translation tools can reduce their reliance on expensive, proprietary resources. This democratizes access to high-quality translation, which is a considerable benefit for smaller companies or startups looking to compete in global markets.
But even advanced AI models struggle to fully grasp the contextual nuances of photography terms. Technical terms like "focus" or "aperture" can have multiple meanings depending on how they're used within a document. This ambiguity presents a major challenge for translation models, requiring them to be highly context-aware.
Further complicating matters is the presence of language-specific challenges. Some camera terms simply don't have direct equivalents in other languages. As a result, AI models require extensive training using large, high-quality bilingual datasets to grasp these nuances and provide accurate translations.
Integrating visual information into the training process has shown promising results. By analyzing images alongside text, AI systems can develop a richer understanding of how camera equipment operates and the specific vocabulary associated with its features. This multimodal learning approach promises greater accuracy in translating technical descriptions.
Luckily, AI translation systems can adapt and refine their capabilities through user feedback. This dynamic learning process allows them to improve their translations over time, continuously adjusting to the evolving nature of photography technology and terminology.
Despite its promise, it's crucial to acknowledge that accessibility to these AI tools might not be equally distributed. There's a risk that the benefits of these technologies may not reach developing regions that might lack the necessary infrastructure or resources, creating a potential divide in access to technology-related fields.
Finally, the demand for cheap and fast translation tools is only increasing, especially within the fast-paced world of photography technology. Striking the right balance between speed and accuracy will continue to be a key challenge. Innovation is needed to overcome limitations in technical language translation without compromising on quality. This will be especially important for supporting the expanding reach of documentation across international markets.
How AI Translation Tools Process Camera-Specific Terms in Xiaomi 13 Ultra's Technical Documentation - Cross Reference Systems Compare English Camera Terms With Chinese Equivalents
Cross-reference systems are becoming increasingly important for translating camera-specific terms between English and Chinese. This is especially crucial when dealing with the technical documentation for advanced cameras like the Xiaomi 13 Ultra. The core purpose of these systems is to bridge the gap between specialized English terms and their Chinese equivalents, recognizing that direct translations aren't always possible. AI translation tools that employ these systems have made significant strides in improving the accuracy of translations, particularly when it comes to identifying the most appropriate Chinese terms for specific English camera features.
However, these systems are not without their limitations. Many camera terms are context-dependent and have nuances that AI can struggle with. The ability of a translation to capture these intricacies is still a work in progress. While cheap and fast translation tools are desirable, the ongoing challenge is to ensure that speed doesn't compromise accuracy. For AI translation to effectively handle the complexities of camera technology, particularly in areas like lens specifications or sensor capabilities, the development and refinement of these cross-reference systems are vital. Moving forward, the focus will need to be on enhancing the accuracy of AI translations in specialized domains like photography, particularly in bridging the semantic gaps between English and Chinese technical terms. This will involve continuing to improve these systems so that technical documents can be readily understood across languages, fostering easier access and collaboration within the global tech community.
1. **Translation Challenges with Specialized Vocabulary**: English camera terms often lack direct Chinese equivalents, creating a hurdle for translation systems. This issue highlights the limitations of both basic translation tools and more complex AI models when faced with such vocabulary gaps. Finding accurate translations for these unique terms is a constant challenge.
2. **Context Matters**: Camera terminology is heavily dependent on context, making accurate translation tricky for AI systems. For instance, "zoom" could mean either optical or digital zoom, requiring careful analysis of the surrounding text to correctly translate, something basic tools struggle with. This context-dependent nature necessitates more sophisticated methods.
3. **Training Data Quality Impacts Accuracy**: While translation memory banks offer benefits, research shows that AI models trained on low-quality bilingual datasets tend to produce poor results in technical areas like photography. This implies that achieving fast, cheap translations might come at the cost of accuracy, particularly when dealing with complex camera terms.
4. **AI's Limits with Complex Language**: Despite their strengths in handling high-resolution image data, neural networks still find it difficult to grasp the nuances of camera-specific language. Terms with multiple meanings often lead to translation errors, especially crucial in technical documentation where precision is paramount. We're still figuring out how to bridge this gap.
5. **Visual Clues Enhance Translation**: Combining image and text data helps AI understand camera-related terms better. Models trained on annotated images alongside camera documentation can build a more comprehensive understanding of the terminology. However, this approach requires significant computational power and a substantial amount of well-structured training data for it to be truly effective.
6. **Cost of Advanced Translation**: Implementing advanced translation technologies like neural networks comes with hefty operational costs, hindering adoption by smaller companies. Even though there's a push for more affordable tools, the expense of maintaining high-quality translation resources remains a barrier for many.
7. **Self-Learning's Trade-offs**: Self-training models allow AI to continuously learn and adapt to new camera terminology. But the speed of this learning process needs careful monitoring. Rushing the self-training can lead to suboptimal results if the model isn't properly guided, highlighting a necessary balancing act between speed and accuracy.
8. **Keeping Up with Innovation**: The relentless pace of camera technology necessitates constant updates to translation models to keep them current with new terms and features. The speed and effectiveness of these updates are vital, particularly in fast-moving industries where staying up-to-date with documentation is crucial.
9. **Crowd-Sourced Knowledge**: Utilizing community-driven corrections and suggestions from platforms like GitHub enhances AI translation systems. This open-source approach significantly improves model accuracy. However, it relies heavily on the engagement and expertise of users actively contributing corrections, creating a collaborative but dynamic challenge.
10. **OCR Accuracy as a Foundation**: The effectiveness of AI translation hinges on the quality of the OCR process. If the initial text extraction from the documents is poor due to inaccurate or cheap OCR, the translations will inherit these errors and misinterpretations. This reliance on good OCR underscores the importance of accurate data as the basis for accurate translations.
More Posts from aitranslations.io: