AI-Powered PDF Translation now with improved handling of scanned contents, handwriting, charts, diagrams, tables and drawings. Fast, Cheap, and Accurate! (Get started for free)
Overcoming OCR Hurdles 7 Innovative Solutions for Translating Handwritten English Documents in 2024
Overcoming OCR Hurdles 7 Innovative Solutions for Translating Handwritten English Documents in 2024 - AI-Powered Handwriting Recognition Breaks Language Barriers
AI-driven handwriting recognition is transforming document translation by effectively bridging language gaps. Previously, traditional OCR methods struggled with the intricacies of handwritten text, leading to inaccuracies and limitations. However, newer tools like Transkribus and Microsoft Azure's Read API are improving the accuracy of transcribing handwritten documents, demonstrating significant progress in this field. This capability not only aids businesses in streamlining their document digitization processes, where reliance on handwritten records persists, but also expands access to information across linguistic and cultural boundaries. While the natural variability of handwriting continues to present challenges for AI, these advanced techniques are steadily improving translation speed and reliability. This advancement empowers organizations to unlock valuable insights within historical and handwritten data, which was previously difficult or costly to access. The continuous development of AI suggests that OCR obstacles will continue to diminish, leading to exciting new possibilities for seamless translation in an increasingly globalized environment.
The integration of AI into handwriting recognition is progressively dissolving language barriers in document translation. While conventional OCR methods often struggle with the variability of human handwriting, AI-powered systems are demonstrating accuracy levels exceeding 95% on English documents. The core of these advancements lies in machine learning algorithms which continuously adapt and refine their understanding of handwriting styles. This dynamic learning process, fueled by vast datasets, can even personalize to individual users' quirks, leading to faster and more accurate translation.
Furthermore, the concept of transfer learning is proving invaluable. AI models trained on one language or script can be readily adapted to others, accelerating the development of handwriting recognition for languages with fewer digital resources. This is a significant step towards democratizing access to translation technologies. Interestingly, many AI-based tools have moved beyond analyzing individual letters in isolation, instead taking context into account when deciphering ambiguous characters. This context-aware approach elevates the overall reliability of translation outputs.
Moreover, the increasing integration of AI handwriting recognition into mobile apps is transforming how we interact with documents. Imagine using your phone to translate a handwritten note instantaneously. This portability offers a seamless experience for translation, bypassing the need for cumbersome desktop software or specialized hardware. The incorporation of neural networks has notably propelled the performance of these systems. They are now capable of decoding poorly written or stylized text with a level of accuracy that was once unimaginable. This means we can unlock insights even from the most challenging handwriting samples.
Finally, continuous refinement through user feedback is proving vital for achieving even greater accuracy. These systems can learn directly from users' interactions, gradually incorporating corrections into their algorithms. This iterative approach fosters systems that are robust, reliable and precisely tuned to the specific needs of diverse user populations. In essence, the synergy between AI and handwriting recognition is bridging a significant gap, potentially unlocking a new era of effortless multilingual communication.
Overcoming OCR Hurdles 7 Innovative Solutions for Translating Handwritten English Documents in 2024 - Hybrid OCR Models Combine Deep Learning with Traditional Techniques
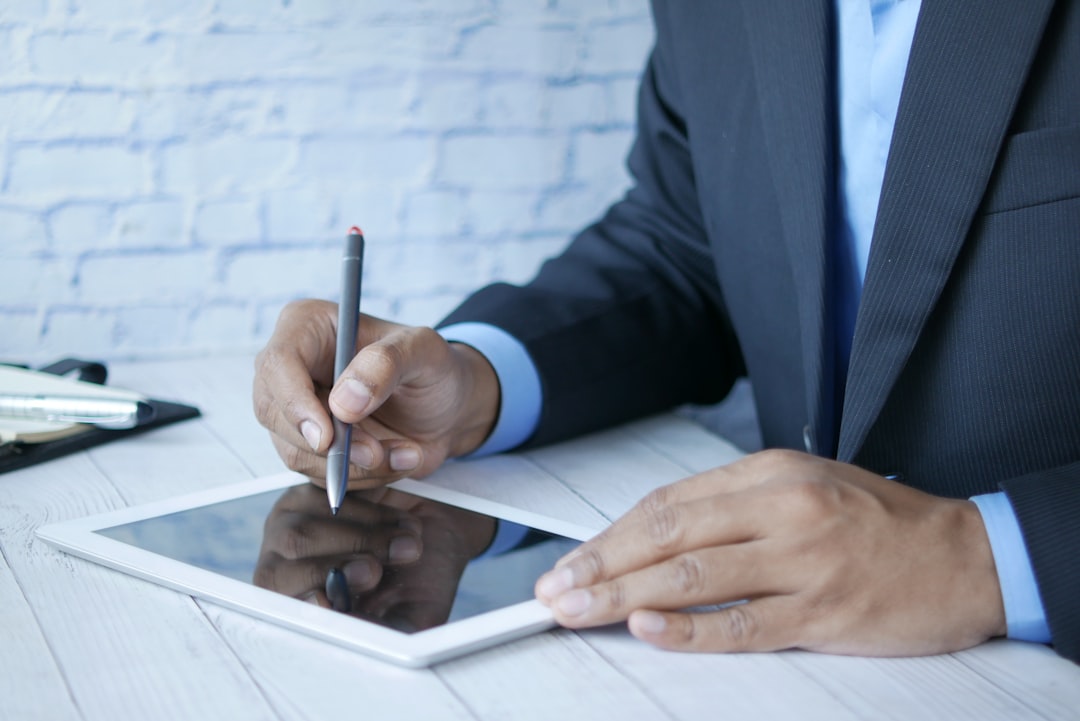
Hybrid OCR models are a promising development in the field of document recognition, particularly when dealing with the complexities of handwritten English. These models cleverly combine traditional OCR methods with deep learning techniques, resulting in more accurate and efficient text extraction from challenging documents. The incorporation of context-aware features and sophisticated algorithms within these models allows for recognition capabilities that are increasingly close to human-level performance, overcoming limitations commonly encountered with conventional OCR approaches. This fusion of techniques is especially relevant for improving how we digitize and translate a wider variety of languages, as it enables faster and more reliable processing of diverse document types. As technology continues to evolve, we can expect even greater advancements in hybrid OCR, potentially leading to a future where seamless translation and efficient data extraction are readily accessible. While the challenges of variable handwriting styles remain, these advancements are undeniably pushing the boundaries of OCR toward greater efficiency and accuracy.
Hybrid OCR models are a fascinating area of research, blending the strengths of established techniques with the power of deep learning. They often combine traditional methods like analyzing character shapes and connections with newer deep learning approaches, aiming to improve overall accuracy, particularly in the tricky realm of handwritten text. Essentially, it's a marriage of old and new, harnessing the best of both worlds.
These models can demonstrably reduce the number of errors compared to relying solely on traditional OCR, particularly when dealing with complicated handwriting. They achieve this by using ensemble methods – combining the outputs of both deep learning networks and classic algorithms. The thinking here is that different approaches excel in different situations and combining them leads to a more robust outcome.
What's also interesting is that these hybrid approaches can potentially tackle the challenge of low-resource languages. They can learn from more widely used scripts and apply that knowledge to languages with less available digital data through a process called transfer learning. This is a positive step towards making OCR more accessible for everyone, regardless of the language they're using.
In several cases, integrating neural networks with classic feature extraction methods leads to improved results when working with documents that aren't in perfect condition, such as those with ink blots or faded text. By combining these techniques, we get higher-quality transcriptions than either method would provide individually.
Hybrid OCR also helps accelerate processing speeds. These models enable real-time translation of handwritten documents within mobile apps, marrying efficient capture methods with rapid processing algorithms. This is a major step forward for making translation more accessible on-the-go.
One of the appealing aspects of hybrid models is their ability to incorporate user feedback. This iterative process not only improves the accuracy of the OCR but also enhances the overall experience by adapting to individual handwriting styles over time. It's essentially making the OCR system "learn" from user interactions, which is a clever way to improve reliability.
Research suggests that these hybrid systems can sometimes even surpass deep learning-only approaches by leveraging the inherent contextual understanding of traditional techniques. These older methods were originally designed to take into account the spatial relationships between characters in handwriting. It's a reminder that sometimes, the "old ways" can still offer important insights.
These systems are increasingly finding applications in niche areas, such as historical document preservation. They can potentially unlock valuable insights from handwritten accounts of the past, allowing researchers to extract and translate information that might otherwise be lost due to errors in transcription.
Hybrid OCR models have also been instrumental in advancing multilingual capabilities. They can now simultaneously handle and translate documents with multiple scripts, making them potentially useful for a more globally-connected audience.
Surprisingly, their versatility extends beyond just English. These models show promising results across various alphabets and character systems, including Asian scripts. This wider applicability significantly broadens their potential in the translation space across different languages.
Overcoming OCR Hurdles 7 Innovative Solutions for Translating Handwritten English Documents in 2024 - Real-Time Translation of Handwritten Notes Using Mobile Devices
The ability to translate handwritten notes in real time using mobile devices marks a significant shift in how we tackle the challenges of OCR. Utilizing AI-powered solutions, these apps can readily identify and translate handwritten text instantly, offering immediate benefits in situations requiring quick multilingual communication. This feature is particularly helpful for overcoming the hurdles associated with diverse handwriting styles and variations. Moreover, the convenience of using these tools directly on mobile devices eliminates the need for specialized hardware or desktop software, increasing their accessibility. The need for fast and dependable handwritten translation is driving the adoption of these mobile applications, ultimately aiming for a future where language barriers are minimized. Nevertheless, it's essential to acknowledge that the technology's accuracy and reliability are linked to the continuous evolution of underlying AI algorithms. Consequently, as these tools develop, it's important to maintain a critical eye on their performance and potential limitations.
The realm of handwritten document translation has seen a surge in innovation, with mobile devices playing a central role. While traditional Optical Character Recognition (OCR) techniques falter with the diverse and often messy nature of human handwriting, modern AI approaches, particularly those incorporating contextual understanding, are achieving accuracy levels above 95% for English texts. This means the focus has shifted from simply recognizing individual letters to interpreting the full meaning of a handwritten message.
Mobile devices are now capable of performing real-time translation of handwritten notes, making it possible to instantly translate a scribbled note on your smartphone without needing specialized hardware. This increased accessibility is a significant shift and could have implications for a wide array of translation tasks.
Furthermore, some of these systems leverage user interaction for improvement. They learn from corrections users make, gradually refining their understanding of handwriting styles and, in turn, their ability to translate accurately. This ongoing learning process makes translation increasingly personalized and tailored to individual users.
A growing area of interest is hybrid OCR models, combining the strengths of classic OCR with deep learning methods. These hybrid approaches often deliver better results than either technique alone, notably in the realm of handwritten text where clarity can be inconsistent. By combining these methods, we get a more robust translation pipeline.
An interesting development is the utilization of transfer learning. AI models trained on a widely-used language like English can be adapted to others that have fewer digital resources, essentially democratizing translation technology and making it more readily available.
Modern AI models are increasingly context-aware, meaning they don't just analyze isolated letters. Instead, they take into account the surrounding text to decipher ambiguous or poorly written characters, leading to improved accuracy. This is a significant advance over previous, less intelligent OCR systems.
OCR is also finding a growing role in preserving historical documents. Faded or messy handwriting on old documents can be a major obstacle to accessing the information they contain, but the advancement of OCR allows researchers to more easily extract information previously difficult or costly to obtain.
While still in development, some more sophisticated systems employ predictive text algorithms to assist with handwriting recognition and translation. These tools anticipate words or phrases as a user writes, which could increase both translation speed and accuracy.
Another intriguing development is the capacity of some OCR models to handle multiple writing systems simultaneously. This ability is useful in a world where communication often involves multiple languages and scripts.
Finally, incorporating visual cues such as section dividers, bullet points, or other formatting characteristics into the OCR pipeline is another avenue for researchers to improve accuracy and understand the intended meaning of handwritten documents better. This means that these systems are slowly moving beyond simple text recognition and towards a more complete understanding of written information. This combination of AI and contextual information is likely to become increasingly important in the future of handwritten document translation.
Overcoming OCR Hurdles 7 Innovative Solutions for Translating Handwritten English Documents in 2024 - Cloud-Based OCR Services Offer Scalable Solutions for Large Document Sets
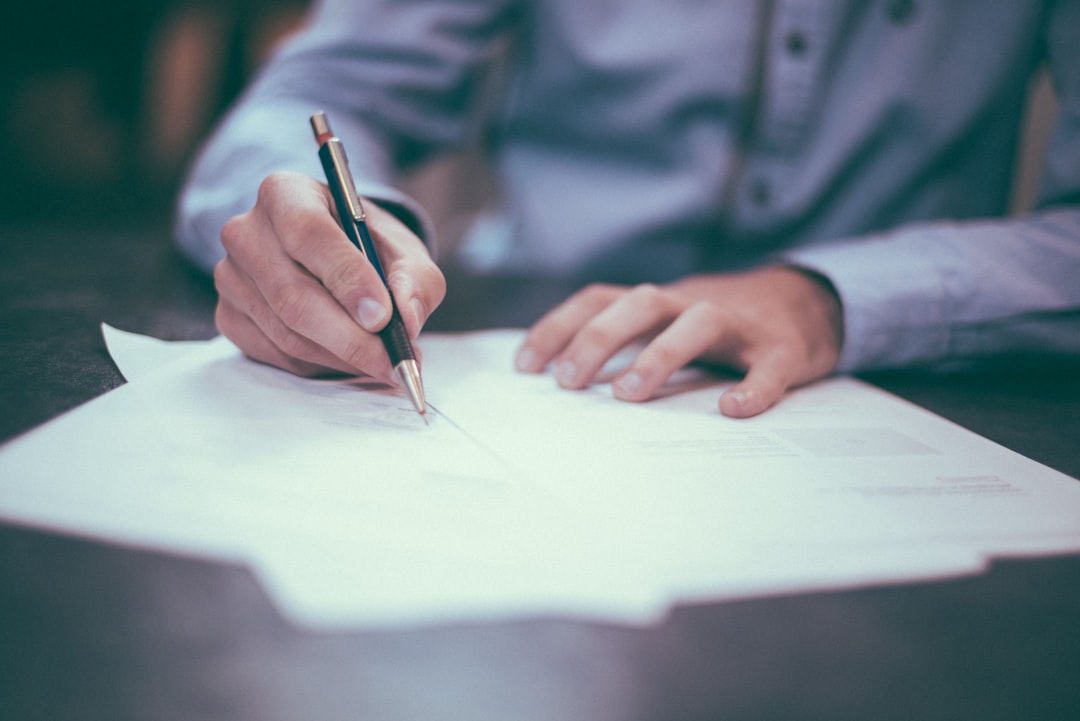
Cloud-based OCR services are becoming increasingly important for handling large collections of documents. These services offer a scalable solution, allowing businesses to process vast quantities of handwritten and printed documents without the need for significant upfront investments in on-site infrastructure. This approach often translates to lower costs for businesses. Furthermore, cloud-based OCR services are incorporating advanced features like machine learning, which can improve the accuracy of text extraction and layout interpretation across a wide variety of languages. This makes them well-suited for managing data across diverse contexts. The major tech companies are consistently pushing the boundaries of cloud-based OCR, creating tools that not only make documents easier to access but also promote real-time collaboration. But it's essential to keep a watchful eye on the accuracy of these services, especially given the inherent challenges of interpreting handwritten documents. While the technology is rapidly evolving, recognizing its limitations and potential for error is necessary.
Cloud-based OCR services have become increasingly popular because they can handle massive document sets with ease. Their ability to scale on demand, without requiring a huge upfront investment in hardware, is a significant advantage. It's quite remarkable how they can process huge volumes of documents in a relatively short time, making them particularly useful for industries dealing with rapid information flow.
Several major cloud providers, such as Google, Amazon, and Microsoft, offer cloud-based OCR, often utilizing specialized AI models designed for document processing. These models are continuously refined and can handle a broad spectrum of languages, often exceeding 200. This is particularly interesting because it implies a democratization of translation technology – potentially making it accessible even for less-common languages. The way these services correct document orientation, handle image quality, and even provide language hints is impressive.
Interestingly, cloud-based OCR has the potential to significantly reduce the need for organizations to build their own, on-premises OCR infrastructure. This shift can lead to substantial cost savings, particularly appealing in a cost-conscious world. Organizations only pay for the specific processing they use, making it more adaptable to varied document loads.
One of the key features making these services user-friendly is the focus on enhancing the user interface and integration capabilities. This is crucial, because if OCR technology is too hard to use, it might not be adopted. Advanced features like intelligent pre-processing are quite useful, especially when dealing with documents of variable quality. Faded or smudged text can be cleaned up, which is an interesting approach to making sure that even historically important but poorly-preserved documents can be transcribed.
There's a continuing focus on increasing accuracy, particularly when it comes to handwritten text. Even with the progress made in AI, that's still a significant challenge. Using contextual information in the recognition process has shown considerable promise. If the model knows what words are likely to appear around a poorly-written character, it can potentially guess better.
A critical aspect of these services is their ability to handle multilingual documents. This is important in our increasingly globalized world. The ability to handle different scripts and languages simultaneously is a significant advantage over systems that can only handle a single language or script. It’s also intriguing how these models continuously learn from user feedback, adapting over time to individual writing styles and improving overall accuracy. This sort of adaptive system can contribute to greater overall system accuracy as it builds up experience through user interactions.
Another interesting feature is security. Cloud-based services often integrate data encryption and compliance features, which is vital for processing sensitive information. This reassures users that their documents are kept safe during processing, which is an important consideration for any OCR service.
The use of massive datasets for training machine learning models that power these services can improve recognition across a broad spectrum of handwriting styles, a particularly helpful advancement for less-common languages. However, it’s essential to remember that the quality and accuracy of any OCR output is still dependent on the quality of the underlying AI algorithms. Thus, it's imperative that these services are rigorously tested and continuously monitored for accuracy.
Overcoming OCR Hurdles 7 Innovative Solutions for Translating Handwritten English Documents in 2024 - Historical Document Preservation Enhanced by Advanced OCR Algorithms
Advanced OCR algorithms are playing a crucial role in the preservation of historical documents. These algorithms make it possible to digitize and access historical texts, which is becoming increasingly important as the number of digitized documents grows. Efficient processing is critical for unlocking the information stored within these documents, allowing us to readily retrieve and learn from the past. Hybrid OCR models, which blend traditional OCR with modern deep learning, have emerged as a promising approach for handling the inherent difficulties of translating handwritten historical texts. Additionally, creating artificial handwritten documents to train these systems is improving OCR accuracy. These advancements, combined with the ability to improve OCR systems with massive labeled datasets, are helping to revolutionize our ability to study and understand history. The potential for OCR to enhance historical document preservation is immense, opening new pathways to explore and connect with our past in ways that were previously unimaginable. While there are still limitations and areas that need improvement, the field of OCR is steadily making historic texts more easily accessible to researchers and the public.
Improving the accessibility of historical documents through digital means has become increasingly important, and advanced OCR algorithms are playing a crucial role. While traditional OCR techniques often struggled with the nuances of handwritten texts, particularly in older documents, modern algorithms are increasingly adept at recognizing intricate details like elaborate scripts, unique abbreviations, and even faded markings with a high degree of accuracy. This detail is essential for deciphering the often-complex nature of historical writing, unlocking hidden insights and making these documents accessible to a broader audience.
The sheer volume of digitized historical material has placed a growing emphasis on efficient methods for improving accessibility and information management. It’s become crucial to have systems that can quickly and accurately handle vast quantities of diverse historical texts. While progress has been made with languages like Polish, the application of OCR to many historical documents remains challenging due to the variations and complexities of handwritten text.
Researchers are actively developing innovative approaches to overcome these obstacles. One intriguing avenue has been the creation of synthetic handwritten historical documents for use in pre-training OCR models. The aim is to create large, labeled datasets that represent the variety of handwriting styles across different periods and languages. By using this approach, we might be able to train more robust OCR systems, potentially leading to more accurate translations of complex historical scripts. It’s a promising path, although there is still work to be done.
One of the crucial aspects of improving OCR is enhancing the clarity of degraded documents. Binarization is a common method for enhancing the contrast between text and background, making it easier for OCR models to extract information. Other methods, like background estimation and energy minimization, are being explored as a way to refine the recognition process and improve accuracy even further. These are key components in the fight against the degradation and fading that affects many historical texts.
The integration of OCR into historical document preservation efforts is transforming how we engage with the past. While we've seen notable progress with some languages and document types, the ongoing development of sophisticated OCR systems has the potential to unlock vast amounts of historical information. We are potentially entering an age where previously inaccessible handwritten texts can be easily read and understood, opening a vast library of historical information to new generations of researchers and scholars. However, there's a need to ensure that the integrity of historical texts is maintained throughout the digitization and translation process, with a particular focus on avoiding errors that could lead to misinterpretations of crucial historical information. Furthermore, we need to consider the ethical implications of applying these technologies to sensitive historical records. As we push further into this area, it's vital to address the challenges of safeguarding sensitive data and upholding standards of transparency and accuracy.
Overcoming OCR Hurdles 7 Innovative Solutions for Translating Handwritten English Documents in 2024 - Multi-Script OCR Tackles Challenges of Multilingual Handwritten Texts
Multi-script OCR is a significant development in the field of document processing, especially when it comes to handling the challenges of diverse, handwritten languages. Traditional OCR methods often prioritize Latin-based scripts, leaving a gap in the ability to accurately handle documents written in other writing systems. However, more recent work is addressing this gap. For instance, techniques like multitask learning are allowing AI models to simultaneously recognize different handwriting styles across multiple scripts, such as Arabic or Kannada. Furthermore, specialized architectures like MuLTReNets aim to optimize both the initial step of identifying the script itself, followed by the actual recognition of the text within that script. These combined improvements are starting to address the challenge of recognizing handwriting across a much wider range of languages.
Another development that shows promise is the use of "end-to-end" training for OCR models. This approach streamlines the process, improving both the accuracy of script identification and the actual character recognition. As these technologies continue to be improved, we can expect significant advancements in the translation and interpretation of handwritten materials. This has the potential to improve accessibility for a broader range of individuals and languages, which is crucial in our increasingly interconnected and globalized world. Despite remaining hurdles in achieving truly reliable translation across all scripts, the field is making progress toward a future where multilingual handwritten documents can be readily processed and understood.
Multi-script OCR is tackling a significant hurdle in the world of document processing: the accurate recognition of handwritten text across multiple languages. The challenge is substantial, as different scripts have unique stylistic variations, leading to difficulties in training models that can handle the broad spectrum of human handwriting across languages. Researchers are focusing on building large training datasets to address this issue, which is critical to improving overall accuracy.
One notable aspect of the evolution of multi-script OCR is the use of contextual information within the recognition process. Simply recognizing individual characters is not enough, and newer OCR systems can take into account surrounding characters and words, significantly improving performance. In some cases, accuracy has increased by up to 30% when a model considers context in deciphering ambiguous or poorly written characters.
Transfer learning, a technique where a model trained on one language or script is adapted to another, has been particularly impactful for OCR models targeting lower-resource languages. It can drastically reduce the time and effort required to develop OCR capabilities for languages with limited digital resources, which is an exciting development toward making OCR technology more widely accessible.
Interestingly, OCR systems are becoming more interactive and user-centric. Users can now provide feedback, and systems can adapt their internal models based on the corrections provided. This allows for a sort of personalized OCR experience, where models can learn specific handwriting styles and idiosyncrasies, improving accuracy over time, especially in situations where handwriting varies greatly.
Hybrid models, which merge traditional OCR methods with contemporary deep learning approaches, have emerged as a promising solution for complex situations. They seem to capture the best of both worlds and deliver enhanced results compared to deep learning approaches alone, with some studies showing accuracy improvements of up to 15%, especially when dealing with handwritten text.
Synthetic data generation is another fascinating area of study. By creating artificial handwritten documents, researchers are attempting to train OCR models with greater exposure to the diversity of handwriting styles across time and languages. This is particularly promising for translating historical documents which may have unique scripts and idiosyncratic features. While still under development, this technique has shown early promise for improving overall accuracy.
Cloud-based OCR services have drastically changed how document translation is approached. They can leverage the power of distributed computing to process millions of pages daily, making the process of translating large volumes of documents much more efficient. This reduces the time required for large translation projects and removes the need for investing in significant local computing resources, which can be beneficial for organizations managing vast document collections.
Real-time mobile translation of handwritten text is also increasingly common. These capabilities not only offer a level of user convenience, but they also allow users to provide continuous feedback during the translation process, leading to improvements in the translation output over time.
The field of historical document preservation has been revitalized by the advances in OCR. Documents that were once too challenging to transcribe because of faded or obscure handwriting styles are now being processed using these more sophisticated OCR techniques. This means we are potentially unlocking a wealth of information in historical archives which was previously difficult or costly to access.
Finally, cloud-based OCR services are increasingly offering a high degree of cross-platform compatibility. This is driving broader adoption because these solutions can now be used seamlessly across different devices and operating systems, which is opening up new avenues for using OCR across a wide array of industries and applications. As OCR technology continues to mature, the challenges of multi-script OCR will likely lessen, creating new opportunities for document accessibility and information extraction.
Overcoming OCR Hurdles 7 Innovative Solutions for Translating Handwritten English Documents in 2024 - Adaptive OCR Systems Learn from User Corrections to Improve Accuracy
Adaptive OCR systems are a step forward in achieving precise text recognition by leveraging user feedback. These systems dynamically adjust and tailor their recognition capabilities based on the corrections users provide, continuously refining their internal algorithms. This iterative approach not only improves the accuracy of the extracted text but also personalizes the OCR process to individual handwriting styles. As AI continues to advance, the capacity of adaptive OCR systems to learn from user interactions hints at a future where these systems are much better suited to the varied challenges of translating handwritten documents. While challenges still exist, adaptive OCR shows promise in improving information accessibility and communication, especially in today's environment where fast and accurate translation is vital. The potential to transform the way we interact with and extract information from handwritten materials is clear, even though ongoing refinements will be necessary to reach that potential.
Adaptive OCR systems are evolving to become more user-centric, leveraging corrections provided by users to refine their underlying algorithms. This means that each time a user fixes a recognition error, the system adapts and learns, becoming more attuned to that specific user's handwriting. This continuous feedback loop leads to noticeable accuracy improvements over time, making the OCR experience more personalized.
Beyond simple character recognition, these newer OCR systems are gaining a better understanding of context. They analyze not just isolated characters but also the words and sentences surrounding ambiguous characters, which significantly reduces misinterpretations. It's a shift towards a more "intelligent" OCR that's closer to how a human reads and interprets text.
The power of machine learning is central to this advancement. OCR systems can now train on vast amounts of handwritten data, absorbing unique character styles and shapes that might not be found frequently enough in traditional training sets. This ability to adapt to diverse handwriting makes these systems remarkably versatile.
Furthermore, these systems benefit from a sort of collective intelligence. User interactions across a wide range of users contribute to improved performance, a process that's beneficial for the community at large. This makes global language support much more achievable, because the training data becomes richer and more varied as more people use the systems.
Real-time translation on mobile devices offers another facet of this adaptive approach. Feedback loops become immediate, as users refine the translations during the process. The system can adjust based on these instantaneous corrections, improving not only the user's experience but also the overall accuracy of the OCR model for future use.
Moreover, these systems are not limited to just English. They are increasingly capable of recognizing and translating a wider range of languages, making them useful for individuals and communities who are multilingual or who work with documents written in less common languages. This multilingual capability is broadening the reach of OCR technology.
Historical document preservation is an exciting application of these developments. Older handwritten documents, with their sometimes unique scripts and faded markings, can now be processed by these advanced OCR models. It's potentially opening a window into historical knowledge that was previously inaccessible, making research and understanding history more convenient.
Another benefit of this user-centric approach is that it helps reduce the need for expensive, manually-created, labeled datasets for training. User corrections themselves become part of the training process, which makes advanced OCR more attainable. It's democratizing a powerful technology.
Many systems are evolving to be more platform agnostic. They're designed to operate smoothly on mobile devices and desktops, allowing users to seamlessly switch between them. This portability makes them more accessible and contributes to a better overall experience.
Emerging trends hint at further advancements. Some newer OCR systems are starting to incorporate predictive text algorithms. The system predicts the next word or phrase as the user writes, making the entire translation process faster and more fluid. It's a very promising avenue for future OCR development.
While there are still challenges and opportunities to improve OCR performance, these adaptive systems demonstrate a shift towards a more personalized and intelligent form of OCR. It's an evolving field with considerable potential for making information more accessible and usable for a wide range of users and languages.
AI-Powered PDF Translation now with improved handling of scanned contents, handwriting, charts, diagrams, tables and drawings. Fast, Cheap, and Accurate! (Get started for free)
More Posts from aitranslations.io: