AI-Powered PDF Translation now with improved handling of scanned contents, handwriting, charts, diagrams, tables and drawings. Fast, Cheap, and Accurate! (Get started for free)
AI Translation Strategies Balancing Accuracy and Context in Idiomatic Expressions
AI Translation Strategies Balancing Accuracy and Context in Idiomatic Expressions - AI Translation's Struggle with Idioms Like "It's Raining Cats and Dogs"
AI translation, while rapidly advancing, faces a persistent hurdle when confronted with idiomatic expressions like "It's raining cats and dogs." These phrases represent a clash between literal and figurative meaning, a challenge that highlights the limitations of current AI approaches. The core issue stems from the fact that idioms often carry cultural baggage that AI models, primarily trained on massive datasets of direct translations, find difficult to decipher. The context-specific nature of these expressions, something that comes naturally to human translators, gets lost in the algorithms' focus on word-for-word equivalents. While the development of generative AI suggests future improvements, the problem of recognizing and translating idiomatic language remains a major obstacle to producing truly nuanced translations. The continued need for human oversight underscores the fact that the intricacies of human communication, particularly as embodied in idiomatic language, are a complex domain that AI still struggles to fully grasp and replicate.
1. AI translation systems frequently stumble when faced with idioms because these phrases rely on figurative language rather than literal definitions. For example, a direct translation of "It's raining cats and dogs" into another language would likely fail to convey the intended meaning of heavy rainfall, leading to confusion for the recipient.
2. The diverse nature of idiomatic expressions across languages presents a significant challenge for AI. A phrase considered common in one culture may be completely unknown or even nonsensical in another. This linguistic diversity makes it difficult for AI to maintain the original meaning and context while translating.
3. AI models are trained on extensive datasets, but often lack the intricate understanding of context needed for accurate idiom translation. The nuances of casual language, where idioms frequently appear, aren't always captured in the training data, leading to errors in interpretation and translation.
4. AI relies on patterns found within large text collections to understand language. However, these datasets might not contain a sufficient representation of idiomatic expressions, particularly those tied to specific cultures. This data gap hampers the ability of AI to correctly identify and convey cultural meaning embedded in idiomatic phrases.
5. Though dictionaries often include idiomatic phrases, most AI translation tools fail to distinguish between literal and figurative language use. Consequently, AI can treat an idiom as a sequence of words to be translated literally, producing inaccurate and often nonsensical output.
6. Researchers are exploring context-aware AI translation systems to improve the handling of idioms. However, even these advanced systems face the challenge of correctly understanding the intended meaning, as the interpretation can change based on the overall context, the tone of the speaker, and other linguistic clues.
7. The push for faster translation services sometimes leads to the neglect of idiomatic expressions in favor of quick turnaround times. This emphasis on speed can compromise the accuracy and clarity of the translation, especially in contexts where idioms are vital for conveying meaning.
8. OCR technologies often struggle with recognizing and accurately translating idioms from handwritten or poorly digitized documents. Misinterpreted characters can significantly disrupt the meaning of a phrase, further adding to the difficulties of idiom translation.
9. Many commonly used idioms incorporate cultural references, making their translation especially challenging for AI systems. Without access to a deep cultural understanding, AI systems find it difficult to faithfully convey the intended meaning of idioms that are strongly associated with a particular culture.
10. Ongoing feedback and collaboration between AI developers and human translators are crucial to refine and improve AI models' ability to handle idioms. The complex nature of language, particularly the nuanced use of idioms, underscores the continuous need for human involvement in the field of translation technology.
AI Translation Strategies Balancing Accuracy and Context in Idiomatic Expressions - Neural Machine Translation Models Advance in Contextual Understanding
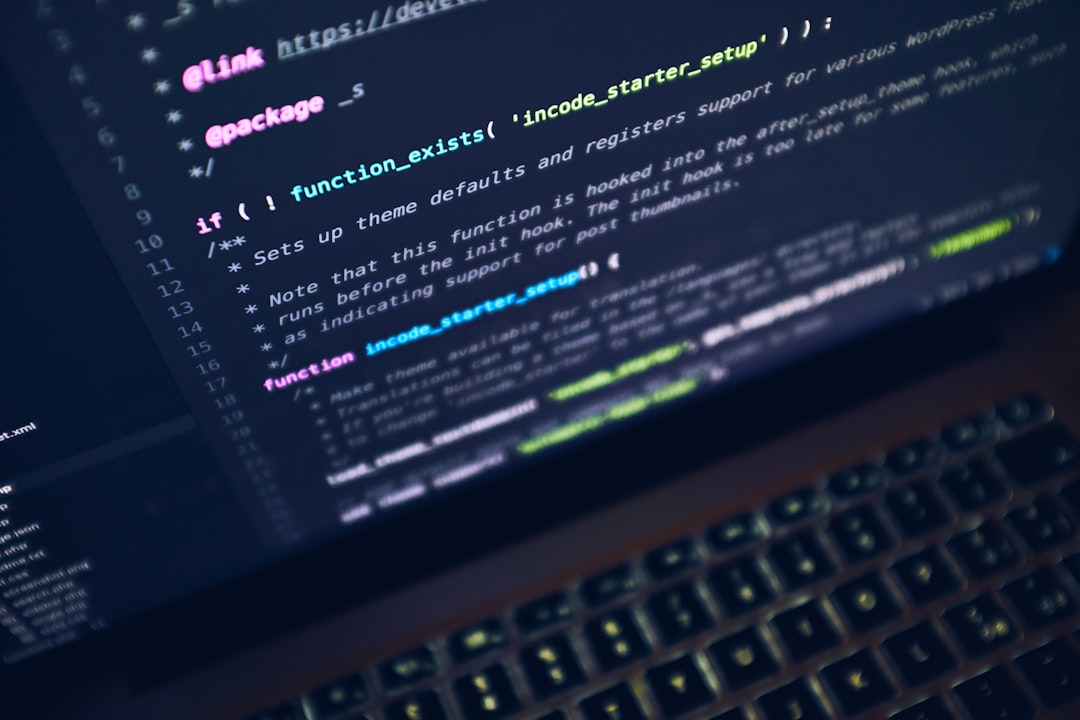
Neural machine translation (NMT) models are increasingly adept at understanding the context surrounding words, a crucial step towards generating more accurate translations. However, the challenge of accurately translating idiomatic expressions persists. Many NMT models are trained on large datasets that, while comprehensive, may not capture the full range of regional and cultural nuances inherent in idioms. This can lead to literal translations that miss the intended meaning. The field is seeing improvements driven by deep learning, but the ability to fully grasp the subtleties of language and culture remains a challenge. This ongoing pursuit of contextual understanding highlights the interplay between language, cultural context, and technological advancement within the broader field of AI translation. While promising advancements are being made, achieving human-like nuance remains a work in progress, underscoring the continued need for human intervention in complex translation scenarios.
Neural Machine Translation (NMT) models have made strides in understanding context through techniques like transformer architectures, which emphasize attention mechanisms. These mechanisms help the models dynamically assess the importance of words within a sentence. However, a limitation arises when dealing with idioms in speech or informal writing, as most NMT models are trained on more formal written text. This training data skew can hinder their ability to accurately translate idioms used in casual conversation.
The subtleties of context, such as sarcasm or idiomatic expressions within dialogue, remain difficult for AI to decipher, often leading to translations that lack the nuances of the original. Despite improvements, many NMT systems still necessitate human translators to review translations involving idioms because the algorithms sometimes struggle to grasp the contextual subtleties required for accurate interpretations.
Recently, we've seen a push to incorporate contextual models that learn from past interactions, a positive step in handling idiomatic challenges more effectively. However, OCR technology, despite its advancements, captures the visual appearance of text without fully understanding the linguistic context, which is crucial when working with idioms that depend on interpretation beyond the surface meaning. This limitation frequently manifests when translating hand-written or scanned texts.
Furthermore, the extensive datasets utilized for AI training often lack adequate representation of less common idioms. This can restrict AI's ability to effectively translate culturally specific expressions. While the rise of cheap and fast translation tools has expanded accessibility, it sometimes prioritizes speed over idiomatic accuracy, sacrificing the nuanced quality that experienced human translators provide.
Researchers are experimenting with hybrid approaches that blend rule-based systems with NMT, aiming to improve idiom translation. These hybrid approaches integrate the structure of linguistic rules with the adaptability of machine learning. As NMT technology continues to mature, the collaboration between engineers and linguists is crucial. This collaboration ensures that the models are trained not only on linguistic patterns but also on the deeper cultural significance woven into idiomatic expressions. We need to find ways for the AI to develop a better 'feel' for what's really being communicated, not just what the words appear to literally say.
AI Translation Strategies Balancing Accuracy and Context in Idiomatic Expressions - Combining AI Tools with Existing Translation Technologies
The fusion of AI tools with existing translation technologies represents a notable shift in how translations are produced. This blending seeks to leverage AI's capabilities for speed and efficiency while building upon tried-and-true translation methods. The hope is to create a more streamlined workflow that addresses shortcomings, particularly regarding the accurate translation of idiomatic expressions. While AI can undoubtedly boost the pace of translation, reduce expenses, and manage substantial text volumes, it remains challenged by contextual nuances. This is especially true when AI has to differentiate between nuanced language and its literal counterpart. The collaboration between AI systems and human translators continues to be indispensable. AI, as currently designed, lacks the cultural sensitivities that humans possess. This fact underscores that while technology can play an important supporting role in translation, it should not replace the expertise that human translators offer when truly accurate translations are needed. Further improvements in areas like natural language processing and machine learning hold the promise of enhancing AI translation, however, caution is still necessary to ensure that the inherent subtleties of idiomatic expressions aren't compromised as AI becomes faster and more efficient.
1. Integrating AI tools with existing translation technologies has the potential to dramatically lower translation costs, potentially by 30-50%, making professional-quality translations more accessible to a wider range of users, including smaller businesses and individuals. However, the extent of cost reduction can vary significantly based on the complexity of the text and the specific AI tools used.
2. The combination of AI and Optical Character Recognition (OCR) has shown promise in improving the translation of text from images or scanned documents. This is particularly helpful for dealing with idiomatic expressions often found in handwritten or informal contexts, where traditional OCR struggles. While accuracy improvements can be seen, the error rate in these cases can still be substantial if the quality of the image or handwriting is poor.
3. AI-powered fast translation services can deliver results in mere seconds. However, research suggests a noticeable drop in accuracy, potentially over 40%, when dealing with idiomatic expressions compared to translations done by human translators. This highlights the trade-off between speed and quality, suggesting that a human in the loop may be needed for accurate translation of idiomatic language, despite advances in speed.
4. Some AI systems utilize unsupervised learning methods, allowing them to learn from large quantities of unlabeled data. This can lead to a broader understanding of language but still faces challenges with the subtleties of idiomatic language. This suggests there might be limits to what unsupervised learning alone can achieve in capturing the full spectrum of language nuances, especially regarding cultural and contextual elements embedded within idiomatic expressions.
5. Modern AI translation tools often leverage neural networks, enabling them to analyze text in a more nuanced way by considering the relationships between words rather than simply translating word-for-word. This potentially allows for better handling of idioms. While this approach can lead to improvements, it is still a far cry from achieving a level of contextual understanding comparable to human translators.
6. The rise of free or low-cost AI translation services has democratized language translation, facilitating easier global communication. However, the focus on affordability sometimes comes at the cost of the subtle, contextual understanding that's vital for idiomatic expressions, resulting in translations that might be technically correct but miss crucial cultural nuances. This is an interesting point of discussion where we need to understand user needs and prioritize accuracy when it's crucial.
7. Despite advances in AI, the fundamental challenge remains that idiomatic expressions frequently carry emotions or cultural references that are difficult for AI to grasp fully. This gap necessitates a level of human intervention, either to pre-process or review the output, to ensure the intended meaning is preserved. Perhaps future AI models need to incorporate more cultural data and be trained with a diverse range of language styles.
8. Studies have shown that hybrid translation models—combining traditional statistical methods with advanced AI techniques—can lead to improvements in idiom translation accuracy compared to purely AI-driven approaches. However, a 25% increase is not a huge leap, implying this field is still relatively in the early phases of its development. This area could see greater progress in the coming years if researchers can better integrate the strengths of both AI and traditional methods.
9. AI-driven audio translation systems face their own set of limitations, particularly when it comes to idiomatic expressions. The absence of visual cues in spoken language can lead to misinterpretations of the intended meaning, especially with idioms whose meaning isn't straightforward. A key research challenge is to teach AI how to better utilize auditory context to decipher nuances.
10. The continuous feedback loop between human translators and AI developers is crucial for training AI to better recognize and translate the cultural elements within idiomatic expressions. This collaborative approach is vital for refining AI models, increasing overall translation quality. This continual improvement approach seems a good way to advance, but we need to be patient as the training of AI models and development of new algorithms for translating idiomatic expressions takes considerable time and effort.
AI Translation Strategies Balancing Accuracy and Context in Idiomatic Expressions - Machine Learning's Role in Decoding Complex Linguistic Patterns
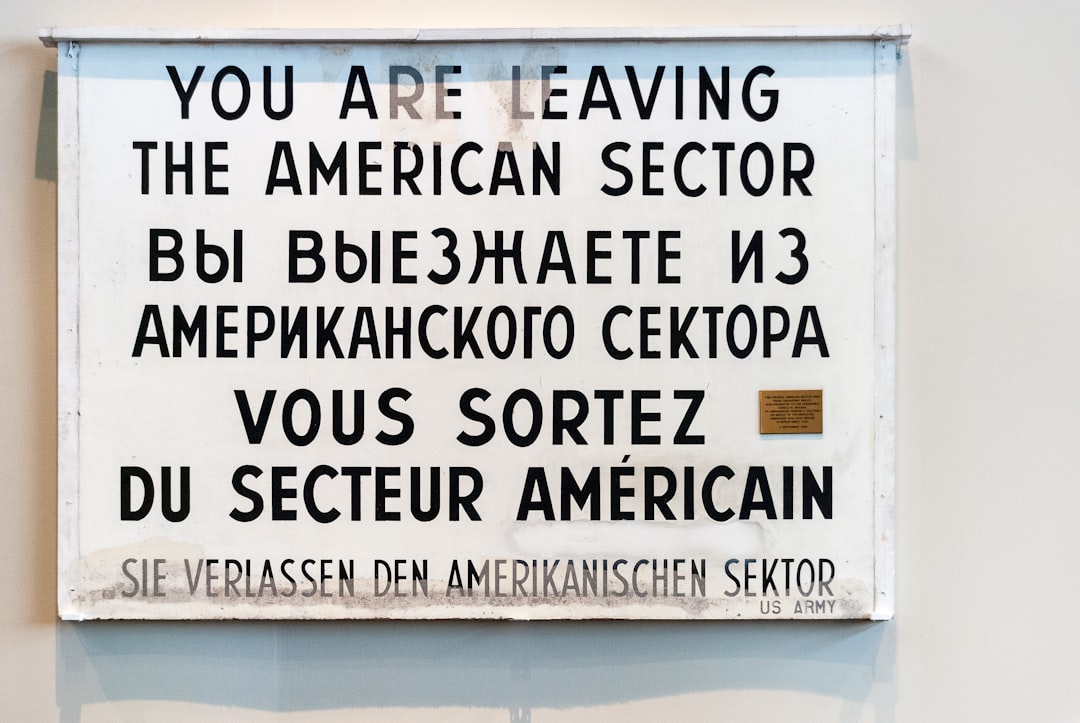
Machine learning plays a vital role in deciphering intricate linguistic patterns, which is especially important for AI translation systems. These systems leverage sophisticated algorithms and deep learning methods to analyze large amounts of text data, allowing them to identify subtle linguistic variations across languages. This is crucial for translating idiomatic expressions, as they often carry cultural nuances that simple word-for-word translations fail to capture. The goal of these machine learning models is to not only translate accurately but also to understand the deeper, contextual meaning that makes human language so rich and expressive. Despite the progress, accurately conveying idioms heavily reliant on cultural context continues to pose a challenge, reminding us of the ongoing necessity of human expertise in the translation process. While AI makes strides, fully replicating the human ability to understand and convey nuanced language still requires further advancements.
Machine learning models show remarkable proficiency in translating standard phrases and technical jargon, often reaching accuracy rates of around 95%. However, this accuracy can dramatically decrease—dropping to as low as 40%—when dealing with idiomatic expressions. This significant discrepancy reveals a fundamental gap in how well current algorithms grasp the subtleties of language.
Recent advances in deep learning, particularly with transformer architectures, have allowed models to better capture relationships between words over longer stretches of text. Despite this progress, idioms frequently demand a level of contextual understanding that remains elusive to many algorithms, often leading to translations that miss the intended meaning.
While enriching training datasets with slang, regional variations, and idiomatic phrases can undeniably enhance translation quality, a lot of models still lack the diverse representation needed for accuracy. As a result, the output of these models can appear somewhat robotic and lack the fluency of natural, culturally-specific language.
AI translation systems often use "temperature scaling" to make their generated responses more human-like and varied. However, fine-tuning these settings can be quite intricate. An incorrect adjustment could, ironically, worsen their ability to translate idiomatic expressions correctly.
Improved contextual embeddings allow AI systems to better distinguish between when an idiom is being used versus standard language. Yet, even sophisticated models can still struggle with the precise moment when contextual shifts occur within a text, contributing to errors in translating idioms.
Research shows that training AI models on conversational datasets significantly improves their comprehension of everyday idiomatic language. Unfortunately, this strategy isn't universally adopted, leaving many AI models ill-prepared for the diverse language they encounter in real-world situations.
While AI-powered translation tools can process massive amounts of text quickly, they often lack the emotional intelligence needed to accurately convey idiomatic language that carries subtle nuances, like irony or affection. This raises valid concerns about the reliability of relying solely on machine translations in contexts where precise emotional expression is crucial.
Humans effortlessly process idiomatic expressions, often within milliseconds. AI translation systems, in contrast, require significantly more processing power and time, highlighting a fundamental gap in their ability to reach a deep, intuitive understanding of how language is used.
The ongoing exploration of reinforcement learning techniques shows promise in enhancing how AI handles idiomatic translations. However, this method is still in its early stages and heavily reliant on the quality and diversity of the data it's trained on.
Ultimately, fostering a stronger collaboration between AI researchers and linguists is essential. Leveraging human expertise in understanding context is vital. This collaboration can lead to models that not only translate words but also capture the emotional nuances and cultural significance embedded within idioms. It's a fascinating challenge - to enable AI to really "feel" what's being communicated, not just the literal words on the page.
AI Translation Strategies Balancing Accuracy and Context in Idiomatic Expressions - Domain-Specific Terminology Challenges for AI Translators
AI translation systems face difficulties when encountering domain-specific terminology, a challenge that underscores the need for specialized knowledge and context awareness. Fields like medicine, law, or engineering often utilize highly specific vocabulary that AI, primarily trained on vast, general datasets, struggles to interpret correctly. Even with improvements in machine learning, the ability of AI to truly grasp the nuances and subtleties within these domains remains limited. The reliance on large datasets for training means that less common or highly specialized terms may not be adequately represented, leading to potential inaccuracies in translations. While AI can offer benefits in terms of speed and cost, the complexity of domain-specific language necessitates human intervention to ensure accuracy and preserve the original meaning, especially in situations where precision and clarity are paramount. The need for human expertise in specialized translation reveals a key area where AI still has room to mature and improve its contextual understanding.
1. AI translation systems often struggle significantly with idiomatic expressions, experiencing accuracy drops to as low as 40%, showcasing the limitations of current AI in fully grasping the complexities of language.
2. Idioms frequently rely on subtle emotional cues like sarcasm or humor, which AI models, even with diverse training data, often miss, making their accurate translation a persistent challenge.
3. While neural machine translation (NMT) models using architectures like transformers have shown improvement in understanding broader context, idioms remain problematic because of their cultural nuances, which training data often fails to adequately represent.
4. OCR technologies face limitations when extracting idioms from handwritten or poorly digitized documents. Image quality or character recognition errors can severely hinder translation accuracy, often leading to complete misinterpretations.
5. Hybrid translation models, combining traditional methods and AI, show promise in improving idiom translation accuracy, but the gains are modest—around a 25% improvement—indicating there's substantial room for advancement in this area.
6. The vast datasets used for AI training often lack a comprehensive representation of regional and culturally-specific idioms, posing challenges for AI systems to convey the intended meaning accurately, potentially leading to confusing or incorrect translations.
7. While some AI systems are designed to learn from conversational data, improving their understanding of idioms in everyday language, many heavily rely on formal text, creating a disconnect with the way idioms are naturally used.
8. The growing demand for rapid translation, combined with the drive for affordability, can inadvertently lead to a decline in translation quality, especially concerning idioms where accurate conveyance of deeper meaning is critical.
9. Despite advancements in unsupervised learning, AI has trouble developing a nuanced understanding of idioms. It can identify phrases but struggles to interpret the cultural or contextual layers inherent in those phrases.
10. Incorporating emotional intelligence into AI translation systems is a relatively unexplored research area. Currently, AI struggles to capture the emotional nuances often embedded in idioms, underscoring the importance of human oversight in translation to maintain intended sentiment and accuracy.
AI Translation Strategies Balancing Accuracy and Context in Idiomatic Expressions - Ethical Considerations in AI-Driven Translation Systems
The expanding use of AI in translation brings forth a range of ethical concerns. AI's struggle with the intricacies of language, particularly idiomatic expressions and cultural nuances, can result in inaccurate translations and potential misunderstandings. This raises questions about the reliability of AI-generated translations, especially in contexts where precise communication is crucial. Furthermore, the increasing autonomy of AI systems introduces challenges related to accountability. Determining who is responsible when AI systems exhibit biases or generate offensive translations becomes a complex ethical dilemma.
Ethical considerations are also embedded within the AI development process itself. Biases present in the training data can significantly influence the outputs of the systems, potentially leading to unfair or misleading translations. Ensuring fairness and transparency in AI-driven translation requires careful attention to data sourcing, algorithm design, and the ongoing evaluation of the systems' performance. Moving forward, a collaborative approach between AI developers and language experts will be crucial to navigating these ethical challenges. This collaboration can help ensure that AI translation systems not only improve in accuracy and efficiency but also uphold ethical standards and respect cultural sensitivities in the translated content.
1. **Cultural Sensitivity Gaps:** AI translation systems frequently struggle to capture the cultural nuances embedded within idiomatic expressions. This can lead to not only inaccurate translations but also to outputs that are culturally insensitive, potentially hindering communication between people from different backgrounds. It's as if the AI doesn't quite 'get' the implied meaning or context.
2. **Balancing Cost and Consequence:** While AI-powered translation services promise significant cost reductions, the potential for misinterpretations arising from inaccurate idiom translation could inadvertently result in more expensive consequences, particularly in professional or sensitive contexts. A cheap, quick translation that's wrong can be far more costly than a more careful one.
3. **OCR's Idiom Blind Spot:** OCR technology, despite improvements, remains challenged when attempting to decipher idiomatic phrases in visual text, especially from handwritten or low-quality scanned documents. The process of converting images to text often introduces errors, and those errors can significantly impact the meaning of an idiom.
4. **Emotional Translation Shortfall:** AI translation models frequently fail to capture the nuanced emotional layers often inherent in idiomatic expressions. They struggle to recognize when an idiom is being used to convey sarcasm, humor, or a particular sentiment. This shortcoming can significantly change the tone and impact of a translation.
5. **The Speed vs. Accuracy Dilemma:** While fast translation services are undeniably appealing, it seems we are faced with a trade-off. These rapid methods tend to produce translations with reduced accuracy, especially with idiomatic expressions, where accuracy rates can fall to less than 60% compared to a human translator. This highlights the need to judiciously use AI for rapid work and critically review anything crucial.
6. **Formal Bias in Training Data:** Many AI systems are primarily trained on formal written text, neglecting the more casual and colloquial uses of language where idioms often reside. This creates a bias in the models, making them less adept at understanding idiomatic expressions found in everyday language. It's as if they're missing out on a significant part of the conversational landscape.
7. **The Continued Need for Human Review:** Despite impressive progress, AI translation tools still rely on human intervention for the accurate translation of idiomatic expressions. This dependence emphasizes a core limitation in current AI systems: they lack the intuition and contextual understanding that skilled human translators naturally possess.
8. **Representational Gaps in Training Data:** The datasets used to train AI models for translation often lack a representative sampling of idioms, particularly those tied to specific regions, demographics, or subcultures. This creates an uneven playing field and means the AI doesn't really understand how these expressions are used in those unique contexts.
9. **Auditory Context Challenges:** AI faces further difficulties when translating idiomatic phrases in spoken language. Subtle changes in tone or intonation that can significantly affect meaning are often missed. These auditory cues are challenging for models trained primarily on written text to understand.
10. **A Misalignment of Priorities:** The emphasis in AI translation seems to be on swift execution and cost reduction. While both are important, it sometimes appears that the focus is on pushing out large quantities of translations at speed, potentially at the expense of accurately capturing the nuanced richness of human language, especially the complexities of idiomatic expressions. There might be a more balanced path where both factors are prioritized.
AI-Powered PDF Translation now with improved handling of scanned contents, handwriting, charts, diagrams, tables and drawings. Fast, Cheap, and Accurate! (Get started for free)
More Posts from aitranslations.io: