7 Cost-Effective AI Translation Tools with OCR Capabilities in 2024 A Technical Analysis
7 Cost-Effective AI Translation Tools with OCR Capabilities in 2024 A Technical Analysis - DeepL Photo Scans Images at 10 Languages Under $6 Monthly
DeepL has brought out the capability to translate text directly from images. This feature, sometimes described as photo scanning, is said to handle a core selection of 10 languages and is reportedly offered at a monthly cost under $6. The way it works involves users uploading an image file, after which the system attempts to identify the language within the picture automatically before generating a translation. While this particular low-cost option might be tied to those 10 languages, the image translation feature in its entirety reportedly covers a wider range of 31 languages. DeepL is often noted for delivering generally accurate translations in many common scenarios. However, as with other automated translation tools, its effectiveness can sometimes be limited when dealing with idiomatic phrases or highly casual language, potentially performing less consistently in these areas compared to services that might support a significantly larger number of languages overall. There are also numerous other mobile applications available that provide similar image-based translation through OCR, sometimes at comparable prices.
Examining DeepL's image translation offering, the system reportedly handles various file formats like JPG, PNG, and PDF, providing a degree of flexibility for input. The advertised price point, noted as being under six dollars monthly, positions it as a remarkably inexpensive option compared to many enterprise-level solutions, potentially democratizing access to AI-driven OCR translation, though the feature set available at that specific tier would warrant closer technical scrutiny. Its architecture is said to employ advanced neural networks aiming for strong contextual accuracy, often cited as a strength, though as with any statistical model, performance can vary unexpectedly with unusual sentence structures or domain-specific jargon. The claim of continuous refinement via machine learning based on user interaction suggests an adaptive model, theoretically improving recognition and translation over time, assuming sufficient diverse data flow and effective update mechanisms. Users are told to expect relatively rapid results, with processing and translation aiming to complete within seconds, which is practical for quick tasks but could be sensitive to image complexity, resolution, or current system load. The service apparently covers ten languages, a relatively focused set compared to some hyper-scale models, but potentially valuable if it includes niche languages often overlooked by broader platforms. Integration capabilities are mentioned, suggesting potential for incorporating the tool into workflows, which is key for utility beyond standalone use. The system reportedly leverages user feedback loops to ostensibly accelerate error detection and model correction. Furthermore, the OCR component includes features for separating text from potentially complex or cluttered visual backgrounds, a non-trivial task in image processing. Finally, data handling considerations are addressed with a stated policy of not retaining uploaded images indefinitely, a necessary factor for privacy and security assurances in a service processing user-provided content.
7 Cost-Effective AI Translation Tools with OCR Capabilities in 2024 A Technical Analysis - Mathpix OCR Extracts Formulas from PDFs Starting at $99
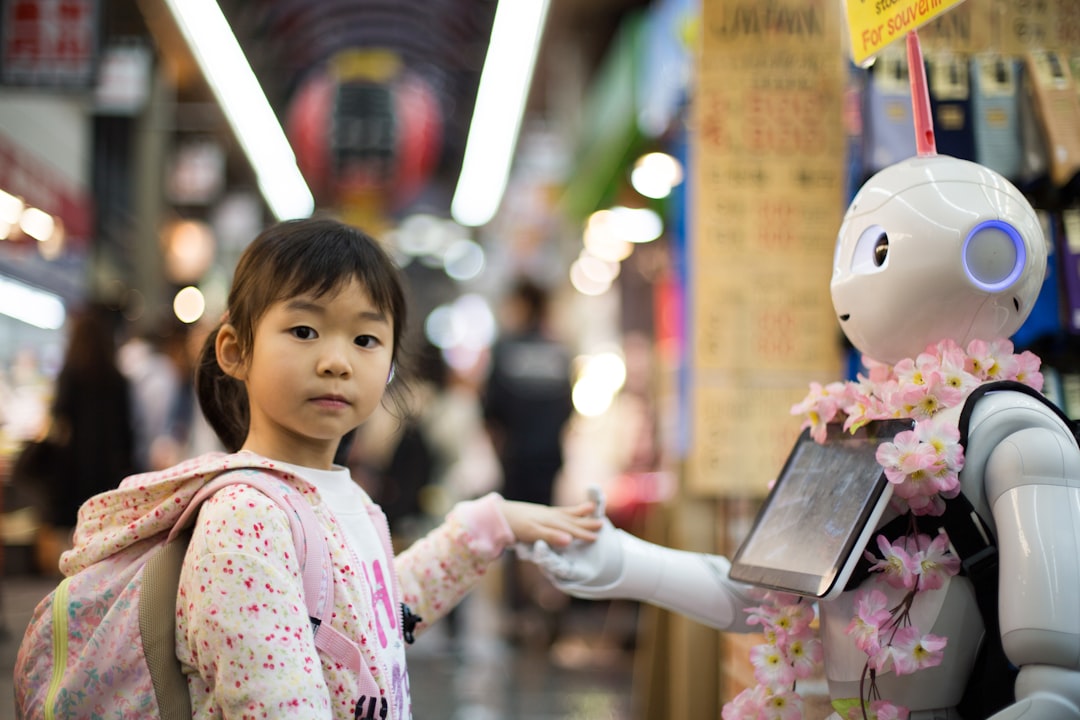
Mathpix OCR offers a distinct approach by focusing its optical character recognition capabilities on mathematical and scientific content within PDFs. This tool is designed to handle complex expressions like equations, chemical diagrams, and tabular data, converting them into structured formats suitable for technical work, such as LaTeX or Markdown. It positions itself as particularly valuable for individuals in STEM fields, including researchers and educators, claiming high accuracy for both typeset and handwritten technical notation. While featured alongside tools offering general text translation and OCR, Mathpix's function is purely extraction and formatting for scientific data. Access begins at a $99 price point for certain service levels, though lower-cost options are available through limited free usage tiers or specific API access paths. This specialization addresses a niche but critical need in academic and technical workflows, contrasting with broader OCR tools that may struggle with the intricacies of scientific notation, though the landscape of high-accuracy technical OCR is continuously developing across the field.
Mathpix offers a tool focused squarely on extracting mathematical expressions from PDF documents and images. Its pricing starts around $99, which, from a purely cost perspective, sits somewhere between the ultra-low-cost monthly subscriptions and the significantly more expensive one-time or annual licenses for general-purpose document processing suites that might cost hundreds of dollars. This initial price point appears aimed at individuals or small groups requiring its specific function. There are different access tiers, including limited free options, suggesting a model that scales use or features based on payment, a common software approach. Educational users reportedly get slightly more generous free usage allowances, acknowledging academic needs.
Delving into its reported technical capabilities as of early 2025, the core function is high-accuracy recognition of complex mathematical notation. This is a non-trivial task, as equations involve intricate symbols, superscripts, subscripts, and structures that standard text-based OCR often mishandles. Reports suggest it performs well here, vital for fields where notation fidelity isn't just helpful but essential for meaning. It processes standard document formats like PDFs and common image types. The promise is rapid conversion, aiming to turn visual math into editable digital formats reasonably quickly. For users dealing with significant amounts of technical documentation, this speed could be a practical advantage over manual input, assuming consistent accuracy.
From an engineering viewpoint, its specialization is notable. Rather than attempting broad OCR across countless languages and document types, it targets a specific domain – scientific and technical content heavy on formulas. This focus potentially allows for deeper optimization of its recognition models for that particular structure and vocabulary (the language of mathematics itself). It's said to support output formats like LaTeX and Markdown, which are standard in technical writing and publishing workflows, indicating it's designed with these specific user pipelines in mind. An interesting technical aspect mentioned is a mechanism for user feedback on recognition errors, which, if effectively implemented, could contribute to model refinement over time, addressing edge cases or new notations that might emerge.
However, this specialization also brings constraints. While its math recognition is key, its utility for extracting or translating general text, especially in multiple languages, might be limited compared to broader OCR or translation tools. Its strength appears to be tied closely to English-language mathematical notation, potentially posing challenges when processing scientific texts where explanations or surrounding text are in other languages, or where non-standard notation is used. Integration points with some common note-taking or document tools are mentioned, which helps usability within academic or research workflows. Handling of user data, particularly uploaded documents, is also a factor; systems processing potentially sensitive research content need clear policies on data retention and privacy, a necessary technical and trust consideration. Overall, it appears to be a purpose-built engine for a specific, challenging OCR problem, positioned at a price point intended to be accessible to its target scientific/technical user base, with the caveat that its capabilities are largely confined to that niche.
7 Cost-Effective AI Translation Tools with OCR Capabilities in 2024 A Technical Analysis - OCR Space API Handles 46 Languages with Free Tier of 25k Characters
As of May 2025, the OCR Space API offers optical character recognition support spanning 46 languages. Positioned within the sphere of tools considered for their cost-effectiveness, it notably provides a free usage tier. This free level permits processing up to 25,000 characters per month, constrained by a daily limit of 500 requests from a single IP address. This accessibility facilitates basic conversion needs for images and multi-page PDF documents into editable text formats. A significant update in January 2025 expanded its linguistic reach, incorporating languages such as Korean, Japanese, Russian, Ukrainian, Thai, and Vietnamese. The service is equipped with features like automatic language detection and the capability to handle vertical text recognition. While useful for foundational text extraction often preceding translation workflows, users considering this tool should carefully evaluate the limitations of the free tier's character and request volume, which might prove insufficient for more demanding or frequent use cases without transitioning to a paid plan.
Examining one particular offering, the OCR Space API details indicate support for optical character recognition across 46 languages. This extensive linguistic coverage suggests a capability to process text from a significant variety of global documents.
The service offers a limited initial access tier, capping usage at 25,000 characters per month without direct charge. This allowance permits some level of functional evaluation or minimal use for individuals and smaller operational requirements before potential costs are incurred. A daily request restriction of 500 attempts per IP address is also specified for this free tier.
Reports suggest the service aims for prompt processing of uploaded images and multi-page PDF files, intended to convert them into structured text data, typically returned in JSON format. This speed characteristic could be a factor for scenarios demanding quick textual retrieval from visual sources.
Updates to the system, such as one noted in January 2025, have expanded the language set further, adding specific languages like Korean, Japanese, Russian, Ukrainian, Thai, and Vietnamese, broadening its operational scope for certain regions.
Functionality for automatically attempting to identify the language present in the image is mentioned, alongside the capability to handle text oriented vertically within documents.
Handling documents with visual complexities or inconsistent image quality during OCR is a reported aspect of its operation, which can influence the overall accuracy of the extracted text.
Provision of standard programmatic interfaces, specifically SOAP and REST web interfaces according to documentation, facilitates potential incorporation of the service into other software applications or workflows.
The core technical objective appears to be the transformation of scanned documents, images, or PDFs into a format where the text content becomes electronically accessible and searchable.
Considerations around handling user-provided data, including document content, are acknowledged factors that developers assessing integration would need to review.
The availability of a tiered access structure, including a no-cost initial usage allocation and presumably paid options for higher limits or volumes, presents options for differing scales of technical need. Additionally, an online web interface for basic usage is noted as not requiring registration.
7 Cost-Effective AI Translation Tools with OCR Capabilities in 2024 A Technical Analysis - Easyscreen Batch Processes 500 Images Monthly at $99
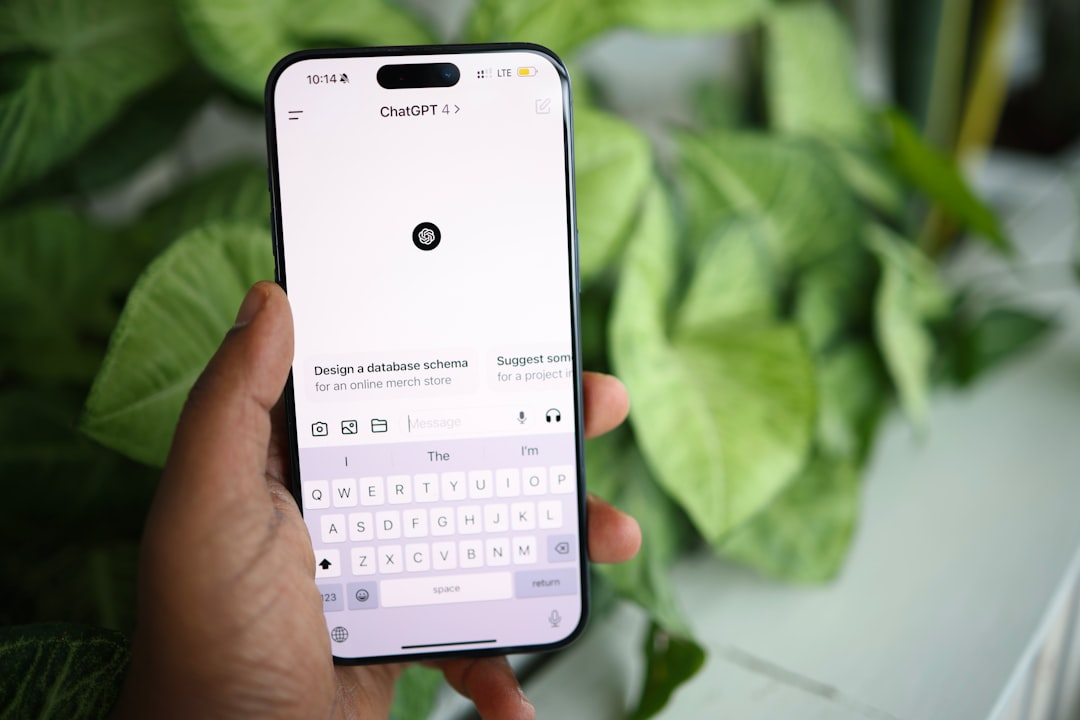
Regarding cost-effective AI translation tools leveraging OCR, Easyscreen presents a specific service model centered around batch processing. As of May 2025, this offering allows users to process a defined volume of image files, stated as up to 500 images per month, for a set cost of $99. This structure is geared towards users who have a consistent, albeit capped, requirement for converting images into editable text via optical character recognition. The underlying technology reportedly employs advanced OCR, claiming an accuracy exceeding 98% in text extraction, which is a key technical metric. Notably, the service is said to support recognizing and potentially translating text across a broad spectrum of over 100 languages, offering significant linguistic flexibility for multilingual image sources. While the fixed price provides budget predictability, the 500-image limit per month requires careful consideration; users with volumes fluctuating significantly above or below this threshold might find other pricing models, such as per-image or subscription tiers based on different usage levels, more aligned with their needs. The integration of translation directly with the OCR capability means a streamlined workflow for generating translated text from visual inputs, operating entirely online, which simplifies access but relies on a stable internet connection for consistent use. Ultimately, its practicality hinges on whether the specific combination of batch volume, accuracy, and language support offered at the $99 price point matches a user's particular demands.
One service positioning itself for handling image-based text processing offers a capacity threshold of 500 images per month at a fixed cost reported as $99. From an engineering standpoint, this translates to a unit cost of approximately twenty cents per image processed within that volume bracket. Evaluating the genuine "cost-effectiveness" requires considering the typical complexity of these images and whether this structure scales predictably beyond the stated limit, alongside comparisons to pay-per-use models or significantly larger enterprise packages.
The core technical component relies on optical character recognition, with claims of achieving high accuracy – specifically noted from some sources as exceeding 98% for text extraction. The underlying OCR engines reportedly leverage Google's technology or a proprietary alternative. A researcher might question how this accuracy figure is derived and maintained across varied fonts, image qualities, and layouts, acknowledging that 98% at the character level does not guarantee 98% accuracy for complete words or sentences, particularly in real-world scanning conditions.
Concerning throughput, the system is said to deliver relatively rapid processing, aiming to extract and potentially translate text from images within seconds. Practical performance, however, would likely be influenced by factors such as image resolution, file size, the density and complexity of text within the image, and the operational load on the processing infrastructure at any given time. Consistent "seconds" across diverse inputs can be a challenging target.
Input flexibility reportedly extends to common image file types, including JPG, PNG, and TIFF. While standard, assessing support for multi-page formats like PDF, which often bundle images or scanned content differently, is a practical consideration for workflow integration.
Language coverage is cited as broad, reportedly supporting over 100 languages for OCR and likely translation. The technical challenge with a large language set lies not just in adding support but maintaining comparable accuracy and performance across scripts and linguistic structures that vary significantly in complexity and available training data. Performance is often non-uniform across such a wide range.
The stated capability for batch processing is central to its value proposition for volume users. The technical details regarding how batch submission is managed (e.g., API, dedicated upload interface), error handling within batches, and the queuing mechanism would be pertinent for understanding its operational reliability for bulk tasks.
Incorporating user feedback into model refinement is mentioned, a common practice in machine learning for improving recognition models. The efficacy of such a system depends on the specific mechanism for capturing errors, the quality and volume of user corrections, and the efficiency of retraining and deploying updated models. It's an iterative process with variable impact on perceived accuracy over time.
While automation inherently tends to be more cost-effective than manual processes for repetitive tasks like text extraction and initial translation, the critical aspect from a workflow perspective is the quality of the output. Machine translation and even highly accurate OCR can produce errors requiring post-editing, which adds back human cost. The assessment of true cost-effectiveness needs to factor in the potential expense of downstream correction and review relative to quality levels achieved.
The $99 monthly price point for this specific volume cap positions the service as potentially accessible to smaller operations compared to much higher-tier enterprise solutions or dedicated human services. However, for users with highly inconsistent volumes or requirements below 500 images per month, this fixed cost might not represent the most economical option, suggesting a need to evaluate usage patterns against the tier structure.
Regarding data handling, standard practice for services processing user content involves implementing security protocols. The mention of not storing uploaded images indefinitely addresses a necessary privacy consideration. Technical specifics around the duration of retention, encryption methods, and compliance standards (if applicable) would be key details for users evaluating data security posture.
7 Cost-Effective AI Translation Tools with OCR Capabilities in 2024 A Technical Analysis - Textract Takes Screenshots to Text in 12 Languages Below $8
Amazon Textract is presented as a system for converting visual material like screenshots and documents into readable text, claiming support for up to 12 languages at price points that can reportedly be below $8 depending on the amount of use. It leverages optical character recognition (OCR) technology but aims beyond simple text extraction, designed to identify and process structured information like data within forms and tables without relying on user-defined templates. This capability helps preserve the relationships between pieces of information, potentially reducing the effort needed to review the extracted output compared to traditional methods that might just produce a stream of text. The pricing structure is based on actual usage, which means the cost directly reflects the volume of processing, intended to offer cost-effectiveness for limited or specific tasks. However, when dealing with documents that blend multiple languages, it might be necessary to preprocess the text using OCR tools better tuned for those individual languages before combining results for Textract to handle, suggesting a potential limitation in handling complex multilingual inputs seamlessly on its own. It is positioned for document-centric tasks where understanding layout and structure is as important as recognizing characters.
Looking at another tool for getting text out of images, this capability is described as taking screenshots and converting them to text, with a reported capacity across up to twelve languages for a cost point cited at below eight dollars. This suggests a focus on affordability for specific tasks.
Technically, this relies on optical character recognition (OCR), processing the visual data in the screenshot to identify characters and words. What's notable here is the ambition to handle multiple languages within this apparently low-cost offering.
From an engineering standpoint, building an OCR system robust enough for varying image qualities inherent in screenshots (different resolutions, compression artifacts, overlays) while also accurately supporting diverse scripts and language structures is complex.
Information indicates the underlying technology aims to go beyond simple text extraction, potentially identifying and extracting data from forms and tables, which hints at a more advanced document processing layer built upon the core OCR.
Unlike some models that rely on fixed monthly fees or specific batch limits, the pricing structure mentioned suggests a usage-based approach for certain tiers, meaning users might only pay for the actual volume processed. This can be more cost-effective for unpredictable or low usage volumes.
The accuracy of conversion, particularly in real-world scenarios with less-than-ideal input images or complex language mixtures, remains a key technical challenge for any OCR system, including this one. How well it handles factors like handwritten text or highly stylized fonts compared to printed text would be worth evaluating.
Positioned as a managed service, its utility often comes from potential integration via APIs into other applications and workflows. This shifts the operational burden away from the user managing the OCR engine itself but means reliance on the provider's infrastructure and service availability.
For documents or images containing elements other than just flat text, such as checkboxes, structured fields, or complex tables, the capability to correctly identify and extract these structured data points is a significant step beyond traditional OCR and appears to be a focus area for this tool.
While the general speed of conversion is often marketed as rapid (e.g., "seconds"), the actual processing time can be influenced by factors like image complexity, file size, and the current load on the service infrastructure. Real-world throughput can vary.
Considering the processing of visual information that might contain sensitive data, understanding the service provider's approach to data handling, temporary storage, and security protocols for uploaded images is a necessary technical and privacy evaluation point for any potential user.
7 Cost-Effective AI Translation Tools with OCR Capabilities in 2024 A Technical Analysis - Nota Converts Handwriting in 8 Asian Scripts at $99 Monthly
A service specifically aimed at digitizing handwritten content in eight Asian scripts is available for a reported $99 monthly fee. This tool, described as a handwriting OCR solution, leverages artificial intelligence to convert notes, documents, and other handwritten materials into digital text. Claims suggest it can achieve accuracy levels ranging from 80% to 90% with clear handwriting, with the AI working to maintain performance even when the input is less legible. While presented as an option for users dealing with handwritten materials in a range of Asian languages, the effectiveness and consistency of such a system across different scripts and individual handwriting styles would need careful technical evaluation. The fixed monthly cost might be appealing for predictable workloads, but evaluating its true value would involve comparing its specific capabilities and accuracy across diverse Asian scripts against alternative methods or tools that might offer different pricing models or broader language support.
Nota is put forward as an optical character recognition (OCR) system engineered with the specific aim of digitizing handwritten content. Its stated scope includes support for processing text written in eight distinct Asian scripts. Access to this tool is offered via a subscription model, priced at $99 per month. The accuracy of its conversion is reported to be in the range of 80-90% for handwriting that is considered clear. While it's said to leverage advanced AI capabilities to potentially handle less legible inputs, the inherent difficulty in achieving consistent, high-fidelity recognition across the vast spectrum of individual handwriting styles, especially in complex scripts, remains a notable technical hurdle. For those needing to convert handwritten notes or documents specifically within this set of Asian languages, the service is presented as a potential technical means to achieve digital text, tackling a problem where standard print-based OCR typically falls short.
7 Cost-Effective AI Translation Tools with OCR Capabilities in 2024 A Technical Analysis - PaperScan Pro Manages Document Archives with $7 Monthly Plan
PaperScan Pro is presenting itself as an accessible option for handling document archives with a monthly cost set around $7. This software is designed to assist users in acquiring and organizing physical documents by supporting scanning and optical character recognition across a considerable range of file formats, reportedly over 100. It offers features intended to streamline document workflows, such as correcting scanned images automatically, managing and viewing documents, and facilitating uploads directly to common cloud storage platforms. The OCR capability itself is said to work with documents in more than 30 languages. While positioned as a cost-effective tool for basic document management tasks, it's worth considering whether such a low monthly price point fully supports robust, scalable archive management for complex organizational needs or if the primary utility lies in simpler digitization and personal filing. Nevertheless, for users seeking a straightforward tool to get scanned documents organized and searchable, particularly across multiple languages, this offering aims to lower the barrier to entry.
Examining tools that facilitate document handling as part of digitization efforts, PaperScan Pro offers a distinct proposition with a monthly access plan priced at seven dollars. This particular tier appears designed to make optical character recognition (OCR) and fundamental document organization more accessible, presenting an alternative to more expensive one-time purchases or high-volume enterprise solutions; for context, the Pro edition itself has reportedly been sold for considerably more, around $149 typically.
From a technical standpoint, PaperScan Pro focuses on the initial phases of document processing. It interfaces with standard scanner drivers, supporting both TWAIN and WIA interfaces, which broadens compatibility with a range of scanning hardware. Once documents are acquired, the software's OCR engine is key, capable of recognizing text across more than thirty languages. This enables conversion of scanned images or PDFs into searchable or editable text formats, a fundamental step for integrating paper documents into digital workflows.
Beyond basic scanning and recognition, the tool includes features for image refinement, such as automatic cropping and straightening, which are useful for improving the quality of the input data before OCR processing. It also handles a large variety of file formats, reportedly over 100 types, for input and output. For higher volume tasks, it offers batch processing capabilities and tools like automatic blank page removal, which streamline the digitization of multiple documents simultaneously. Annotation features are also included, allowing for mark-ups on the processed documents.
Integration with cloud storage services is supported, enabling direct uploads of processed documents to platforms like Dropbox and Google Drive, which can be convenient for storage and sharing. While its OCR supports a decent number of languages, the primary utility seems centered around efficient document capture and conversion into a digital format, rather than integrated AI translation services, distinguishing its role from tools specifically focused on automated linguistic conversion after recognition. Its functionality appears squarely aimed at making the physical-to-digital document transition manageable, especially for users or smaller operations prioritizing cost-effectiveness for this specific stage.
More Posts from aitranslations.io: