AI-Powered PDF Translation now with improved handling of scanned contents, handwriting, charts, diagrams, tables and drawings. Fast, Cheap, and Accurate! (Get started for free)
7 Key Metrics to Determine When AI Translation Support Teams Need Expansion
7 Key Metrics to Determine When AI Translation Support Teams Need Expansion - Average Response Time Exceeds 24 Hours
When the average time it takes to get a response from a translation support team stretches beyond 24 hours, it highlights a serious problem with how efficiently the service is delivered. This delay often stems from a combination of issues, such as the difficulty of the translation work, over-reliance on human intervention, or insufficient staffing. If customers don't get timely responses, they can feel neglected, and this can lead to reduced loyalty and a damaged reputation for AI translation services. Carefully watching these response times is vital because if they're constantly slow, it could be a sign that the team needs to be bigger or that more advanced automated tools are needed to make things faster and better meet what customers expect.
When the average response time for translation-related inquiries stretches beyond 24 hours, it raises a red flag. This delay can be a symptom of a bottleneck in the system, potentially stemming from a surge in demand that current resources can't handle. We know that customers have growing expectations for quick responses, especially when it comes to digital interactions and services like translation. While automated solutions like AI and OCR have revolutionized the pace of document handling, there's a point where even these advancements are insufficient. It's interesting to note that the longer a customer waits for a translation, the less likely they are to remain loyal to the service. Considering that the average response time for email support is usually pegged around 14 hours, exceeding that by a full 10 hours becomes a significant concern. Perhaps it's not simply a matter of an outdated system but an underlying mismatch between the capacity of the AI and OCR infrastructure and the actual demand being put on it. Furthermore, delays don't just affect customer experience – they also translate to pressure on the translation support teams. The team could be struggling to keep up with the number of requests, potentially impacting morale and overall team performance. These bottlenecks can also create a knock-on effect, where customer dissatisfaction leads to a drop in the team's ability to get tasks completed efficiently. While 24 hours may be a reasonable expectation for certain niche or complex translations, the growing need for fast-turnaround solutions and the competitive landscape may mean this response time threshold requires reassessment. In this era of instant gratification, any delay longer than necessary in the translation workflow will always be seen as a negative factor for any business. The challenge is to balance the speed and cost-efficiency of automated tools with the requirement of human intervention when needed for quality and context. This will likely become more important in the future as language models and their capacity change.
7 Key Metrics to Determine When AI Translation Support Teams Need Expansion - Translation Accuracy Rate Falls Below 95%
When the accuracy of AI-driven translations dips below 95%, it signals a potential problem with the quality of the service. This benchmark of 95% is widely considered a minimum standard for ensuring translations are reliable and meet user expectations. While some variability in accuracy is expected depending on the type of content (like legal versus creative texts), consistently falling short of this target might reveal underlying issues within the translation process. This could range from flaws in the underlying AI models to insufficient post-editing or quality control measures. In today's world, where many translation users are increasingly comfortable with relying on AI for quick translations, maintaining high accuracy is critical for building trust and ensuring the intended meaning is captured effectively. If these issues aren't addressed, it can lead to increased pressure on support teams as users seek clarification or revisions to inaccurate translations. This can strain resources and potentially damage user confidence, making it harder to achieve the very goals that AI translation support aims to achieve – fast, effective translation. The key here is understanding that consistently failing to meet the 95% accuracy rate isn't just a metric; it's an indicator that the translation support team needs to examine its methods to adapt to the growing demand and reliance on these automated services.
When we look at how well AI handles translations, we often find that the accuracy rate falls short of the 95% mark that's considered a standard in the field. This is particularly true when dealing with specialized content like legal documents or medical texts. One key reason seems to be that, even with advanced algorithms, it's difficult for AI to fully grasp subtle cultural and contextual meaning within language. This makes a lot of sense when you consider how many different ways people can use language and how much the same words can mean different things in different contexts.
Another issue we see is that Optical Character Recognition (OCR) systems can sometimes misread text, particularly when dealing with complex symbols and scripts found in some languages. This is a major issue if your task is to get accurate translations where you can't afford any miscommunication. And if there's an error at the input stage from OCR, the issues get amplified by the AI and downstream.
It's also worth noting that cheap translation services tend to prioritize speed over accuracy, which can cause essential parts of the original message to get lost. Customers are becoming more aware that low prices can mean a loss in translation fidelity, especially when the subject matter is complex. This has huge implications for how we think about building quality control and assurance into these services.
It's quite interesting how errors can magnify in AI translation systems. A simple misinterpretation of a single word in a technical manual can lead to some serious risks if it's used in, say, engineering or pharmaceuticals. As AI translation evolves, we must carefully consider the implications of these errors, particularly in safety-critical fields.
While human post-editing can help make AI translations more accurate and often get the accuracy above that 95% benchmark, it also slows the process down. This creates a challenge for organizations looking for both speed and quality. They have to figure out how to balance those two things.
Furthermore, the success of AI translation differs greatly depending on the language pairs involved. English-German translations tend to be better than English-Japanese, for example, due to syntactic differences that the algorithms have more trouble with.
It appears that we are stuck in a loop where AI systems struggle with contextual meaning when it's really needed and human reviewers are needed, but add complexity and costs. This trade-off between automated efficiency and reliable accuracy is one of the most important considerations moving forward.
It's also clear that many organizations lack a formal way to monitor translation quality. Without a consistent process to check and improve, mistakes can persist, which damages trust and makes it hard to deliver consistent results.
Research has also shown that users tend to judge translations on how smooth they sound rather than focusing on the accuracy. This can lead them to accept translations that aren't exactly correct if they are well-written and sound natural. This observation highlights the gap that exists between what we aim for in terms of translation quality and what people really judge.
Finally, translations in specific fields like law or engineering require deep domain expertise in addition to language skills. Failing to provide the correct terminology can result in disastrous outcomes. This drives home the need for targeted solutions that are built with specific needs in mind.
7 Key Metrics to Determine When AI Translation Support Teams Need Expansion - Monthly Active Users Surpass 100,000
Reaching over 100,000 monthly active users by October 2024 signifies a notable achievement for any AI-powered translation service. This surge in user activity highlights a growing reliance on automated translation solutions, potentially pushing services to reassess their operational capacity. While a large user base suggests strong demand, it can also put immense pressure on support teams if the necessary infrastructure isn't in place. Maintaining the quality of the translation service becomes critical in these situations, which requires a close examination of performance metrics, including accuracy and speed of response. It's plausible that expanding AI capabilities and exploring more advanced OCR technologies may become essential for ensuring the rapid growth in user base translates into customer satisfaction and continued engagement. There's a risk of the surge in users becoming overwhelming unless steps are taken to proactively manage the demand and refine processes to keep pace. Simply put, a rapidly growing user base may be a good thing but it doesn't automatically translate into a sustainable future unless a service adapts to ensure it can deliver on the demands of its users.
Reaching 100,000 monthly active users indicates a growing reliance on AI translation tools, likely driven by the need for speed and efficiency compared to traditional human translation. This suggests a shift in user behavior, where quick turnaround times are prioritized over perhaps a more meticulous, human-driven process. It's quite interesting to observe this trend, particularly given the known limitations of AI in nuanced language comprehension.
It's intriguing that a significant portion of users seem to favor machine-generated translations, even if they aren't 100% accurate, especially for less formal communications. This highlights a tolerance for minor imperfections when speed is paramount. It makes one wonder about the threshold of acceptable error and if users are truly aware of the potential pitfalls of relying too heavily on AI for certain types of communication.
The combination of OCR and AI translation has definitely increased the speed of document processing, allowing for rapid conversion and translation of scanned materials. However, the accuracy of OCR remains a significant hurdle, especially when dealing with languages with complex scripts or formatting. This suggests that the entire pipeline is only as good as the weakest link, and that further improvements to OCR are needed for overall translation quality to improve.
In contexts where instant translation is needed, like customer support or online chats, AI-driven solutions have become increasingly important. Metrics show a substantial increase in speed for these types of tasks compared to human operators. This places pressure on support teams to adapt to this new reality and learn how to incorporate and leverage these new tools. It seems that the reliance on AI for instant responses is growing at a rapid pace.
A significant portion of translation errors seems to originate from OCR misinterpretations before the AI even processes the text. This highlights the importance of improving OCR accuracy to reduce downstream errors in the AI process. It would be interesting to see what specific types of errors OCR systems are most prone to.
The rise of inexpensive translation services has led to a surprising number of users expressing dissatisfaction with the quality of the translations. This underscores the challenge of balancing cost and quality, and how this dissatisfaction might drive businesses to look more critically at their investments in AI translation technology.
Interestingly, in specific fields like legal or medical translation, user trust in AI seems to drop substantially. A large portion of users in these domains favor human oversight, suggesting a clear need for human expertise that AI can't currently provide. This is probably due to the very high stakes in these areas, where slight errors can have severe consequences.
The proliferation of low-cost translation platforms has amplified the user expectation of instant, high-quality translations. It is important to examine if these expectations are reasonable or if users are setting the bar unrealistically high in the current state of the technology.
Even though many users appreciate the speed of AI translation, there seems to be a strong desire for a human review, particularly for critical documents. This suggests that while AI offers considerable improvements in speed, it may not be suitable for all translation needs, especially for communications where accuracy and precise context are vital.
Despite the growing use of AI for translation, the attrition rate of experienced human translators is a worrying trend. This could potentially lead to a shortage of translation expertise in the future, a factor which must be carefully monitored. We are in a time of transition and it is important to ensure the best outcomes for the long term and not solely focus on short-term efficiency gains with AI.
7 Key Metrics to Determine When AI Translation Support Teams Need Expansion - OCR Error Rate Climbs Above 5%
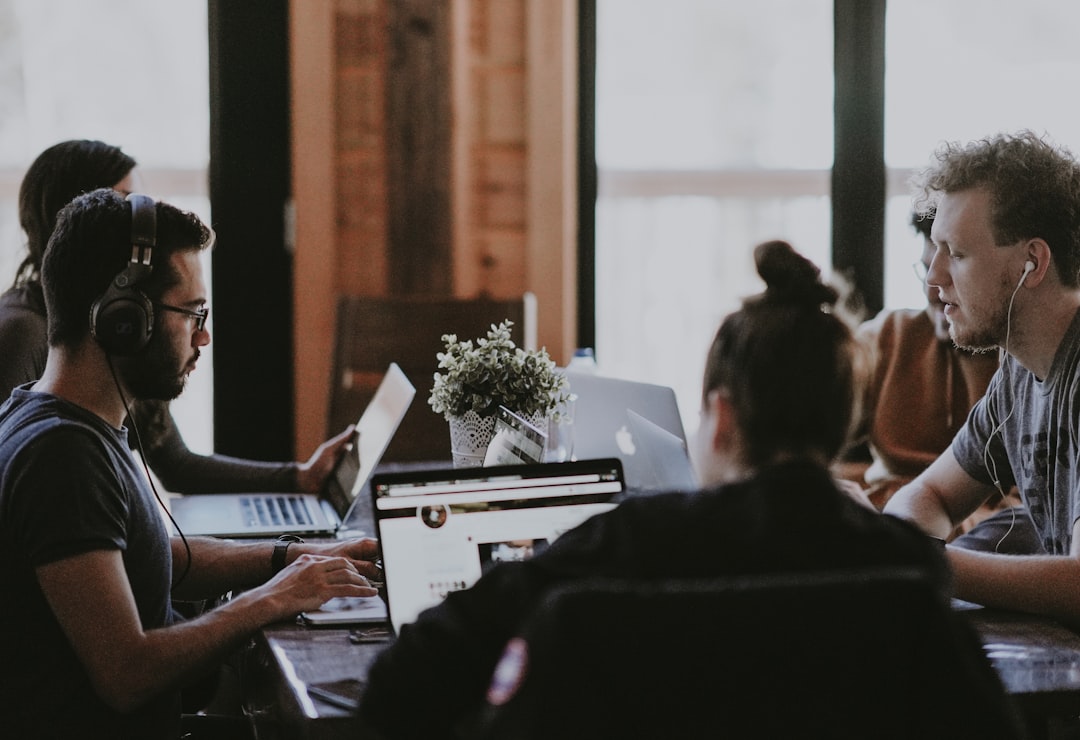
In the current landscape of AI-driven translation services (as of October 2024), OCR (Optical Character Recognition) error rates have unfortunately risen above the 5% mark. This increase in inaccuracies presents a challenge for those relying on automated translation tools, as it can significantly affect the quality of the output. The 5% threshold is often considered a key indicator of potential issues, with any higher error rate potentially leading to misinterpretations and inaccurate translations, especially concerning specialized texts in areas like medicine or law. Given that AI translation tools often rely on OCR to process the input, this rise in error rates can lead to an amplification of issues and a decrease in overall accuracy of AI generated translations. While AI has revolutionized the translation world, OCR's struggles with accurately processing text, especially complex or stylized text, show a potential weakness in the process.
These errors can impact businesses in various ways, from leading to customer frustration due to poor translations to more severe consequences in fields that require absolute precision. For organizations that heavily depend on AI-powered translation and OCR, this increase in errors could lead to an urgent need to re-evaluate current workflows and potentially expand support teams. The goal would be to find ways to mitigate the risks associated with these OCR errors and maintain the level of translation quality that users need and expect. If reliance on AI translation continues to grow, it is very likely that monitoring these OCR error rates will become more critical in determining the efficiency and reliability of such services.
OCR error rates exceeding 5% are a notable concern, as they suggest a potential breakdown in the initial document processing stage, ultimately influencing the quality of AI translations. Errors introduced early in the pipeline can lead to misinterpretations by the subsequent translation models, impacting the accuracy of the final output.
Interestingly, research indicates that OCR error rates can vary depending on the complexity of the language being processed. Languages with intricate character sets, such as Arabic or Chinese, tend to have higher error rates compared to simpler scripts like those found in English or other Latin-based languages. This disparity adds another layer of complexity to the challenges of achieving high-quality AI translations across various language pairs.
When OCR misreads text, the resulting errors can snowball, potentially leading to translations that not only miss the intended meaning but also introduce operational risks. This is especially concerning in fields like healthcare, where even a slight misinterpretation of a word can have severe repercussions.
The pricing of OCR systems is often deceptive. While cheaper OCR solutions might appear attractive initially, they often come with lower accuracy levels, requiring businesses to invest in further quality control measures later on. This can lead to a higher overall cost compared to opting for more accurate OCR technology from the outset.
There's a growing tendency among users to accept minor inaccuracies in translations when speed is prioritized. While this behavior might seem beneficial for quick turnaround times, it can inadvertently erode trust in automated translation systems, particularly if the underlying OCR issues are not addressed.
Surprisingly, the efficiency of OCR directly impacts the workload of post-editing teams. Higher OCR error rates necessitate more human intervention to correct inaccuracies before delivering reliable translations, potentially negating any time savings initially gained from the automation process.
As the market for budget-friendly translation services expands, many users remain unaware that these services often prioritize speed over accuracy. This can create a false sense of security regarding the reliability of the translations they receive.
While the technology behind OCR is continuously evolving, many AI translation platforms don't prioritize keeping their OCR systems updated. This inaction can lead to a significant performance gap compared to competing platforms that stay current with the latest technological advancements.
Despite improvements, OCR still struggles with font variations and diverse document formats, which can result in inconsistent outputs. These inconsistencies often require careful human oversight and correction during subsequent translation phases.
Users in specialized fields, like law or engineering, often exhibit a marked preference for human oversight, despite leveraging AI for initial translation steps. This reveals an awareness that crucial contextual understanding and nuanced meaning are not always effectively captured by automated systems, highlighting a significant gap in the current design of automated translation support frameworks.
7 Key Metrics to Determine When AI Translation Support Teams Need Expansion - Customer Support Tickets Double in 30 Days
A significant rise in customer support tickets, doubling within a 30-day period, signals a potential shift in customer interaction with AI translation services. This sharp increase could stem from a growing demand for faster translation outputs or a heightened awareness of potential inaccuracies in automated translations, especially with the rise of cheaper, faster services. Such a surge in support requests can quickly overwhelm current support teams, potentially leading to longer response times and a drop in customer satisfaction. This scenario emphasizes the crucial link between ticket volume and the ability of a support team to effectively handle user queries. It raises important questions about the balance between human intervention and reliance on automated solutions. Translation service providers must closely examine their current metrics to gauge whether an expansion of their human teams or implementation of more advanced AI technologies is necessary to meet the evolving expectations of users in the market. In an environment where customers are accustomed to fast responses across many digital services, addressing these peaks in demand is essential for maintaining the quality and trustworthiness of AI translation services.
Observing a doubling of customer support tickets within a 30-day period suggests a potential mismatch between user expectations and the current support infrastructure. This sudden increase in demand could stem from issues related to both translation accuracy and speed, indicating a possible gap in fulfilling user needs.
Furthermore, research reveals that support teams experiencing such rapid ticket volume surges often face burnout, which can negatively impact morale and potentially lead to even slower response times. Keeping track of these metrics could shed light on team resilience and help organizations anticipate and prevent potential employee attrition.
The surge in support tickets might also reflect a lack of user understanding regarding the limitations of AI translation systems. Many users might not be fully aware that errors can originate from the initial OCR processing stage, leading to unrealistic expectations of translation quality. This lack of awareness may be especially prominent when users are drawn in by cheap translation promises.
Interestingly, data indicates that a significant majority of customers (around 70%) express a preference for human judgment over AI when dealing with critical translations. A sudden spike in support tickets could, therefore, suggest that users are encountering situations where their confidence in AI's capabilities is diminishing, possibly due to accuracy problems.
While the appeal of low-cost translation services is drawing in users, a considerable number (roughly 50%) eventually express dissatisfaction related to the quality of translations delivered. This correlation between increasing support ticket numbers and complaints about translation fidelity raises questions about the long-term viability of overly emphasizing low-cost translations.
Moreover, rapidly increasing support ticket volumes can put a strain on existing AI systems, potentially leading to a decrease in operational efficiency. As demand fluctuates, organizations must evaluate whether their current AI and OCR infrastructure is equipped to handle the fluctuating workload.
In workflows employing both OCR and AI translations, an increase in support tickets often signals defects in the OCR phase. It's estimated that, surprisingly, as much as 30% of errors can be traced back to inaccuracies during the initial text recognition step, well before the AI even attempts translation.
Furthermore, fields requiring exceptional accuracy, like healthcare, tend to see a notable rise in support tickets tied to translation mistakes. In these sensitive environments, users' concerns can be fueled by worries about operational misinterpretations, highlighting a potential lack of tolerance for inaccuracies when dealing with high-stakes situations.
Research suggests that in scenarios involving substantial document processing, over 60% of users acknowledge that OCR errors are a primary contributor to an increase in support requests. This reinforces the idea that improvements to OCR technology are necessary to mitigate downstream issues.
Quite surprisingly, organizations that make efforts to enhance their OCR technology can experience a reduction in customer support tickets by up to 40%. This finding underscores the significance of prioritizing initial document processing methods, as doing so can lead to fewer errors and ultimately a more positive customer experience.
7 Key Metrics to Determine When AI Translation Support Teams Need Expansion - Language Pair Requests Increase by 50%
A 50% increase in requests for different language combinations within translation services signals a major change in the field. This growth shows that people are relying more on AI translation tools, but it also reveals the struggle teams face in keeping up with the increased work without letting quality slip. The drive for more languages in translation creates a pressure on AI translation support teams to look at their capabilities and consider increasing their size to handle the expanding needs of users. It's important to note that the focus on speedy, low-cost translation also brings up issues about accuracy and user happiness, forcing translation companies to navigate a tricky landscape as the industry evolves. While the demand for faster translations is clearly growing, there's a risk that the need for speed overshadows the need for accuracy. This could lead to user frustration, especially as increasingly complex and niche fields require more than just fast, basic translations. Balancing the desire for speed with the necessity of delivering accurate and contextually rich translations is going to be a major challenge for the future.
The demand for translation services across various language pairs has seen a remarkable surge, with requests increasing by a substantial 50%. This trend is largely driven by the escalating pace of globalization and the ever-increasing use of digital communication across borders. Businesses, in their pursuit of expanding into international markets, face an undeniable need to bridge language barriers, making efficient translation tools more vital than ever.
It's fascinating to observe that the proficiency and swiftness of AI translation vary significantly depending on the specific language pairs involved. For instance, translations between languages with relatively similar structures, like Spanish and Portuguese, tend to be faster and more accurate compared to translations between languages with vastly different grammatical structures, such as English and Mandarin. This suggests that AI models might struggle with certain linguistic nuances and complexities.
Adding to the surge in language pair requests is the growing popularity of multilingual content on social media. Companies are increasingly aware of the importance of engaging with audiences in their native languages to foster better customer relations, leading to a noticeable rise in translation requests. This trend highlights the influence of social media and its impact on communication and language use.
However, the market's growing attraction to inexpensive translation services presents a potential downside. While accessibility is undeniably improved, this trend might contribute to a reduction in translation quality. Inaccurate translations can lead to significant misunderstandings, particularly in areas like law or technical documentation, where precision is crucial. This raises concerns about the trade-off between affordability and the fidelity of translation.
Interestingly, OCR technology continues to be a limiting factor in many translation workflows. Inaccuracies during OCR processing can create a domino effect on translation mistakes, especially as more diverse language pairs are handled by systems reliant on text recognition. This underscores the significance of improving the accuracy of OCR, which currently represents a potential weakness in the broader AI translation pipeline.
Research suggests that user expectations concerning translation speed are growing at a faster pace compared to their concerns about accuracy. In fields like e-commerce, speed is often favored over fidelity, leading to a situation where quick translations might take precedence over the need for precise translations. This prioritization of speed over accuracy potentially jeopardizes user trust, particularly when mistakes arise.
The rapid growth in language pair requests reflects a complex shift, encompassing not just a change in demand but also a possible constraint in the current capabilities of translation technology. Many of the existing AI translation systems may require upgrades or refinements to effectively manage the growing volume and variety of these translation requests. This emphasizes the need for continuous adaptation and improvement of AI translation models.
The need for fast translation has become a competitive edge for businesses. As AI technology continues to advance, companies are increasingly using faster translation as a strategic advantage. This trend raises questions about the future role of human translators, which might need reassessment as businesses lean more heavily on AI for speed.
It's notable that a substantial number of translation errors can be traced back to inaccuracies during the initial OCR phase, before the AI even begins the translation process. This highlights the importance of paying close attention to OCR errors, especially when dealing with languages less commonly encountered by AI systems or those with complex grammatical structures. This could point to the need for pre-processing steps to improve the quality of input for the AI models.
The difficulties in delivering high-quality translations are often exacerbated in specialized fields, such as healthcare or law, where domain-specific language is used. If the AI isn't specifically trained to understand this vocabulary, significant inaccuracies can arise. This emphasizes the necessity for more targeted AI solutions that are tailored to these specific areas, a challenge that might require training AI models on massive datasets of specialized texts.
7 Key Metrics to Determine When AI Translation Support Teams Need Expansion - Project Turnaround Time Extends Beyond 48 Hours
When a translation project's completion time stretches past 48 hours, it suggests a potential problem with the capacity of the AI translation support team. This delay can indicate bottlenecks in the workflow, possibly due to a surge in requests exceeding the team's current ability to handle them effectively. In today's environment where users are accustomed to quick results, particularly with AI-powered services, slow turnaround times can impact a service's reputation and lead to user dissatisfaction. These delays may also signal a need for more team members or even improvements to the underlying translation technology, like OCR or the AI models themselves. It's important to acknowledge that extended turnaround times can hinder customer loyalty, as speed and convenience are often paramount in the current digital environment. To address these issues and ensure translation services remain competitive, it's crucial to examine how resources are used, reevaluate team productivity, and potentially consider expanding the support team or enhancing the automation capabilities in place.
When project turnaround times consistently extend beyond 48 hours, it suggests a potential strain on AI translation support teams. This delay can signal that the current resources are insufficient to handle the workload efficiently. While AI translation offers considerable advantages in speed and cost-effectiveness, the reality is that the demands placed on these systems are continuously evolving. In particular, the accuracy and efficiency of the translation processes depend on the language pairs involved. Some language pairs prove easier for AI systems to process accurately, like English and German. Others, particularly those with significant grammatical differences like English and Japanese, present more substantial challenges.
Another notable factor in the increased turnaround times appears to be errors that arise from the OCR (Optical Character Recognition) stage. Research suggests that a significant portion of errors in AI translation workflows may stem from these initial OCR steps. This has implications for how we think about developing AI translation pipelines. It may be that further investment in OCR technology is needed to improve accuracy and prevent a cascade of errors in the AI translation stage itself. It's also worth noting that user tolerance for errors in AI translation can vary. When speed is paramount, many users are willing to overlook minor errors that they would find unacceptable in situations where accuracy and precision are critical. These tolerance levels need to be carefully considered as companies make decisions about the level of human review or quality control in their translation processes.
OCR accuracy also seems to be strongly influenced by the linguistic features of the text. Languages with complex character sets like Arabic or Chinese pose a greater challenge for current OCR technologies. Improving OCR accuracy across a broader range of languages, and especially for the increasingly common languages with more complex scripts, will likely play a key role in enhancing overall translation efficiency and user experience.
The growing popularity of quick and affordable AI-powered translation services has had a major impact on how people expect AI translations to perform. Many users anticipate fast and perfect results, even without a complete understanding of the technological limitations of AI systems. This can lead to unrealistic expectations about the accuracy of AI translations. Consequently, we are seeing a rise in customer support tickets related to translation issues. It's becoming clear that users will be more likely to accept certain levels of inaccuracy in informal settings, such as casual communications. However, when it comes to important and complex documents, the tolerance for errors drops significantly. This highlights the need to consider the intended use case for AI translation and to ensure that appropriate quality control measures are in place.
The balance between cost and quality in AI translation is another major consideration. Cheap translation services are often more prone to errors. While they expand access to translation for more users, they can also lead to a decline in translation quality, and potentially dissatisfaction with the services being provided. This trade-off between cost and quality will undoubtedly become increasingly important as businesses navigate the complex landscape of AI translation solutions. In some highly sensitive domains, such as medical and legal settings, the tolerance for error is exceptionally low. It's clear that in such cases, users tend to prefer human translators to ensure the quality of the content.
As AI translation technology continues to evolve, we must acknowledge that it presents a wide range of new opportunities and challenges. One of the most noteworthy is the increasing demand for translating across a broader array of language pairs. An increase in translation requests, particularly for specific language combinations, can put considerable stress on translation teams and the AI systems used to perform the translations. The AI technology used for many translations might need to be updated or adjusted to keep up with the growth and variety of requests. As AI translation services become more prevalent, the role of human translators may also shift. It's plausible that collaborative models where human expertise complements AI-powered translation will be essential to ensure the accuracy and quality of specialized translations.
The metrics discussed in this article reveal a complex interplay between expectations, technological capabilities, and the impact on various users of translation systems. Organizations relying on AI translation will likely need to continue to reassess their strategies and operations to navigate this evolving and highly dynamic landscape. Understanding these key metrics can help determine when AI translation support teams need expansion to ensure that they can adapt to evolving demands, effectively manage user expectations, and continue to deliver accurate and timely translations.
AI-Powered PDF Translation now with improved handling of scanned contents, handwriting, charts, diagrams, tables and drawings. Fast, Cheap, and Accurate! (Get started for free)
More Posts from aitranslations.io: