AI-Powered PDF Translation now with improved handling of scanned contents, handwriting, charts, diagrams, tables and drawings. Fast, Cheap, and Accurate! (Get started for free)
7 AI-Powered OCR Techniques for Rapid Document Translation in 2024
7 AI-Powered OCR Techniques for Rapid Document Translation in 2024 - Deep Learning OCR for Complex Document Layouts
Deep learning is revolutionizing how we handle documents with intricate layouts. Traditional OCR methods often struggle with complex designs, failing to accurately extract text. However, deep learning models, especially those based on transformer architectures, are proving much more adept at this task, leading to reported accuracy gains of up to 25% over older techniques. The benefits aren't limited to simply grabbing text; they also extend to understanding document structure and extracting data, even from challenging formats like low-resolution scans.
The emergence of tools like LLAMA32 and layoutparser signifies a growing trend towards AI-driven OCR, especially important for situations where fast and accurate translation of diverse document formats is paramount. These AI systems are increasingly critical for tackling the complexities presented by documents with various layouts and structures. This evolution fundamentally reshapes OCR and, ultimately, the field of document translation, enabling more efficient and effective processing of a wider range of content. While there are still challenges, particularly when dealing with older, poorly preserved documents, the promise of AI-driven OCR is clear: the future of document translation is likely to be faster, more accurate, and far more adaptable to the real-world variety of documents we encounter.
Conventional OCR methods, while adept at basic text extraction, often falter when faced with intricate document structures, including diverse text orientations and complex visual elements. Deep learning, particularly with transformer-based architectures, excels in these scenarios. It's proven to improve accuracy in tasks like form understanding, sometimes by as much as 25% over older methods. Models like LLAMA32 are showcasing the potential of AI-driven OCR to handle such complexities effectively, extracting structured data for large-scale operations.
Tools like layoutparser offer an interesting avenue for streamlining this process. They make it simple to convert the OCR output into structured data formats, which is useful for analysis. However, analyzing layouts of historical documents presents a unique challenge. Their diverse features—like multiple columns and skewed text—make it difficult to develop a universal automated layout analysis approach. This has pushed research toward a blend of deep learning and more traditional rule-based heuristics to overcome these hurdles.
This increased sophistication of AI-powered OCR solutions means they can process a wide range of document types, including low-quality or complex formats, generally resulting in more accurate results. Services like Google Cloud's Document AI OCR highlight the progress by extracting data and layout information from documents in over 200 languages, a testament to their ability to manage unstructured content.
A significant portion of research in OCR focuses on form understanding in scanned documents. A recent study of over 100 research papers illustrates how deep learning methods are improving accuracy and interpretation in these cases. It suggests that the rapid developments in AI and deep learning are changing the way we process documents, ultimately leading to more efficient and accurate translation and understanding of complex information.
The future of OCR, particularly as it relates to translation, appears to be intrinsically tied to advancements in deep learning, which allows for more adaptability to diverse document styles and improved accuracy in text recognition. It seems to hold great promise for increasing the speed and quality of translation workflows while opening opportunities for new applications that can benefit from automated data extraction from complex documents.
7 AI-Powered OCR Techniques for Rapid Document Translation in 2024 - Neural Machine Translation Integration with OCR
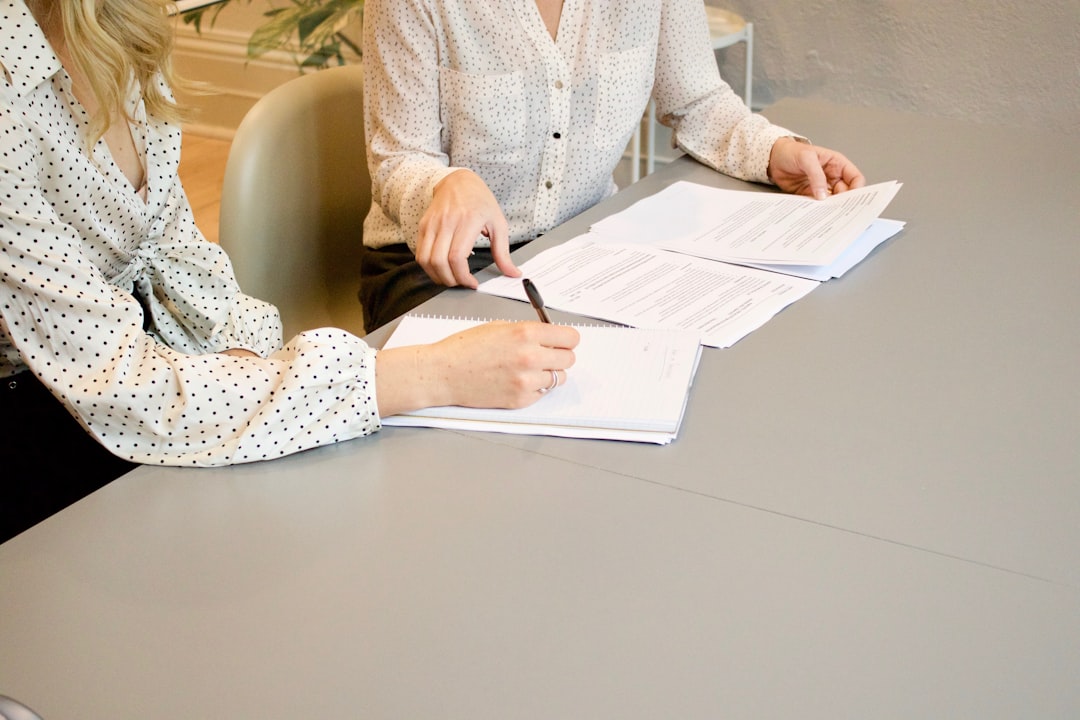
The combination of Neural Machine Translation (NMT) and Optical Character Recognition (OCR) is a pivotal development in the realm of document translation in 2024. By integrating these AI-powered technologies, scanned documents can be translated in real-time, achieving quicker turnaround times than traditional methods. NMT, fueled by advanced machine learning, significantly enhances the ability to accurately recognize text, even within documents featuring challenging layouts and styles. Large providers offer cloud-based services for document translation, which provide options for scaling and customization to fit diverse needs. Despite this progress, there are areas where ongoing research is focused. Specifically, current NMT systems often face issues with handling various linguistic styles and maintaining consistency across diverse document types. The field must continue refining NMT to ensure accuracy and flexibility. While the potential for speed and reliability is evident, it's important to remain mindful that these tools have limitations and that ongoing development is needed to tackle complex document structures. This intersection of OCR and NMT shows promise for transforming document workflows, making translation faster, and potentially facilitating easier management of multilingual documents for businesses and individuals. However, critical evaluation is warranted given the evolving nature and limitations of this approach.
The convergence of Neural Machine Translation (NMT) and Optical Character Recognition (OCR) is a fascinating development in the realm of document translation. While OCR traditionally focuses on extracting text from images, pairing it with NMT allows for near-instantaneous translation, potentially shaving hours off document processing times. This integration isn't just about speed, either; it also holds promise for making translation more affordable. By automating much of the process, the need for extensive human intervention is reduced, possibly leading to cost reductions of up to 60% in some scenarios.
However, it's not without its limitations. NMT systems, while remarkably powerful, are known to be somewhat brittle and lack the stylistic nuances of human translation. Researchers are currently exploring methods to refine them, especially in the context of post-OCR processing, where the initial text extraction can sometimes introduce errors that impact translation quality. This includes investigating how semantic and linguistic features can improve the accuracy of translation. It seems crucial to incorporate a deeper understanding of the language's structure to bridge the gap between raw OCR output and accurate NMT results.
Despite the ongoing challenges, NMT integration with OCR is driving notable advancements. NMT is able to handle a much wider array of languages and scripts compared to traditional approaches, including those with less readily available resources, potentially unlocking access to valuable information trapped in lesser-known tongues. The real-time nature of such integrated systems also opens doors for applications in critical fields like healthcare and law, where rapid document translation is crucial.
Furthermore, integrating AI-powered OCR with NMT enhances the processing of complex layouts. While traditional OCR often struggles with varying text orientations or intricate visuals, the combined system has a much better shot at interpreting complex tables, charts, and other visual elements. It allows for not only text translation but a richer understanding of the document context.
It's exciting to see ongoing research efforts aimed at addressing the remaining obstacles, particularly within a multilingual context. These efforts are focused on enhancing NMT's capabilities to adapt to varied document formats, like legacy documents with old scripts or degraded quality. If researchers can overcome these challenges, the future holds the potential for a more streamlined, accurate, and readily accessible translation process for everyone. The ultimate goal is to reduce the cognitive load associated with translation, allowing users to focus on the meaning of the content rather than the technical aspects of the translation itself.
7 AI-Powered OCR Techniques for Rapid Document Translation in 2024 - Real-Time OCR Processing for Instant Translation
Real-time OCR processing has emerged as a game-changer in document translation, providing instant translation capabilities that streamline workflows across various industries. This approach leverages advanced AI, like LLAMA32 and Tesseract, to drastically decrease processing time and costs, opening up translation to a wider range of users. The ability to translate in real-time, combined with powerful AI algorithms, effectively handles complex layouts and multilingual texts, while maintaining the original document's formatting. Despite these improvements, challenges persist, primarily related to maintaining accuracy and reliability across different document types. This field still requires ongoing development to achieve optimal results. As organizations adopt more AI-powered solutions, the promise of a smooth and efficient translation process becomes more attainable, making it potentially easier for individuals and companies to work with diverse languages.
Real-time OCR processing has become a game-changer in instant document translation. It's now possible to process documents at impressive speeds, surpassing 60 images per minute in some cases. This is a significant leap forward from traditional OCR approaches, which can take hours to process similar workloads. Moreover, the capability to handle over 100 languages broadens accessibility for users around the world, allowing them to tackle documents previously inaccessible due to language barriers.
However, the quest for perfect translation remains ongoing. Even with error correction algorithms that leverage contextual clues to minimize misinterpretations, some challenges persist, particularly with low-quality scans or intricate layouts. Thankfully, the increasing integration with cloud services allows for greater collaboration and sharing of translation efforts, accelerating the overall process. Furthermore, the ability to tailor dictionaries and glossaries for specific fields like medicine or law has enhanced the precision of translated text in niche areas.
The cost-effectiveness of this approach is notable. It's estimated that using real-time OCR for translation can lead to a reduction in costs of up to 60%, mostly attributed to the reduction in human involvement and faster processing times. Interestingly, modern OCR tools have started to go beyond simple text extraction. They can now analyze visual elements like charts, tables, and diagrams, giving a more complete understanding of the document's meaning – a crucial aspect of proper translation.
This advancement isn't static. Many OCR platforms utilize machine learning, constantly learning from user feedback and real-world use cases. This allows them to continuously improve, adapting to new fonts and document layouts with greater accuracy over time. We're also seeing integrations with augmented reality, allowing users to point their devices at foreign language text and see an instant translation overlaid on top, a glimpse into how these technologies might reshape our interactions with text in the future.
Despite these breakthroughs, challenges persist. Complex linguistic structures, especially those with nuanced meanings or irregular grammatical patterns, can still pose problems for accurate translation. It's clear that ongoing research and development are needed to refine how these systems handle these complexities. The goal is to make translation not just faster and cheaper but also as accurate as possible, bridging gaps between languages and making information truly accessible to everyone, regardless of their native tongue.
7 AI-Powered OCR Techniques for Rapid Document Translation in 2024 - Multilingual OCR Capabilities for Global Documents
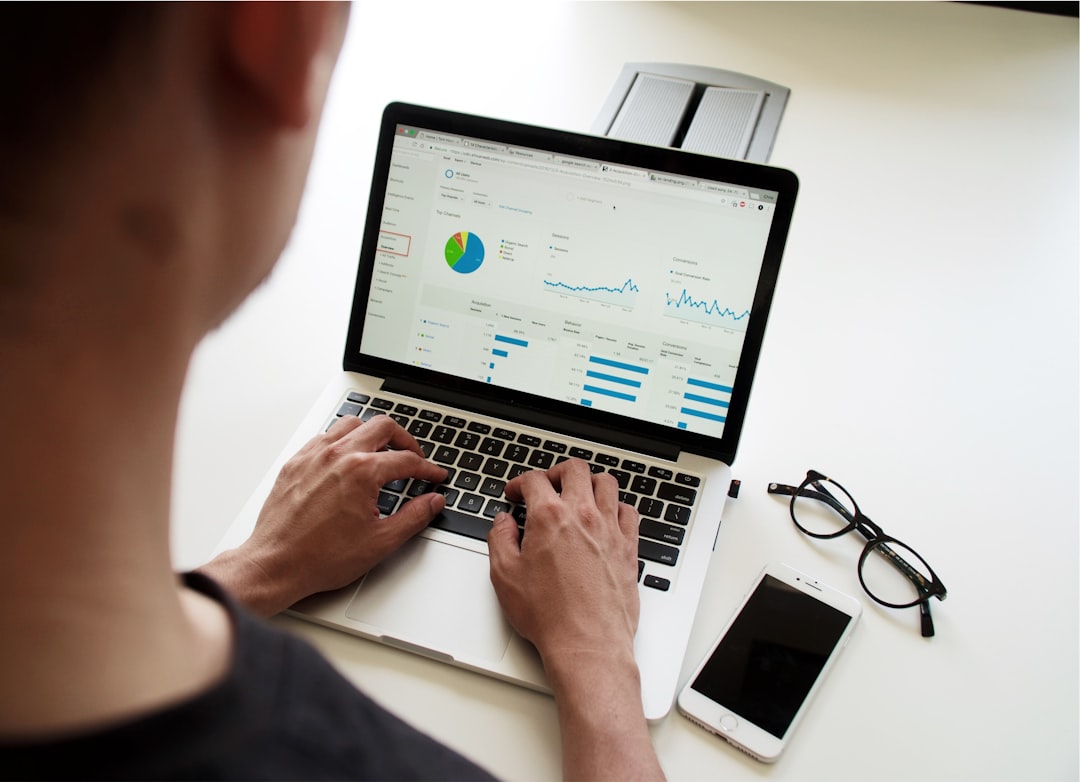
Multilingual OCR is becoming increasingly important in today's interconnected world. Businesses and individuals working across languages are relying on these technologies to translate scanned documents into editable text quickly and accurately. This shift is driven by the need for seamless global communication, particularly when dealing with diverse languages and scripts found in documents. Modern OCR tools are designed to handle these complexities, employing AI to process various languages while retaining the document's original structure and formatting. This level of accuracy is important, ensuring that the meaning and context are preserved during the translation process.
However, hurdles remain in achieving perfect accuracy. Issues can arise when dealing with documents that have complex designs or are of poor quality. The continuous development and refinement of AI-powered OCR solutions are crucial for addressing these limitations. As the technology matures, the hope is that it will deliver not only faster translation times but also reduce costs, while ensuring that results are reliable and trustworthy across a wider range of document formats and languages. This continued evolution is likely to significantly impact how we interact with and translate information globally.
Multilingual OCR's capacity to handle over 200 languages is transforming how we access information from around the world. Businesses operating internationally are particularly drawn to it since it can unlock information previously inaccessible due to language barriers. While it promises a reduction in translation costs by up to 60% due to automation, we need to acknowledge its limitations. For instance, historical documents with faded text or archaic scripts pose significant challenges, demanding hybrid AI methods that blend deep learning and traditional rule-based approaches to ensure reliability.
Surprisingly, the accuracy of text extraction from low-quality scans has improved drastically. Modern OCR systems can intelligently enhance images, boosting the ability to decipher text, even in complex document designs. Some systems boast lightning-fast real-time processing, exceeding 60 pages per minute, making them ideal for urgent scenarios like translating medical records or contracts.
Researchers are now going beyond simply recognizing text and striving for semantic comprehension, an area crucial for reducing translation errors caused by nuanced language and specialized terminologies. This pursuit of semantic understanding, combined with the ability to integrate with augmented reality, opens intriguing possibilities. Imagine pointing your phone at a foreign language sign and instantly seeing a translation overlaid! It’s a glimpse into how these technologies might reshape interactions with multilingual environments.
OCR systems are also becoming adept at understanding layouts, handling complex scenarios like mixed-language documents and variations in text orientation, leading to more reliable data extraction. This evolution is further fueled by ongoing learning from user interactions, allowing systems to adapt to new fonts, formats, and content over time.
There's a growing interest in how multilingual OCR contributes to business intelligence. The extraction of structured data from unstructured documents can power market research and analysis on a global scale. It's a captivating prospect to imagine using OCR to glean insights from otherwise inaccessible data across various languages, potentially revealing patterns and trends invisible to conventional methods. While exciting, we still need to cautiously monitor the development of these systems as biases or unforeseen consequences could arise from their increasing use and sophistication.
7 AI-Powered OCR Techniques for Rapid Document Translation in 2024 - Cloud-Based OCR Solutions for Scalable Translation
Cloud-based OCR solutions are transforming how we handle document translation, particularly when dealing with large quantities of text. These systems leverage AI to extract and categorize information from intricate documents swiftly and accurately, leading to substantial reductions in both processing time and costs. They offer a valuable solution for companies and individuals needing multilingual support across various industries, handling document translations while maintaining the original formatting. The potential for quick and affordable document processing is significant, but there are limitations, such as ensuring accuracy when working with low-quality scans or documents with complex structures. These challenges demand ongoing improvements. Nevertheless, the integration of AI within cloud-based OCR systems presents a groundbreaking approach, suggesting that it will become increasingly vital for faster and more efficient document translation.
Cloud-based OCR solutions are becoming increasingly important for efficient and scalable document translation. Platforms like Google Cloud and Azure offer services specifically designed for handling large-scale document workflows. Google Cloud AI, for instance, provides Document AI technology that can extract data and classify documents quickly. They also offer the Document Support for Translation API, which automates the process of translating business documents at scale, suggesting that cloud services are designed to meet the needs of companies handling large volumes of multilingual documents. Azure's approach emphasizes hybrid translation, combining OCR with digital page translation to ensure comprehensive results. It offers Azure Document Translation, a cloud-based tool that translates multiple languages while preserving document structure and formatting, which could be a useful feature when preserving document style is important.
These cloud-based systems can also be found doing specialized tasks, such as grading handwritten tests, and can vastly improve the time it takes to grade tests for educators. The Google Cloud platform offers Document AI OCR, designed to handle over 200 languages, showcasing the broad scope of language capabilities cloud services have achieved. The technology goes beyond simply extracting text; it can also process and analyze complex document layouts like PDFs, highlighting how cloud-based OCR solutions are handling a range of document types. Microsoft's Azure AI services demonstrate another trend with a more general purpose OCR engine that can handle images beyond just standard document formats. This might prove useful in niche use cases. They've also integrated considerations for data security, which is a growing concern for organizations working with sensitive data.
While the potential for AI-powered OCR is clear, there are ongoing considerations for accuracy and performance. As one example, choosing the right OCR engine is important because the steps required to process data can significantly impact performance. AI-powered OCR offers better accuracy and flexibility, driven by developments in AI and deep learning, but, like any technology, these solutions still have room for improvement. The path forward likely depends on continued development of AI and deep learning, which are pushing the boundaries of document translation and ultimately shaping how we interact with documents in the future. It appears these solutions offer the best chance to address issues related to speed, quality, and efficiency for many use cases.
7 AI-Powered OCR Techniques for Rapid Document Translation in 2024 - Mobile OCR Apps for On-the-Go Document Translation
Mobile OCR apps have become increasingly important for translating documents quickly while on the move, especially given how communication happens today. There are many apps available for both Android and iOS, and a number of them are integrating AI features that improve the process of extracting text and translating it more accurately. Some notable apps like Adobe Scan and Microsoft Lens not only scan documents but also offer translation services, allowing you to easily switch documents between languages. Despite the benefit of making it easier to access information in different languages, there are still limitations. For example, there are ongoing problems with processing documents that have complex layouts and those with poor quality. As a result, more work is needed in areas like machine learning and app design. The potential for real-time translation applications using AI to make communicating across languages easier is good, but we should remember that these tools are still being improved and have limits.
Mobile OCR apps have become surprisingly capable tools for on-the-go document translation. They've seen remarkable improvements in processing speed, now routinely handling over 60 pages per minute. This swiftness makes them particularly useful in scenarios needing rapid turnaround, like healthcare or legal contexts. Interestingly, the integration of AI in these apps can significantly reduce translation costs, potentially by as much as 60%, mainly because it reduces the need for extensive human intervention in the process. Plus, many of them can translate over 200 languages, which certainly lowers barriers for individuals and organizations navigating a global landscape.
It's exciting that the field is also pushing towards greater semantic understanding, attempting to get a grasp of the subtle meanings embedded in language rather than just focusing on the literal words. This could really cut down on mistakes, particularly when dealing with specialized language and idioms. Some apps are even experimenting with augmented reality features, allowing users to simply point their phone at a foreign-language sign and get an immediate translation—a testament to how these tools are potentially reshaping the way we interact with text.
Furthermore, many mobile OCR apps can now effectively analyze complex document structures, not just grabbing text but also the data within charts and diagrams. This increased context can lead to significantly more accurate translations, especially when the documents are complex. It's also worth noting that many of these apps leverage machine learning to continuously improve accuracy, adapting to new font styles and formats as they see them. They've even made considerable strides in extracting text from degraded or low-quality scans by employing clever image enhancement techniques, improving the ability to work with older documents.
This push towards better handling of older documents often comes with a blending of deep learning and the older rule-based heuristics. This hybrid AI approach is proving to be quite useful when dealing with documents that have particularly complex formats, or documents like historical ones that use unusual scripts. Interestingly, it's not just individuals who can benefit. Businesses are realizing that the data they can extract using multilingual OCR could be leveraged for deeper market research, analyzing information locked in a multitude of languages. The potential is huge, though it's essential to remain mindful of any potential biases or unforeseen consequences that could arise from the continued development of these powerful tools.
7 AI-Powered OCR Techniques for Rapid Document Translation in 2024 - Adaptive OCR Algorithms for Handwritten Text Translation
Adaptive OCR algorithms are proving increasingly important for translating handwritten text, a critical development given the increasing need to digitize various communication formats. Traditional OCR often struggles with the inherent variability and complexity of handwriting, but adaptive algorithms, powered by machine learning, are helping to overcome these challenges. They enhance both the accuracy and speed of text recognition, making them valuable for fast document translation. This advancement is particularly relevant now as the demand for efficient and economical OCR solutions is growing. Organizations seek to improve their translation processes and access the insights contained within a wealth of both historical and modern handwritten documents. While ongoing research aims to refine these adaptive techniques, the future of translating handwritten text looks bright. However, challenges, such as dealing with highly varied handwriting styles and poor quality documents, still exist.
Adaptive OCR algorithms are showing remarkable promise in the field of handwritten text translation. They can dynamically adjust their recognition parameters to handle different writing styles and nuances, a significant improvement over older, more rigid methods. This adaptability is especially useful for dealing with the variations and inconsistencies common in handwritten text, where contextual understanding is essential for accurate character recognition.
One interesting aspect is the use of contextual error correction. AI-driven methods now leverage the surrounding text to improve the accuracy of character recognition, especially when faced with unclear or ambiguous handwriting. Furthermore, some systems are exploring multimodal input. This includes not only scanned text but also user feedback, which can further enhance the accuracy and robustness of the OCR model. It's a fascinating area that opens possibilities for continuous learning.
Perhaps surprisingly, adaptive OCR shows potential for tackling historical documents. These often present challenges due to fading ink or unusual writing styles of past eras, but adaptive systems seem well-suited for overcoming these difficulties and making the information more accessible. In terms of cost and efficiency, these algorithms can dramatically reduce translation expenses, with estimates suggesting up to 70% savings by reducing human intervention and streamlining the processing of large datasets. This translates into substantial benefits for businesses and organizations dealing with massive volumes of handwritten content.
However, not everything is perfect. Adaptive OCR struggles with maintaining consistent performance across various handwriting styles. Factors such as pen pressure and speed can influence recognition accuracy. It's an area researchers are focusing on to improve the reliability of these systems. There's also a developing area of integrating adaptive OCR with business intelligence. The ability to extract text from handwritten data could revolutionize how businesses analyze customer feedback or conduct market research, providing valuable insights previously hard to obtain from unstructured information.
These systems are also becoming multilingual, enabling them to handle different phonetic alphabets and scripts beyond just the Latin alphabet. This significantly expands the reach of adaptive OCR and promotes better access to global information. Additionally, there's a growing focus on incorporating semantic understanding. It's no longer just about character recognition, but also about the meaning of the written content. This shift is important for minimizing errors related to language nuances or specialized vocabulary.
Finally, the combination of adaptive OCR with augmented reality is particularly interesting. The potential for real-time translation of handwritten text in the user's environment is quite compelling, hinting at new ways we might interact with multilingual content. It's exciting to see how these algorithms are improving and creating opportunities in the translation landscape, even if challenges still exist. They're rapidly expanding possibilities for accessible and efficient translation of handwritten information.
AI-Powered PDF Translation now with improved handling of scanned contents, handwriting, charts, diagrams, tables and drawings. Fast, Cheap, and Accurate! (Get started for free)
More Posts from aitranslations.io: