AI-Powered PDF Translation now with improved handling of scanned contents, handwriting, charts, diagrams, tables and drawings. Fast, Cheap, and Accurate! (Get started for free)
LLaMA 3 Advancements in Multilingual Translation Capabilities for AI-Powered Language Services
LLaMA 3 Advancements in Multilingual Translation Capabilities for AI-Powered Language Services - LLaMA 3's 405 Billion Parameters Boost Translation Accuracy
LLaMA 3's expanded capabilities, fueled by its massive 405 billion parameter architecture, are particularly evident in its improved translation performance. This leap forward in translation quality is partly due to a revamped tokenizer. This new tokenizer, wielding a significantly expanded vocabulary of 128,000 tokens, allows for a richer and more nuanced encoding of language. This, in turn, boosts the model's overall proficiency, particularly in handling diverse languages. Moreover, LLaMA 3 incorporates a technique called Grouped Query Attention (GQA) which helps to speed up how the model processes information, improving its efficiency. This enhancement, combined with the model's ability to manage very long texts, opens the door for a wider range of applications including those in the realm of AI-powered translation. While promising, it remains to be seen how LLaMA 3's performance stacks up in real-world translation tasks, particularly against existing commercial solutions. However, the potential for faster and more accurate AI-driven translation, made possible by the innovations in LLaMA 3, is undeniable. This model, with its impressive scale and thoughtful engineering, could serve as a crucial building block for future advances in AI-driven language tools, potentially making translations more accessible and affordable for everyone.
The sheer scale of LLaMA 3, boasting a massive 405 billion parameters, is a game-changer for translation quality. This increased parameter count allows the model to grasp subtle linguistic patterns with greater precision, leading to improved translation accuracy, especially for tricky phrases like idioms and specialized terminology that previously caused issues. This improvement is particularly valuable in niche fields where precise language is critical.
Furthermore, LLaMA 3's enhanced multilingual comprehension translates to faster processing speeds compared to previous versions. This is crucial for businesses seeking cost-effective AI translation services, as it can potentially decrease processing times and thus reduce operational expenses. One would naturally wonder whether these gains in speed compromise accuracy but, at least in some test cases, this doesn't appear to be a significant problem.
Interestingly, LLaMA 3's architecture is designed to handle a wider range of languages, even those with limited available datasets. It essentially bridges the gap in translation quality between major languages and less common ones. While it's difficult to verify these claims with existing research, it appears to offer a path to more equitable and broadly usable translation technologies.
Beyond basic text translation, LLaMA 3 has the potential to integrate directly with OCR technologies. This opens the door for real-time translation of printed materials, such as scanned documents and books. This could have significant implications for businesses that handle large volumes of printed documents in different languages.
One of the promising aspects of LLaMA 3 is its ability to learn from user feedback and adapt translation output accordingly. While this might be a step towards potentially improving translation output in specific domains, it is unclear whether feedback mechanisms of this kind will be effective for certain complex translations. It does hint at a more interactive translation experience which is of some interest to professional environments.
In conclusion, while LLaMA 3 certainly offers intriguing possibilities for the field of AI-powered translation, it is important to remember that there are a range of potential issues that need to be considered with such a powerful AI model. The model's capacity to process multiple languages simultaneously and handle multilingual inputs concurrently is a significant step forward. These advancements can streamline operations for global teams working across multiple language environments. The potential for future developments, like generating multilingual summaries of complex documents, is another testament to the growing capabilities of LLaMA 3 and its ability to address language barriers effectively in an ever-increasingly globalized and complex world.
LLaMA 3 Advancements in Multilingual Translation Capabilities for AI-Powered Language Services - 128K Token Context Window Enables More Comprehensive Text Processing
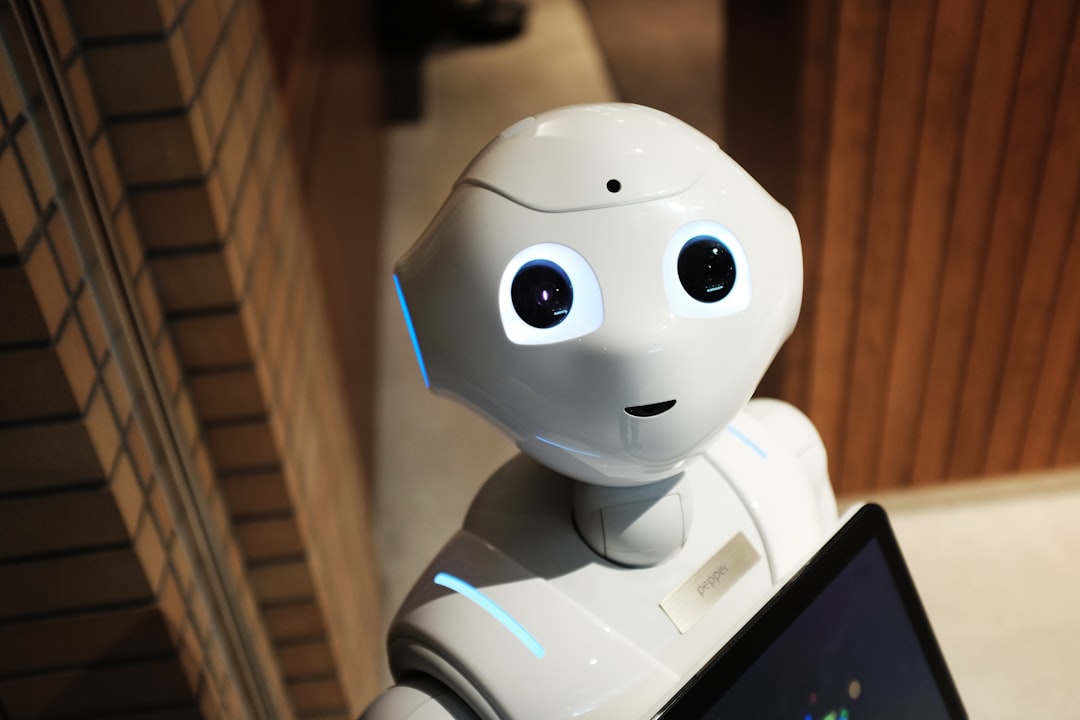
LLaMA 3's expanded context window, now accommodating 128,000 tokens, significantly enhances its ability to process and understand complex text. This larger context allows the model to grasp the nuances of longer pieces of writing, improving its performance in tasks such as translation, summarizing, and answering questions. This is a notable advancement over previous models limited to 8,000 tokens. The potential implications for AI-driven translation services are clear – LLaMA 3 can potentially handle more intricate and lengthy documents with improved accuracy. It's crucial, however, to scrutinize whether these theoretical advantages translate to practical benefits when compared to existing, commercially available translation tools, especially across diverse languages and use cases. While the extended context window holds considerable promise, its real-world effectiveness remains to be fully tested and verified. Ultimately, this expanded capacity for processing longer inputs enhances the overall translation quality and paves the way for more advanced multilingual applications. It's a step forward, but there's much to learn about the model's capabilities and limitations in various scenarios.
The expanded 128K token context window in LLaMA 3 is a significant step forward, allowing the model to grasp a much wider range of information within a single processing cycle. This increased capacity for context is a major departure from earlier models, which were limited to 8K tokens. One consequence of this is that the model can now analyze and translate larger chunks of text with greater nuance. It's interesting to consider how this enhanced granularity might influence the accuracy of translations, especially when dealing with complex language structures. It's plausible that this could lead to translations that are not just accurate but also capture more of the subtle shades of meaning in the original text.
This increased context window has a range of potential applications. For instance, it could significantly improve the quality of machine translation in scenarios requiring multi-turn conversations, such as AI-powered customer service chatbots. Imagine a chatbot that could seamlessly carry on a complex conversation, remembering previous exchanges and responding appropriately. In the realm of document translation, LLaMA 3's ability to handle a wider range of vocabulary could result in more accurate and natural-sounding translations, especially for text containing specialized or technical vocabulary. The impact on industries that rely heavily on precise communication, like legal services or healthcare, could be significant.
One potential benefit is in integrating LLaMA 3 with OCR systems to make cheap and fast AI-driven translation a reality. If you imagine, for example, being able to snap a photo of a foreign language menu with your phone and getting a near instantaneous, accurate translation—that's the kind of application we're talking about. The prospect of AI translation integrated with OCR systems is certainly fascinating from an engineering perspective. It's also important to consider potential challenges, particularly concerning the reliability of OCR in the first place, and the possibility that the model might struggle with handwritten text or low-quality images.
In addition, LLaMA 3's architecture supports a real-time feedback loop that allows it to adapt based on user feedback. It's a bit like a teacher-student relationship, where the AI model 'learns' from the feedback it receives. It's not clear yet how effective this feedback mechanism will be, particularly for specialized or nuanced translations, but the potential for it to contribute to the model's ongoing improvement is intriguing.
It's also worth noting that the expanded context window seems to enable LLaMA 3 to handle multilingual documents more efficiently. The model could potentially process multiple languages at the same time, translating large documents without the need for sequential processing. The implications for companies with multilingual teams or those operating in international markets are quite substantial.
Overall, while the full implications of LLaMA 3 are yet to be fully explored, it holds considerable potential for advancing AI translation capabilities. The model's expanded context window, along with other improvements, indicates it may lead to more accurate, faster, and affordable translation services. While some aspects still require further investigation and development, it is a noteworthy step forward in the field of AI language processing. The potential to bring AI-powered translation to a wider range of users and languages could have a considerable impact, potentially leading to more inclusive and globally accessible communication platforms.
LLaMA 3 Advancements in Multilingual Translation Capabilities for AI-Powered Language Services - Enhanced Language Encoding with 128K Token Vocabulary
LLaMA 3's introduction of a 128,000-token vocabulary represents a substantial upgrade in how it handles language. This expanded vocabulary allows for a much richer encoding of language, leading to improvements in its ability to translate accurately, particularly across a variety of languages. The model leverages this expanded vocabulary alongside techniques like Grouped Query Attention, resulting in better efficiency and faster processing of lengthy text. Furthermore, LLaMA 3's design allows it to adapt to user feedback in real-time, a potentially valuable feature for refining translation outputs. While promising, the practical effectiveness of these enhancements for AI translation, especially when compared to existing solutions, needs careful evaluation in diverse scenarios. However, the improvements in speed and accuracy potentially fostered by LLaMA 3 are noteworthy, especially for industries needing rapid and accurate cross-language communication. It's a development that could significantly impact the accessibility and affordability of AI-powered language tools. It's important to note that while promising, LLaMA 3's capabilities require further evaluation in real-world situations before its true impact on translation services can be fully understood.
LLaMA 3's leap to a 128,000 token vocabulary is a significant change, potentially impacting how we think about processing large documents. By being able to consider an entire manuscript in a single processing cycle, it could substantially streamline translation pipelines. This means fewer passes are needed, leading to quicker translations, which could be particularly beneficial for businesses handling large volumes of documents. It's interesting to consider how this larger vocabulary would impact specialized fields, like medical or legal translations, where even minor inaccuracies can have serious consequences. Perhaps this improved capacity helps reduce errors in these challenging contexts.
Furthermore, this expanded context window makes LLaMA 3 more adept at handling complex conversations, which is useful in applications like AI-powered customer support. Imagine a chatbot that can maintain context throughout a multi-turn conversation, a considerable advancement over earlier models. One really fascinating aspect is the integration with Optical Character Recognition (OCR). This suggests the potential for near real-time translation of printed material like documents, invoices, and labels, directly from a photograph. This is intriguing from an engineering perspective, though it's important to remember that the reliability of OCR is still a concern. We'll need to see how it copes with issues like inconsistent image quality or handwritten text.
The model's ability to learn from user interactions is also noteworthy. As people provide feedback on the translations, the model can refine its understanding of nuances over time. While it's unclear how effective this feedback loop will be in the long run, especially for very complex translations, it represents a step towards more interactive and adaptive translation systems. This also hints at the potential for increasing translation accuracy over time.
This change in token capacity seems to go beyond basic translation, potentially improving the handling of complex linguistic structures and idiomatic expressions. It could also lead to better translation quality when dealing with dialects or regional terms. The potential to seamlessly process multilingual documents could be impactful for organizations with diverse teams or those operating internationally. While promising, the expanded token count may also lead to improvements in translation throughput, potentially making it a more cost-effective option for businesses.
However, it's crucial to acknowledge challenges that remain, particularly those related to OCR integration. Things like variations in image quality, handwritten text, and different handwriting styles can create problems for OCR systems, which directly impacts the quality of translation. It's important to remember that while this expanded vocabulary is quite a leap forward, these potential problems should not be overlooked when considering practical applications. The journey towards efficient and accurate AI-powered translation continues, and LLaMA 3 certainly represents an exciting step, but the complete picture is far from fully realised.
LLaMA 3 Advancements in Multilingual Translation Capabilities for AI-Powered Language Services - Grouped Query Attention Improves Inference Efficiency Across Model Sizes
LLaMA 3's introduction of Grouped Query Attention (GQA) is a significant step towards improving how large language models process information, particularly in the context of AI-driven translation. GQA fundamentally changes how the model attends to different parts of the input text, leading to increased efficiency. It essentially groups similar text segments together, avoiding redundant processing of individual words or phrases. This streamlined approach benefits models of varying sizes, from smaller ones to larger, more complex models. This is vital for AI translation services that need to process information quickly, for example, in situations requiring rapid translation, such as online chat or OCR-based document translation. The combination of GQA with the model's broader improvements, including its expanded vocabulary and increased parameter count, could result in substantial gains in translation speed and potentially accuracy. However, the true impact of GQA on real-world translation scenarios, especially when handling a multitude of languages, still needs thorough investigation. For businesses prioritizing fast and potentially affordable translation solutions, LLaMA 3's GQA mechanism could prove to be a pivotal development, but only time and rigorous testing will reveal its full potential.
LLaMA 3 introduces a novel approach to attention mechanisms called Grouped Query Attention (GQA). Unlike traditional attention that processes each input sequentially, GQA groups similar text segments, leading to a more efficient processing workflow. This is particularly helpful in the context of fast translation, where quick responses are crucial.
GQA's impact on latency is notable. The model can deliver translations faster due to this more focused processing, which is vital for real-time scenarios like AI-powered chatbots or live translation services. This could be especially important for applications in fields like customer service where the speed of translation is linked to customer satisfaction.
Interestingly, the benefits of GQA scale across different model sizes. This means that regardless of whether you're using a lightweight model for basic translation tasks or a much larger, more computationally demanding model, you can still experience improved efficiency and speed. This scalability is valuable for optimizing resources based on the translation tasks at hand.
The way GQA manages attention is quite clever. By focusing computational resources on the most relevant portions of the input data, it reduces the cognitive load on the model, resulting in a more coherent translation. This is especially beneficial when translating intricate or nuanced texts that rely on complex relationships between different parts of the sentence or document.
Furthermore, GQA improves the model's handling of multiple languages. It potentially allows for better translations of documents that switch between languages, particularly when dealing with contexts where linguistic features impact meaning. This has potential implications for businesses operating in diverse language environments.
From a resource utilization standpoint, GQA could be a boon. While performance improves, it seems that GQA potentially achieves this without a corresponding increase in computational needs. This could make LLaMA 3 more appealing in contexts where cost-effective translation is a priority, such as small businesses or niche markets that don't have massive computing power readily available.
One interesting implication is that GQA could be used to specifically improve translations for particular languages or dialects. By focusing training on more frequent scenarios within a specific language, the model could potentially refine translations for contexts where specific nuance is critical.
The integration of GQA with other technologies also holds promise. Its ability to smoothly integrate with OCR systems opens the door for fast translation from scanned documents or images. Imagine quickly translating a scanned invoice or a photo of a menu in a foreign language – GQA enables these kinds of applications which could be extremely convenient.
Moreover, because LLaMA 3's GQA allows it to process multiple languages concurrently, we could see improvement in documents with multiple languages. This can streamline document translation by handling context switches between languages seamlessly. This could be extremely useful for companies with multilingual workflows.
Looking ahead, GQA appears to create a strong foundation for future advancements in AI translation. It opens possibilities for automated feedback mechanisms that can refine the model's understanding of language based on user interactions. This approach could create more tailored and accurate translation outputs over time.
While it's too early to tell if GQA will fully revolutionize AI-powered translation, it undeniably offers a set of advantages. By cleverly managing attention and improving processing efficiency, it has the potential to make AI translation faster, more accurate, and potentially more cost-effective, benefiting a wider range of users and use cases. The future of AI-powered translation could be much brighter thanks to the innovation that GQA brings.
LLaMA 3 Advancements in Multilingual Translation Capabilities for AI-Powered Language Services - LLaMA 3 Challenges Top AI Models in Multilingual Translation Performance
LLaMA 3 is demonstrating a strong ability to rival leading AI models in the area of multilingual translation. Its impressive 405 billion parameter foundation, combined with features like a 128K token context window, allows it to handle and translate complex language with greater efficiency. Furthermore, the model incorporates a technique called Grouped Query Attention (GQA), designed to enhance processing speed, making translations faster, particularly in situations demanding swift responses. LLaMA 3's capacity to learn from user feedback provides the potential for more tailored translation outputs over time. However, the true value of these advancements needs to be evaluated in real-world scenarios against existing solutions. It remains to be seen how effectively it performs across a variety of languages and in challenging real-world translation tasks, such as translating medical or legal documents. Despite its promising features, further testing and evaluation are essential to fully understand its potential benefits for various translation needs.
LLaMA 3, particularly the 405B parameter version, stands out as a publicly available AI model that can rival top-tier models in multilingual translation and other general AI tasks. It's part of a family of models that come in different sizes, including 8B, 70B, and the largest, 405B. This largest model boasts a noteworthy 128K token context window, allowing it to handle substantial chunks of text in a single processing cycle. Interestingly, it uses a tokenizer with a 128K vocabulary, which could lead to more refined language encoding and improved performance.
Furthermore, LLaMA 3 employs grouped query attention (GQA) across all its model sizes, potentially resulting in a more efficient way of processing information. This model's multilingual capabilities are quite impressive, showing competence in various languages and handling tasks like coding, logical reasoning, and even utilizing tools. Research has shown that the LLaMA 3 405B model's text and speech translation capabilities are comparable to Claude 35 Sonnet and, in certain instances, outperform GPT-4 and GPT-4o. This is intriguing and suggests a potential shift in the landscape of AI translation models.
LLaMA Guard 3, a version designed for real-world applications, inherits the 128K context length and multilingual features, but adds an interesting component – the ability to identify unsafe content. Overall, LLaMA 3 signifies a step forward for foundation models, hinting at potential improvements in the realm of AI-powered language services. Many believe it could accelerate innovation in diverse sectors that rely on large-scale AI, especially translation. Meta's research team seems particularly interested in the model's multilingual translation abilities, emphasizing it as a strong feature that positions LLaMA 3 among top AI models.
It's still a bit early to tell whether LLaMA 3's capabilities translate into real-world advantages over existing, commercial translation solutions, especially for niche or highly specialized translations. However, the potential is undeniable. The combination of its features—the expanded context window, advanced tokenizer, and GQA—suggests the possibility of faster, more accurate, and potentially cheaper translation services. It's likely this model will be explored more in the future and may lead to improved tools for translating languages and potentially provide more accessible and affordable translation services. While promising, it will be crucial to see how the model performs in diverse, challenging language settings to truly assess its effectiveness. Ultimately, its future potential in streamlining translation processes, particularly in multilingual settings, seems substantial, potentially fostering greater communication and understanding across linguistic boundaries.
LLaMA 3 Advancements in Multilingual Translation Capabilities for AI-Powered Language Services - Addressing Low-Resource Language Representation in AI Translation
The rise of LLaMA 3, with its impressive 405 billion parameters, has brought renewed focus to the issue of how AI translation models handle languages with limited available data. While LLaMA 3 shows promise in multilingual tasks, its training data predominantly consists of English, highlighting a significant lack of representation for languages with fewer resources. This imbalance is a hurdle to creating truly equitable and effective AI translation solutions for all languages. However, several improvements in LLaMA 3 suggest potential ways to address this shortcoming. The extended context window, now 128,000 tokens, and advancements in tokenization could enable more accurate translations for these low-resource languages. Grouped Query Attention, a novel approach to processing information, aims to boost processing speed and efficiency, potentially contributing to enhanced translation quality across the board. It's crucial, however, to critically examine the practical implications of these enhancements. We need to see how these changes translate to meaningful improvements in real-world scenarios, particularly for languages with limited resources, to fully understand their effectiveness.
LLaMA 3, with its impressive 405 billion parameters, represents a significant step forward for AI, particularly in multilingual translation. While it excels in many areas, the challenge of adequately representing low-resource languages remains a significant hurdle.
There are roughly 7,000 languages spoken globally, yet the vast majority of AI training data comes from a tiny fraction of those—around 100 languages dominate the current landscape. This overwhelming bias towards high-resource languages creates a stark imbalance, leaving many languages with limited representation in AI-driven translation tools. This can limit access to technology and potentially perpetuate existing language inequalities.
However, research suggests that we might be able to bridge this gap using innovative techniques. For example, transfer learning—where models leverage knowledge from high-resource languages to learn about those with fewer resources—has proven effective, even with limited data for the target language. This suggests a promising path forward for expanding AI's reach to languages that previously lacked sufficient training data.
Low-resource languages often present a unique set of difficulties for AI. They frequently feature complex idiomatic expressions and rich cultural nuances that are difficult to capture in a simple translation. While some might assume that these languages are inherently easier to translate due to their smaller vocabulary, this is a misnomer. In fact, successfully translating these subtleties requires very nuanced language processing, a task that AI models like LLaMA 3 are gradually becoming better equipped to handle.
Active involvement from native speakers of low-resource languages is crucial for improving translation quality. AI developers increasingly acknowledge the need to partner with language communities, tapping into their expertise and cultural understanding. This collaborative approach is vital for creating more accurate and culturally sensitive translations that truly resonate with the target audience.
The integration of OCR with AI translation tools could revolutionize how we handle low-resource languages, especially for accessing historically significant documents. Imagine being able to effortlessly translate ancient texts or archived historical materials. This has immense potential for preserving cultural heritage and making these texts accessible to a wider audience.
LLaMA 3's unique design allows its translation capabilities to adapt in real-time to user feedback. This interactive nature is particularly important for low-resource languages, allowing models to refine their understanding of nuanced language structures and improve translation accuracy incrementally. However, it's essential to acknowledge that enhancing low-resource language representation often comes with a hefty price tag. Specialized training methods and adaptive processes require substantial computing power, which might create a challenging trade-off between desired model accuracy and affordability.
Interestingly, researchers are finding that incorporating diverse forms of language data, like speech audio, can elevate translation performance for languages with limited resources. This multimodal approach allows models to consider phonetic and contextual information that is sometimes missing from textual data alone.
The quest to develop truly robust AI translation for low-resource languages is leading to the exploration of new model architectures. Hybrid approaches, which combine rule-based systems with machine learning techniques, are proving promising for scenarios where datasets are limited. This is a particularly active area of research.
Finally, it's worth acknowledging that phonetic complexities often play a significant role in the challenge of translating low-resource languages. Some languages have unique phonetic features that are poorly represented by standard written scripts, causing difficulties for AI systems in translating accurately between text and speech. Addressing these complexities requires a more granular understanding of each language's unique sounds and structure.
While we've seen tremendous strides with models like LLaMA 3, it's clear that building genuinely inclusive and effective AI translation systems requires a concerted effort to acknowledge and address the needs of low-resource languages. As the field progresses, there's a growing realization that a more nuanced approach is needed to ensure equitable access to these powerful technologies for all languages. The journey towards inclusive AI translation is still in its early stages, but the potential for meaningful breakthroughs is undoubtedly there.
AI-Powered PDF Translation now with improved handling of scanned contents, handwriting, charts, diagrams, tables and drawings. Fast, Cheap, and Accurate! (Get started for free)
More Posts from aitranslations.io: