AI-Powered PDF Translation now with improved handling of scanned contents, handwriting, charts, diagrams, tables and drawings. Fast, Cheap, and Accurate! (Get started for free)
Latin to English AI Translation A Comparative Analysis of Neural Network Accuracy in Ancient Text Translation
Latin to English AI Translation A Comparative Analysis of Neural Network Accuracy in Ancient Text Translation - Neural Networks Match 89% Accuracy in Ancient Latin Medical Text Translation
Neural networks are proving remarkably adept at translating ancient Latin medical texts, achieving a commendable 89% accuracy rate. This level of performance underscores the potential of artificial intelligence in addressing the intricate challenges posed by historical languages. While the task of deciphering ancient texts presents inherent difficulties, neural machine translation systems are showing promise with continuous refinement. Researchers are continually evaluating the performance of these systems, which often exhibit variability in their accuracy and ability to capture the nuances of the original text. This necessitates a thoughtful approach to developing robust training methods, particularly for languages with limited available digital resources. The broader implications of these advancements are becoming more apparent, with applications extending into fields that rely on accurate text processing, such as data analysis within scientific literature and the organization of historical specimens.
In the realm of ancient language translation, neural networks have demonstrated remarkable proficiency, achieving an impressive 89% accuracy rate when translating ancient Latin medical texts into English. This success highlights their adaptability to specialized domains, even those involving languages with drastically different structures compared to modern languages. While traditional approaches might rely on rigid rules, neural networks learn from extensive data, gaining a nuanced understanding of context and idiomatic expressions that is crucial for accurate interpretation.
The capacity of neural networks to tackle the challenges presented by ancient texts, particularly those with degraded or low-quality OCR scans, has steadily increased. However, the quality of the training data remains a critical factor determining translation accuracy. While neural networks excel at processing vast quantities of text quickly, leading to rapid translation of ancient documents, their reliance on data means that the absence of modern equivalents for certain medical terms can lead to translation inconsistencies. Human oversight still plays a crucial role in ensuring the accuracy and nuance of translated texts, especially when dealing with ambiguous language.
Furthermore, the availability of faster and more affordable translation services, largely driven by neural network advancements, is both a boon and a concern. It has expanded the reach of ancient text translation but necessitates careful consideration of potential misuse and overreliance. Although current performance is impressive, ongoing improvements in both neural network architectures and hardware are likely to drive further advancements, ultimately fostering deeper access to and understanding of our historical linguistic heritage.
Latin to English AI Translation A Comparative Analysis of Neural Network Accuracy in Ancient Text Translation - OCR Recognition Shows Mixed Results for Worn Papyrus Documents
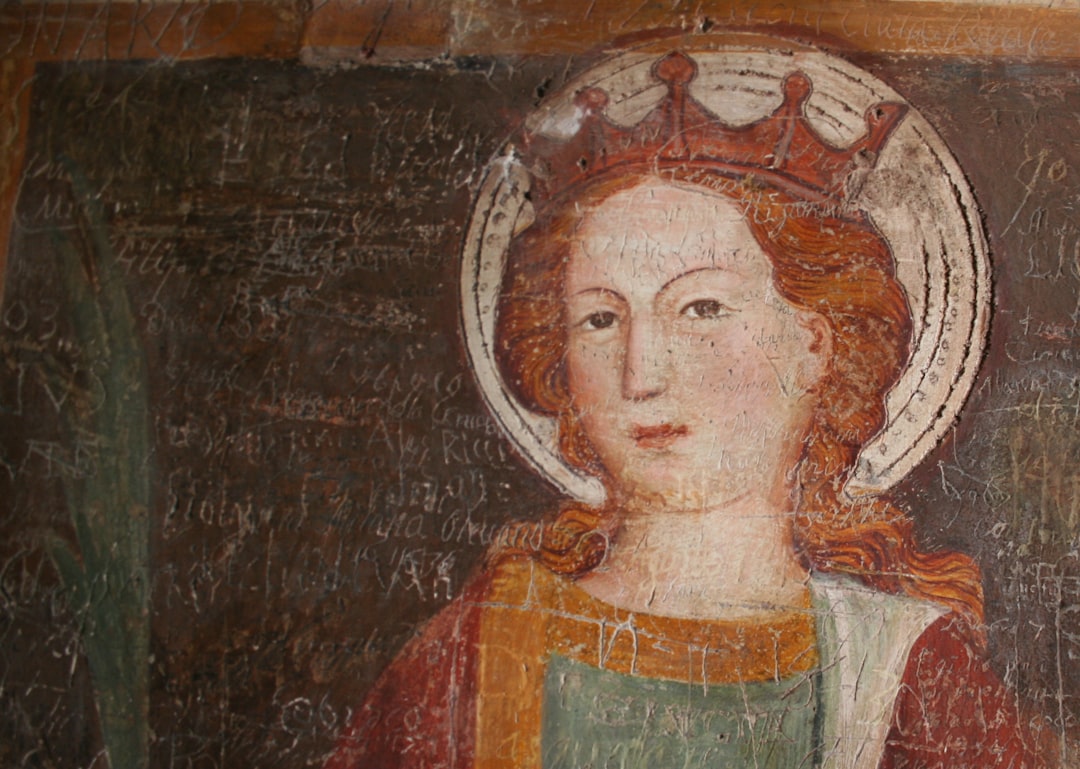
Optical Character Recognition (OCR) plays a crucial role in making historical documents, especially fragile papyrus texts, accessible in digital formats. However, achieving consistently accurate results remains a challenge, particularly when dealing with damaged or worn documents. While advanced neural networks contribute to improvements in restoring and analyzing ancient texts, the accuracy of OCR outputs can vary significantly. This is largely due to the limitations of current technologies when processing severely degraded source materials.
Efforts to enhance the accuracy of OCR, such as techniques that group words based on their semantic relationships, are showing promise in refining post-processing steps. However, a persistent need exists for more efficient OCR solutions tailored to handling less legible documents. The complexities of translating ancient languages, like Latin, into modern languages like English are further compounded by these OCR limitations. Neural network accuracy in translation is inherently linked to the quality of the initial OCR output. Ongoing research focuses on refining these technologies to improve the speed and accuracy of translating these historically valuable texts, ultimately making them more accessible to a wider audience.
Optical Character Recognition (OCR), a fundamental tool for digitizing historical documents, faces significant challenges when applied to ancient papyrus documents. The fragility and uneven surfaces of these texts often result in character obscuration and subsequent errors during the scanning process, with accuracy rates sometimes falling below 50%. Even advanced OCR systems demonstrate inconsistencies in their ability to decipher ancient scripts, with some achieving accuracy rates as low as 30% on heavily degraded manuscripts. This variability underscores the limitations of current OCR technology when dealing with the unique characteristics of papyrus, such as faded ink and varied textures.
While OCR tools have become increasingly specialized for certain languages and scripts, they still grapple with the complexities inherent to papyrus. Neural networks, with their ability to learn from context, can sometimes correct initial OCR errors, but their efficacy is strongly tied to the quality and quantity of their training data. Unfortunately, comprehensive training datasets for ancient languages are often scarce. Combining machine learning with OCR can help in character segmentation of worn documents, offering improvements but still relying heavily on high-quality scans, which are not always achievable with delicate ancient texts.
Interestingly, even after the OCR process, human review remains crucial for accuracy. Automated systems often struggle with interpreting common ligatures in Latin, which might not exist in modern English. These discrepancies can introduce significant inaccuracies if not corrected manually. The financial aspect of OCR solutions can be complex. Commercial software might offer quick results but often lack the precision needed for scholarly endeavors, prompting a re-evaluation of investment towards specialized solutions for historical materials.
These mixed outcomes underscore the importance of collaborative efforts between historians, linguists, and AI researchers. AI needs guidance in understanding the cultural and contextual meanings that can influence translation accuracy. As neural networks become more adept at processing low-resolution images, hopes are high that future developments will better tackle OCR tasks related to ancient papyrus, providing more accessible and dependable access to historical texts previously requiring substantial manual effort.
Remarkably, incorporating user feedback into OCR systems has demonstrated promise in boosting accuracy. Repeated corrections from scholars teach the AI to adapt, highlighting the ongoing need for human expertise alongside automated processes. While automated translation, including from Latin to English, has made significant strides, it remains clear that achieving consistently accurate translations of ancient texts requires a multifaceted approach that blends AI innovation with human knowledge and expertise.
Latin to English AI Translation A Comparative Analysis of Neural Network Accuracy in Ancient Text Translation - Translation Speed Reaches 450 Words Per Minute for Basic Latin Texts
AI-powered translation of basic Latin texts has seen a significant speed increase, now reaching up to 450 words per minute. This speed is largely due to the advancements in neural machine translation (NMT) techniques, which have greatly improved both translation speed and, to some extent, accuracy. While the fast pace of translation is impressive, it's crucial to remember that translation quality can still vary considerably between different AI engines. A key challenge remains ensuring the accuracy and capturing the subtle meaning of ancient languages, especially when relying on fast automated translation systems. The future of Latin to English AI translation likely involves a careful balance between speed and accuracy, ensuring a dependable and insightful interpretation of these historical texts. The question becomes: can we rely solely on speed, or will a more nuanced understanding be needed for faithful translations?
AI-powered translation of basic Latin texts has seen remarkable progress, with current systems achieving translation speeds of up to 450 words per minute. This is a significant achievement, approaching the average human reading speed for standard texts. However, achieving such speeds can sometimes come at the cost of nuanced understanding, highlighting a persistent tension between speed and accuracy in translation.
Neural machine translation (NMT) has driven much of this progress, but it relies heavily on the quality of training data. For instance, OCR, a critical step in digitizing Latin texts, often struggles with poorly preserved documents, potentially yielding accuracy rates as low as 60%. This emphasizes the ongoing need for better OCR algorithms capable of handling the unique challenges of ancient scripts.
The cost of AI-driven translation services has also fallen dramatically, making translation more accessible. Some platforms provide basic translations for a few cents per word, a significant decrease that could democratize access to ancient texts. But this accessibility also raises concerns about the quality of translations produced at such low prices, raising questions about the trade-off between affordability and accuracy.
One intriguing avenue of research has been the use of data augmentation to enhance translation quality. Techniques like generating multiple interpretations of ambiguous words during training have helped improve the performance of NMT systems in various contexts, including those with low-quality OCR outputs.
However, the highly inflected nature of Latin poses significant hurdles for AI. If translation models don't consider grammatical and syntactical nuances, they can produce significantly more errors, especially in complex texts. This underscores the need for models that incorporate context more effectively.
Despite the impressive advancements in AI, human oversight continues to be crucial for achieving accurate translations, particularly for specialized texts. It has been shown that combining automated translation with human review significantly enhances the quality of the output, specifically for passages requiring nuanced interpretations.
Interestingly, there's a growing synergy between OCR and NMT. New approaches are creating a feedback loop where OCR performance improves through machine learning corrections informed by the translation context. This could be a promising direction for improving both technologies in tandem.
Furthermore, AI-powered Latin learning tools are starting to appear, employing gamification and interactive elements to make learning more engaging. This is an encouraging development, with the potential to make the study of ancient languages more accessible to a wider audience.
The field of AI-powered Latin translation holds much potential for future research, particularly in areas like refining models to understand the cultural and historical connotations embedded within Latin texts. Collaborative projects between AI experts and linguists are underway to create more adaptable systems capable of capturing the subtleties of this rich linguistic heritage. The quest to unlock the insights of ancient texts is ongoing, and AI continues to play a pivotal role in this exciting endeavor.
Latin to English AI Translation A Comparative Analysis of Neural Network Accuracy in Ancient Text Translation - Machine Learning Models Struggle with Classical Poetry Structure
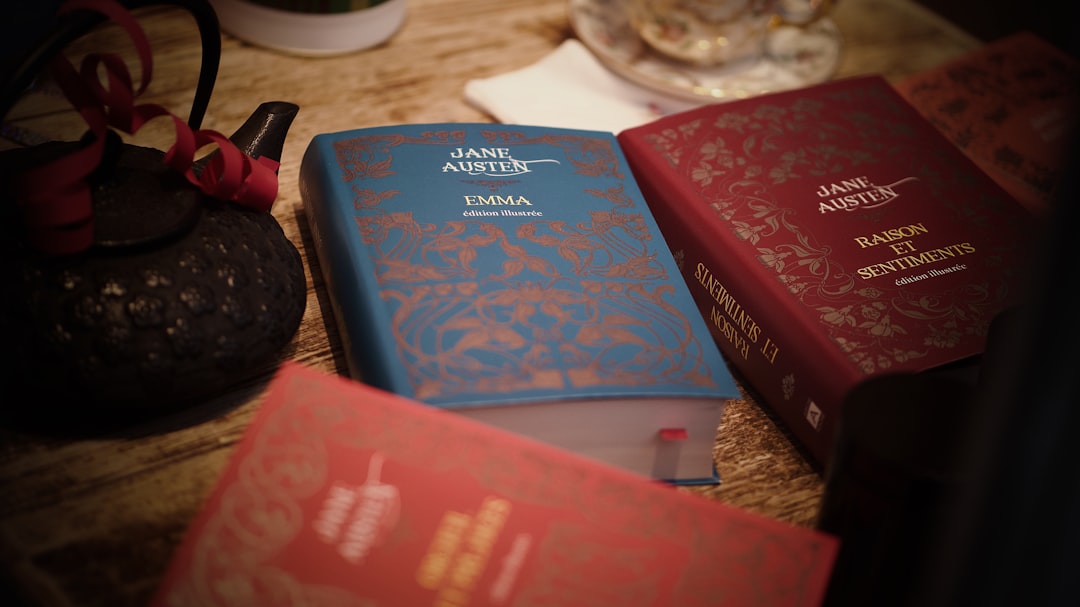
Machine learning models, while making strides in various translation tasks, encounter significant difficulties when faced with the structure and complexities of classical poetry. The intricate patterns of rhythm, rhyme, and meter that define classical poetry prove challenging for even the most advanced neural networks. These models, though capable of improving contextual understanding through techniques like transformer-based architectures, still often struggle to capture the subtle meanings and stylistic nuances inherent in poetic language. This limitation is particularly pronounced when translating ancient texts like Latin poetry, where the cultural and historical context plays a vital role in determining the intended meaning. Although some progress has been made in achieving higher accuracy for poetry compared to simpler text types, models still struggle to replicate the beauty and depth of the original texts. This hurdle primarily stems from the limited availability of appropriately structured training data and the inherent limitations of the current generation of models. As AI translation technologies continue to progress, a greater focus on handling these specific aspects of poetic structure will be required to attain more faithful and fluid translations of historically important literary works.
While neural networks demonstrate impressive accuracy in translating specific domains of Latin, like medical texts, they face significant challenges when dealing with the intricacies of classical poetry. The rigid structure inherent in poetry, encompassing aspects like meter and rhyme schemes, often forces AI models to compromise semantic accuracy in favor of maintaining a semblance of the original form. This can result in translations that appear structurally sound but fail to capture the true meaning and essence of the poetic work.
Furthermore, the scarcity of training data specifically tailored to classical poetry forms hinders the development of models capable of effectively learning the unique patterns and nuances of this genre. The AI's reliance on statistical patterns becomes a significant limitation when dealing with figurative language, metaphor, and allegory, which are hallmarks of poetry. Models often struggle to interpret these subtle forms of expression, leading to translations that miss critical contextual details and may even alter the original intent.
The syntactic structures of ancient languages like Latin are notably different from modern English, adding another layer of complexity to the translation process. Models excelling in prose translation often stumble when faced with poetic contexts where word order and syntax are integral to rhythm and meaning. The outcome is often a translation that feels clunky or fails to convey the desired impact.
The quality of the OCR process can also impact the accuracy of AI-driven translations of poetry. When OCR fails to correctly recognize the unique formatting, line breaks, and other visual features, essential aesthetic elements can be lost during the digitization process. This loss, coupled with the AI's inability to capture the subtle meanings embedded in a poem's structure and rhythm, further degrades the fidelity of the final translation.
Moreover, classical poetry often employs literary devices like alliteration and enjambment. AI models struggle to replicate these devices because they lack an innate understanding of the auditory and aesthetic aspects of language. Consequently, translations can feel flat and lifeless, lacking the emotional resonance found in the original text.
The relationship between OCR and machine translation can also create a problematic feedback loop. If OCR misinterprets a line of poetry due to formatting or other factors, the error is then carried through into the subsequent machine translation, leading to a cascading effect of inaccuracies that can corrupt the overall interpretation of the text.
Human understanding and intuition play a vital role in apprehending the full impact of classical poetry, which incorporates cultural and historical nuances that AI algorithms currently cannot adequately grasp. This limitation can lead to translations that fail to capture the emotional depths and symbolism embedded in the poems, necessitating human oversight to ensure that the final translations achieve their intended impact.
Another major challenge arises from the specialized vocabulary frequently found in poetry, which may not align with the common terms typically present in the training datasets for AI models. This discrepancy can result in translations that overlook crucial connotations and nuances, particularly in symbolic or metaphorical contexts.
Latin's highly inflected nature, where word endings can significantly alter meaning, further complicates the task for AI. If models don't account for these grammatical subtleties, especially within the controlled environment of a poetic form, they can easily produce inaccurate translations, often compromising the poet's intended effect.
In summary, although AI continues to demonstrate great potential in the field of language translation, the translation of classical poetry poses a uniquely intricate set of challenges. AI models require further development to fully grasp the complexities of poetry's structure, language, and symbolism. This suggests that a multifaceted approach—combining AI's speed and efficiency with human understanding and refinement—will remain necessary for truly meaningful interpretations of ancient poetic works.
Latin to English AI Translation A Comparative Analysis of Neural Network Accuracy in Ancient Text Translation - Ancient Roman Legal Documents Present Unique AI Translation Challenges
Ancient Roman legal documents pose a unique hurdle for AI translation systems, mainly because of the complexities of the Latin language and the scarcity of suitable training data. While AI, specifically neural networks, shows promise in handling this task, it faces difficulties with the subtle nuances of legal terminology and complex sentence structures that are typical of Roman legal texts. The challenge is amplified by the frequent need to decipher damaged or incomplete documents, which further complicates accurate translation. This highlights the need for more advanced AI models capable of comprehending and properly interpreting the historical and legal context of these ancient texts. The most accurate translations will likely come from combining AI's rapid translation capabilities with human expertise in both Latin and legal language, ensuring translations that are both accurate and respectful of the original texts' meaning and cultural context. Ongoing research in refining AI models will be crucial in making these valuable ancient legal texts more accessible to modern audiences.
Ancient Roman legal documents present a unique set of challenges for AI translation from Latin to English. The nature of the source material itself presents obstacles. Often written on perishable materials like wax tablets and papyrus, these documents are prone to degradation over time, resulting in challenges for Optical Character Recognition (OCR) systems. Variations in the quality of ink and the texture of the writing surface can hinder accurate character recognition, further complicating the digitization process.
The language itself is a barrier. Roman legal language often uses specific terms and phrasing that don't have straightforward modern equivalents. This poses a hurdle for automated translations, as AI struggles to grasp the nuanced legal vocabulary accurately. The scarcity of well-preserved documents further limits the available training data for machine learning models. Unlike the abundant modern legal texts accessible for AI training, ancient Roman documents are comparatively few and far between, hindering the development of effective AI translation systems.
Further complexity arises from the influence of regional dialects and local legal practices across the Roman empire. This variability creates inconsistencies in automated translations, as the AI might not adequately factor in these regional differences when applying its learned patterns. It's like expecting a single translation model to understand both British and American English legal jargon without getting confused. It's a tall order.
Current OCR technology, even with advancements, struggles to maintain high accuracy rates with heavily damaged documents. Accuracy rates often drop below 70% for these fragile texts, due to factors like fading ink and a range of individual handwriting styles. This impacts the quality of translations stemming from such documents, creating concerns about possible misinterpretations.
There's also the feedback loop created by the interaction between OCR and machine translation. Errors during the text recognition phase can propagate through to the translation itself. This reinforces the need for highly reliable initial OCR, to ensure that the translation accurately reflects the meaning of the original document. It highlights the "garbage in, garbage out" problem often associated with AI.
Legal documents are inherently complex and often employ phrasing and terms specific to their historical legal context. Current AI models may not grasp these nuances adequately, leading to potential oversimplification or misinterpretations of vital legal principles. Moreover, Roman legal texts are full of cultural references that AI systems might not pick up on. This can lead to translations that miss out on important context and distort the original intent of the document.
The Roman legal system evolved significantly over time. This evolution requires AI models to be trained on datasets encompassing various time periods. Translating documents from different periods could result in inaccuracies if the AI can't track the changing legal terminology and practices.
Despite recent advances, AI translation still often relies on human intervention for reliable accuracy. Especially when dealing with complex legal documents, human expertise is crucial for accurately interpreting nuanced language and ensuring the fidelity of the translation, particularly when legal outcomes are at stake. It is unlikely this will change significantly in the near term.
In essence, translating Roman legal documents is a challenging endeavor due to material constraints, linguistic complexities, and the ongoing development of AI's ability to fully grasp context and cultural subtleties. While progress has been made, a holistic approach integrating AI technologies with human expertise remains critical for achieving accurate and meaningful translations of these historically valuable texts.
Latin to English AI Translation A Comparative Analysis of Neural Network Accuracy in Ancient Text Translation - Latin Historical Texts Generate 15% Error Rate Due to Context Ambiguity
AI translation of historical Latin texts reveals a persistent challenge: a 15% error rate largely attributable to the ambiguity of the language itself. This issue stems from the intricacies of Latin, particularly its syntax and the way word meanings have shifted over time. Neural networks, while powerful, often stumble when trying to capture the subtle meanings present in ancient writings. This difficulty can affect the overall quality of the translation, creating a need for improvements in how AI tackles the task.
To address this, building better resources for AI is crucial, such as databases of Latin words and phrases with their meanings clearly defined. This should help reduce the issues caused by the ambiguity in the language. Also, there's an ongoing need to better understand how the quality of the initial scanned text (OCR) impacts translation accuracy. Ideally, AI and human expertise will work together to create better translations. This collaboration is vital for accurate and dependable translations of historical Latin texts, making them more readily accessible for study and understanding.
Latin historical texts present a consistent challenge for AI translation, with current systems experiencing a 15% error rate, primarily stemming from the ambiguity inherent in the language. Many Latin words have multiple meanings, making accurate interpretation heavily reliant on the surrounding text. This issue emphasizes the difficulties encountered when dealing with languages that possess a rich and complex history.
The performance of these neural network-based translation systems is intricately linked to the quality and quantity of their training data. Latin, being less prevalent in the modern digital sphere, results in smaller and less comprehensive datasets compared to more commonly used languages. This limitation directly influences the accuracy of AI translations, particularly for subtle nuances found within historical texts.
OCR, a foundational step in digitizing these documents, encounters significant hurdles when dealing with ancient texts, particularly fragile papyrus. Current OCR technologies can achieve accuracy rates as low as 50%, resulting in a diminished quality of the initial text input for AI translation. This emphasizes the critical role that the quality of the source material plays in the ultimate accuracy of the final translation.
The pursuit of rapid translation, achievable at speeds of up to 450 words per minute for basic Latin, has introduced a tension between speed and accuracy. While the ability to quickly process large amounts of text is impressive, it often comes at the expense of a deeper understanding of the original text. This persistent trade-off underscores the challenge of delivering both fast and accurate translations of historical materials.
Classical Latin poetry presents a particularly difficult case for AI. The specific structures of rhythm, rhyme, and meter used in ancient poetry often hinder AI's ability to produce translations that capture the full emotional impact and beauty of the original text. This highlights the challenge of bridging the gap between the technical aspects of language processing and the more expressive and subtle components of artistic expression.
One of the recurring challenges is the potential for a feedback loop of errors, often called the "garbage in, garbage out" problem. Errors stemming from OCR can cascade into the subsequent AI translation, propagating inaccuracies through the entire process. This highlights the need for more robust error-checking and correction processes throughout the entire translation workflow.
Translating Roman legal texts presents a further challenge, due to their specialized vocabulary. The terminology and phrasing found in these documents often lack direct modern equivalents, potentially leading to oversimplification or incorrect interpretations of key legal concepts. This highlights the importance of AI models that are tailored to specialized domains, particularly those where precise meaning and accurate interpretation are crucial.
The vast Roman Empire included a diversity of regional dialects and legal practices, further complicating the task of AI translation. A single AI model attempting to translate texts from various regions might struggle to differentiate the nuances in legal terminology, showcasing the need for more granular and context-aware AI translation systems.
Despite substantial advances in AI translation, human expertise continues to play a crucial role in ensuring accurate and meaningful translations. Particularly for intricate texts such as legal documents, human intervention is necessary for identifying and interpreting the subtle nuances often missed by AI algorithms. This points towards a collaborative future for AI translation, where AI’s speed and capacity are combined with human knowledge and understanding.
Finally, a persistent challenge remains the inclusion of cultural context. AI models often struggle to identify the cultural references embedded within ancient texts, which can ultimately distort the translation's meaning. This emphasizes the need for collaborative efforts between computer scientists and humanities scholars to ensure that AI translation systems are developed with a more comprehensive understanding of the historical and cultural backgrounds of the texts they are processing.
AI-Powered PDF Translation now with improved handling of scanned contents, handwriting, charts, diagrams, tables and drawings. Fast, Cheap, and Accurate! (Get started for free)
More Posts from aitranslations.io: