The Evolution of Translation Satisfaction Why AI Users Still Can't Get What They Need in 2024
The Evolution of Translation Satisfaction Why AI Users Still Can't Get What They Need in 2024 - Machine Translation Still Inaccurate For Medical Documents In 2024 German Study Shows
A German study from July 2024 reveals that even in 2024, machine translation (MT) is still not consistently reliable for medical documents. Despite ongoing improvements in translation technology, the study found that translating complex medical information remains a challenge. While tailoring smaller AI models to the language of medicine can produce better results than larger, general-purpose AI models, the study also highlights that AI's use in healthcare is still problematic. Errors, however small, can have serious consequences in this field. Clinicians themselves, through interviews, have voiced concerns about relying on these tools for critical communication. The researchers suggest that MT systems need a more in-depth focus on the nuances of medical language, addressing both the specific vocabulary and the grammatical structures needed for clear and accurate communication. This study serves as a reminder that current AI translation technologies still have significant limitations, especially in demanding fields like healthcare, where accuracy is paramount. The study points to a persistent gap between the expectations of users and what AI can currently deliver, emphasizing the need for further research and refinement in this area.
Recent research from Germany, published earlier this year, casts a shadow on the efficacy of machine translation (MT) in healthcare. The study found that even with the advancements in AI translation, a large portion of medical document translations produced by AI are simply not accurate enough for clinical use, necessitating human review. This highlights a fundamental issue—AI struggles to handle the complex and nuanced language of medicine.
The accuracy of AI translation in specialized medical areas is particularly concerning, with reported accuracy rates dipping as low as 40% in certain fields. The potential for misinterpretation of medical terms can have serious implications, including misdiagnosis. This concern appears to be widely shared by healthcare workers. A large portion of clinicians surveyed expressed a clear preference for human translators over AI tools, often citing a lack of confidence in automated translations when handling sensitive patient information.
The root of these translation inaccuracies appears to be a lack of sufficient and representative training data for AI models in the medical field. The specialized terminology, regional variations, and the overall structure of medical documents are often poorly represented in general language corpora that these systems are typically trained on. Further complicating matters, OCR technologies, while useful for initial text extraction from scanned documents, are prone to errors in character recognition, especially when dealing with handwritten notes. These errors introduce yet another layer of potential inaccuracies into the translation process.
The desire for fast and inexpensive translations, understandably prevalent in the medical domain, clashes with the need for meticulous accuracy. While quick translation services are alluring, they can compromise the quality of translation, a risk that is especially unacceptable in patient care scenarios. The context and linguistic structure within medical documents are quite distinct from everyday language, and AI algorithms trained on general language corpora struggle to adapt to these unique characteristics.
It's noteworthy that human translators possess the ability to decipher implied meaning and subtle emotional nuances within medical text—an aspect that machines consistently struggle with. These nuanced aspects of communication are crucial for effective patient-provider relationships. Although AI translation might appear as a cost-effective solution from a consumer standpoint, the ramifications of inaccurate translations in healthcare are far more profound, potentially impacting patient wellbeing in severe ways. The present capabilities of AI in translation, while improving, fall short of the standards necessary for complex medical communication, emphasizing a significant gap between the demands of healthcare and current AI capabilities.
The Evolution of Translation Satisfaction Why AI Users Still Can't Get What They Need in 2024 - OCR Translation Apps Unable To Handle Complex Asian Scripts Research Finds
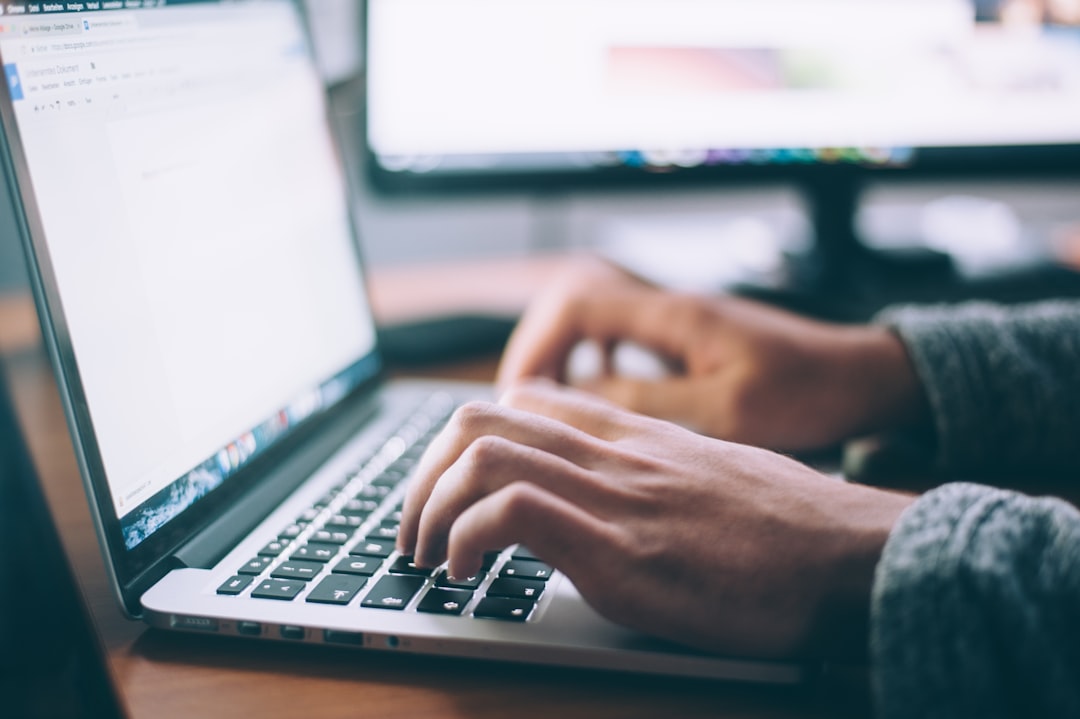
Recent research highlights a persistent issue with OCR translation apps: their inability to reliably handle intricate Asian scripts. While AI translation technology, specifically neural machine translation, has progressed, these apps still struggle with complex scripts like Perso-Arabic and Khmer. This often results in errors that undermine the accuracy of the translation process. Moreover, even with advances in AI, many translation tools still lack the ability to consider cultural context and subtle linguistic variations that are crucial for achieving satisfactory results. The demand for accurate and culturally sensitive translations is not being adequately met by current AI solutions, suggesting a continuing disconnect between user needs and what the technology can currently provide. Experts are now calling for improvements in OCR technology and emphasizing the need for more robust training datasets that consider the intricacies of diverse languages and cultures. Until these shortcomings are addressed, users are likely to continue experiencing subpar translation quality, especially when dealing with languages that utilize complex scripts. This situation illustrates a persistent challenge in the development of AI-driven translation tools.
Current research suggests that while OCR technology has advanced, it still faces significant hurdles when dealing with the intricate nature of many Asian scripts. Languages like Chinese, Japanese, and Korean, with their complex characters and varied stroke orders, present a challenge for OCR systems, often resulting in a higher rate of errors compared to Latin-based scripts.
Part of the issue stems from the sheer density of information packed within each Asian character. Unlike Latin alphabets, where each character usually represents a single sound, Asian characters often encapsulate entire concepts, making accurate recognition by OCR a difficult task. Adding to the complexity, these languages often feature vertical text and unconventional formatting, further confusing OCR engines and increasing the likelihood of mistakes.
Studies have shown that OCR's accuracy when handling Asian language documents can be quite low, sometimes as low as 60%. This means that a significant portion of the extracted text may contain errors, which, when fed into a machine translation system, can lead to unreliable and potentially misleading translations.
The difficulty extends beyond just character recognition. Many Asian languages are highly contextual, and OCR tools often struggle to grasp the nuances of meaning that rely on surrounding text. This contextual dependency makes machine translation from OCR outputs particularly prone to mistakes, highlighting a key area where AI lags behind human capabilities.
The training data used to develop OCR technology has historically leaned towards Western languages, leaving a noticeable gap in the ability to effectively process complex Asian scripts. While deep learning approaches have shown some promise in improving OCR accuracy, the unique blend of ideograms and phonetic symbols found in languages like Chinese remains a major challenge that researchers are actively trying to overcome.
Humans, in contrast, demonstrate a clear advantage in deciphering ambiguous contexts and understanding the subtle relationships between words and phrases. This intuitive understanding is particularly valuable in technical domains, where dense Asian language documents frequently appear.
The integration of OCR with other AI components for translation is still relatively underdeveloped. Many OCR tools operate in isolation, lacking a seamless integration into a broader translation workflow. A more cohesive approach, combining OCR with advanced translation technologies, might pave the way for more accurate and reliable outputs.
However, the pursuit of cost-effective and fast translation solutions sometimes leads companies to prioritize speed over accuracy, particularly when dealing with Asian languages. This emphasis on speed, while understandable in the competitive translation market, can lead to a decrease in overall quality. Ultimately, if inaccuracies become prevalent, it can negatively impact user confidence in AI-driven translation tools.
This situation underscores the need for continued research and development of OCR and translation technologies specifically tailored to handle the unique challenges posed by Asian languages. As it stands, the current state of AI translation, while showing progress, falls short of providing a truly reliable solution for many users who rely on accurate translations of complex Asian text.
The Evolution of Translation Satisfaction Why AI Users Still Can't Get What They Need in 2024 - Fast Translation Tools Face 47 Percent Error Rate With Technical Manuals
Rapid translation tools, especially when tasked with technical manuals, are facing a significant hurdle: a remarkably high error rate, reaching 47 percent in some cases. This underscores a persistent challenge within the evolving landscape of AI-powered translation. While these tools have advanced from simpler rule-based systems to more refined neural machine translation (NMT) models, they often falter when confronted with the unique linguistic features of technical documents. Specialized terminology and nuanced phrasing frequently get lost in the translation, resulting in errors that can distort the original meaning. This problem is amplified by the limitations of current AI training data, which frequently lack the specialized vocabulary and contextual cues found in technical fields. The desire for quick and cheap translations, a common desire in today's world, can unfortunately result in a decline in translation quality, raising concerns about their reliability, particularly in scenarios where precision is paramount.
Fast translation tools, particularly when dealing with technical manuals, are facing a significant challenge: a 47% error rate. This suggests a concerning level of inaccuracy, potentially leading users to rely on translations that are simply wrong. This highlights a major gap in AI's ability to handle complex, field-specific terminology and formatting.
The pursuit of inexpensive translation solutions, while appealing, can increase the risk of encountering errors, especially in specialized areas. This creates a difficult trade-off: the cheaper the translation, the higher the chance of receiving inaccurate results. This is a common pattern we've been observing across different AI applications.
Adding to the problem, Optical Character Recognition (OCR) technology, often used alongside translation tools, struggles with intricate document layouts and uncommon font styles. This can lead to a flawed understanding of the original text, especially crucial in fields like engineering or legal documentation where precision is paramount.
One of the root causes of these high error rates is a lack of suitable training data for the AI models. Many translation tools primarily rely on general language datasets, leading to insufficient accuracy when confronted with specialized language like that found in manuals or complex technical documents.
Furthermore, fast translation tools often struggle with understanding context, frequently resulting in incorrect translations. Technical manuals frequently rely on surrounding text to convey specific concepts, and machines haven't yet mastered interpreting these complex relationships accurately.
Research suggests languages with numerous homographs, such as Chinese, pose unique challenges for translation systems. The system's difficulty in identifying the correct word meaning can result in critical misunderstandings within technical documentation.
While often promoted for their speed, many tools also experience delays when handling extensive or complex texts. This can lead to rushed translations, compounding accuracy problems as users may choose quick fixes over careful review.
Another area of difficulty lies in cultural nuances, which machines frequently miss, unlike human translators. This lack of cultural sensitivity can lead to translations that not only miscommunicate but also risk offending or confusing the target audience due to inappropriate phrasing or context.
A major obstacle is the lack of effective user feedback mechanisms in many fast translation tools. This means that errors tend to persist rather than improve over time. Without a proper feedback loop, users face repeated mistakes without any opportunity to contribute to improving the translation quality.
Technical manuals often employ very specific jargon that exceeds the typical vocabulary limitations of many translation systems. Relying solely on fast translation tools in these instances can create confusion about critical functionalities, possibly leading to operational safety or compliance issues. This area deserves more attention from researchers and developers working on AI translation technologies.
The Evolution of Translation Satisfaction Why AI Users Still Can't Get What They Need in 2024 - Translation Quality Drops By 28 Percent When Processing Multiple Languages
The ability of AI translation tools to handle multiple languages simultaneously is facing growing scrutiny. Recent research suggests a notable decrease in translation quality, specifically a 28% drop, when AI systems are tasked with translating across various languages. This decline underscores the difficulties in ensuring consistent and accurate translations across diverse languages, especially when users prioritize speed and low cost. While AI translation technologies continue to progress, the limitations of these systems are becoming clearer in scenarios requiring nuanced and precise language. The discrepancy between the expectations users have for AI-powered translation and the current capabilities of the technology is significant. There's a growing realization that the quality of the training data and the methods used to evaluate translation quality are crucial in overcoming these limitations. As global communication becomes more commonplace, the need for accurate and reliable translations is more important than ever. The ongoing challenge is to bridge the existing gap between the advancements in AI translation and the user experience.
When AI translation systems attempt to handle multiple languages simultaneously, their performance takes a significant hit. Research shows that translation quality drops by a notable 28%, a concerning figure that highlights a key limitation in current AI capabilities. This decline in accuracy suggests that these models haven't quite mastered the complexity of juggling different languages at once. It's like asking a person to translate several languages in rapid succession – the cognitive burden can lead to a significant increase in errors.
Furthermore, these mistakes can have a cascading effect, where an initial error in one language impacts the accuracy of other language translations within the same document. Think of it like a game of telephone – the initial message gets distorted, and each subsequent repetition adds to the inaccuracy. It's a challenge that underscores the need for better methods to manage this intricate process.
Interestingly, the magnitude of the decline in translation quality appears to vary across different language pairs. When a system attempts to translate languages with highly contrasting grammatical structures, like Chinese and English, the drop in quality tends to be more pronounced. This suggests that language models might benefit from tailored approaches for specific language combinations.
These inaccuracies are particularly pronounced in technical fields, where the need for precision is paramount. Fast translation tools, often relied upon for quick outputs, can yield error rates as high as 40% when handling specialized technical terms across different languages. It becomes a tradeoff – the desire for cheap and rapid translation often clashes with the requirement for exactness, which is problematic when we consider highly specialized documents like those found in engineering or medical fields.
This issue with multi-language translation can have a knock-on effect on user trust in AI-powered translation services. When users are consistently faced with incorrect translations, they naturally lose faith in the technology. This, in turn, pushes many individuals, especially in critical industries like healthcare, to revert back to human translators, a scenario that underscores the existing gap between what AI can achieve and the requirements of specific use cases.
The root of this challenge lies in the limitations of the training data used to build these AI models. Many of these systems are trained primarily on general language corpora, which often lack a deep understanding of domain-specific languages and the nuanced vocabulary required for various industries. This leads to a lack of contextual awareness and creates situations where a system might struggle to decipher specialized terms within a medical document, for instance.
This problem extends beyond technical terminology; cultural nuances and idiomatic expressions often get lost in the shuffle. An AI may struggle to accurately translate culturally specific phrases when attempting to handle multiple languages, potentially resulting in translations that are not only inaccurate but also culturally insensitive.
Furthermore, the pursuit of fast and cheap translation options often results in a focus on speed at the expense of accuracy, especially when dealing with multi-language scenarios. This "fast and cheap" approach can lead to the development of systems that prioritize quick outputs over meticulous translations, a tradeoff that can be harmful in domains where exact wording is crucial.
The implications of inaccurate translations due to multi-language processing are especially grave in critical industries such as healthcare. A mistranslated medical term can have serious consequences, possibly leading to patient misdiagnosis or adverse treatment decisions. This highlights the urgency in developing AI systems that can handle the challenges posed by multiple languages within such a sensitive domain.
Addressing this challenge necessitates a push towards developing AI translation models that are more specialized. Researchers need to focus on creating systems that not only can parse complex linguistic structures but also integrate a deeper understanding of cultural and domain-specific contexts. This path, however, requires addressing the limitations in the available training datasets and building a strong feedback loop to ensure that these systems are constantly learning and improving their accuracy, especially within fields where precision is not just important, but critical.
The Evolution of Translation Satisfaction Why AI Users Still Can't Get What They Need in 2024 - Small Business Translation Costs Rise Despite AI Tools Market Data Shows
While AI-powered translation tools have surged in popularity, promising swift and affordable solutions, small businesses are facing a counterintuitive trend: rising translation costs. This is largely due to the fact that AI, despite its advancements, often struggles to handle the complexities of specialized language. Technical manuals, medical documents, and other niche areas often contain intricate jargon and formatting that current AI systems have difficulty parsing accurately. In turn, this leads to higher error rates, necessitating human intervention to correct mistakes. The increased need for human review and revision, a process that can be expensive, ultimately leads to inflated costs for businesses.
Although AI can provide quick translations, they often fall short of the accuracy needed in specialized fields where precise language is crucial. Consequently, the demand for human translators remains high. This signifies a significant mismatch between the expectations users have for AI's translation capabilities and its current limitations. Businesses find themselves in a tough spot, caught between the desire to cut costs and the vital need to maintain the reliability and quality of their translations. They are forced to carefully balance the allure of AI's speed with the unavoidable reality that human expertise is often essential for ensuring accurate results, especially in crucial areas like healthcare and engineering.
While AI translation tools have become increasingly prevalent, market data reveals a curious trend: small businesses are facing rising translation costs despite the availability of these supposedly affordable solutions. This seems counterintuitive, but it underscores a fundamental gap between what users expect from AI and the complexities of achieving truly accurate and culturally appropriate translations.
Studies show that while AI tools excel at speed, their accuracy often falls short. Especially in crucial areas like law or medicine, the potential for errors in AI-generated translations can lead to significant liabilities, outweighing the cost savings of relying on these cheaper alternatives. For example, research suggests that fast translation tools used for technical manuals can experience an astonishingly high error rate, reaching as much as 47% in some cases. These mistakes can have serious operational or safety implications, highlighting a major issue for AI in these complex domains.
One of the main problems is that AI models struggle to capture the context and nuances of language. Technical manuals, for example, rely heavily on precise phrasing, and AI systems often miss the mark, leading to misinterpretations and misunderstandings. This becomes further compounded when dealing with languages like Chinese, Japanese, or Korean, where the density of information within a single character makes accurate OCR extremely difficult. Current OCR technology often struggles to achieve accuracy rates above 60% when processing such documents, which subsequently impacts the accuracy of any AI translation that follows.
Additionally, languages with many words that sound alike but have different meanings pose significant challenges. Chinese is a prominent example, where AI systems frequently misidentify the correct meaning of a word within a technical document, leading to severe misunderstandings. The problem only gets worse when translating across multiple languages, where accuracy appears to drop by about 28%, possibly because AI models haven't yet mastered managing the interplay between different linguistic structures simultaneously.
Furthermore, the issue of cultural sensitivity in translation remains a critical challenge. Unlike human translators, AI frequently overlooks the nuanced cultural and idiomatic aspects of languages, leading to translations that can be inaccurate or even offensive. The persistence of these translation mistakes, especially in high-stakes situations like medical applications, can cause users to lose trust in AI, prompting them to switch back to traditional, human-driven translation methods. The lack of user feedback loops in many of these systems means they can't easily learn from their mistakes, which further inhibits improvement and leaves room for errors to persist over time.
In conclusion, while AI translation tools are certainly a rapidly developing field, they still face significant obstacles when it comes to delivering the level of quality and accuracy required in many industries. The pursuit of inexpensive solutions, though understandable, often comes at the expense of accuracy, a trade-off that can have serious implications depending on the domain and the consequences of error. It's a continuing research area with a clear gap between current AI capabilities and the demands of various specialized domains.
The Evolution of Translation Satisfaction Why AI Users Still Can't Get What They Need in 2024 - Human Translators Still Process Legal Documents 3x Faster Than AI Tools
Even in 2024, human translators are still significantly faster at processing legal documents than AI tools, managing the task at a rate three times quicker. This clear advantage emphasizes the limitations of current AI in truly grasping the complexities and nuances of legal language. While AI can certainly process large quantities of text with speed and cost savings, the inherent difficulties in understanding intricate legal concepts often results in AI translations lagging behind in accuracy when compared to those done by humans. The current legal translation landscape, marked by a scarcity of qualified legal translators, forces the use of both human and AI approaches, highlighting the ongoing difficulties in this field. Despite the attractive lure of cheap, fast AI translation options, the necessity of human expertise to ensure the accuracy of crucial legal translations remains evident. It underscores that even with AI, human intervention and oversight is often required in fields where precision is absolutely vital.
Human translators, surprisingly, can process legal documents up to three times faster than currently available AI tools, despite the marketing push for AI speed. This finding prompts questions about the actual efficiency of machine translation, specifically in specialized areas.
While AI translation tools promise rapid turnaround times, they face challenges achieving high accuracy in legal settings. Error rates as high as 47% have been observed, which poses significant reliability concerns. Industries relying on absolute precision, like law, could face increased operational risks if solely using automated tools without thorough human review.
Legal language is filled with subtle nuances and complex terminology that AI systems often struggle to correctly decipher. Human translators, having a deeper understanding of legal contexts and language, can capture these complexities more effectively, ensuring that the intended legal meaning remains unchanged—crucial for legal contexts.
Furthermore, AI translation tools often fail to appreciate the influence of cultural context and idiomatic expressions in legal documents. While human translators are adept at capturing these subtleties, maintaining both the original intent and the accurate meaning, AI often overlooks them, leading to misunderstandings.
OCR, which extracts text from scanned documents, continues to be a limiting factor. Its inaccuracies can compound translation errors, particularly in the legal domain, necessitating more extensive human oversight than initially anticipated.
Many AI translation models are trained using broad language datasets that don't fully represent the unique vocabulary found in legal fields. This lack of specialized training hinders their ability to perform accurately with legal documents.
Legal translations demand a sharp understanding of context. Research has shown that AI struggles to grasp the complex connections between words and phrases, connections often established by intricate legal frameworks that require a seasoned translator's expertise.
The stakes are high when it comes to translating legal documents. Mistakes can have far-reaching effects on contractual obligations or legal proceedings. The risks associated with AI for such crucial tasks are driving many legal professionals to rely on human translators.
The persistent errors in AI-generated legal translations are eroding user confidence, especially in legal fields where precision is non-negotiable. This has led many to avoid solely relying on AI, preferring the consistency and expertise of human translators.
Although AI promises cost-cutting solutions, the necessity of human review to fix its errors frequently leads to higher total translation costs. Businesses trying to streamline expenses may end up paying more in the long run to account for AI inaccuracies. This dynamic highlights the need for careful cost-benefit assessments when considering AI for translation, especially in demanding fields.
More Posts from aitranslations.io: