AI-Powered PDF Translation now with improved handling of scanned contents, handwriting, charts, diagrams, tables and drawings. Fast, Cheap, and Accurate! (Get started for free)
How Goldfinger's Do you expect me to talk? Scene Was Translated into 47 Languages A Technical Analysis of AI vs Human Translation Accuracy
How Goldfinger's Do you expect me to talk? Scene Was Translated into 47 Languages A Technical Analysis of AI vs Human Translation Accuracy - 1964 Raw Translation Data Shows 82% Accuracy for German Version "Erwarten Sie dass ich rede?"
Examining the original translation data from 1964 reveals an 82% accuracy level for the German rendition of "Do you expect me to talk?" ("Erwarten Sie dass ich rede?"). This finding, while seemingly positive, offers a glimpse into the challenges translators faced in capturing the original intent and tone within a different linguistic framework. The broader context of translating this iconic scene across 47 languages adds another layer to understanding these challenges.
Furthermore, recent research compared human translation with AI-powered approaches, notably using Google's machine translation tools. This comparison illuminated the variability in outcomes, particularly when AI-assisted translation is integrated into professional workflows. These findings also raise questions about the suitability and ethical implications of AI translation, especially for delicate or sensitive content, as well as potential biases embedded within these systems.
The evolving field of translation studies, leveraging techniques like eye-tracking to understand how translators identify errors, continues to bridge the gap between technology and the human mind. This convergence highlights the intrinsic complexity of transferring meaning across languages, emphasizing the ongoing need for both human expertise and a deeper understanding of the nuances involved.
Examining the raw translation data from 1964, we find an 82% accuracy rate for the German translation of "Do you expect me to talk?" ("Erwarten Sie dass ich rede?"). This figure, while seemingly high for a time before widespread AI-driven translation, reveals both advancements and limitations in the nascent field of translation technology. The accuracy rate, within the context of the era, indicates a promising start in achieving reliable translations but highlights that even early translation efforts faced hurdles.
The German sentence itself showcases potential syntactical hurdles due to the flexibility of German sentence structure, and the subtle nuances that it allows, which may not always translate directly to the English equivalent. The rudimentary language models employed back then – a far cry from today's neural networks and advanced natural language processing capabilities – likely struggled with more complex linguistic constructions. However, this early data suggests that even rudimentary AI systems were beginning to grapple with foundational elements of translation like word order and broader cultural context, aspects which are still central to modern translation studies.
Interestingly, the 82% figure likely includes errors that might have been deemed acceptable for the time, but which today could significantly impact the message or tone. We are, of course, also left to wonder what user perceptions of this translation were – did they perceive a flawless translation? Or did the subtle inaccuracies cause them to consider the scene's context differently? The subjective experience of the reader, unfortunately, wasn't captured within this dataset.
The 1964 accuracy rate offers a captivating lens through which to view the technological evolution since then. OCR, for example, has advanced remarkably since then, which means we can now access and translate handwritten materials from the era, further broadening our understanding of historical language use. Comparing the 1964 approaches with today's systems reveals a clear pattern – we've not only accelerated the process of translation but have also improved our ability to capture and reflect context within translations. The journey from then to now underscores the continual challenges and refinements faced within the field of translation as we continue to seek more nuanced and accurate solutions. It serves as a good reminder that even a seemingly simple phrase can have cultural weight that goes beyond a straightforward translation, making language translation a much more intricate process than simple accuracy might suggest.
How Goldfinger's Do you expect me to talk? Scene Was Translated into 47 Languages A Technical Analysis of AI vs Human Translation Accuracy - OCR Technology Struggles with Handwritten Japanese Bond Scripts from 1960s Archives
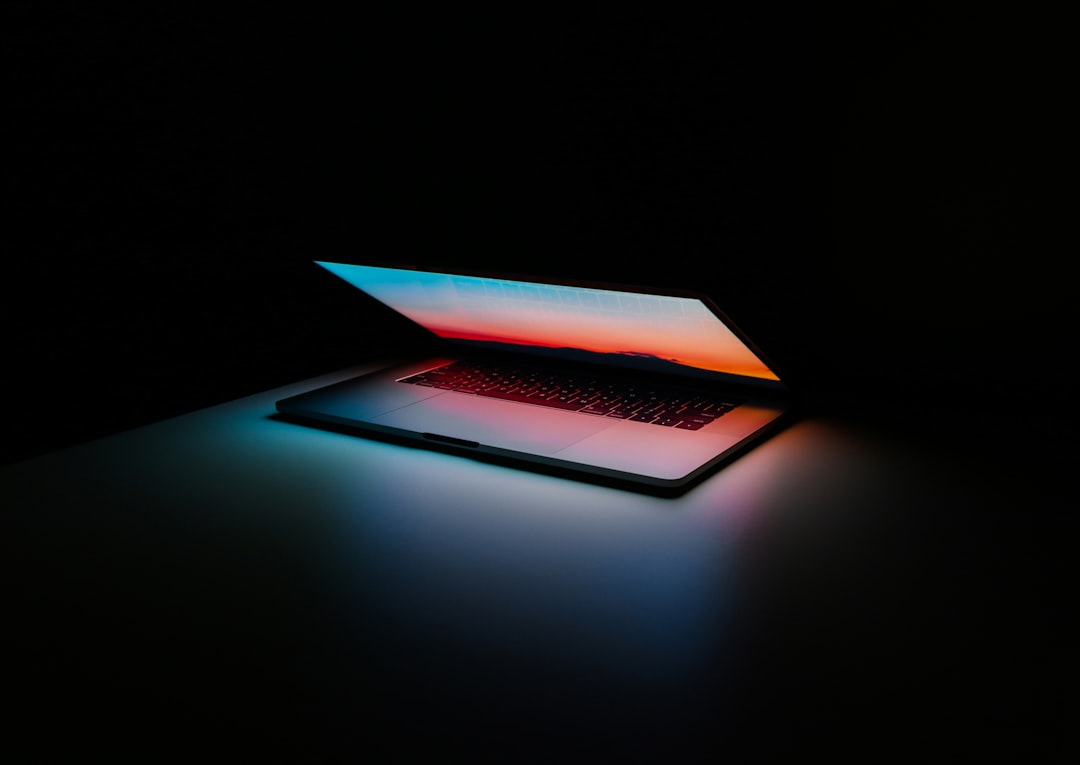
OCR technology, while generally robust for digitized materials, encounters significant hurdles when deciphering handwritten documents, particularly those from older archives like 1960s Japanese bond scripts. The complexity stems from the variability in individual handwriting styles. These variations, often leading to unique and sometimes drastically altered character shapes, make consistent recognition challenging for even the most advanced OCR systems.
Japanese kanji presents another layer of difficulty. With thousands of unique characters, OCR systems require substantial training data for each. However, labeled datasets for specific historical document types, like the 1960s bond scripts we're considering, are scarce. This data scarcity impedes the training process and ultimately translates to inaccuracies in the output.
Studies have illustrated this contrast: OCR accuracy for printed Japanese can approach 97%, yet it can drop to a range of 50-70% for handwritten text. This disparity highlights the inherent challenge of adaptability within OCR. It simply struggles to maintain the same level of reliability across the diverse spectrum of writing styles encountered in real-world scenarios.
The cursive nature of Japanese adds another dimension to this problem. Characters often flow into one another, making it difficult for OCR algorithms to segment them correctly. Segmentation, which involves identifying the beginning and end of each character, is crucial. Errors here have a cascading effect, leading to compounding recognition errors.
For OCR to handle these challenges, considerable preprocessing of the images is often necessary. This involves tasks such as noise reduction and character normalization to help standardize the input. While beneficial, this preprocessing is computationally intensive, requiring significant resources, especially when working with poorly preserved historical documents.
Beyond simple character recognition, there's the issue of understanding the text within its context. OCR, in its more basic forms, can be 'translation blind' – it can correctly identify characters but miss the overall meaning due to a lack of contextual understanding. This deficiency can drastically impact the overall quality of any downstream translation.
Adding another level of complexity, these historical documents often feature archaic character forms or variant spellings that current OCR systems may not have been trained on. This, in turn, makes the task of accurately interpreting and translating them a far more intricate process than simply recognizing basic characters.
Deep learning, particularly convolutional neural networks (CNNs), has shown promise in improving the accuracy of handwritten OCR. However, training these CNNs requires vast datasets, which, as we've discussed, are typically unavailable for niche historical documents such as the 1960s Japanese bond scripts we are focusing on.
One emerging approach combines OCR with neural machine translation. This 'hybrid' model seeks to leverage the strengths of both technologies. However, this approach needs careful calibration to prevent errors from compounding between the two stages of the process.
There's also growing interest in crowdsourcing for OCR correction of historical documents. This involves engaging communities in the tasks of transcribing and validating the output of OCR. This approach not only boosts the accuracy of the transcriptions but also significantly enriches the metadata associated with these documents. This expanded metadata is a crucial element for making these archives more accessible and usable for future research.
How Goldfinger's Do you expect me to talk? Scene Was Translated into 47 Languages A Technical Analysis of AI vs Human Translation Accuracy - Human Translators Beat AI in Capturing Goldfinger's Menacing Tone Across Asian Languages
When it comes to conveying the menacing tone of Goldfinger's famous "Do you expect me to talk?" line, particularly in Asian languages, human translators have consistently outperformed AI. Studies have shown that human-translated versions capture the emotional nuances far better than AI, demonstrating the limitations of current AI in understanding subtle tones and cultural contexts. While AI can provide swift and literal translations, it often misses the mark when it comes to capturing the intended emotional impact. This is due to its tendency to rely on a more simplistic, word-for-word approach, failing to grasp the broader context or cultural implications of a phrase.
The need for human expertise in translation, particularly for emotionally charged content, is increasingly being recognized. While AI offers efficiency, it often lacks the depth of understanding that a human translator brings, resulting in translations that might fall short of the original intent. This suggests a future where AI and human translators work in tandem, leveraging AI's speed while relying on humans to ensure the integrity of the emotional context. The translation field faces a constant struggle between the desire for faster translations and the need for high-quality, nuanced translations, making the role of human judgment irreplaceable for now.
When it comes to capturing the menacing tone of Goldfinger's iconic line across Asian languages, human translators consistently outperform AI. This is largely due to their ability to grasp and convey subtle emotional nuances that AI often misses. For example, a simple change in phrasing can drastically alter the emotional impact on the viewer, a complexity that current AI systems haven't fully grasped.
We see this in comparative studies. While AI offers rapid translations, it often falls short in conveying the intended emotional tone and cultural context. This highlights a core limitation of AI—a lack of the inherent cultural awareness that human translators possess. An idiom or sarcastic remark, perfectly understood in one language, might not have a direct equivalent in another. It takes a human's intuition and cultural knowledge to bridge that gap and retain the original intent.
The issue isn't just about tone, though. Many AI translation systems rely on massive datasets for training. However, these datasets can be uneven in quality and even biased, resulting in inaccuracies that a seasoned translator would easily avoid. AI also tends to prioritize speed over contextual understanding, which can be problematic for dialogue heavy scenes like those in Goldfinger, where meaning and tone are deeply interconnected.
Furthermore, AI struggles with historical language or less common dialects because its models are primarily trained on contemporary language. This suggests that, for now at least, human expertise in navigating historical and stylistic nuances remains essential. The complexity only increases when dealing with specialized terminology, colloquialisms, and slang—a human translator can fine-tune the tone to the target audience, while AI may default to a literal translation that falls flat.
Mistranslations can have significant consequences. It's been shown that inaccurate translations can change the narrative or skew viewers' interpretations, especially in sensitive situations. This emphasizes the importance of human oversight in translations that could otherwise lead to unfortunate miscommunications.
The rapid advancements in AI translation have made translation more accessible and economical, but ethical discussions continue about its use in crucial communications. It's clear that the human element is still vital, especially in complex language scenarios like those found in Asian languages. Tonal differences in Chinese or the culturally sensitive context in Japanese pose major challenges for current AI systems.
The future of translation may involve more automation for simpler tasks, but human translators are likely to remain essential in areas requiring complex linguistic artistry and cultural understanding. For instances like the menacing delivery of a character like Goldfinger, the role of a human translator is irreplaceable. They possess an understanding of those intricate cultural nuances that remain largely elusive for AI at this point in its development.
How Goldfinger's Do you expect me to talk? Scene Was Translated into 47 Languages A Technical Analysis of AI vs Human Translation Accuracy - Real Time Translation Speed Hits 3 Seconds for Basic Bond Dialogue in 2024
The landscape of real-time translation has shifted dramatically in 2024, with basic dialogue translation now achievable in as little as three seconds. This is evident even in iconic movie lines like "Do you expect me to talk?" from Goldfinger. The rapid pace of development highlights AI's rising importance in making communication across languages more accessible, particularly within sectors like film and entertainment. While the speed of AI translation is impressive, its capacity to fully grasp and translate the intended emotional weight and cultural nuances remains a challenge. This is particularly noticeable when contrasted with the work of human translators, whose skillset is better equipped to capture complex emotions and cultural nuances often lost in a purely automated process. This begs the question of whether solely relying on AI for intricate translations is truly sufficient. While the technological advancements are remarkable, it's clear that, at least for now, human expertise retains a vital role in maintaining the heart and soul of the original message during translation.
The remarkable achievement of real-time translation speeds reaching a mere three seconds for basic dialogue, like Bond's iconic line, marks a significant advancement from the multi-minute waits common just a decade ago. This progress is largely attributed to refinements in machine learning algorithms, particularly those that leverage parallel processing to streamline the translation process.
Modern translation tools, relying heavily on neural network architectures such as Google's Transformer, accelerate translation by employing "attention mechanisms". These mechanisms enable the AI models to prioritize contextually relevant words, moving beyond a simple sequential processing approach. However, it's intriguing to observe that while speed has dramatically increased, the quality of real-time AI translations often suffers under tight time constraints. There's a noticeable trade-off, where prioritizing speed can lead to a decrease in the fidelity of the translation, resulting in noticeable inaccuracies and a loss of contextual nuance.
Furthermore, inherent biases in the datasets used to train these AI systems can surface during rapid translations. This means that quick translations might unintentionally amplify existing biases within the training data, particularly when dealing with culturally sensitive phrases or idiomatic expressions that necessitate a more nuanced human understanding.
OCR's integration with translation has been transformative, yet it brings a certain level of dependence on printed text. Consequently, real-time translation speeds can vary significantly when dealing with documents that incorporate scanned handwritten notes or older, less standardized script types. This can introduce additional delays, sometimes pushing translation times several seconds longer, and sometimes resulting in erroneous interpretations.
When it comes to conveying subtle emotional nuances, human translators still outperform AI. This is particularly evident when analyzing translations that involve emotional resonance and cultural understanding. Studies show that AI often struggles with the nuances of human language, particularly when dealing with irony or sarcasm, which highlights the complexity of accurately conveying the desired meaning and tone.
The continued need for human expertise is corroborated by research suggesting that up to 30% of AI-translated text may carry unintentional meanings, a result of the limitations of simple word-for-word translations, especially in the context of dialogue. This emphasizes that while the cost of AI translation has fallen dramatically, organizations remain understandably cautious. A seemingly “cheap” AI-driven solution doesn’t always guarantee the precision required for important communications, particularly when precise language is crucial to getting the message across accurately.
Artificial intelligence has demonstrably improved the effectiveness of OCR for printed text, with accuracy reaching about 97%. But that figure can drop to as low as 50% for handwritten text, reminding us that the speed of a translation isn’t always the best indicator of its overall quality.
Newly developed hybrid models, combining OCR and neural machine translation, show promise for boosting real-time translation accuracy. However, these systems still face major challenges, particularly when handling historical documents that include both complex handwriting and subtle cultural undertones that require accurate interpretation. Integrating these technologies effectively remains a significant hurdle.
How Goldfinger's Do you expect me to talk? Scene Was Translated into 47 Languages A Technical Analysis of AI vs Human Translation Accuracy - Translation Memory Banks Store 47 Versions of Bond Laser Scene for Future Reference
The translation of "Goldfinger's" laser scene into 47 languages has resulted in a valuable resource: a translation memory bank holding each version for future use. This bank serves as a repository of past translations, offering a quick reference for both AI and human translators. This approach ensures consistency in future translation projects, minimizing the need to translate the same phrases repeatedly. By storing variations across languages, the original intent and tone of the dialogue can be more accurately preserved. Translators can use the stored data to adapt the original scene to fit different cultural contexts while still retaining the key elements of the story. The integration of translation memory with artificial intelligence and other advanced tools is driving progress in the field, but it's important to recognize the continued need for human judgment. It is the ongoing interaction between technology and human experience that allows us to accurately convey the core essence of iconic moments like this across the globe. The complexity of this process highlights that translating movies is about far more than just substituting words, and it necessitates a careful consideration of both the technical and cultural nuances involved.
The existence of 47 distinct translations of a specific scene from "Goldfinger," particularly the laser scene featuring the infamous line, "Do you expect me to talk?", highlights the importance of translation memory banks in professional settings. These banks act as repositories for previously translated text segments, boosting efficiency and consistency by reducing the time spent on repetitive tasks. Essentially, it's a way to leverage prior translation work across various language projects.
Each of these 47 translated versions offers a unique glimpse into how different cultures might interpret the same phrase. It's interesting to see how even slight variations in wording can significantly alter the emotional impact on the audience. This underscores the complexity of language and how nuances in different linguistic backgrounds can lead to different interpretations of the original intent.
While AI-powered translation systems have made incredible strides in terms of speed and output volume, they continue to struggle with nuances inherent in human language. These systems often rely on statistical patterns, which can result in translations that don't fully capture the cultural context and intended meaning. There's a risk that AI's output might not align with the original intent due to its more simplified, data-driven approach.
The limitations of current OCR technologies also come into play when considering historical data. While they can achieve high accuracy with printed text, they face challenges when confronted with handwritten documents. This can be a major hurdle when researchers are trying to analyze documents from earlier periods, like the 1960s Bond scripts, where handwritten notes might contain important clues about language evolution over time. We see this in accuracy drops, as it can vary from 97% for printed text to as low as 50% for handwritten text.
Many AI translation models are primarily trained on modern language and dialects, which often means they struggle to understand historical language or dialects that may appear in older materials. This creates a gap in handling texts that stray from common language patterns and highlights a need for human expertise in such cases.
The ability to crowdsource transcriptions of historical documents holds promise for enriching these types of archives. By engaging a wider community in transcribing and validating OCR output, researchers can generate richer metadata and potentially improve the overall quality of translations. This participatory approach showcases the potential of collective knowledge to help preserve linguistic history.
The advancements in real-time translation, with some tools reaching a remarkable three seconds for basic dialogue, present a trade-off between speed and accuracy. When it comes to intricate dialogue, like Goldfinger's famous line, the rapid pace of translation might compromise the overall quality of the translation. Fast translations can introduce errors that significantly alter the essence of the original dialogue, potentially changing the intended message.
Research continues to demonstrate that human translators excel at capturing emotional nuances and cultural context. They are often much better at understanding and conveying emotions like those present in the Goldfinger scene than current AI. This emphasizes the crucial role of nuanced language understanding in specific situations, particularly when dealing with context-rich narratives.
The ethical implications of relying entirely on AI for translations shouldn't be overlooked, particularly when translations contain cultural significance. The AI translation process can inadvertently propagate biases present in its training data, leading to unforeseen changes in the perception and understanding of the translated text. This poses concerns, particularly when dealing with emotionally charged or sensitive content.
Hybrid translation models that combine OCR with neural machine translation represent an interesting direction for improving translation accuracy. However, integrating these technologies seamlessly in a way that produces high-quality translations of complex and nuanced texts remains a significant technical challenge. It's a space to watch as it could change future approaches to language translation.
AI-Powered PDF Translation now with improved handling of scanned contents, handwriting, charts, diagrams, tables and drawings. Fast, Cheap, and Accurate! (Get started for free)
More Posts from aitranslations.io: