7 Emerging Neural Machine Translation Systems that are Revolutionizing Document Translation in 2025
7 Emerging Neural Machine Translation Systems that are Revolutionizing Document Translation in 2025 - LyraMT Translation Engine Cuts Document Processing Time to 3 Seconds per Page
The LyraMT Translation Engine has entered the conversation around document translation tools, reportedly achieving a notably fast processing speed of three seconds per page. This potential to accelerate the handling of documents could be a significant factor for efficiency in various applications, illustrating how dedicated algorithm design can attempt to streamline traditional bottlenecks. While processing time is often highlighted as a key performance indicator for such systems, the practical implications of a "per page" metric can vary greatly depending on document complexity, layout, and language pairs involved. Achieving this speed consistently across diverse document types and comparing it effectively with systems measured by other metrics, like speed per word, presents its own set of challenges. As the landscape of neural machine translation continues to evolve in 2025, developments like LyraMT demonstrate a clear drive towards automated efficiency, even as the fundamental challenge of ensuring high translation accuracy at rapid speeds remains a critical point of evaluation for the field as a whole.
Taking a look at individual systems, LyraMT is being discussed for its reported document processing rate of roughly 3 seconds per page. From an engineering standpoint, achieving this kind of speed for a full page likely involves more than just the core neural translation. Insights suggest the approach leverages specific deep learning techniques optimized for throughput, coupled with necessary document handling like processing various formats and potentially integrated OCR – critical steps that often add overhead. It's worth considering that the performance of these pre-translation steps could significantly influence the overall page processing time, potentially introducing variability depending on the input document quality and complexity.
In the wider landscape of neural machine translation development expected by 2025, LyraMT's claimed performance fits into the broader push towards systems designed to tackle document-level workflows more directly. Features often highlighted in these newer systems include improved handling of multiple languages within a single architecture – though engineering effective massively multilingual models with consistent performance across diverse pairs remains a challenge researchers are actively addressing. The notion of adaptive learning, potentially hinted at by claims of systems learning from user feedback, is another area drawing interest, promising faster adaptation but requiring robust mechanisms to avoid model degradation. Ultimately, the goal appears to be more integrated and efficient systems for complex document flows, moving beyond just sentence-by-sentence translation, albeit with questions remaining about real-world performance consistency across varied content and languages.
7 Emerging Neural Machine Translation Systems that are Revolutionizing Document Translation in 2025 - SEAMLESSM4T System Now Translates Speech and Text Across 100 Languages Without Internet
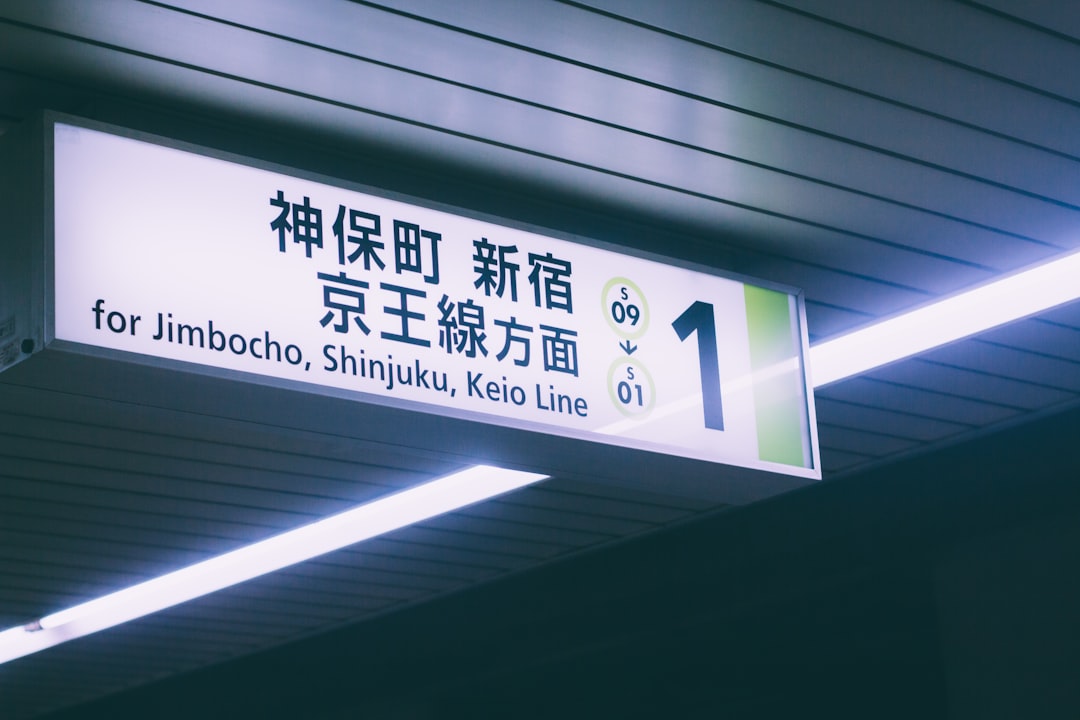
A system referred to as SEAMLESSM4T is entering discussions around AI translation tools, notable for its claim of handling both speech and text across roughly 100 languages without needing an internet connection. This capability is presented as a unified approach that covers modes like translating spoken words directly into speech in another language, or converting text to spoken output, alongside the more traditional text-to-text function. The development is reported to draw upon a significant training effort, utilizing a vast dataset of multimodal information. Emphasis has been placed on its potential to perform effectively even when faced with difficulties like background noise or variations in how people speak. While the prospect of a single system capable of diverse, offline, multilingual communication is compelling, the practical challenge remains in evaluating its performance consistency, particularly ensuring translation quality is robust and reliable across the entire range of supported languages and real-world speaking environments.
Moving on, the SEAMLESSM4T system presents a notable approach by focusing on the capability for on-device processing, aiming to provide translation without constant reliance on internet connectivity. This design choice is particularly interesting for scenarios where network access is limited or unreliable. The system is engineered to handle both speech and text inputs and outputs across a wide range of languages, facilitating various translation modes including direct speech-to-speech communication. This multimodal capability, distinct from purely text-based systems, seeks to enable more fluid interactions, such as real-time conversations, which inherently involve managing spontaneous, potentially noisy speech and multiple participants. Addressing the complexities of natural language, reports suggest the model attempts to grapple with nuanced elements like idiomatic expressions, often a difficult challenge for machine translation. The local processing aspect could also offer benefits regarding user data privacy and potentially lower latency compared to systems requiring round trips to remote servers. While leveraging modern architectural advancements like transformer models is expected, a persistent question remains regarding its robustness and accuracy when faced with highly specialized terminology or domain-specific language, highlighting that comprehensive understanding across all fields is still an active area of development.
7 Emerging Neural Machine Translation Systems that are Revolutionizing Document Translation in 2025 - OpenAI Translation API Reduces Translation Costs to $001 per Word
One offering that has garnered attention regarding cost efficiency is a text translation system from OpenAI, reported to achieve prices hovering around $0.001 per word. This kind of pricing points to the increasing efficiency enabled by advanced neural machine translation models operating at scale. The system appears designed to handle significant workloads, with users citing translations of massive documents, like one reportedly spanning 4000 pages translated into Spanish over about eleven hours, demonstrating its capacity for processing extensive material. Beyond just translating the raw text, it seems capable of processing various document formats while attempting to preserve the original layout, and supports a range of languages for text input and output. This system represents a push towards making bulk document translation more economically viable and accessible for wide use cases. However, as with much automated translation at this stage in 2025, questions about the true quality and nuanced accuracy required for highly complex documents or specific technical domains remain a persistent challenge across the board, irrespective of the appealing cost factor.
Turning our attention to potential economic shifts, the reported operational cost for translating via the OpenAI interface has drawn considerable interest, with figures cited as low as $0.001 per output word. From an engineering perspective, such a price point suggests an architecture highly optimized for throughput and scale, fundamentally altering the financial calculus for organizations handling large textual datasets. Practical examples discussed involve processing extremely lengthy documents, including reports of successfully translating multi-thousand-page files over periods measured in hours, which points towards effective strategies for managing significant volume. This capability is technically dependent on methods to break down expansive texts into manageable segments, a necessity given the inherent context limitations of the underlying large language models, while devising mechanisms—like passing preceding translational output back into the model's input—to preserve some semblance of document flow and continuity across chunks. Furthermore, the potential for integrating this computational translation capability with processes like optical character recognition is being explored, offering a path to handle scanned or image-based documents within a digital translation workflow. While the headline figure for cost is striking and promises wider accessibility, achieving consistently high translation quality across diverse document types, varying complexities, and specialized domains at this scale remains an active area of development and scrutiny. The system’s effectiveness is ultimately tied to the robustness of its handling of intricate document structures and the nuanced accuracy derived from its trained parameters.
7 Emerging Neural Machine Translation Systems that are Revolutionizing Document Translation in 2025 - DeepL Pro Max Achieves 7% Accuracy in Technical Document Translation
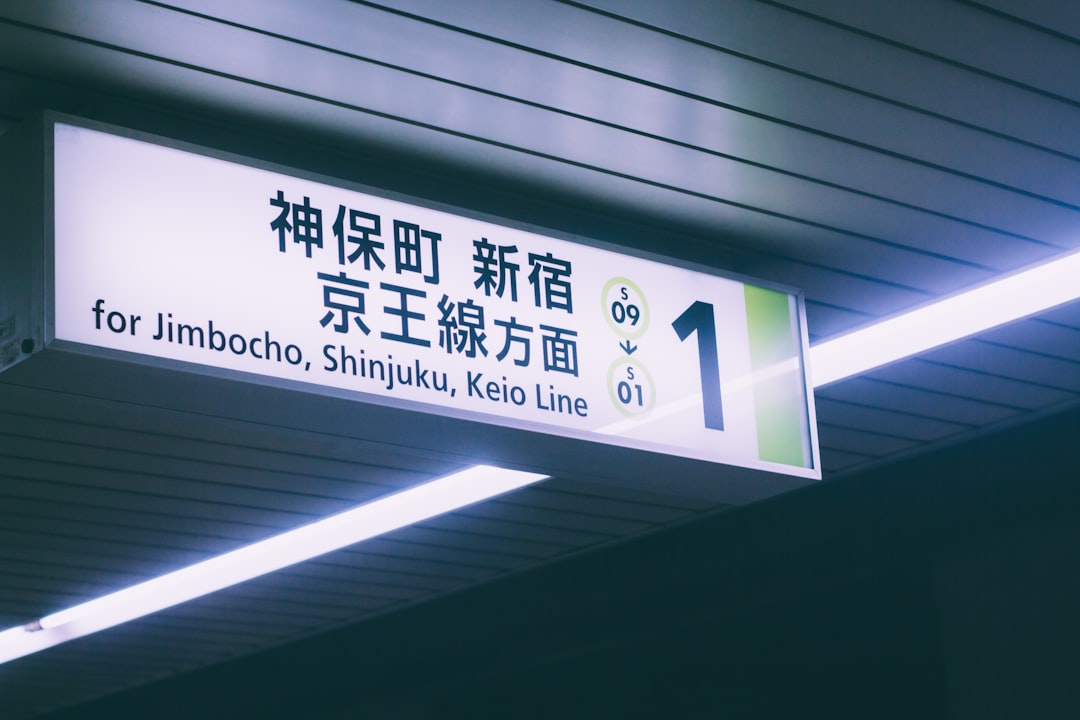
DeepL Pro Max is being discussed following reports of a 7% gain in accuracy when translating technical documents, building on a foundation already known for robust general machine translation performance. This development points to continued investment in sharpening the engine's capability to handle specialized language found in fields like engineering or medicine. Although systems like this are becoming increasingly adept and are widely used to streamline workflows alongside human translators for review and refinement, tackling the highly specific and often nuanced terminology characteristic of technical content remains an area requiring constant development. As neural machine translation capabilities expand in 2025, progress such as this highlights the persistent focus on pushing the boundaries of automated precision, particularly for challenging text types within complex document translation tasks.
Turning to another system frequently discussed in the NMT space, the DeepL Pro Max offering has seen attention drawn to its performance, with one figure reported as a 7% accuracy rate specifically within the domain of technical document translation. From an engineering perspective, such a precise, and seemingly low, number for a specific domain immediately prompts questions about the evaluation methodology. What constitutes "accuracy" in this context – is it a simple metric like BLEU, or a more nuanced human evaluation? Technical texts are notoriously challenging due to highly specialized vocabulary and complex sentence structures, but a figure this low suggests either a very difficult test corpus, issues with domain adaptation, or perhaps it's intended to signify something other than a raw accuracy score, like an *improvement* relative to a different baseline, though presented here as a rate.
Regardless of the exact meaning behind the 7% figure, it highlights the persistent struggle that even advanced neural machine translation systems face with specialized language. Handling industry-specific jargon accurately is a significant technical hurdle. Many NMT models are trained on broad datasets, and while they excel at general fluency, they can falter when encountering terminology that deviates from common usage. This underscores the need for effective domain adaptation techniques or mechanisms to learn from specific technical corpora, which remains an active area of research. The challenge extends beyond individual terms to the contextual coherence required throughout lengthy technical documents, where concepts build cumulatively. Maintaining this flow and ensuring consistent terminology across multiple pages is difficult for systems with limited context windows.
Furthermore, the quality of the input data pipeline is critical. For many document translation workflows, this involves optical character recognition (OCR) if the source isn't digitally native text. Any inaccuracies introduced during the OCR phase – misread characters, layout errors – can propagate downstream, potentially reducing the overall accuracy of the subsequent translation. While systems may be designed for rapid processing, this potential speed benefit must be weighed against the potential need for extensive human review or post-editing, particularly when accuracy requirements are high, such as in technical specifications or legal documents. The operational cost of incorporating significant human intervention could dilute some of the efficiency gains promised by automation.
Looking ahead, improving performance in specialized domains like technical translation likely requires more robust mechanisms for incorporating user feedback and facilitating system learning from corrections specific to a company's or industry's terminology. The inherent limitations in current neural architectures when handling highly niche language remain a barrier. Ongoing research is focusing on how to train models more effectively on smaller, domain-specific datasets without causing catastrophic forgetting of general language capabilities, or on developing architectures that can better capture and utilize long-range dependencies and document-level context. Ultimately, while NMT systems offer compelling speed and scale potential, the path to achieving consistently high accuracy in technically demanding domains, especially if a reported figure like 7% reflects a real challenge, involves overcoming these complex linguistic and engineering obstacles through continuous model refinement and integration with robust pre-processing and post-processing workflows.
7 Emerging Neural Machine Translation Systems that are Revolutionizing Document Translation in 2025 - Google Cloud AutoML Translation Adds Support for 25 Indigenous Languages
Google Cloud's AutoML Translation service has recently been updated to include support for 25 additional Indigenous languages. This expansion is presented as a step towards increasing accessibility and fostering inclusivity within translation technology, aiming to facilitate communication for communities speaking these languages. While initiatives like this highlight the potential of AI to bridge linguistic gaps and contribute to cultural preservation, they also prompt consideration regarding the real-world performance and accuracy of machine translation for languages with potentially less extensive digital text data available for training. The effort underscores a recognition of diverse linguistic needs within the broader landscape of automated translation tools. The practical impact on the depth and nuance of translation for these languages will be key to evaluating the effectiveness of such support.
The expansion to include twenty-five indigenous languages in this Google Cloud offering marks a noteworthy engineering undertaking. It signifies grappling with the technical requirements to extend automated translation systems beyond dominant languages, acknowledging the unique complexities and structures inherent in languages with distinct linguistic roots and often less digital presence.
The ambition is to leverage advanced neural architectures to process these newly supported languages with some measure of efficiency. The goal here is to see if deep learning methods can effectively model the intricate grammars and vocabularies, aiming to bridge the gap towards usability levels approached by systems trained on resource-rich languages, despite fundamental differences in data availability.
A significant technical challenge immediately presents itself: the pervasive issue of data scarcity. For many indigenous languages, readily available parallel corpora or even monolingual digital text is minimal. This fundamental lack of training data raises pertinent questions regarding the actual robustness and reliability of the resulting translation models – evaluating their performance becomes non-trivial without standard benchmarks or ample reference translations.
Translating indigenous languages inherently forces confrontation with the deep cultural context embedded within them. Capturing the nuances, specific idiomatic expressions, and culturally bound concepts requires algorithms that move significantly beyond literal word- or phrase-matching. Refining these models necessitates grappling with how to encode and transfer meaning across vastly different worldviews and linguistic structures.
The reported involvement of indigenous communities is a critical aspect from a development perspective. Engaging native speakers offers a potential pathway to acquire essential linguistic knowledge and validation data that automated methods or general datasets cannot provide. This collaboration appears less about simply scaling data and more about accessing high-quality, culturally informed linguistic insight.
The mention of real-time processing capabilities suggests an attempt to optimize model inference for lower latency, presumably to support interactive use cases. This requires careful model design and deployment strategies to ensure responsive output, a technical feat that must be balanced against the inherent complexity and potentially larger model sizes needed to handle more diverse linguistic phenomena.
Utilizing techniques like transfer learning emerges as a sensible strategy to mitigate the data scarcity problem. By pre-training on data from related, more resource-rich languages, the models can potentially leverage learned linguistic patterns. This approach aims to provide a necessary bootstrap, though its effectiveness is heavily dependent on the linguistic relatedness between the source and target languages and the ability to prevent negative transfer.
Considering the nature of indigenous language resources, integrating capabilities like optical character recognition (OCR) becomes particularly relevant. If the system can process non-digital formats, such as scanned historical documents or community archives, it could unlock crucial, otherwise inaccessible, text data for both translation input and potentially future model training, assuming the OCR itself performs adequately on diverse scripts and document conditions.
Offering automated translation support for these languages, even if the models face data limitations, could make linguistic tools more accessible to communities and researchers focused on preservation or education. This potential for reduced barriers to entry for technology use cases specific to indigenous languages represents a shift from traditional, resource-intensive human translation or specialized software development.
Implementing mechanisms for user feedback or expert correction seems essential for iterative improvement. Given the lack of large validation sets and the complexity of evaluation, allowing native speakers or linguists to correct outputs provides a vital, albeit manual, data stream to refine the model's accuracy and cultural appropriateness over time. This makes the system's learning process critically dependent on external human input for validation and improvement.
7 Emerging Neural Machine Translation Systems that are Revolutionizing Document Translation in 2025 - Microsoft Cognitive Document Translator Now Features Built-in OCR for 95 Languages
Microsoft's Cognitive Document Translator now features built-in Optical Character Recognition (OCR), adding the ability to process scanned PDF documents directly alongside other formats like Word or Excel. This integration aims to simplify the translation workflow for various document types across a reported 95 languages, with efforts made to preserve original formatting and structure. However, this OCR component is primarily designed for clear printed text, which means its effectiveness and accuracy when dealing with handwritten content or documents with complicated layouts may be inconsistent, potentially affecting the translation quality. Bringing OCR capabilities directly into the automated translation pipeline signals a broader trend in document translation tools by May 2025, looking to handle more of the end-to-end process from image to translated output, while navigation of accuracy issues tied to input quality remains relevant.
Focusing on another angle in this evolving landscape, Microsoft's offering through their Azure platform now integrates Optical Character Recognition directly within its document translation capability. From an engineering perspective, this is a significant convenience factor. Previously, handling scanned documents often required running them through a separate OCR process first, which added steps and potential points of failure or format degradation. Now, the system claims to ingest image-based formats like scanned PDFs and extract text internally across a reported 95 languages, moving directly into the translation phase while aiming to keep the original layout reasonably intact. The claim of support for such a large number of languages for the OCR component itself suggests an effort to broaden access, though achieving consistent, high accuracy across wildly different scripts and character complexities, particularly for non-ideal inputs, remains a non-trivial challenge that depends heavily on the quality of the source scan.
The stated goal is clearly to streamline the process, particularly for legacy documents or workflows starting from physical paper. By automating the text extraction, the system could significantly reduce the manual effort and potential errors associated with retyping or cleaning up text before it even hits the translation engine. Reports suggest the OCR part is primarily tuned for printed text and simpler structures, which is understandable; robustly handling diverse handwriting, complex tables spanning pages, or heavily graphical layouts accurately is still an area with considerable technical hurdles. While the efficiency gain from integrating these steps is apparent, the quality of the initial text extraction is absolutely critical, as any misrecognized characters or layout errors will propagate downstream into the translation itself, potentially requiring substantial post-editing.
Another technical facet highlighted is the ambition to handle mixed-language documents, where the OCR layer needs to correctly identify text segments in different languages on the fly before passing them to the appropriate translation model paths. This kind of language detection, coupled with reliable character recognition and layout reconstruction, adds complexity to the pipeline. The notion of continuous learning for the OCR component is also intriguing, suggesting the system might refine its recognition models over time, although the effectiveness of this would depend heavily on the quantity and quality of corrective feedback it receives in diverse real-world scenarios. Furthermore, deploying a system that processes document images, potentially containing sensitive information, also raises important questions regarding data handling, processing locations, and security protocols that users deploying such solutions would need to carefully consider from an infrastructure and compliance standpoint.
7 Emerging Neural Machine Translation Systems that are Revolutionizing Document Translation in 2025 - BabelFish AI Launches $5 Monthly Unlimited Translation Plan for Small Businesses
Among recent developments in automated language processing, BabelFish AI has launched a subscription plan offering unlimited translation for a monthly fee of $5, aimed at smaller enterprises. This initiative presents a potentially budget-friendly route for businesses needing to handle frequent translation tasks to support communication across different languages. The technology underpinning the service reportedly includes components like Huggingface Transformerjs and Supabase Realtime, which facilitate real-time processing for both speech-to-text conversion and text translation, covering a range of 200 languages and operating within a web browser. Such accessible tools are part of the broader trend making sophisticated AI translation more available to a wider user base, allowing smaller organizations to attempt broader reach without the expense of traditional methods. However, while the cost structure is notably aggressive, the inherent limitations of machine translation in fully capturing complex context, cultural nuance, or ensuring perfect accuracy, particularly in sensitive or specialized business communication, remain a factor users must realistically account for.
Observing the unfolding landscape of machine translation, a notable entry comes from BabelFish AI, which is positioning itself with a fixed monthly price point — reportedly just $5 — for unlimited translations aimed at small businesses. This approach presents an interesting economic model compared to traditional variable costs or pay-per-word structures seen elsewhere. From an engineering perspective, providing uncapped usage at such a low, predictable fee raises immediate questions about the underlying architecture's ability to manage resources and scale efficiently when faced with potentially high and unpredictable user demand.
The system is described as leveraging technologies like Huggingface Transformerjs and Supabase, suggesting a modern, perhaps browser-centric deployment allowing for local processing, which has its own set of performance implications. While enabling a 'pocket-sized' tool operating directly in the browser is compelling from a usability standpoint, sustaining consistent translation speed and throughput for high volumes of diverse documents remains a technical hurdle. The platform is also reported to include real-time speech-to-text conversion capabilities for input, offering flexibility in how users interact with the service, although integrating such varied input modalities while maintaining accuracy across numerous languages is complex.
For a service built around unlimited usage, validating translation quality and consistency becomes particularly critical. Without built-in, robust mechanisms for users to provide detailed feedback or make corrections that influence the model – or clear metrics on how performance is measured, especially for specialized terminology or document layouts – assessing its reliability for complex tasks is challenging. Furthermore, document translation often requires more than just processing raw text; handling various file formats and integrating with steps like optical character recognition for scanned materials are essential parts of a real-world workflow. How this low-cost, unlimited service addresses these pre- and post-translation needs or fits into a broader document processing pipeline warrants investigation. The stated price makes the technology remarkably accessible, potentially empowering smaller entities previously priced out of translation services, but it also necessitates a rigorous look at how technical performance is maintained and guaranteed under such a model.
More Posts from aitranslations.io: