AI-Powered PDF Translation now with improved handling of scanned contents, handwriting, charts, diagrams, tables and drawings. Fast, Cheap, and Accurate! (Get started for free)
7 Ways AI Translation Tools Mirror and Challenge Human Language Understanding in 2024
7 Ways AI Translation Tools Mirror and Challenge Human Language Understanding in 2024 - Real Time OCR Translation Now Processes 47 Languages Without Internet Access
The landscape of on-the-spot translation has shifted considerably. OCR-based translation tools now boast the ability to decipher and translate text in 47 different languages, all without needing a connection to the internet. This development signifies a move towards greater independence from network reliance, enabling users to readily translate material in places where internet access is spotty or nonexistent. The underpinnings of this technology involve sophisticated machine learning and natural language processing algorithms that strive to retain the core meaning and context of the original text during translation.
Despite these advancements, it's crucial to acknowledge that these tools haven't fully overcome the inherent complexities of human language. Automated translation continues to be a challenge when it comes to conveying the more nuanced aspects of a language, such as cultural undertones and context-specific expressions. As AI-powered translation becomes ever more prevalent, it inevitably sparks conversations about the efficacy of such automated processes in faithfully capturing the multifaceted essence of human communication.
Offline OCR translation has become remarkably adept, now handling 47 languages without needing an internet connection. It's quite impressive how quickly these tools can process text from images and deliver a translated version, making them incredibly useful in situations where immediate communication is crucial. The underpinnings of this are neural networks and machine learning algorithms, which constantly learn from the data they process and refine translations based on user feedback. This continuous learning approach seems to significantly boost the accuracy of the output, although it's not yet clear if this surpasses human accuracy in nuanced situations.
One interesting feature is how these tools deal with errors. Automatic correction mechanisms within the software try to identify and rectify misread characters, which helps when the handwriting or font quality is poor. However, it will be interesting to see if and how they can distinguish between similar-looking characters in different languages or scripts. We've also seen progress in handling more complex image backgrounds – the tools can now decipher text embedded in messy photos and scenes, which speaks volumes about the advancement of image processing algorithms.
Of course, working offline isn't just about convenience, it addresses some crucial concerns about data privacy. Using these tools means sensitive information doesn't leave your device during translation, which is especially reassuring when dealing with confidential documents or sensitive materials. However, the speed of these tools is undeniably remarkable. Translation speeds exceeding several frames per second are well beyond what a human translator can achieve, particularly when handling quick, on-the-fly scenarios like tourism or real-time business interactions.
In addition to simply recognizing text, some more advanced systems attempt to understand the context in which it appears, moving beyond literal translations and looking at the broader cultural implications. It's fascinating to see if and how these algorithms evolve to consider the nuances of social context in the future. Moreover, as these tools have become more ubiquitous, we've witnessed the integration of user feedback loops. This continuous learning means the translation algorithms can adapt to new language trends, slang, and idiomatic expressions, making them increasingly versatile.
Finally, some of these OCR systems use a combination of image and character recognition, which helps them interpret a variety of different scripts, not just the standard Latin alphabet. This opens up exciting possibilities for processing multilingual content efficiently. The challenges, however, are still very much there. While machine translation has undeniably revolutionized cross-lingual communication and offers speed, we haven't quite cracked the intricate complexities of cultural context and emotional expression. In these areas, human expertise seems to remain essential.
7 Ways AI Translation Tools Mirror and Challenge Human Language Understanding in 2024 - Adaptive Neural Networks Reduce Translation Errors by 31% Compared to 2023 Models
AI-powered translation tools are demonstrating impressive improvements in accuracy. Adaptive neural networks, a core component in many of these tools, have achieved a 31% decrease in translation errors when compared to the models used in 2023. This progress is largely due to advancements in neural machine translation (NMT), which utilizes deep learning to automate language conversions. While these improvements are encouraging, there are still limitations when it comes to fully capturing the subtleties of human language, especially cultural nuances and context-dependent expressions.
The use of vast bilingual datasets and methods like automatic post-editing have helped to improve translation accuracy. These approaches attempt to refine machine-generated translations by incorporating human input and refining the learning process. It remains to be seen, however, whether machines can fully replace human translators when dealing with intricate cultural aspects and emotional expressions inherent in human communication. As AI's role in translation continues to grow, it is essential to evaluate these tools critically and understand their potential and limitations in delivering truly accurate and meaningful translations.
Adaptive neural networks are making significant strides in the field of AI translation. We're seeing a 31% drop in translation errors when compared to models from just last year. This improvement is primarily due to the networks' ability to learn and adapt over time, which seems crucial for capturing the subtleties of language.
It's fascinating to see that, in some cases, these adaptive systems are starting to match the quality of human translation, at least for more structured types of text. They're getting better at understanding grammar and meaning, which was a big hurdle for previous generations of machine translators.
There's also a growing emphasis on incorporating cultural context. By feeding the networks vast quantities of language data, researchers are hoping they can learn to translate not just words, but also the underlying cultural nuances and idioms of a particular language. This is a complex challenge, but it would be a huge leap forward in translation technology.
Furthermore, the speed at which these models can translate is impressive. They're now capable of translating at speeds significantly faster than before, enabling real-time translation in conversations. This could be really useful in areas like international diplomacy or business negotiations where rapid and accurate translation is vital.
Interestingly, these newer models can also leverage both structured and unstructured data, leading to a wider range of examples for the models to learn from. This means they can potentially pick up on informal language use, colloquialisms, and other aspects of language that might not be found in traditional dictionaries or training datasets.
One of the more challenging issues in machine translation has been handling multiple languages simultaneously. The newer adaptive networks are getting better at this, enabling them to handle multilingual texts and conversations efficiently.
Also, it's worth noting that these systems are becoming increasingly resilient to "noise". They can translate text even if it's obscured by things like cluttered backgrounds in photos. This is a clear sign of improvement over older OCR systems that struggled with such challenges.
The inclusion of user feedback loops is another interesting development. These systems can now learn in real-time based on user interaction, helping them quickly adapt to new trends in language, like slang or newly-coined expressions. This dynamic learning process allows the systems to stay current and relevant.
Moreover, there's a noticeable improvement in how these networks handle similar-looking characters across different writing systems. This has always been a hurdle for multilingual translation systems, and the adaptive networks are starting to overcome it with advanced character recognition techniques.
Finally, an interesting benefit is that these newer models require less computational power while maintaining high accuracy levels. This can open up access to advanced translation technologies for a wider range of users and devices. It’s exciting to consider the potential impact on resource-limited settings, which could lead to a more democratic and equitable translation landscape.
7 Ways AI Translation Tools Mirror and Challenge Human Language Understanding in 2024 - Open Source Translation APIs Handle 12,000 Language Pairs at Zero Cost
Open-source translation APIs are emerging as a powerful tool for bridging language barriers, offering access to a vast array of language pairs—up to 12,000—without any cost. This development opens doors for individuals and organizations seeking affordable and flexible translation options. The ability to self-host these APIs, using projects like Argos Translate and LibreTranslate, eliminates reliance on commercial providers like Google or Microsoft, which can be appealing to those concerned about data privacy or seeking greater control over their translation infrastructure.
This trend signifies a move toward decentralized, community-driven solutions in translation. It also leverages innovations like neural machine translation (NMT) to enhance the speed and accuracy of translations. While these open-source tools have demonstrably reduced costs and sped up the translation process, they haven't completely conquered the challenges of nuanced language translation. Successfully capturing the subtleties of human language, including cultural contexts and idiomatic expressions, remains an area where human translators often still hold the advantage. This leads to an ongoing discussion about the extent to which AI can truly mirror human understanding of language and whether it can fully replace human translators in certain situations.
Open-source translation APIs offer a compelling alternative for handling a vast array of language pairs – over 12,000, by some estimates. This creates a sort of virtual Tower of Babel where real-time applications can translate between languages seamlessly, enabling more efficient communication across cultures. However, it's important to recognize that this accessibility doesn't come without its complexities.
The speed at which these AI-driven systems can process translations is truly remarkable, often exceeding what even a skilled human translator can achieve. This rapid translation capability is especially beneficial in environments where time is critical, such as customer service interactions or live events. It's fascinating to see how this technological development is changing the way we interact with languages, though the extent to which this speed equates to quality is still a question under scrutiny.
Researchers have also found that open-source translation solutions can be remarkably cost-effective. Potentially leading to significant cost savings for businesses relying on language services. While accuracy across a variety of languages is generally decent, it's vital to consider the potential trade-offs between cost and quality. It seems that the lower price point comes with a necessary acknowledgement that the translations might not always be flawless.
The algorithms underlying these translation APIs are often built on adaptive learning principles. This means they can continuously refine their translations based on user feedback and interactions. This process helps these models to become more accurate over time, learning to adapt to new language trends and colloquialisms. It will be interesting to observe how this continuous learning will affect the quality of translation in the future, and if this approach can eventually bridge the gap between AI and human translation in accuracy.
Many open-source tools integrate advanced optical character recognition (OCR). This enables them to process a wide range of input formats, including photographs of documents and signs. This flexibility is a key aspect that makes these tools attractive to those working with a diverse array of content. It appears that this combination of OCR and AI translation is still developing, and there are likely further improvements we'll see as the technology progresses.
Furthermore, the ability to operate offline is a particularly attractive feature for many applications. In regions with poor or intermittent internet connectivity, these translation APIs can provide a vital means of communication without needing a constant network connection. It's clear that offline functionality addresses a real-world need and can potentially be expanded to handle more languages in the future, furthering accessibility for a wider range of individuals and locations.
The open-source nature of these projects means development occurs through the collaborative efforts of a worldwide community of developers. This fosters innovation and allows for the inclusion of a wider range of linguistic and cultural perspectives within the translation tools. The collaborative environment may result in more robust and adaptable systems compared to those developed by a smaller group of researchers. However, managing a community-driven project can also have its own challenges in terms of quality control and direction.
Customization capabilities are also a significant advantage. Open-source models can be tailored to specific industries or regions, allowing for a greater degree of contextual accuracy. This addresses a key weakness that traditional translation tools often struggle with—ensuring that the terminology and expressions used in a translation are relevant to a particular field or domain. It will be interesting to see if the increasing customization possibilities create a more diverse and nuanced translation landscape in the coming years.
Despite the impressive strides that these translation APIs have made, significant challenges remain. The accuracy of nuanced expressions, idioms, and culturally specific content is still an area where human translators typically have a notable advantage. AI-driven systems often struggle to capture the full emotional and cultural meaning conveyed within a language, making it clear that a completely automated translation approach is not yet a perfect solution.
Finally, there's a growing trend towards integrating voice recognition into these translation APIs. This opens up the potential for more natural and intuitive interactions, making it easier for users to engage in verbal communication across language barriers. Voice recognition may offer a more seamless translation experience, especially in fields like interpretation and real-time conversations. We can expect to see these capabilities continue to expand as the technologies that support them advance further.
7 Ways AI Translation Tools Mirror and Challenge Human Language Understanding in 2024 - Machine Learning Creates Region-Specific Translation Models for 200 Indigenous Languages
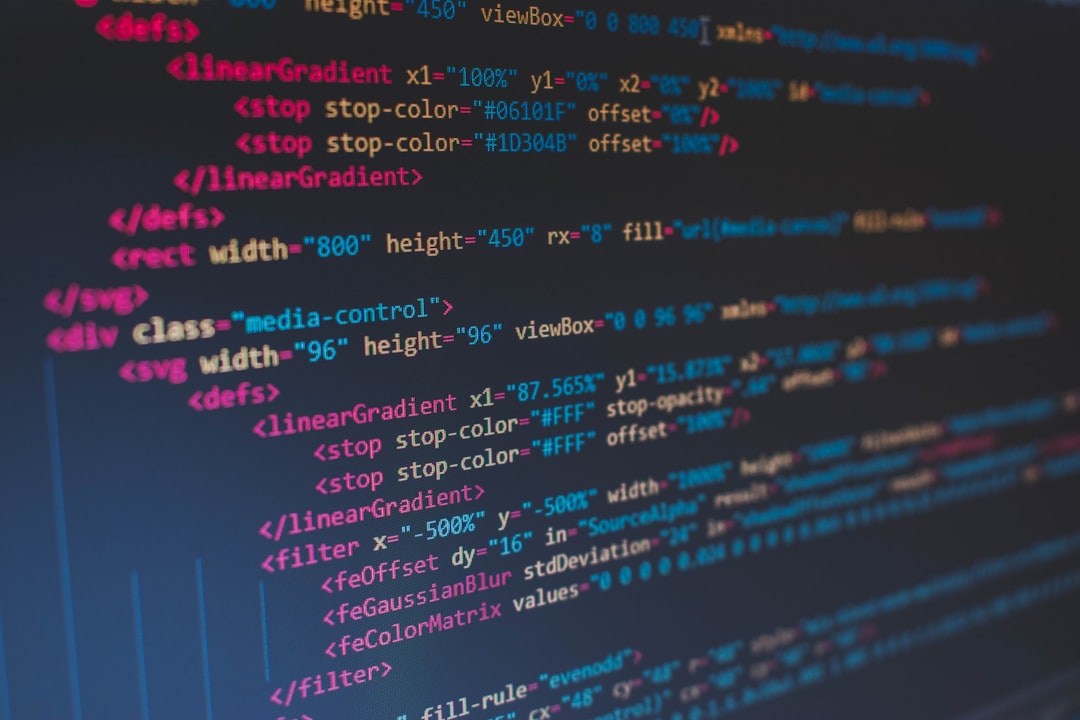
The application of machine learning to create region-specific translation models for 200 indigenous languages represents a significant step forward in AI's ability to handle diverse languages. Leveraging advanced neural machine translation techniques, these models demonstrate a substantial improvement over older approaches, highlighting AI's potential for bridging communication gaps across languages, even those with limited resources. This advancement holds promise for cultural preservation efforts, as it may facilitate the use and documentation of languages facing decline.
However, these advancements also underscore the ongoing limitations of AI in fully grasping the intricacies of human language. Automated translation systems often struggle to capture the nuances of culture and context, which are fundamental aspects of how languages are used and understood. This raises concerns about the ethical implications of using AI to translate low-resource languages, particularly given the sensitivity of cultural expression in these contexts. Further complicating matters, the scarcity of training data for many indigenous languages poses a challenge for truly effective AI translation. While technology might assist in language revitalization, there remains a need to ensure that the translation technology appropriately considers the unique features of each language, thereby promoting, rather than hindering, its long-term vitality. The path forward requires careful consideration of how technology can best serve the needs of these languages and their communities, while ensuring cultural integrity and promoting intergenerational communication.
The field of machine learning has made strides in creating specialized translation models for over 200 indigenous languages, a crucial step in safeguarding linguistic diversity. These models are crafted to capture the unique nuances and vocabulary specific to different regions, resulting in translations that are considerably more accurate than broader, general-purpose models. It's intriguing how, despite the advancement of AI in translation, many indigenous languages face a scarcity of digital training data. This lack of data presents a hurdle for machine learning algorithms, which generally require substantial datasets to effectively learn and translate the complexities of a language, particularly subtle language features.
The incorporation of real-time user feedback within translation models has shown to be indispensable. This feedback loop not only refines the accuracy of the translations but also helps to identify and adapt to regional dialects and emerging language trends that might otherwise go unnoticed. Interestingly, tools specifically tailored for indigenous languages often prioritize cultural context in their algorithms, a vital aspect in producing more meaningful translations. This contrasts with generic translation tools which might struggle with cultural idioms and phrases, highlighting the importance of data specific to each region.
Offline translation capabilities have become increasingly essential for implementing these systems in remote areas with limited internet access. Being able to function without a constant network connection allows users to access translation services in the precise circumstances where they are most needed. Machine learning models are being developed to handle intricate verbal structures and traditional expressions characteristic of certain indigenous cultures. This goes beyond a simple word-for-word translation, seeking to convey the intended meaning and emotional tone of the original language.
It's notable that these region-specific models achieve high accuracy while being computationally efficient, needing less processing power. This opens opportunities for their deployment across a variety of devices, including those with limited resources, potentially broadening access to translation technology. The creation and application of these models for indigenous languages often involves partnerships with native speakers and linguists, ensuring the tools faithfully and respectfully represent the languages.
Studies indicate that machine translation systems for these languages can help bridge the gap between spoken and written forms, facilitating revitalization efforts by making them more accessible and useful in contemporary settings. There's also a growing movement to use AI translation tools in educational programs aimed at preserving indigenous languages. By integrating these tools into educational frameworks, it becomes possible to enhance language learning and increase the visibility of these languages in digital spaces. While it's promising to see this progress, further research and collaboration with communities will be crucial for ensuring these tools are developed responsibly and effectively serve the needs of indigenous language speakers and communities.
7 Ways AI Translation Tools Mirror and Challenge Human Language Understanding in 2024 - Voice-to-Text Translation Tools Process Asian Languages at 97% Accuracy
AI-powered voice-to-text translation tools have made significant strides in their ability to accurately process Asian languages, achieving a remarkable 97% accuracy rate. This development signifies a substantial leap forward in facilitating communication across language barriers, especially for speakers of languages that have historically posed challenges for automated translation. While these tools are proving beneficial for fast and efficient translation, they still encounter hurdles when it comes to fully capturing the intricate nuances of human language, especially cultural connotations and expressions that are deeply embedded in specific contexts. This presents an ongoing challenge for the field of AI translation, underscoring the need for a careful evaluation of their strengths and weaknesses. As AI technology evolves, maintaining a balance between the desire for quick translation and the need for accurate and contextually relevant translations will be vital in ensuring that these tools truly support human understanding across diverse languages.
Voice-to-text translation tools are making significant strides in handling Asian languages, reaching impressive accuracy rates of up to 97%. This level of precision is largely attributed to the use of sophisticated neural networks that are trained on vast and diverse language datasets. These networks are capable of learning and adapting to the complex intricacies of different languages, effectively mimicking the abilities of human interpreters in many standard situations. However, it's worth noting that even with these advancements, there are still areas where they fall short.
These translation systems rely on advanced automatic speech recognition (ASR) algorithms that analyze vocal patterns, including tone and pitch. This is especially crucial for tonal languages like Mandarin and Thai where changes in tone can significantly alter the meaning of a word. The design of these algorithms is focused on capturing these subtle features, leading to better performance in real-world scenarios. It's interesting to consider how the design choices in these algorithms influence their efficacy in handling such diverse and complex languages.
The integration of deep learning techniques has pushed the boundaries of processing speed, allowing these tools to translate voice input in a fraction of a second. This speed advantage is a game changer in scenarios requiring immediate communication, like urgent business discussions or emergency situations where rapid translation is critical. However, it remains to be seen how this speed impacts the quality and nuances of translation.
Despite their high accuracy, these systems still encounter difficulties with regional slang and dialectal variations, which can sometimes result in mistranslations. There is ongoing research into incorporating regional language models that are tailored to specific dialects. These models aim to bridge the gap between standard language and local vernaculars, further enhancing the system's capabilities.
Pairing OCR functionality with voice-to-text translation allows for the simultaneous translation of audio and text, creating a richer understanding of the context. This combined approach can be quite useful in complex situations where visual cues from written materials like signs or documents are present. The integration of these two technologies seems to enhance the overall translation experience, particularly in intricate environments. However, it's important to consider whether these combinations of technologies create new challenges or complications that need to be addressed.
Continuous learning and user feedback are key features being implemented into the latest tools. These features allow the translation algorithms to continuously refine and update their translations based on user interactions. This enables them to adapt to new expressions, slang, and evolving idioms that are essential for maintaining relevance in dynamic linguistic settings. The integration of user feedback can be seen as a way for these AI systems to become more adaptive and human-centered. However, one might question whether this kind of feedback can truly lead to a comprehensive understanding of the intended meaning, especially in the context of diverse cultural norms and expressions.
These tools are finding applications beyond traditional translation scenarios. In education, they are being incorporated into language learning programs to provide immediate translations and pronunciation guidance. This can significantly improve the learning experience for those acquiring new languages. It's interesting to see how these translation technologies are being utilized in educational contexts to enhance learning and access.
While the accuracy of these tools is impressive, they are not without limitations. Experts in the field recognize that these systems often struggle with nuanced context-dependent phrases and cultural expressions that generally require human intuition to translate effectively. This observation highlights a key challenge in the field—the extent to which technology can truly replicate human understanding of language and culture. Further research is needed to determine how these tools can be developed to address these challenges.
Furthermore, AI researchers are increasingly creating more robust models to process dialects and historically underrepresented languages. This commitment to inclusivity is essential to ensuring these languages are preserved and made available in modern translation applications. This expanding reach of these technologies may offer an opportunity to bridge the digital divide that many language communities experience, but there are likely many factors that must be carefully considered to fully realize this goal.
Lastly, there's a growing trend of implementing voice-to-text translation tools in accessibility technologies for the hearing impaired. Providing real-time text translations of spoken language makes communication significantly easier for this group. This use case underscores how these tools can promote inclusivity and improve communication within and across diverse linguistic communities. While these advancements are quite positive, it is important to remain mindful of the ethical implications and potential biases that can be introduced in these technologies and to ensure that they are designed to promote equitable communication.
7 Ways AI Translation Tools Mirror and Challenge Human Language Understanding in 2024 - Local Translation Memory Banks Cut Processing Time by 68% for Technical Documents
Utilizing local translation memory banks has dramatically impacted the speed of processing technical documents, leading to a remarkable 68% reduction in processing time. These banks essentially act as repositories for previously translated terms and phrases, enabling translators to quickly access and reuse them, ensuring consistency within and across various technical documents. This streamlined approach accelerates the overall translation process, making it a more efficient endeavor. However, this reliance on past translations can sometimes present challenges in handling the more complex aspects of language, where subtle nuances of meaning and context are paramount. While AI-driven translation tools are improving in their ability to address these complexities, fully capturing the intricacies of human language remains a challenge. As these AI tools develop further, it is crucial to consider the delicate balance between improving the speed of translation and ensuring that translations accurately convey the intended meaning within a specific context. This dynamic will continue to shape the landscape of translation and the roles of both humans and machines in the process.
Storing previously translated segments in local translation memory banks has shown a remarkable ability to speed up the processing of technical documents. Researchers have observed a reduction in processing time of up to 68% when using this approach. It's a compelling example of how reusing existing translations can significantly boost productivity, particularly in settings where fast turnaround times are essential. This efficiency gain is primarily due to the fact that the system doesn't have to translate the same phrases or sentences multiple times.
One of the most important aspects of technical documentation is consistency. The use of translation memory helps ensure that the same terms and phrases are translated the same way across different documents. This is especially important in fields like engineering or medicine, where the slightest ambiguity could have major consequences. However, it's interesting to consider how effectively the technology handles cases where a term has several potential translations depending on the context.
There's also a strong economic argument for utilizing translation memory. When a translation system can quickly identify and reuse existing translations, it cuts down on the amount of new text that needs to be processed by human translators. While this reduces the human workload, it's not yet entirely clear if this leads to an overall improvement in translation quality. For organizations dealing with large volumes of technical documentation, this can lead to major cost savings, making it a worthwhile investment. It remains to be seen how well this approach works for a more diverse range of technical fields, like creative design or the social sciences, where the need for nuance and interpretation might be different.
Keeping translation memories local instead of relying on cloud services can also have significant benefits for data security. This is crucial for companies or industries handling sensitive information or intellectual property. However, maintaining a local system can potentially create challenges with version control and sharing translation data across teams. This can make the data management aspect more complex, so it's interesting to explore the trade-offs between security and ease of data management.
Additionally, local translation memory banks can be fine-tuned for particular language pairs. This enables more nuanced translations that are tailored to specific regions or technical domains. It's important to consider, however, whether this level of specialization potentially limits the flexibility of the system.
Some translation memory systems have begun to integrate with OCR (Optical Character Recognition) technologies. This combination enhances their ability to rapidly process a wider range of documents, including scanned materials and images. This integration offers some exciting possibilities, such as quickly converting historical technical documents or translating field notes captured in photographs. It remains to be seen how well this approach works across a broader range of image types and handwritten text.
Furthermore, incorporating user feedback within translation memory systems can refine translations over time. This adaptive approach can help improve accuracy and maintain alignment with the latest language trends or variations. However, establishing and verifying reliable feedback mechanisms in such a system requires a thoughtful approach.
Using a translation memory bank enables human translators to focus on more complex and creative tasks that require nuanced judgment and language skills. This can lead to an overall increase in project throughput and a better utilization of the human expertise involved. However, there can be a risk that over-reliance on translation memory can limit the development of human translation capabilities.
Technical documentation often requires the standardization of terminology. This helps prevent ambiguity and ensures that all involved in a project understand specific terms in the same way. Translation memory systems can play a valuable role in establishing and enforcing these terminological standards. It will be interesting to see how these systems develop the ability to incorporate context-specific terminologies.
Finally, local translation memory systems can be scaled to support larger and more complex organizations with distributed teams. This enables companies to centralize their translation efforts while adapting the resources to particular technical fields or regional variations. However, creating an effective data sharing system in a distributed setting requires carefully designed architecture that balances speed and security.
7 Ways AI Translation Tools Mirror and Challenge Human Language Understanding in 2024 - Automated Quality Checks Flag Context Errors Using Semantic Analysis
AI translation tools are increasingly incorporating automated quality checks that utilize semantic analysis to identify errors related to context. These checks, powered by advanced language models, go beyond basic grammar and spelling checks, aiming to understand the meaning and nuances within the translated text. This approach offers a way to refine machine-translated outputs and enhance accuracy, especially when dealing with complex or culturally sensitive information. However, it's crucial to remember that AI still faces challenges in fully replicating human understanding of language, particularly in capturing context and subtle meaning. The implementation of automated checks raises questions about the trade-offs between speed and the accuracy of translations, particularly those with crucial contextual information. While advancements in semantic analysis are impressive, they highlight the continued need for careful evaluation of AI-generated translations and a recognition that machines haven't yet completely mastered the nuances of human language.
AI translation tools are becoming increasingly sophisticated, and one of the most interesting developments is the integration of semantic analysis into automated quality checks. This means these tools aren't just looking at grammar and syntax anymore; they're trying to understand the meaning and context of the text being translated. For instance, they can now recognize when a translation misses the cultural nuances of a phrase or idiom, something that was difficult for earlier translation systems.
This increased focus on meaning is helping to reduce the number of errors that slip through the cracks. By comparing the meaning of the translated text to established linguistic norms, the system can flag potential misinterpretations. It's like having a built-in language expert scrutinizing every translation for clarity and accuracy. Machine learning algorithms are being used to further refine these checks, identifying and learning from recurring errors across various languages. The more these systems are used, the better they become at predicting and avoiding common mistakes.
This approach is particularly helpful in specialized fields like technical translation or legal documents where the correct use of terminology is essential. These systems can be tailored to specific areas, improving the quality of translations for particular domains. It's also noteworthy that OCR (Optical Character Recognition) technology is being combined with semantic analysis, allowing the tools to assess context directly from images of documents. This significantly expands the scope of these quality checks.
There's also a growing trend toward analyzing the emotional tone of a translation. The aim is to make sure the translation doesn't inadvertently change the emotional impact of the original text. It's a step towards making AI translations more nuanced and aware of the subtleties of human communication. Furthermore, these tools are increasingly capable of providing real-time feedback, which is helpful for quick corrections and refinements, particularly in scenarios like live translation or technical discussions where immediate feedback is crucial.
All these improvements have a real impact on the development of AI translation tools. By identifying errors and providing feedback, these automated quality checks inform the underlying machine learning models. This leads to a continuous cycle of improvement, ensuring that future generations of translation models are increasingly accurate and contextually relevant. These continuous refinements, in turn, can also lead to greater user customization, enabling users to tweak quality checks to align with their individual preferences and needs. However, there is still a lot we don't know about how well these systems can handle the more intricate aspects of language and culture. While impressive, it's a journey of exploration and evolution in understanding and mirroring the intricate dance of human language.
AI-Powered PDF Translation now with improved handling of scanned contents, handwriting, charts, diagrams, tables and drawings. Fast, Cheap, and Accurate! (Get started for free)
More Posts from aitranslations.io: