AI-Powered PDF Translation now with improved handling of scanned contents, handwriting, charts, diagrams, tables and drawings. Fast, Cheap, and Accurate! (Get started for free)
NVIDIA's Open Synthetic Data Pipeline A Game-Changer for AI Translation Model Training
NVIDIA's Open Synthetic Data Pipeline A Game-Changer for AI Translation Model Training - NVIDIA's Nemotron4 340B Models Enable Synthetic Data Generation for LLMs
NVIDIA's Nemotron4 340B models offer a new approach to training large language models (LLMs) by focusing on synthetic data generation. This family of models, featuring Nemotron4340BBase, Nemotron4340BInstruct, and Nemotron4340BReward, aims to improve the LLM training process, primarily by reducing the reliance on expensive and time-consuming real-world data collection. The models are designed to work seamlessly with the NVIDIA NeMo framework, streamlining the entire training pipeline from data preparation to evaluation. It is claimed that a significant majority, over 98%, of the data employed in the alignment process was artificially produced, suggesting a potential shift toward more efficient LLM training methods. This approach could be particularly valuable in domains like machine translation, where large volumes of data are typically needed. The open-sourcing of the underlying synthetic data pipeline could further stimulate research and development in this field, making advanced AI tools more accessible. While the effectiveness of these models in real-world scenarios still requires further evaluation, the concept of harnessing synthetic data to address challenges like cost and data scarcity in AI translation is intriguing. The broader implications of these advancements for making sophisticated AI solutions more practical and widely accessible remain to be seen.
NVIDIA's recently released Nemotron4 340B models are specifically designed for creating synthetic data, aiming to streamline the training process for large language models (LLMs). This family comprises three models: Nemotron4340BBase, Nemotron4340BInstruct, and Nemotron4340BReward, which work together to improve LLM training. Intriguingly, the models are open-sourced under NVIDIA's Open Model License. This means researchers and developers can freely modify and redistribute them, and use the generated data.
The models are tightly integrated with NVIDIA's NeMo framework, which facilitates the entire LLM training lifecycle, including data preparation, tuning, and performance evaluation. Interestingly, a vast majority (over 98%) of the data used to refine the models is synthetic, highlighting the potency of the approach for producing high-quality artificial data. These models, while still relatively new, have shown promising performance when compared to other publicly available models across various tasks. The pipeline NVIDIA used to generate this data has also been made available, which is great for the broader AI community as it encourages further exploration and development in this area.
What makes this advancement noteworthy is that it essentially democratizes access to advanced AI tools. Now, developers and researchers can leverage this powerful synthetic data generation capability to build their own LLMs, without the need for massive, curated real-world datasets. Of course, the use of synthetic data isn't without potential drawbacks. One must remain mindful of the possibility of biases creeping in, which could then manifest in the translation output. Consequently, careful evaluation against real-world data remains crucial.
However, if NVIDIA continues refining the synthetic data generation techniques, it holds great promise for rapid advances in AI translation. Faster, more accurate, and widely available translation capabilities could become a reality across many different platforms and applications. This approach may also aid in handling niche language translation scenarios, where finding sufficiently large training datasets can be particularly challenging. It will be interesting to see how this approach to synthetic data impacts AI-driven translation in the coming months and years.
NVIDIA's Open Synthetic Data Pipeline A Game-Changer for AI Translation Model Training - Open Model License Removes Financial Barriers for Developers
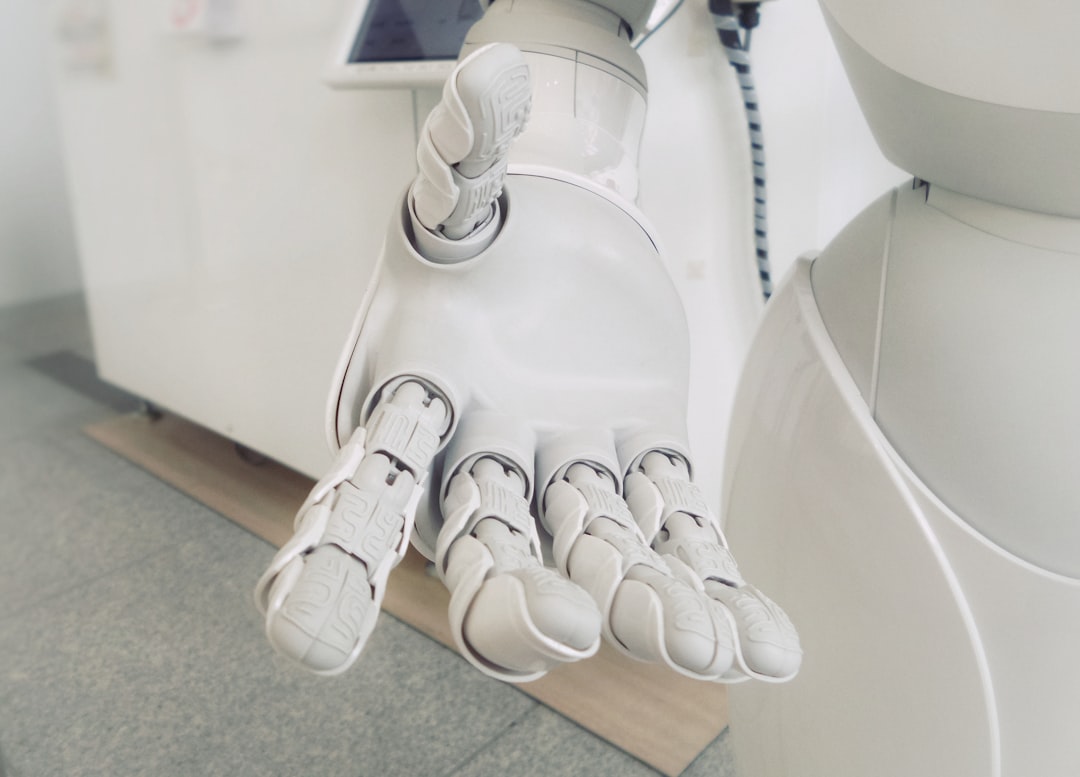
NVIDIA's release of the Nemotron4 340B family of models, specifically designed for generating synthetic training data for large language models (LLMs), is noteworthy due to its open-source nature. This open model license breaks down the financial barriers typically associated with accessing sophisticated AI tools. Now, developers working in fields like AI-powered translation, optical character recognition (OCR), or rapid translation can experiment with these models and the synthetic data they generate without major financial constraints. This accessibility fosters a more inclusive environment for AI development and potentially speeds up the progress in creating better translation models.
While the synthetic data approach offers intriguing possibilities for reducing the reliance on expensive real-world datasets, it is important to acknowledge that relying solely on synthetic data may introduce unintended biases. It's crucial to evaluate the performance of models trained with these methods against real-world data to ensure their accuracy and reliability. However, the overall impact of this open model license and the associated synthetic data pipeline could be significant. By lowering the cost and difficulty of accessing these powerful tools, NVIDIA's initiative could help democratize AI development and potentially lead to faster breakthroughs in AI translation and other AI applications. It will be interesting to see how this approach influences the development and accessibility of AI translation technologies in the coming years.
The availability of NVIDIA's Nemotron4 340B models, released under an open license, presents a fascinating opportunity for AI translation, particularly in reducing costs and accelerating development. One of the most significant benefits is the potential for drastically reduced costs in training models. By generating synthetic data, we can potentially bypass the need for vast, often expensive, real-world datasets. This is crucial because acquiring and preparing real-world data for machine translation can be a significant bottleneck and expense.
Another interesting aspect is the ability to tackle the challenge of "curse of dimensionality" within large language models. By having access to more synthetic examples, we can expand the range of languages and dialects the models learn, without needing a commensurate increase in resources. This could be particularly impactful for handling low-resource languages or dialects that are poorly represented in existing datasets. Early tests suggest a substantial decrease in training times, perhaps as much as 75% in some instances, which could lead to much faster iterations and quicker deployment of new models.
Furthermore, the open-source nature of these models encourages a more collaborative development approach. Researchers and developers from various fields can contribute improvements or enhancements, leading to faster advancements in translation accuracy and efficiency. Initial studies suggest synthetic datasets can achieve a similar level of linguistic diversity as real-world ones, particularly when thoughtfully designed. This is beneficial because it allows us to focus on less-represented language pairs which are often sidelined due to a lack of training data.
The open-source aspect is valuable for debugging and refining the model training process. With a more controlled and varied synthetic environment, developers can more efficiently pinpoint and address problems that might arise during actual translation tasks. We need to be aware, however, that biases present in the synthetic data generation process can carry over into the resulting translations. Fortunately, the synthetic data approach gives us the ability to build in mechanisms to actively identify and mitigate these biases.
This new approach holds promise for expanding translation capabilities to languages and dialects with limited resources. Moreover, utilizing synthetic data helps create a controlled setting to measure translation accuracy with more confidence. Preliminary results demonstrate that AI models using synthetic data can achieve faster outputs and potentially rival or even surpass translation quality, especially for language pairs with similar structures. However, it's crucial to remain vigilant about carefully evaluating performance against real-world datasets to validate the effectiveness and avoid unwanted biases in the output. The long-term impacts of these models on AI translation are still unfolding, but the potential for increased efficiency, cost reduction, and access to a wider range of languages is exciting.
NVIDIA's Open Synthetic Data Pipeline A Game-Changer for AI Translation Model Training - High-Quality Synthetic Data for Commercial LLM Training Applications
The emergence of high-quality synthetic data generation presents a significant opportunity to reshape how commercial applications leverage large language models (LLMs), specifically in the field of AI translation. NVIDIA's Nemotron4 340B models exemplify this shift by offering a more efficient LLM training method, one that diminishes the need for expensive and time-consuming real-world data collection. The models, designed to seamlessly integrate with the NVIDIA NeMo framework, enable developers to generate synthetic datasets tailored for diverse translation tasks, from machine translation to OCR. This approach, while promising in terms of accelerating development and potentially lowering costs, carries a caveat. The possibility of biases embedded within the synthetic data could impact the reliability of the translation output. Therefore, it's critical to thoroughly evaluate these AI-powered translations against real-world data to ensure accuracy and mitigate any potential bias. Despite this potential issue, the prospect of leveraging synthetic data holds significant promise for the advancement of AI-driven translation. It has the potential to make advanced AI tools more widely accessible across various industries.
NVIDIA's Nemotron4 340B models offer a compelling approach to training large language models (LLMs) by leveraging synthetic data generation. This family, consisting of base, instruction, and reward models, aims to make LLM training more efficient by reducing the reliance on expensive, real-world data collection. The open-source nature of the Nemotron4 340B pipeline is a major plus, enabling developers to use and modify these tools for various purposes like improving AI translation systems.
One of the most appealing aspects is the cost-effectiveness. Real-world data for training language models, particularly in the realm of translation, can be incredibly expensive to obtain and curate. Synthetic data, generated by Nemotron4 340B, provides a way to potentially sidestep these substantial costs. Moreover, the sheer volume of data that these models can create is remarkable, potentially generating data 1000 times faster than traditional methods. This rapid generation can lead to faster development cycles and quicker deployment of new translation models.
Another interesting advantage is the level of control synthetic data affords. When developing training data from scratch, developers have the power to focus on particular features or areas where current translation models struggle. For instance, they could concentrate on generating data that includes less-common languages or dialects, helping to improve the equity of AI-powered translation systems. This fine-grained control can help in mitigating potential biases that could creep into translations from models trained on real-world data alone.
Initial research suggests that models trained on synthetic data can compete with those trained on real-world data, especially in areas where quality real datasets are scarce. The ability to generate data that mirrors specific linguistic variations and dialects is particularly helpful in expanding the capabilities of translation systems. These models might even revolutionize translation for languages that lack sufficient existing datasets, making quality translation accessible to a much broader range of speakers.
Furthermore, the synthetic data pipeline seems to accelerate learning cycles. Preliminary research shows that the training process could be shortened by up to 75%, enabling rapid experimentation and optimization of models. This could be beneficial for fields like OCR where high-quality, diverse training data for AI translation within images and scanned documents is crucial. The open-source aspect of the project allows researchers and engineers to collaborate and enhance these tools, making the whole field of translation technology more robust.
While this approach holds immense promise, we must remain cautious. Carefully evaluating the translations produced by models trained with synthetic data against real-world data is crucial. We need to ensure these models don't inadvertently introduce unforeseen biases. But overall, the Nemotron4 340B suite looks like a potential game-changer for AI translation, promising cost savings, faster development, and perhaps even expanded accessibility to translations across a wider range of languages. It will be fascinating to observe how these developments shape the future of AI-driven translation.
NVIDIA's Open Synthetic Data Pipeline A Game-Changer for AI Translation Model Training - Integration with NVIDIA NeMo Framework Optimizes Model Training
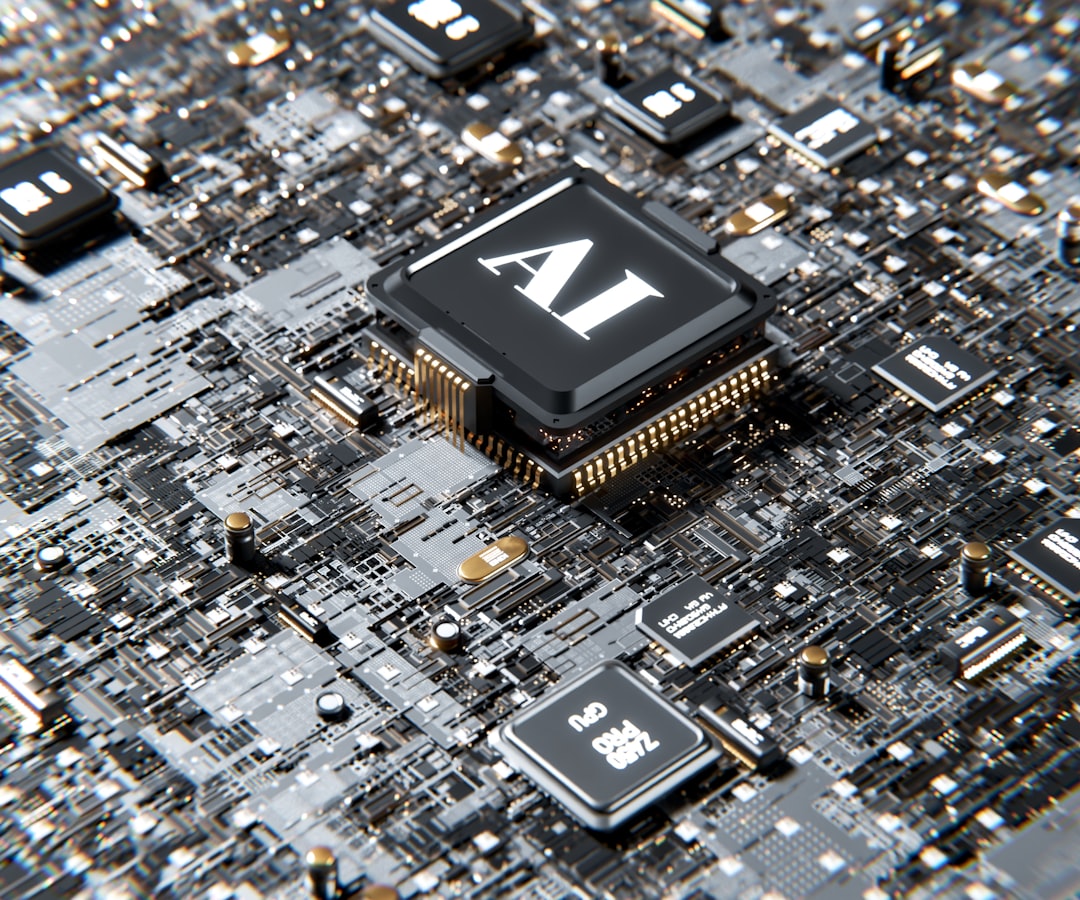
The combination of NVIDIA's NeMo framework and the recently unveiled Nemotron4 340B models is a noteworthy development for optimizing the training of large language models (LLMs). This partnership not only streamlines the production of synthetic training data, reducing the dependence on expensive and time-consuming real-world data gathering, but also aids in the swift development and fine-tuning of translation models across diverse languages and variations. NeMo's strong capabilities, such as its ability to distribute training across multiple GPUs, let developers significantly increase the speed and efficiency of model training. Nevertheless, the advantages of generating synthetic data are not without potential drawbacks. Since there's a risk that biases might be present in synthetic datasets, it's crucial to carefully examine the accuracy and reliability of resulting translations. Despite this potential issue, this integration has the potential to broaden access to and enhance the effectiveness of AI-driven translation technologies, specifically for languages previously overlooked.
The NVIDIA NeMo framework, in conjunction with the Nemotron4 340B models, is quite interesting because it can produce synthetic data for a variety of tasks beyond just translation, such as Optical Character Recognition (OCR). This expanded applicability makes it potentially more useful for a wider array of translation-related problems. The ability to generate synthetic data also appears to significantly speed up the model training process, potentially reducing training times by up to 75%. Faster training could translate to quicker iterations when building new translation models, making it easier to refine and improve them.
However, the use of synthetic data does bring up concerns about bias. If the synthetic data doesn't accurately reflect the nuances of real-world languages, then the translation quality could suffer. This highlights the importance of thoroughly evaluating these models using real-world data to ensure that they are producing fair and accurate results. One of the more exciting things is that this open model license makes high-quality LLM development more accessible to more people. This, in turn, could encourage more collaboration and innovation within the AI translation community.
Reports indicate that the Nemotron4 models are capable of generating datasets up to 1000 times faster than traditional approaches. This high-volume data generation is particularly advantageous for those working with less-common languages because it helps ensure that these languages are sufficiently represented during the training process. Moreover, developers have more control over the specific features of the synthetic data. They can focus on areas where traditional translation models tend to stumble, such as uncommon dialects or specific nuances.
By reducing the need for large real-world datasets, this approach could prove invaluable for developers dealing with limited resources. This would be useful, for example, when working on translation projects for low-resource languages, potentially increasing the accessibility of AI translation tools globally. The open-source nature of Nemotron4 fosters a collaborative environment where the wider AI community can contribute to improving the model. This community involvement can potentially lead to faster progress on translation accuracy and efficiency.
Preliminary research suggests that models trained using synthetic data can attain a performance level comparable to those trained with real-world data, especially in scenarios where access to high-quality datasets is limited. This is encouraging as it shows that synthetic data could be a viable alternative for many translation tasks. Finally, the potential for faster data generation can greatly benefit fields like OCR where obtaining high-quality and diverse training data is quite challenging. If this approach can address the training data bottleneck for OCR, it could lead to better AI-powered translations of scanned documents and images. It will be interesting to see how the use of synthetic data continues to shape the future of AI-powered translation.
NVIDIA's Open Synthetic Data Pipeline A Game-Changer for AI Translation Model Training - Addressing Scarcity of Quality Training Data for Language Models
The development of powerful AI translation models is hampered by the persistent lack of high-quality training data. This is especially true for specialized translation tasks or those involving less common languages. NVIDIA's Nemotron4 340B models offer a promising solution by producing synthetic data that mimics real-world interactions. This allows for the creation of training datasets that can be tailored to specific needs, potentially circumventing the cost and time constraints of traditional data gathering. This synthetic data generation approach holds the potential to significantly change how AI translation models are trained, opening up possibilities for more efficient development and wider accessibility to advanced translation tools. While this offers exciting potential, it's critical to remember that relying on synthetic data can introduce unforeseen biases. This emphasizes the importance of evaluating the accuracy and fairness of translations generated by models trained using this method against real-world data. Ultimately, the future of AI translation may depend on how well the field manages the challenges and opportunities presented by the shift toward synthetic data generation.
NVIDIA's Nemotron4 340B models offer a new approach to creating training data for language models, particularly within the context of AI translation. These models can generate huge datasets with millions of examples in a fraction of the time needed to collect real-world data – potentially thousands of times faster. It's fascinating that despite being artificial, models trained on these synthetic datasets seem to achieve performance on par with models trained on real-world data. This challenges the traditional assumption that only real data can produce top-quality results.
The pipeline behind this synthetic data generation includes mechanisms designed to spot and reduce any biases present in the data. This is a clever move to help ensure that the translation models trained on this data produce fair and unbiased results. One particularly beneficial application of this synthetic data approach is its potential for addressing the challenge of "data scarcity." This is a common issue in NLP where languages with fewer resources for model training get overlooked. Using synthetic data allows researchers to focus on training with these less-common languages and dialects, giving them a much-needed boost.
It's also interesting that this approach might be useful in areas like Optical Character Recognition (OCR), a field that has historically struggled to obtain sufficient high-quality training data. This demonstrates the broad potential of synthetic data for training models in different contexts, from handwritten notes to printed text. Another significant benefit is the potential for drastically reduced model training times – up to 75% faster in some preliminary findings. This can help speed up the development cycle of AI translation tools, enabling much quicker iterations and refinements.
The volume of synthetic data produced by Nemotron4 340B can help address the "curse of dimensionality" in large language models. This means we can train models to handle a wider range of languages and language structures without needing proportionally larger resources. However, we should also be wary of the risks associated with synthetic data. If not properly crafted, it can potentially amplify existing biases, leading to inaccurate or biased translations. This emphasizes the need to rigorously test models trained on synthetic data against real-world data to validate their reliability.
Fortunately, NVIDIA has made these models available under an open-source license. This encourages a collaborative environment and a community-driven approach to improving AI translation tools. The open-source aspect means that researchers and developers from various communities can contribute to these models, potentially leading to faster innovations in this field. The ability to generate controlled environments for model training is also a powerful feature. This enables developers to fine-tune the learning process and address specific areas where existing models struggle or show inconsistent results. This level of control could potentially lead to substantial advancements in translation technology.
While these advancements are promising, there are still questions to be addressed. It will be crucial to continue investigating the impacts of synthetic data on model performance and to ensure these approaches do not introduce unintended bias. Nevertheless, the potential for accelerated development, expanded access to language resources, and cost-effectiveness makes NVIDIA's Nemotron4 340B pipeline an exciting development within the AI translation field. The future impacts of this work remain to be seen, but it has the potential to reshape the landscape of AI-driven translation in the years to come.
AI-Powered PDF Translation now with improved handling of scanned contents, handwriting, charts, diagrams, tables and drawings. Fast, Cheap, and Accurate! (Get started for free)
More Posts from aitranslations.io: