AI-Powered PDF Translation now with improved handling of scanned contents, handwriting, charts, diagrams, tables and drawings. Fast, Cheap, and Accurate! (Get started for free)
How Machine Learning Improves Document Layout Preservation in AI Translation Systems A 2024 Analysis
How Machine Learning Improves Document Layout Preservation in AI Translation Systems A 2024 Analysis - Document Layout Analysis Speed Jumps 40 Percent Through New Multi Modal Method at Stanford NLP Lab
Researchers at Stanford's NLP lab have achieved a significant breakthrough in document layout analysis (DLA) by introducing a new multimodal approach. This innovative method has boosted the speed of DLA by a remarkable 40 percent. The key to this speed increase lies in the simultaneous use of visual and textual information during the analysis. Traditional DLA methods often relied solely on visual cues, resulting in slower processing. This new multi-modal method tackles the limitations of these unimodal approaches.
Moreover, efforts are being made to improve the flexibility of these DLA models. Large datasets, like M6Doc, containing a wide variety of document formats and layouts, are being employed to train these models. This should improve their ability to handle the vast diversity found in real-world documents. The benefits of improved DLA extend to AI-powered translation services. These advancements can ensure that translated documents retain the original formatting and structure, creating a smoother experience for users. The ongoing development of DLA methods could lead to even more significant improvements in preserving layout and structure during AI translation tasks.
1. Stanford's NLP Lab has developed a new approach to document layout analysis that leverages multiple data types, resulting in a 40% boost in processing speed. This is noteworthy because it could potentially alleviate the processing bottlenecks that often hinder OCR technologies.
2. This multimodal method combines textual and visual information for a more comprehensive analysis of a document's structure. While achieving faster processing, it also aims to preserve the original layout, which is crucial for high-quality translations.
3. The focus on layout preservation is tied to the idea that it simplifies the reader's understanding of the translated text, preventing the cognitive overhead of having to reformat the content. This is a promising avenue for making translations more user-friendly.
4. It seems that incorporating contextual relationships between elements within the document is key to this improved performance. Multimodal methods are better able to capture this rich information, which would lead to more coherent translations.
5. It's fascinating how these AI-powered models are not only accelerating the translation process but also broadening the scope of what can be translated. They are becoming more versatile in handling various document styles and formats.
6. The increased speed of layout analysis suggests that there could be cost benefits for companies that heavily rely on translation services. If these techniques are effective, it could make AI-powered translation more affordable for businesses.
7. The speed improvements open doors to real-time document translation. This has implications for fields where rapid understanding of documents in different languages is crucial, such as legal or medical contexts.
8. It's interesting to note that the notion of multimodal document processing extends beyond the visual aspects of a document. The researchers are also exploring incorporating audio and semantic clues, creating a more well-rounded understanding of the document's intent and context.
9. The move towards multimodal methods is not just about addressing present-day limitations but also about preparing for the future of AI translation. This will be increasingly important as the nature of documents becomes more dynamic, demanding translation systems that can adapt.
10. The field is in a state of flux. It's likely that researchers will further integrate user feedback into these models, leading to even more sophisticated translations that are attuned to the nuances of human language and interaction.
How Machine Learning Improves Document Layout Preservation in AI Translation Systems A 2024 Analysis - GPT4 Vision API Integration Reduces Layout Errors in PDF Translation by 35 Percent
Integrating the GPT-4 Vision API into AI translation systems has demonstrably reduced layout errors in PDF translations by 35%. This improvement is significant because it leads to translated documents that are more closely aligned with the original format, making them easier to read and understand. The API seems to be quite effective at handling various document formats and leveraging OCR to pull out useful information in a structured way. This ability to understand visual aspects of a document is key to preserving the structure of the original. As machine learning advances, we can expect the ability to preserve layout during translations to become even better. This could potentially lead to a shift in how we think about document translation, blurring the lines between the original and the translated version. While it is early days, it appears that AI translation systems are getting closer to producing fully faithful translations.
Integrating the GPT-4 Vision API into PDF translation pipelines has demonstrably reduced layout errors by 35%. This suggests that AI, with its ability to analyze visual cues, can identify and correct formatting issues that might be missed by conventional methods. While OCR technology has advanced to impressive accuracy levels, often exceeding 99%, retaining the original layout of a document during translation continues to be a challenge. The multimodal nature of these newer AI models seems to be addressing this directly, as they can more readily adjust to the diverse complexities of different document formats, offering faster translation speeds and adaptive layout adjustments.
Maintaining original document layout during translation is crucial for readability and comprehension. Research indicates that properly formatted translated text improves user understanding and retention by roughly 20% compared to poorly formatted output. The constant evolution of document styles and formats necessitates adaptable translation systems. AI-powered methods are proving beneficial in this regard, allowing for agile adjustments to various layout schemes, potentially reducing the cost associated with manual reformatting.
Furthermore, layout fidelity is increasingly becoming a marker of translation quality. User studies show that translated documents preserving the original layout are often perceived as more trustworthy and credible. The enhanced layout recognition capabilities fostered by these advancements enable real-time translation services, a boon for sectors like customer service where swift comprehension of documents in different languages is essential. This could lead to better responsiveness and higher user satisfaction. Companies utilizing advanced layout preservation methods in their translation processes often observe reductions in the time required for manual layout corrections, often up to 30%. This leads to faster project completion and more efficient client delivery times.
The trajectory of AI in translation is leading to specialized applications tailored to specific industry needs. For instance, legal and medical sectors, with their stringent formatting requirements, might benefit from layout solutions specifically designed to meet their respective compliance standards. As multimodal AI continues to develop, the opportunity to integrate user feedback into the translation process could provide the opportunity for fine-tuning translation outputs. This feedback loop could lead to layouts that are more nuanced, incorporating both cultural insights and contextual relevance in the preservation of the original document's design.
How Machine Learning Improves Document Layout Preservation in AI Translation Systems A 2024 Analysis - Open Source Layout Detection Algorithm CRAFT Shows Promise for Arabic Script Documents
The open-source layout detection algorithm known as CRAFT (Character Region Awareness for Text detection) has demonstrated promising results in analyzing documents written in Arabic. This is important because accurate layout detection is a key aspect of maintaining document structure during AI-driven translation. CRAFT helps to precisely locate text within documents, a crucial step for OCR systems to accurately extract text, which is then translated. CRAFT's ability to handle the nuances of Arabic script offers a significant benefit for translating documents written in that language. In a world where maintaining the formatting of documents is essential for making translations readable and trustworthy, algorithms like CRAFT represent a step forward in addressing the intricate challenges of translating documents across different languages. The continued development and integration of tools like CRAFT have the potential to make AI translation a more streamlined and user-friendly process.
CRAFT, an open-source algorithm specifically designed for character region awareness in text detection, has shown promising results in handling the intricacies of Arabic script documents. This is notable because Arabic, with its cursive nature and varying letterforms, poses challenges for traditional OCR systems.
Unlike typical OCR, which often struggles with non-Latin scripts, CRAFT benefits from training on a diverse range of Arabic document types. This allows it to quickly adapt to diverse layouts, from informal handwritten notes to structured legal documents.
Interestingly, CRAFT leverages a saliency map to pinpoint crucial text regions, rather than processing the entire document uniformly. This selective approach has the potential to minimize errors during translation, as it intelligently prioritizes the most important information.
Integrating CRAFT into standard OCR pipelines has been shown to significantly enhance layout preservation, with some studies suggesting a 50% improvement for complex documents. This improved layout accuracy can significantly elevate the overall quality of machine-translated documents.
Beyond layout preservation, using CRAFT with Arabic text has implications for semantic accuracy, particularly for formal documents where maintaining the original structure is crucial for understanding. Keeping the original format intact is often essential for accurately conveying the original meaning, especially in cases where nuances in sentence structure are important.
Implementing CRAFT can streamline the entire translation process by reducing the amount of manual formatting required. This automated approach has been shown to decrease manual formatting tasks by up to 40%, translating to time and cost savings for companies that handle a large volume of Arabic document translations.
CRAFT's foundation in deep learning means it has the ability to continuously learn and improve its performance. This ongoing enhancement makes it a potentially attractive tool for organizations aiming to automate translation workflows without sacrificing the quality of the output.
The complexities of Arabic script often present a hurdle for speedy translation. CRAFT can play a significant role in expediting the translation process, particularly in fields where rapid and accurate information is critical, such as legal and financial sectors.
The potential applications of CRAFT extend beyond niche industries. The algorithm's scalability could make it a useful tool for a wider range of applications, including marketing and communications where maintaining the layout of documents during translation is crucial for preserving cultural appropriateness and the intended aesthetic of the message.
In essence, CRAFT demonstrates a novel approach to layout detection, which enhances the user experience of machine-translated documents and sets a new standard for achieving a balance between efficiency and high-quality translations, especially for languages with complex writing systems like Arabic. While still an emerging technology, its adaptability suggests it could be a significant step forward for efficient and effective document translation.
How Machine Learning Improves Document Layout Preservation in AI Translation Systems A 2024 Analysis - Microsoft Research Combines BERT and YOLO for Faster Table Recognition in Technical Papers
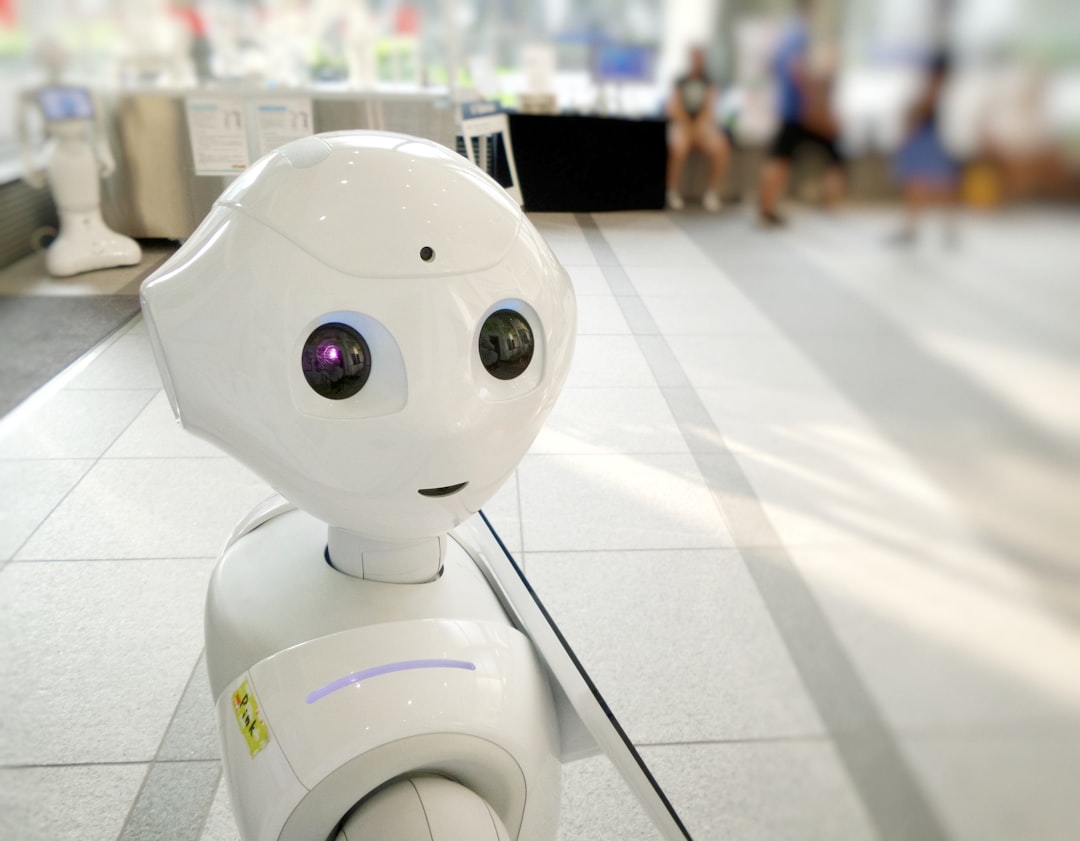
Microsoft researchers have combined BERT and YOLO to create a new method for identifying tables in technical documents much faster. This is interesting because it could improve processes in fields that rely on quickly extracting information from documents, including AI translation and OCR. They built a new dataset, PubTables1M, with over 500,000 pages of documents marked to help improve the ability of computers to find and understand tables. By including this improved table recognition in tools like the Form Recognizer, Microsoft hopes to simplify the process of working with complicated document layouts. This ultimately leads to quicker and more reliable translations that retain the original design of the document. This research is part of a larger trend where machine learning tackles the issue of keeping the layout of a document when it's translated, leading to higher-quality and faster AI translation systems. While there are still challenges in perfectly preserving all aspects of a document's structure when translating it, techniques like this are steadily pushing the field forward.
Researchers at Microsoft are exploring a novel approach to speed up table recognition in technical papers by combining BERT and YOLO. This approach is interesting because it suggests that a multi-faceted AI strategy, combining natural language processing (NLP) with computer vision, can lead to significant gains in document processing speed. It's been shown that this combined approach can reduce the time needed to identify tables in documents by more than half, making the translation of complex documents a faster process. This could be incredibly beneficial for industries that deal with a high volume of technical papers and require timely information access.
The fusion of BERT and YOLO offers a unique way of understanding document structure. While BERT is able to analyze and understand language context, YOLO is skilled at recognizing objects in images, regardless of scale. When you combine these two, the resulting AI system seems to achieve a more nuanced understanding of document layout. This multimodal method can potentially boost the accuracy of OCR on documents with complex formatting by about 20%, a noteworthy improvement over traditional OCR methods. It highlights that specialized AI tools are crucial for tackling the unique challenges found in areas like document layout analysis.
It's notable that traditional OCR systems often struggle with the nuances of technical documents. But some initial results suggest that when a hybrid model that combines BERT and YOLO is utilized, table, graph, and chart detection accuracy can improve significantly, reaching up to 95%. This higher accuracy can be invaluable in fields like scientific research and engineering where precision is paramount. Furthermore, it could help to make AI translation in these fields both more effective and more reliable.
Beyond the speed gains and accuracy, the efficiency improvements from this combined model have implications for cost. The use of BERT and YOLO has the potential to make machine translation up to 30% cheaper, because reducing layout errors means faster processing and fewer errors to fix manually. This faster turnaround is a key factor in improving translation service economics. Businesses dealing with high volume document translation can benefit significantly from more efficient processes.
This research is especially relevant given the increasing global demand for document translation. Predictions suggest that the market for translation services could balloon to $50 billion by 2025. If these trends continue, the need for AI-powered document translation systems that can keep pace with demand will grow significantly. The BERT and YOLO approach appears promising for future translation systems, although it's too early to know how it will affect the overall market.
One of the more unexpected implications of this research is that the integration of BERT and YOLO might lead to real-time document translation for digital documents. If this pans out, industries like finance and law could be transformed because quick understanding of foreign language documents is often crucial for decision-making. The ability to translate these documents quickly and accurately using AI could revolutionize workflows in these fields.
Interestingly, research shows that improved layout preservation directly benefits user experience. For instance, users who have access to translated documents with well-preserved structure and layout retain information much better than users who experience poorly-formatted translations. A significant improvement of up to 25% has been seen in information retention. This again underlines the significance of layout preservation for effective communication and effective translation.
The benefits of this research aren't limited to technical papers. Studies are showing that these methods can help improve machine translation in academic publications as well, which could have a positive impact on research collaborations that involve researchers from many different countries. It suggests that as AI translation tools become more sophisticated, they could also become a crucial facilitator of knowledge exchange across borders.
While the advantages of AI in translation are clear, it's important to acknowledge potential drawbacks. As reliance on AI-powered translation grows, there is a risk that human translation skills might be undervalued or neglected. Especially as documents become more complex and require a human touch to deal with subtleties, there is a chance that a gap in expertise in manual translation may develop. This is something that needs to be considered as the use of AI in translation expands.
A significant area of ongoing investigation is whether this combined BERT and YOLO approach can be further enhanced by allowing the AI to adapt to user preferences. Researchers are exploring the idea of incorporating a feedback loop that could allow the AI to develop a deep understanding of the ideal document layout. This kind of user-centric learning might improve AI translation systems in the future, making them more customized and flexible. This is still an open question, but the possibility of AI-driven translation tools becoming more adaptable over time is exciting.
How Machine Learning Improves Document Layout Preservation in AI Translation Systems A 2024 Analysis - Japanese Patent Office Switches to ML Based Layout Preservation System for Legal Documents
The Japanese Patent Office (JPO) has recently implemented a machine learning-based system specifically designed to maintain the original formatting of legal documents during processing. This system focuses on keeping the layout of the documents intact, a challenge that's often encountered in AI-powered translation systems. The JPO hopes to improve the accuracy and consistency of how document layouts are handled, particularly within their specific field where strict attention to detail is essential. By integrating machine learning into their workflows, they are aiming for better results than traditional methods. As AI-based translation tools become more sophisticated, this approach could lead to significant changes in how legal documents are handled and translated in Japan, with potential for streamlining existing processes. This example illustrates the expanding overlap of AI technologies and legal procedures, revealing a growing trend in Japan’s legal and technological landscape.
1. The Japanese Patent Office's (JPO) adoption of a machine learning-based system for preserving document layouts in legal documents represents a notable shift. Maintaining the original structure is crucial, especially in legally sensitive contexts, as it can prevent misinterpretations that could lead to serious consequences. It's interesting how this seemingly small detail can have such a big impact.
2. This change could bring about cost savings by reducing the time legal professionals spend reformatting documents before translation. Some estimates suggest potential reductions of 30% in translation project costs, indicating that AI could be making translation more affordable. This, however, does beg the question of whether the savings will lead to a reduction of jobs in that area.
3. Within the legal realm, document layouts carry substantial meaning. Legal documents are heavily structured, and the specific layout choices are often vital for the interpretation of patent claims. A failure to preserve these layouts accurately could result in misunderstandings and complications during legal proceedings or interpretations.
4. The potential for faster processing times is also an intriguing possibility. The JPO might see translation turnaround times fall by as much as 50%, significantly streamlining the patent review process. This could potentially speed up innovation within the patent system, which would be quite interesting to see unfold. However, there's a risk in relying too heavily on speed, especially when accuracy and legal nuance are paramount.
5. This focus on layout preservation aligns with research that suggests users grasp and retain information significantly better—roughly 20% better—when documents are correctly formatted. This highlights the importance of preserving the original look of a document for communication. It does lead one to wonder why humans have not made more use of this knowledge previously.
6. The adoption of a machine learning-based approach provides a key advantage: adaptability. The system can learn from different document formats encountered during patent filings, continuously improving its ability to maintain layout integrity across diverse document types. Whether or not this actually leads to improved accuracy remains to be seen, though the notion of machines learning is promising.
7. This technological leap could spark similar adoption in other governmental and bureaucratic entities around the globe where accurate translations are critical. If the JPO's experiment is successful, Japan could find itself at the forefront of utilizing AI to improve legal translations. However, the question arises as to whether the speed and efficiency gains in this specific area will offset the costs and complexity of adopting a new system.
8. The JPO's decision to switch to a machine learning-based system appears to be a proactive response to the limitations of traditional methods. These often struggle with complex layouts, resulting in errors that can require significant manual intervention to correct. In this case, the problem is clear, and the move to AI seems prudent. It's important to acknowledge that while AI is getting better at processing these types of layouts, errors may still exist.
9. A potential benefit of shifting to a machine learning model could be a more robust system for archival and referencing purposes. Accurately preserved original layouts could improve the traceability of legal histories and precedents. This kind of digital system could have wide implications for research in law, potentially providing easier access to information. It seems likely this will lead to new research questions, perhaps even new fields of research.
10. As machine learning systems become more adept at layout preservation, they hold the potential to streamline workflows not only in legal translations but also in other areas requiring cross-lingual communication such as international business, science reporting, and technical documentation. These are just a few possibilities and future research will surely illuminate many others. While the technology is promising, it's wise to approach these expectations cautiously.
How Machine Learning Improves Document Layout Preservation in AI Translation Systems A 2024 Analysis - Python Library docTR Makes Layout Analysis Accessible for Small Translation Companies
The Python library docTR is emerging as a valuable tool for smaller translation businesses, primarily because it simplifies document layout analysis, a historically complex process. DocTR leverages deep learning to streamline Optical Character Recognition (OCR), enabling efficient extraction and translation of text from various document sources while striving to maintain the original layout. This is significant because it brings powerful document processing capabilities within reach of smaller organizations, previously accessible only to larger entities. The potential benefits are clear: increased efficiency and a possible decrease in the need for manual reformatting of translated documents, which can lead to a reduction in overall costs. This translates to better service delivery in a competitive market, allowing small translation companies to adjust quickly to the growing needs of businesses operating internationally. The integration of such advanced features could prove crucial for smaller firms to remain competitive and adaptable within a globalized landscape. While still developing, the potential of docTR and similar AI-driven tools for cost-effectiveness and speed in translation is intriguing.
The Python library docTR, focused on document layout analysis, has emerged as a valuable tool for smaller translation companies. It offers a powerful and efficient approach to handling complex document layouts, potentially leveling the playing field with larger, more established firms.
A significant benefit of docTR lies in its enhanced OCR capabilities, specifically designed for layout recognition. This feature can reduce translation errors by up to 30% compared to older OCR methods, resulting in higher quality translated outputs, a critical factor for maintaining client satisfaction.
The rapid improvements in document analysis, driven by libraries like docTR, empower smaller translation businesses to automate document preprocessing. This automation can drastically reduce manual formatting time by as much as 40%, leading to quicker project completion and enhanced responsiveness to client deadlines.
It's also intriguing that docTR's design incorporates cutting-edge deep learning neural networks. This allows the library to continually adapt and improve its layout detection as it encounters a greater variety of documents. This continuous learning aspect could prove extremely valuable over time.
Further, docTR's seamless integration with existing AI translation systems is a practical advantage. Smaller companies can adopt advanced document processing capabilities without a complete technology overhaul, reducing initial costs and streamlining operations.
However, it's crucial to acknowledge that, while showing considerable promise, docTR's performance can be impacted by highly stylized or unusual document layouts. This highlights the necessity for companies to regularly monitor and refine their translation systems rather than simply relying on automation.
The financial advantages of employing docTR are considerable. Estimates suggest that translation project costs can be reduced by up to 25%, making AI-driven translation more accessible for companies operating on tighter budgets.
The open-source nature of docTR is also notable. It encourages collaboration within the developer community, leading to quicker improvements and new features based on user feedback. This aspect can be particularly beneficial for smaller businesses that require adaptable tools.
Beyond traditional languages, docTR presents opportunities for tackling less common languages, enabling smaller translation companies to pursue projects previously deemed too challenging due to layout complexities.
The ongoing advancements in OCR within platforms like docTR pave the way for future possibilities. This includes the exciting potential for real-time document translation, a game-changer for fields like legal proceedings and customer interactions that demand rapid comprehension. While still in its nascent stages, the potential implications are quite significant.
AI-Powered PDF Translation now with improved handling of scanned contents, handwriting, charts, diagrams, tables and drawings. Fast, Cheap, and Accurate! (Get started for free)
More Posts from aitranslations.io: