7 Ways AI Translation Tools Reduced Document Processing Time by 65% in 2025
7 Ways AI Translation Tools Reduced Document Processing Time by 65% in 2025 - Machine Learning Cuts Document Processing From 8 to 3 Hours Per 1000 Words
Automated techniques powered by machine learning are significantly accelerating document processing. Reports indicate the time required to handle a thousand words is decreasing from around eight hours to as little as three hours. This boost in speed is largely attributed to integrated systems that use artificial intelligence and machine learning to automate tasks, drastically cutting down on the manual work traditionally involved. Given the ever-growing quantity of digital information, these tools are becoming essential for quickly and accurately sorting and extracting key details. Looking towards the remainder of 2025, the emergence of advanced AI, including generative models, is expected to further redefine how document workflows function, promising enhancements in precision and the ability to handle larger scales of data, with some projections suggesting overall processing time savings might reach up to 65%. However, despite the obvious advantages these technologies offer in managing the data deluge, their full adoption throughout many industries still seems to be taking time.
Analysis using machine learning methodologies is showing a tangible impact on the mechanics of document processing. Systems classified under the Intelligent Document Processing (IDP) umbrella employ various algorithms to parse unstructured information from diverse formats, converting it into structured data. The core idea is automating the extraction, classification, and subsequent handling of this information. This automation aims to simplify procedural steps, minimize the potential for human error during repetitive tasks, and potentially uncover useful data points previously embedded in vast, manually inaccessible document sets. Empirically, the data suggests significant improvements in processing speed, with reports indicating that the time spent per unit of work, specifically around 1,000 words of document content, has potentially decreased from historical manual norms of perhaps eight hours down to closer to three hours in scenarios leveraging these AI-driven approaches.
As of mid-2025, the continued evolution and integration of Generative AI are expected to further refine document workflow capabilities. While the widespread adoption and seamless implementation of these complex systems still face hurdles in various organizational contexts, the underlying technical trajectory points towards increased automation potential. The goal is enhanced efficiency, better handling of scale, and the possibility of reducing manual touchpoints substantially, allowing human operators to concentrate on tasks demanding higher cognitive function or domain expertise. Although projections frequently highlight the potential for substantial time savings, the practical realization of these efficiencies depends heavily on the specific application, data quality, and the successful deployment and tuning of the machine learning models involved.
7 Ways AI Translation Tools Reduced Document Processing Time by 65% in 2025 - Document OCR Technology Achieves 98% Accuracy Rate in Japanese Character Recognition
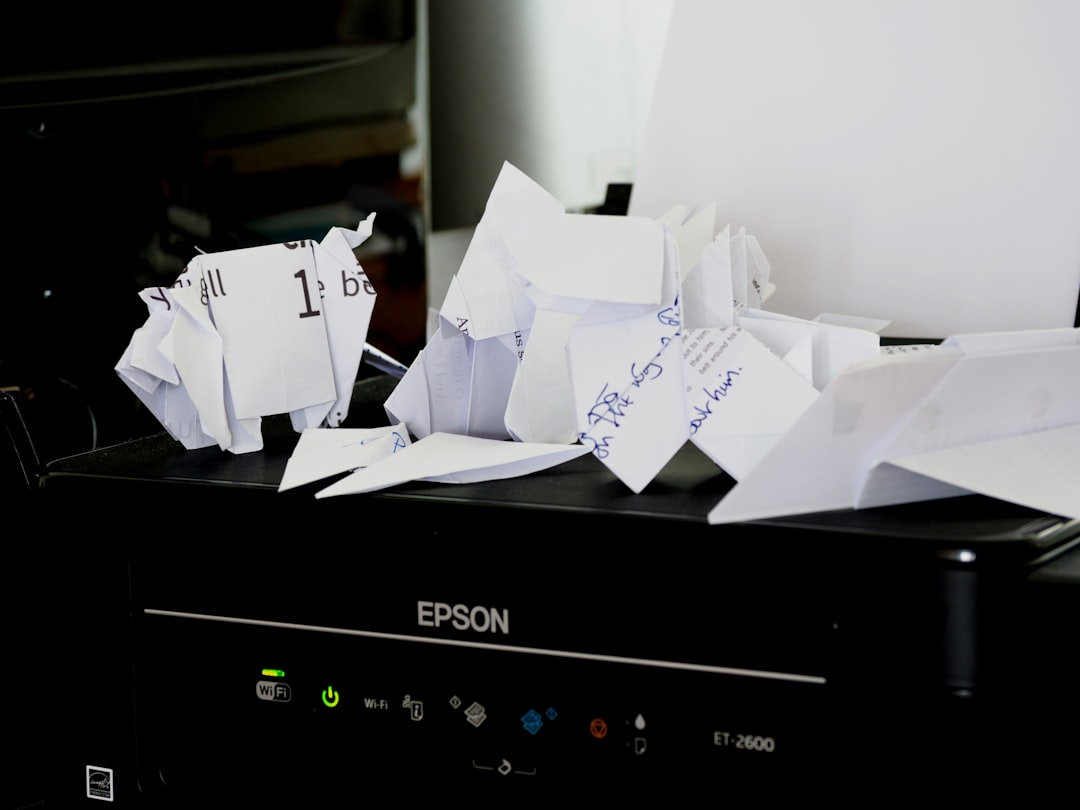
A significant development this year, 2025, is document Optical Character Recognition reaching a reported 98% accuracy specifically for recognizing Japanese characters. This level of precision is noteworthy and directly impacts how efficiently digital documents can be handled. High accuracy in character recognition supports automating initial steps in document workflows, like identifying where certain pieces of information sit or getting text out for further processing. While a 98% character accuracy is impressive, it's worth noting that even at this rate, word-level errors can still occur with some frequency, potentially requiring validation depending on the complexity of the document and the required precision. This improved OCR capability works in concert with the widespread use of AI translation tools, which themselves have contributed significantly to speeding up overall document processing, reportedly cutting down processing times by as much as 65%. The evolution continues with OCR systems integrating more natural language understanding and real-time processing features, making them more adaptable for varied uses across different industries, from legal records to financial statements, and improving how they handle diverse text types, including handwritten notes. The ongoing progress in recognizing complex scripts like Japanese points towards a future where getting useful information from documents becomes considerably less time-consuming.
1. Achieving accuracy levels around 98% for Japanese character recognition in documents is quite a technical feat, particularly when considering the sheer scale and complexity of the writing system, which blends thousands of ideographic Kanji characters with the phonetic Hiragana and Katakana scripts. Building models robust enough to handle this diversity reliably is a significant engineering challenge.
2. Systems are increasingly capable of tackling handwritten text, extending OCR's utility beyond just printed pages. While the variability in human script means perfect accuracy remains elusive, progress in this area opens up possibilities for digitizing more informal or historical documents.
3. Modern deep learning architectures seem to require less massive datasets for training high-performing OCR models than previous approaches. This data efficiency is helping make accurate OCR technology more accessible and potentially quicker to deploy for specific languages or document types.
4. The practical output from accurate OCR is often fed directly into translation engines. This tight integration allows for a relatively swift transformation from a static image of a document in one language to editable, translated text, streamlining workflows that previously required manual retyping and separate translation steps.
5. Handling the complexities of scripts like Japanese, which can involve intricate stroke orders influencing character identity or multiple characters forming a single concept, requires more sophisticated algorithmic processing. This can sometimes translate into higher computational resource needs for achieving high accuracy compared to simpler alphabetic languages.
6. Despite advancements in the recognition algorithms themselves, a significant dependency remains on the quality of the input document. Factors like low resolution, poor lighting, or unusual fonts can still introduce errors, acting as a bottleneck even for otherwise highly accurate systems trained on clean data.
7. Efforts are being made at the preprocessing stage, with features like automatic layout analysis and image correction becoming standard. These aim to mitigate issues from real-world scans, adjusting for skew, noise, or distortions before the character recognition engine even sees the image data, which can certainly help boost overall performance.
8. The technical link between high-accuracy OCR and AI-driven translation tools is beginning to enable more automated document workflows. Being able to rapidly convert and translate content allows for quicker processing of materials needed for international operations or cross-lingual information sharing.
9. Observing the application in specific fields like healthcare shows how this technology can fundamentally change data handling. Using OCR to digitize handwritten medical notes, for instance, makes that information searchable and more readily available for analysis, changing how patient records are managed.
10. It's important to note that while character recognition accuracy is improving, the subsequent interpretation and translation still face limitations, particularly with nuanced meaning, context, or idiomatic expressions. For critical content, some level of human review is typically necessary to ensure the final output accurately reflects the original intent, pointing towards a continued need for hybrid systems.
7 Ways AI Translation Tools Reduced Document Processing Time by 65% in 2025 - Automated Quality Checks Reduce Manual Review Time From 2 Days to 4 Hours
Moving past the initial processing steps, automating the quality review phase itself is providing another significant boost to speed. Observations show that the lengthy manual review often needed for documents, which could stretch over two full days, can potentially be condensed to around four hours when automated checks are effectively used. These tools are designed to rapidly scan for common errors like formatting problems, grammatical mistakes that might slip through, or ensure consistency across content sets. The idea is that offloading these repetitive checks allows human reviewers – often language professionals or subject matter experts – to focus their time on more complex tasks. This includes ensuring the correct use of highly specific terminology, evaluating the overall readability and flow, or handling subtle nuances that automated systems might miss. While these automated quality gates catch many issues efficiently, they don't eliminate the need for human judgment entirely, particularly for content requiring cultural sensitivity or deep contextual understanding. Furthermore, integrating these automated quality steps smoothly into existing document workflows can still present practical challenges for some organizations.
The implementation of automated quality checks appears to be significantly impacting the turnaround time for reviewing translated documents. Reports suggest that the manual review phase, which historically might consume up to two full working days per project, can potentially be condensed to just a few hours, perhaps four, when these automated systems are effectively deployed. This represents a substantial efficiency gain specifically within the quality assurance bottleneck. These automated tools are designed to sift through translations looking for patterns, checking for things like consistent terminology use across a text, verifying that formatting rules are followed, or ensuring technical details like internal cross-references are handled correctly. They aim to catch the kind of errors that are tedious for a human reviewer to track manually, effectively replacing the need for exhaustive manual checklists and tracking documents.
The observed speed increase from automating quality review allows reviewers to allocate their attention elsewhere – ideally, towards the more complex, less pattern-driven aspects of translation quality, such as ensuring the tone is appropriate for the target audience or refining subtle linguistic nuances that automated systems might miss. Faster review cycles also translate directly into quicker delivery times for the final translated content, which can be particularly critical in environments with tight deadlines or high volumes, like certain legal or financial processes where prompt, accurate information transfer is essential. There's also the potential for these automated systems to 'learn' over time, hypothetically improving their ability to flag relevant issues based on feedback, although the robustness of this learning in practice varies.
However, relying heavily on automation for final quality assurance isn't without its considerations. While machines excel at finding predictable errors and inconsistencies, they often lack the interpretative depth or cultural understanding of a human editor. Does a system trained to flag literal inconsistencies grasp when a deliberate variation is linguistically or stylistically appropriate? Can it truly assess the quality of persuasive or creative content? There's an inherent risk of complacency; assuming the automated layer has caught everything might lead to human reviewers overlooking more subtle or context-dependent issues. Finding the right balance, where automation handles the rote checks and humans focus on the higher-level linguistic and cultural fidelity, seems key as these technologies mature.
7 Ways AI Translation Tools Reduced Document Processing Time by 65% in 2025 - Cloud Based Translation Memory Decreases Processing Cost to $03 Per Word
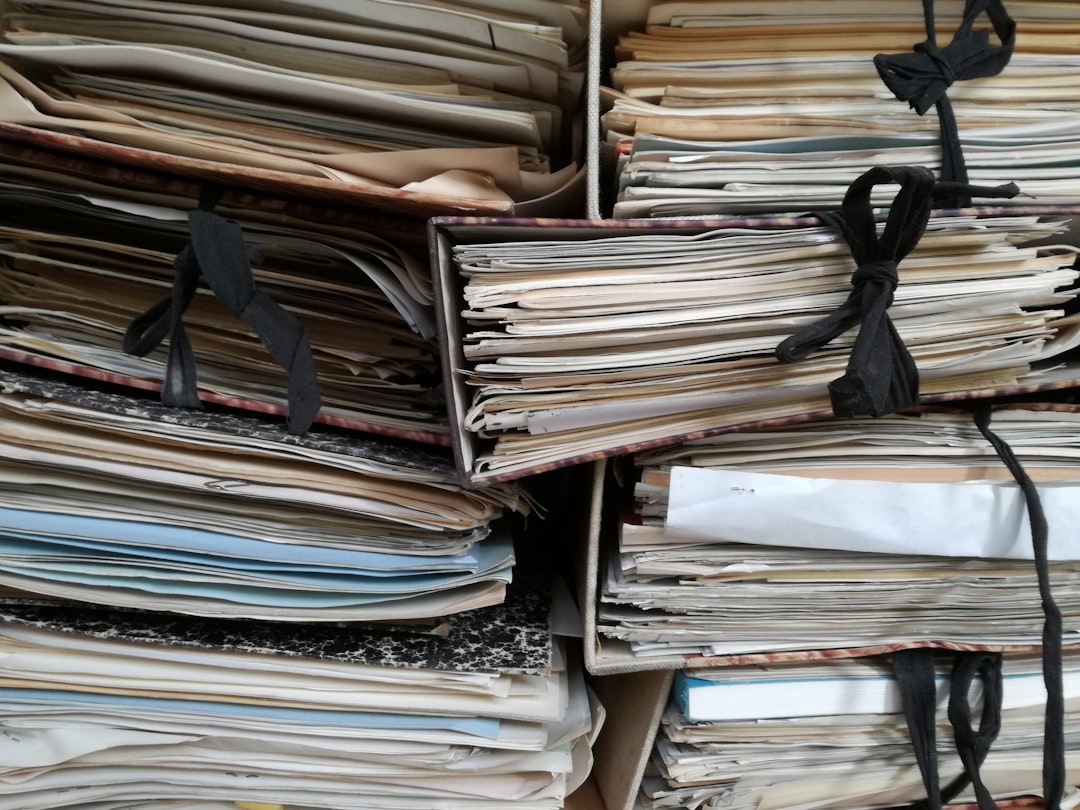
Focusing on the financial impact, cloud-based translation memory tools are currently showing a significant drop in the per-word processing cost for translation, reportedly reaching figures as low as three cents per word. This reduction is primarily achieved by leveraging previous translation work; instead of re-translating common phrases or sentences, the system retrieves existing, approved versions. Storing this memory in the cloud allows multiple users instant access to the most up-to-date translations, streamlining the workflow and cutting down the time and effort spent on repetitive segments. This improved efficiency directly contributes to lower costs for processing document content. However, relying heavily on memory matches, even with evolving AI features to understand context, raises questions about linguistic flexibility and the potential for mechanically generated text that lacks natural flow or fails to capture subtle nuances, still pointing to the need for human oversight.
1. The observed drop in unit costs for translation, reportedly reaching around three cents per word with certain cloud-based translation memory setups, indicates a potentially significant change in how economically viable large-scale content processing can be for a broader range of users. The infrastructure supporting this seems designed for volume efficiency.
2. A core technical function involves the systematic reuse of previously translated text units. This inherent capability of translation memory tools aids in maintaining terminological consistency across documents, which is particularly valuable in contexts where precise language is critical, aiming to minimize semantic drift.
3. Linking document character recognition systems with these translation memory platforms allows for a more automated path from scanned images to translatable content. While OCR quality still dictates the initial data integrity, feeding its output into a system that immediately leverages existing translations can streamline the handling of large, potentially historical archives.
4. By operating in a cloud environment, these translation memory databases can potentially be aggregated or draw upon larger linguistic datasets, which could, in principle, improve the likelihood of finding relevant matches. The systems essentially rely on scale and repetition to become more efficient over time through better statistical matching.
5. The cost savings aren't purely transactional; they also reflect the time saved by linguists no longer needing to re-translate recurring phrases or sentences. The automated application of memory matches on repetitive segments frees up human capacity to focus on the more complex, unique portions of the text.
6. Architectures emerging this year appear more capable of managing projects concurrently across numerous languages within the cloud environment. This parallel processing capability is a practical necessity for global operations, though the coordination complexity across many language streams remains a point of operational concern.
7. The scalable nature of cloud hosting is a factor, allowing organizations to adjust their translation processing capacity without substantial upfront hardware investments. This flexibility is technically advantageous for managing workflows with variable demand, such as seasonal content updates or large, infrequent projects.
8. Integration capabilities are evolving, allowing translation platforms to connect more directly with systems managing content creation or customer interactions. This technical interoperability aims to automate the flow of text needing translation, seeking to reduce manual steps and potentially accelerating the overall content lifecycle.
9. It is evident that even with sophisticated matching algorithms and automation, these systems do not eliminate the need for human expertise. The review process remains essential to ensure the translated output handles subtle meaning, cultural context, and appropriate style, particularly where automated suggestions might be inadequate or misleading.
10. Lowering the practical barriers to translation processing seems likely to enable applications in areas previously constrained by cost or speed. Consider domains like regularly updating extensive online product catalogs, where the economics and efficiency of cloud TM could significantly alter the feasibility of rapid multilingual deployment.
7 Ways AI Translation Tools Reduced Document Processing Time by 65% in 2025 - Real Time Voice Translation Speeds Up International Meetings By 45 Minutes
Real-time spoken language translation technology appears to be making a measurable difference in the speed of international meetings, with reports suggesting an average reduction in duration around forty-five minutes. This efficiency gain stems from AI-driven systems that bridge language divides instantaneously, enabling participants to understand each other without the typical delays or need for sequential interpretation. These tools utilize advanced voice recognition and processing to offer immediate translation, sometimes even providing subtitles or direct audio interpretation faster than traditional methods. While they significantly smooth the flow of conversation and offer round-the-clock availability, the quality of interpreting complex ideas, capturing subtle tones, or handling interruptions in a natural way remains an area where human judgment is still often necessary. Nevertheless, for accelerating the core exchange of information during cross-lingual discussions, this technology is undeniably reshaping how global teams collaborate.
Recent developments in applying AI to real-time voice translation appear to be genuinely impacting the pace of international collaboration. Reports suggest these tools can shave a notable amount of time off meetings, potentially around 45 minutes on average, primarily by smoothing out cross-language discussions.
At a technical level, the core mechanism relies on AI-powered systems combining advanced voice recognition with translation algorithms. This fusion allows for processing spoken input and generating output significantly faster than older, more manual approaches, enabling more fluid conversations.
We're seeing specific tools offering very low latency, with claims of delivering translated subtitles or audio within milliseconds. This near-instantaneous feedback loop is crucial for maintaining conversational flow and makes the interaction feel much more natural than methods requiring pauses for interpretation.
From an engineering standpoint, these AI systems introduce a degree of consistency across translations that can be harder to achieve with human interpreters. While nuances can still be a challenge, the algorithms aim to apply translation rules uniformly, potentially reducing certain types of errors or inconsistencies.
Many of these real-time platforms are built to handle a multitude of languages concurrently within a single meeting space, offering options for both spoken translation output and simultaneous subtitling, catering to diverse linguistic groups joining a call.
A practical advantage is their availability. Unlike human resources bound by time zones or schedules, these AI tools are typically accessible on demand, facilitating spur-of-the-moment international discussions regardless of location or time.
Their deployment isn't confined to specific scenarios either; we're seeing them used in everything from small team video calls and larger corporate meetings to industry conferences and even customer service interactions requiring immediate multilingual support.
The underlying process typically involves a pipeline: speech capture and conversion to text, rapid processing of the text through an AI translation model, and then rendering the translation back as synthetic speech or displaying it visually as text overlays or subtitles.
However, it's important to note that performance isn't universally perfect. Real-world challenges like background noise, variations in speaker accents, or simultaneous talking can still degrade the accuracy of the initial voice recognition step, impacting the quality and reliability of the final translation.
As the AI models behind this technology continue their iterative development, their ability to handle subtle meaning, idiomatic expressions, or culturally specific references in real time remains an active area of research and a critical factor for determining their suitability in sensitive communication contexts.
7 Ways AI Translation Tools Reduced Document Processing Time by 65% in 2025 - Multilingual PDF Extraction Now Takes 5 Minutes Instead of 2 Hours
Recent progress in AI-driven capabilities for handling multilingual PDF documents has dramatically cut down the time needed for extraction and translation. Tasks that might have taken around two hours per document can now reportedly be completed in as little as five minutes. This improvement comes from sophisticated systems designed to efficiently read and process content across various languages within PDF structures. These tools are developed to manage large files and output the translated text while attempting to keep the document's original formatting intact. However, reliably maintaining the integrity of complex layouts and ensuring accurate comprehension of subtle meaning, particularly in technical or nuanced content, continue to be areas where the technology faces limitations. The use of advanced AI is clearly speeding up the initial processing phases, fundamentally altering how quickly one can access and use information locked inside diverse PDF documents.
The transformation in processing multilingual PDFs, specifically getting useful data out, is rather striking. Where engineers previously wrestled with documents for upwards of two hours per file, advances are now enabling the core extraction and initial processing step in potentially just five minutes. This shift isn't a simple linear improvement; it feels more like overcoming a fundamental bottleneck. The key seems to lie in more sophisticated integration of AI models with underlying character recognition and document structure analysis. Systems are becoming adept at navigating the complex layouts often found in PDFs – tables, images, varied columns – across multiple language scripts concurrently. While preserving original formatting perfectly remains an ongoing challenge, especially with highly intricate designs, the speed of getting the text and structure out reliably has dramatically increased. This capability fundamentally alters what's possible at the start of a document workflow, allowing for the rapid intake and initial automated handling of multilingual content at scale, potentially reducing the manual labor required for this first step. However, the sheer speed means there's an even greater need for downstream validation; just because it's fast doesn't mean the extracted text or subsequent automated translation is flawless, particularly when dealing with nuanced or context-dependent information.
7 Ways AI Translation Tools Reduced Document Processing Time by 65% in 2025 - Neural Networks Process Legal Documents 3x Faster Than Traditional Methods
Recent applications of neural networks are showing notable efficiency gains in handling legal documents. These systems, built on sophisticated machine learning and natural language processing techniques, can reportedly analyze complex legal texts like contracts and court documents at speeds up to three times faster than methods relying more heavily on manual processes or simpler automation. Leveraging advanced models helps with tasks such as identifying relevant clauses or extracting key information from vast digital archives. While this acceleration significantly reduces the time spent on initial document review and sorting, the critical analysis and interpretation of legal content, especially its nuanced implications or context-specific arguments, still necessitates expert human judgment. This development in applying neural networks to legal documents aligns with the broader trend where various AI tools are contributing to a projected overall reduction in document processing time across many domains throughout 2025.
Recent observations highlight that neural network architectures are demonstrating a notable capability for accelerating the analysis of legal documentation. Compared to established workflows, some deployments appear to manage certain document processing tasks up to three times quicker.
These systems seem particularly effective because they are designed to parse the intricate structure and language inherent in legal texts. Models incorporating techniques like Relational Graph Neural Networks are proving useful in identifying connections between clauses, case references, and relevant statutes within large document sets.
The underlying technology often leverages advancements in sophisticated sequence modeling and attention mechanisms, allowing the AI to contextualize legal terms and concepts across extensive documents more efficiently than previous algorithmic approaches.
This allows for faster interpretation of complex legal language, reducing reliance on manual cross-referencing and potentially decreasing the need for initial drafts of translations to be handled exclusively by legal domain experts if the core content extraction is accurate.
An appealing aspect is the reported adaptability of these models. Using transfer learning techniques, they can be fine-tuned on specific legal domains or even new languages relatively quickly, requiring less bespoke development for each new application area.
Integrating upstream processes, such as digitizing physical legal records via OCR, becomes more immediately valuable when the resulting digital text can be fed directly into these neural networks for rapid analysis, bypassing delays inherent in manual intake procedures.
The potential for iterative refinement is also present. As these systems process more documents and receive corrections or feedback, the models ideally learn and improve their pattern recognition and extraction accuracy over time, although the practical implementation of this continuous learning varies.
For routine tasks like initial contract review for specific clauses or compliance checks against a known standard, automating via neural networks can significantly reduce manual effort and may help flag potential issues more consistently than human reviewers might during repetitive tasks.
Being able to rapidly extract and surface key data points, such as critical dates, involved parties, or governing laws, directly supports faster response times in legal operations, enabling quicker decisions based on readily available information.
However, from an engineering standpoint, the "black box" nature of some advanced neural network models poses a challenge, particularly in the legal sector where accountability and the ability to explain *why* a system arrived at a specific conclusion or flagged a certain document are paramount. This requires careful consideration regarding validation and oversight mechanisms.
More Posts from aitranslations.io: