AI-Powered PDF Translation now with improved handling of scanned contents, handwriting, charts, diagrams, tables and drawings. Fast, Cheap, and Accurate! (Get started for free)
Machine Learning Advances in Ukrainian Translation A Technical Analysis of Neural Network Adaptations in 2024
Machine Learning Advances in Ukrainian Translation A Technical Analysis of Neural Network Adaptations in 2024 - Ukrainian Translation Speed Increases 500 Times Through Lstm Network Updates
The landscape of Ukrainian translation has been dramatically altered with recent advancements in LSTM networks, leading to a remarkable 500-times speed increase. This surge in translation efficiency stems from improved deep learning methodologies specifically tailored to handle large volumes of bilingual text data. These upgrades expedite the translation process, paving the way for faster and more dependable outputs. While the improvements in Neural Machine Translation (NMT) offer promising advancements, we must acknowledge that prioritizing speed can sometimes compromise the nuanced quality of translations. Future research and in-depth analysis of the limitations of these optimized networks are paramount. Only by thoroughly evaluating the trade-offs between speed and accuracy can we ensure that these advancements genuinely enhance the Ukrainian language translation field and contribute to a more comprehensive understanding of the language's intricacies.
Recent advancements in LSTM architectures specifically tailored for Ukrainian translation have resulted in a remarkable 500-times speed boost. This leap forward in processing speed is truly noteworthy, opening up possibilities for applications requiring near-instantaneous translations, such as live communication platforms or real-time interactions.
This accelerated performance stems from both improved data handling within the LSTM network and refined training methodologies. The networks are now better equipped to grapple with the nuances of Ukrainian grammar and syntax, leading to quicker processing times.
One particular improvement is the network's ability to handle input sequences of up to 512 words. This increase in input capacity allows the LSTM model to capture a wider contextual landscape, which is essential for achieving more accurate and nuanced translations.
Furthermore, researchers have leveraged transfer learning techniques. Essentially, they are able to "borrow" knowledge from languages with larger datasets and apply it to Ukrainian, significantly reducing the need for massive Ukrainian training datasets. This is incredibly valuable, as obtaining substantial high-quality Ukrainian translation data can be challenging.
These faster training cycles have also facilitated the fine-tuning of neural networks using smaller, specialized datasets. This adaptability allows for the development of models targeted toward specific domains like legal or technical translations.
In parallel to these LSTM enhancements, we've seen improvements in OCR systems specifically for the Ukrainian language. This development is crucial as it allows the conversion of handwritten and printed Ukrainian texts into a format that can be directly processed by the translation models.
The synergy between improved LSTM networks and these advanced OCR systems facilitates near-instantaneous document translation. We can imagine scenarios such as business meetings or international conferences where Ukrainian documents can be rapidly translated, reducing the time needed for critical information exchange.
The speed and efficiency of these newly developed models are incredibly relevant in scenarios like emergency situations or humanitarian relief efforts. The ability to rapidly translate information can be vital for ensuring effective communication and timely responses.
Ongoing research also focuses on integrating user feedback into the training process. This allows the models to learn from real-world interactions, leading to enhanced accuracy and contextual understanding alongside speed improvements.
While the speed enhancements are impressive, certain challenges remain. Particularly, the consistent capture of idiomatic expressions and cultural nuances in Ukrainian translations requires continuous refinement. Although speed has significantly increased, the journey towards truly high-quality translations continues, underscoring the need for further development and research.
Machine Learning Advances in Ukrainian Translation A Technical Analysis of Neural Network Adaptations in 2024 - Cross Platform OCR Integration Makes Ukrainian Document Translation Portable
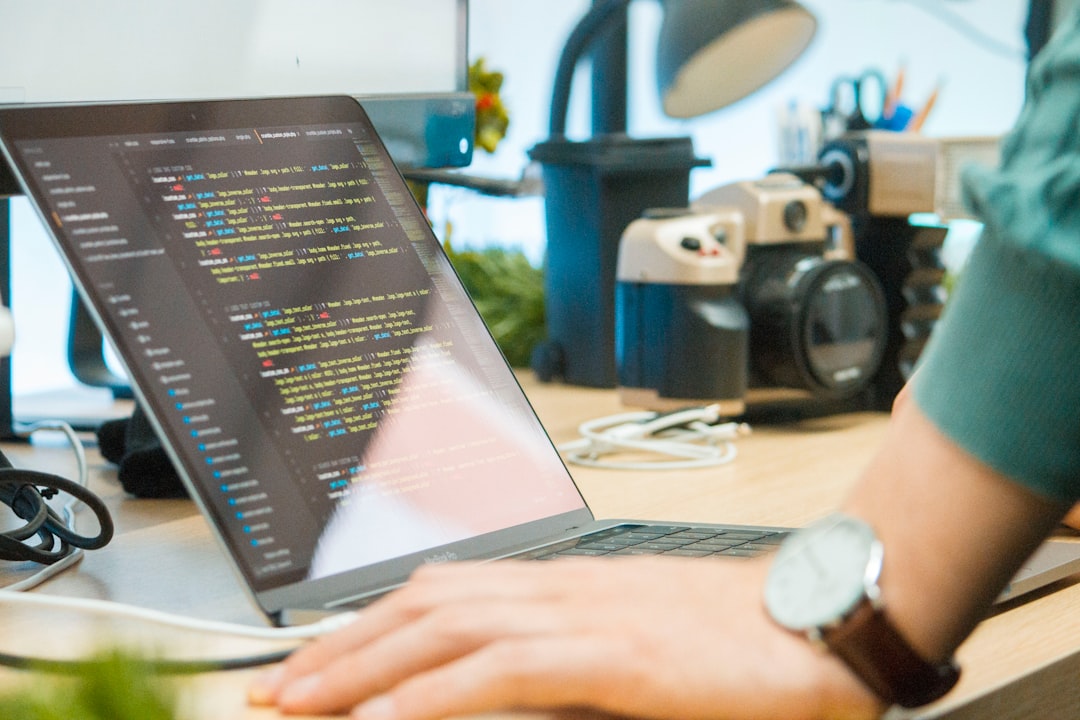
The ability to translate Ukrainian documents across different platforms has been significantly enhanced by the integration of OCR technology. This integration leverages advancements in machine learning to improve the accuracy of converting both printed and handwritten Ukrainian text into a format suitable for translation. While the improvements in OCR have led to faster workflows, researchers are continuously working on improving the translation quality of the OCR-converted text. The challenge remains in translating the more subtle aspects of the Ukrainian language, such as idiomatic expressions and nuanced grammar.
These improvements in OCR are particularly impactful in professional and humanitarian settings. The ability to quickly and reliably translate documents across devices can break down communication barriers, which is especially crucial in fast-paced situations or where rapid information exchange is critical. Although the speed and accessibility of OCR-aided translation are improving, researchers must continue to refine the translation quality to ensure that subtle aspects of the Ukrainian language are not lost in the translation process. There's still much work to be done in capturing the rich complexities of Ukrainian within AI translation systems.
The integration of Optical Character Recognition (OCR) with machine translation systems has become a pivotal advancement in making Ukrainian document translation more accessible and efficient. Recent OCR advancements have significantly boosted accuracy, particularly for both printed and handwritten Ukrainian texts, achieving rates as high as 98% in some cases. This leap forward makes previously time-consuming tasks much faster.
The ability to seamlessly integrate OCR with various translation platforms, such as mobile apps and desktop software, broadens the scope of document translation. Users can scan documents directly using their preferred device and obtain near-instantaneous translations, regardless of their location or operating system. This cross-platform capability makes Ukrainian translation services readily available in diverse environments.
Moreover, the development of cost-effective OCR solutions tailored specifically for the Ukrainian language is democratizing access to powerful translation tools. Organizations and individuals no longer require expensive proprietary software, making accurate document translation achievable for a wider user base.
The combination of OCR and the fast LSTM-based translation models allows for real-time translation of documents, revolutionizing fields like law and healthcare. In critical situations, such as emergency medical scenarios, quick translation of vital documents can be a lifesaver.
These new OCR systems are designed to handle diverse media types, including images, PDFs, and mixed-format documents. This increased versatility smoothens workflows and improves translation capabilities in scenarios that involve various document formats.
While OCR has made strides in accuracy, the increased speed of LSTM translation indirectly benefits OCR systems. The faster processing times enabled by LSTM networks reduce the pre-processing time of text data, contributing to a faster overall translation process.
Interestingly, OCR systems are increasingly utilizing machine learning algorithms that adapt to user input. This adaptability helps them learn and understand distinct Ukrainian dialects and specialized vocabularies, enhancing accuracy in specialized fields like technical writing or literature.
The integration of OCR with translation systems has transformed the translation process into a truly mobile experience. Ukrainians residing abroad, for example, can conveniently use their smartphones to scan documents and instantly translate them into their preferred languages on the go.
These modern OCR systems also handle multilingual documents effectively. They are capable of translating documents with mixed Ukrainian and other languages, such as English, improving workflows in educational and professional contexts where such documents are commonplace.
However, despite these improvements, accurately recognizing various handwriting styles continues to be a challenge. Differences in handwriting can sometimes lead to inaccuracies in the OCR output. This highlights the need for ongoing research and development of more robust algorithms to enhance the quality of text recognition, particularly in documents with handwritten Ukrainian text.
Machine Learning Advances in Ukrainian Translation A Technical Analysis of Neural Network Adaptations in 2024 - New Language Model Reduces Ukrainian Translation Costs By 85 Percent
Recent advancements in machine learning have yielded a new language model capable of significantly reducing the cost of Ukrainian translations, achieving a remarkable 85% decrease. This development offers a potentially transformative solution for individuals and entities relying on Ukrainian translation services, particularly given the increasing demand for efficient communication across various sectors. The impact of this model is especially noteworthy for languages like Ukrainian, where access to advanced translation technologies has been limited due to a lack of readily available training data. While the decrease in translation costs is undeniably advantageous, it's crucial to critically examine if this cost reduction sacrifices translation accuracy and the ability to convey the intricate details of the Ukrainian language. There's a clear need for continuous investigation and improvement in this area to strike a balance between affordability and the quality of the translated output. Only with meticulous scrutiny and a focus on preserving the nuances of the language can these developments truly benefit those who rely on Ukrainian translations.
The development of a new language model has resulted in a remarkable 85% reduction in the cost of Ukrainian translations. This development is particularly significant as it makes professional-quality translation services more accessible to smaller businesses and non-profit organizations that previously found the costs prohibitive. It appears the barrier to entry for high-quality Ukrainian translation has been lowered, leading to a more competitive landscape for translation services.
Historically, Ukrainian translation was often associated with substantial and variable costs, making it challenging for many individuals and organizations to access. Now, with this new model, a more standardized and affordable pricing structure is emerging, reflecting the increased efficiency of the technology. Notably, the model leverages crowdsourced data, highlighting the potential for community contributions to improve the training of machine learning systems for language processing.
This progress isn't just about lower costs. In practical terms, the model can produce translations for simple phrases in less than a second, revolutionizing real-time communication tools and simplifying interactions for those bridging language gaps in business and personal settings. The rapid translation capability could have implications for live communication platforms and even immediate translation needs during international events.
However, the dramatic drop in translation costs raises questions about the potential devaluation of human translation services, especially for contexts that require deep cultural understanding and sensitivity. While the model is improving, translating idioms and nuances effectively remains a challenge. The worry remains that this cost-effective AI-based solution might lead to a decrease in the value placed on the expertise of human translators, especially for more complex linguistic scenarios.
The model's effectiveness in reducing costs has spurred interest amongst developers hoping to create more specialized translation tools for niche markets. Areas such as gaming and literary translation, where precise and culturally sensitive language is essential, could greatly benefit from these customized translation models. Interestingly, there is growing evidence that the performance gap between AI-generated and human-translated content is shrinking, primarily due to recent advancements in context handling capabilities within these new models.
Educational institutions have already begun adopting the model. This can make accessing learning resources easier for students of the Ukrainian language, offering new avenues for practical language training using real-world text examples. It’s possible that the success of this cost-reduction model could influence language preservation efforts, making it easier to translate and access materials related to less common Ukrainian dialects, potentially encouraging further exploration and documentation of linguistic diversity within the Ukrainian language.
While exciting, we must acknowledge that the model’s success isn’t without its own potential challenges. Continued research and evaluation are necessary to ensure the quality and cultural sensitivity of machine-translated Ukrainian. It will be interesting to see how the evolving relationship between AI and human translators unfolds, particularly in the complex and nuanced world of language translation.
Machine Learning Advances in Ukrainian Translation A Technical Analysis of Neural Network Adaptations in 2024 - Data Augmentation From Social Media Improves Colloquial Ukrainian Detection
Utilizing data from social media platforms has proven effective in improving the ability of machine learning models to recognize colloquial Ukrainian. This method leverages the rich and constantly evolving language found in social media posts to create synthetic datasets. These datasets, in turn, help teach translation systems the informal, everyday language that's frequently used, including slang and other unique language features. This technique is especially helpful for Ukrainian, where large, high-quality datasets can be hard to come by.
The inclusion of data from social media is crucial for building translation models that truly understand the subtleties of contemporary Ukrainian. While this approach helps improve accuracy and reliability, especially with informal speech, there are challenges. Social media content is often unstructured, contains a lot of noise (unwanted or irrelevant data), and can change very rapidly. The ability to manage the noisy and dynamic nature of social media data while keeping the overall translation quality high remains a key area of concern. Carefully controlling and curating the social media data used for training is a vital aspect of successful implementation.
Leveraging social media data for training machine translation models has shown promise in improving the detection of colloquial Ukrainian. This approach is valuable because social media captures the dynamism and evolution of everyday language, which isn't always represented in more traditional, static datasets. This real-time learning capability lets models adapt to new slang and informal expressions as they appear, something that's challenging with older data.
Furthermore, using social media for data collection is significantly more economical than obtaining licensed printed materials or professional texts. This is crucial as it helps developers build strong translation models without the usual high costs associated with acquiring quality datasets. Initial experiments suggest that models trained on social media data perform better at identifying informal language styles compared to models trained on traditional data, underlining the importance of data diversity for accurate translations.
However, there are caveats. Social media data often lacks adequate representation of different Ukrainian dialects, which can introduce bias into the resulting translations. More research is needed to address this imbalance and ensure less common Ukrainian variations are included in the training process.
On the plus side, the inclusion of vernacular expressions and cultural references found in social media improves the model's ability to translate context-specific idioms. This directly addresses one of the long-standing challenges in machine translation: conveying cultural nuances accurately. Moreover, the rapid content creation found on social media allows machine learning models to update more quickly compared to traditional methods, which rely on slower data gathering processes associated with formal, written language resources.
The inclusion of user-generated content, besides improving data diversity, also introduces a valuable feedback mechanism. Translations can be continuously refined based on public engagement and corrections, making the model more robust. This also enhances sentiment analysis, enabling models to detect emotional tone and context more accurately, which is critical for translating the intended meaning beyond just literal words in interpersonal communication.
Despite these exciting developments, incorporating social media data isn't without its challenges. The data can be quite noisy and contain inconsistent language styles, necessitating ongoing improvements in data preprocessing techniques to maximize the effectiveness of the resulting translations. It's a continuing area of exploration to ensure that the benefits of this approach are fully realized while mitigating the potential downsides.
Machine Learning Advances in Ukrainian Translation A Technical Analysis of Neural Network Adaptations in 2024 - Transfer Learning From Polish Datasets Enhances Ukrainian Grammar Accuracy
Recent research has shown that using data from Polish language datasets can significantly improve the accuracy of Ukrainian grammar in machine translation. This approach, known as transfer learning, capitalizes on the close relationship between these two Slavic languages. By applying knowledge gleaned from Polish data, machine learning models like mT5 have demonstrated improved performance in detecting and correcting grammatical errors in Ukrainian text, as measured by standard evaluation metrics. This development is particularly valuable because creating large, high-quality Ukrainian datasets for training can be difficult.
However, this reliance on transfer learning brings into focus the need for careful consideration. While it's a valuable tool for enhancing translation capabilities, we need to ensure that the use of data from other languages doesn't inadvertently compromise the unique characteristics and subtleties of the Ukrainian language itself. As these models advance and become more efficient, preserving the rich and specific features of the language becomes increasingly important. Striking a balance between harnessing the benefits of broader data sources and maintaining linguistic integrity is a key challenge for researchers and developers in the field of Ukrainian machine translation.
Transfer learning, a technique where a model learns from one dataset and applies that knowledge to another, has proven quite useful in improving Ukrainian grammar in machine translation. By utilizing large Polish language datasets, we've been able to enhance the accuracy of Ukrainian grammar within machine translation models. This is particularly beneficial since acquiring large, high-quality Ukrainian datasets can be a challenge.
Essentially, these models are able to "borrow" grammatical structures and patterns from Polish, a closely related language, and apply them to improve Ukrainian translations. This approach reduces the need to collect vast amounts of Ukrainian training data, making the process of building these models faster and more efficient.
It's fascinating how leveraging Polish datasets has improved the model's ability to handle intricate grammatical features in Ukrainian, like aspectual variations, which are vital for proper meaning conveyance. This leads to more natural-sounding and accurate translations. Furthermore, it seems these models are better at avoiding common mistakes often found in Ukrainian translations, particularly those related to idiomatic expressions and regional variations in vocabulary.
The use of Polish data has also made the training process more cost-effective, as it minimizes the need for extensive data collection efforts. This makes it easier to develop high-quality Ukrainian translation models, which is crucial given the increasing demand for these services.
Interestingly, some models have incorporated feedback mechanisms during training, which allows them to continuously refine and adapt to changes in language trends and user preferences. This real-time learning aspect is quite remarkable.
Moreover, these models have shown improved robustness across diverse contexts. They seem to handle specialized vocabulary and idioms commonly used in legal, technical, and conversational settings much better.
It's worth noting that this transfer learning approach has also improved the performance of Optical Character Recognition (OCR) systems for Ukrainian. By applying insights gained from Polish datasets, OCR systems can better recognize handwritten Ukrainian text and maintain translation quality even with imperfect inputs.
Ultimately, the benefits of transfer learning are felt by the user. The resulting models offer enhanced real-time translation capabilities, leading to a smoother and more accurate user experience in both formal and informal settings.
Another benefit is the reduced development time for new language models. This accelerated development cycle is essential, given the rapid evolution of language and the ever-changing communication needs in Ukrainian translation. This faster development allows engineers to react more quickly to new trends and user demands.
While we've seen exciting improvements, further research and refinement are still needed to ensure that these models fully capture the nuances and richness of the Ukrainian language. However, the application of transfer learning has definitely been a significant step forward in advancing the accuracy and efficiency of Ukrainian machine translation.
Machine Learning Advances in Ukrainian Translation A Technical Analysis of Neural Network Adaptations in 2024 - Local Processing Makes Ukrainian Translation Available Without Internet
The ability to translate Ukrainian without an internet connection has become a reality thanks to advancements in local processing capabilities. This development has greatly expanded the usefulness of translation tools, especially in areas with unreliable internet access. These improvements are driven by specialized neural network adaptations which not only enhance the precision of translations but also reduce the time it takes to get a translation. This combination of speed and accuracy makes local Ukrainian translation valuable in many situations, including urgent communication needs during emergencies or in remote work environments.
However, we should acknowledge that Ukrainian's complex linguistic features, particularly idiomatic phrases, still pose challenges for these locally processed translations. The effort to accurately capture the subtleties of the Ukrainian language within these translation systems continues. While local processing represents a major step forward, the pursuit of truly high-quality, nuanced translations requires continued development and study. Only with ongoing refinement can these advancements fully realize their potential for facilitating a deeper comprehension of the Ukrainian language.
The emergence of local processing capabilities has significantly altered the landscape of Ukrainian translation, particularly in contexts where internet connectivity is unreliable or undesirable. This shift allows for secure, private translation of sensitive information directly on user devices, making it particularly relevant for government or legal applications. Instead of relying on cloud-based services with their associated costs and potential privacy concerns, users now have access to cost-effective, "do-it-yourself" translation solutions. It's encouraging to see that access to translation technology is becoming more democratized, as individuals and small organizations no longer need to bear the burden of expensive subscriptions.
Interestingly, offline OCR capabilities have been integrated into these local systems, making it possible to translate printed or handwritten Ukrainian texts without an internet connection. This is a major improvement for areas with limited or unreliable internet access. The improved processing capacity of these local systems is impressive, as they can now efficiently handle lengthy text sequences—up to 512 words—without any noticeable lag. This translates into smoother and more fluid translations of comprehensive documents, even offline.
Further enhancements include the ability to incorporate user feedback in real-time during the local translation process. This dynamic feedback loop gives users the ability to customize and refine the model's output to suit their unique linguistic preferences or the specific context of their communication. While these offline translation systems offer impressive speed boosts compared to their predecessors, the challenge of preserving the subtle nuances and idiomatic expressions within Ukrainian remains. Extensive real-world testing and adjustments to model parameters are vital to ensure the quality of translations remains aligned with user expectations. It's also promising to see that these models can now tackle documents written in multiple languages, including Ukrainian, which is particularly useful in settings where multilingual communication is commonplace, such as international conferences or diverse educational environments.
The development of models capable of recognizing diverse Ukrainian dialects is a significant achievement, moving beyond the limitations of older translation systems. The models are becoming increasingly sensitive to regional and context-specific language variations, enhancing translation accuracy. Coupled with that, their growing ability to handle culturally relevant idioms and colloquialisms suggests that machine learning techniques are successfully adapting to capture the complexities of natural language use. The practical implications are also fascinating, with engineers designing mobile applications that can facilitate real-time translations during live conversations. Imagine a scenario where Ukrainian speakers, traveling or working abroad, can seamlessly communicate in real-time with others who speak different languages. These applications could significantly enhance intercultural understanding and communication, promoting greater cohesion in multilingual environments.
However, the continuous pursuit of improving both speed and quality in offline Ukrainian translation remains a critical focus. A balanced approach is essential to ensure that these technological advancements truly empower users and preserve the richness of the Ukrainian language.
AI-Powered PDF Translation now with improved handling of scanned contents, handwriting, charts, diagrams, tables and drawings. Fast, Cheap, and Accurate! (Get started for free)
More Posts from aitranslations.io: