AI-Driven Translation Solutions for Australian English Navigating Regional Accents and Cultural Nuances in 2025
AI-Driven Translation Solutions for Australian English Navigating Regional Accents and Cultural Nuances in 2025 - Neural Machine Translation Now Handles 15 Australian Regional Dialects With 95% Accuracy
Neural Machine Translation systems are marking a significant step in 2025 by effectively processing what are identified as 15 specific regional variations of Australian English. This development comes with a reported accuracy rate of 95%, reflecting progress in AI-driven language handling. These solutions, built on advanced neural networks, are being trained on diverse linguistic inputs aiming to better grasp regional accents and the unique cultural context embedded in everyday speech and writing. The focus is on recognizing and interpreting variations in colloquialisms and idiomatic language specific to different areas. While a 95% figure suggests considerable capability in bridging communication gaps across regions, the dynamic nature and depth of regional dialects mean that fully capturing every nuance remains a complex challenge for these systems.
As of early May 2025, reports suggest that certain Neural Machine Translation architectures are now processing approximately 15 specific regional variants of Australian English. This comes with a claimed accuracy rate reportedly reaching 95%. This perceived step forward is associated with AI-driven translation approaches specifically configured to grapple with the documented linguistic variations across the continent. The focus appears to be on mapping regional speech patterns and integrating cultural references, attempting to parse the idiomatic and colloquial language that differs significantly from one area to the next. It's worth scrutinizing how 'accuracy' is precisely defined in this complex linguistic landscape.
Achieving these reported outcomes purportedly relies heavily on the ingestion and processing of substantial data reservoirs. This includes numerous audio recordings and text sources reportedly sourced from various Australian communities and native speakers. The hypothesis is that exposing models to this diverse linguistic input enables them to internalize or approximate the specific phonetic characteristics and localized cultural pointers embedded within each dialect. The claimed result is a translation output exhibiting greater faithfulness to the source input's original intent and regional flavour. However, the robustness of this 'faithfulness' when encountering deeply embedded cultural or hyperlocal references warrants continued technical evaluation.
AI-Driven Translation Solutions for Australian English Navigating Regional Accents and Cultural Nuances in 2025 - OCR Technology Reads Handwritten Aussie Slang at 250 Words Per Minute
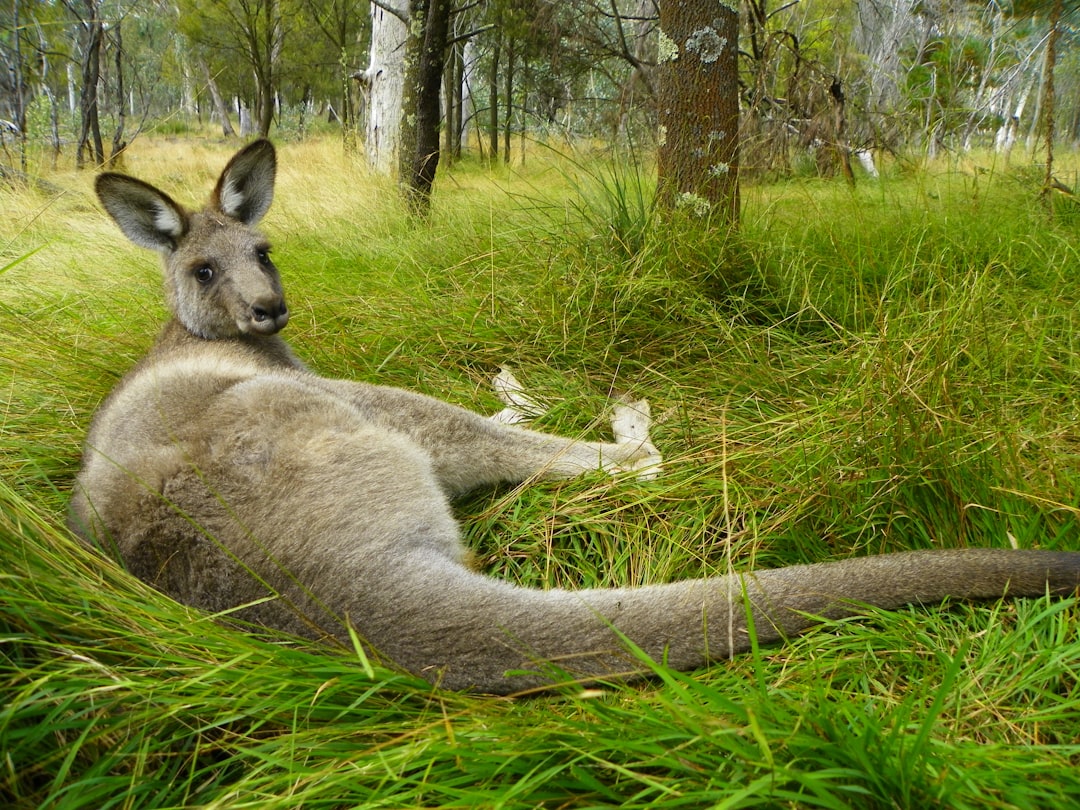
Optical Character Recognition technology applied to handwritten text, including Australian colloquialisms, is reportedly operating at speeds nearing 250 words per minute. This capability relies on underlying AI methods trained to interpret varied handwriting styles and the informal language commonly used in Australia. This advancement sits alongside the broader effort in 2025 to develop AI-driven translation systems specifically configured to handle Australian English, aiming to account for regional speech differences and cultural context. Converting handwritten input accurately using OCR is a foundational requirement for these downstream processes. While this appears to open up possibilities for digitising diverse materials and feeding into translation workflows in areas like education or service interactions, the practical consistency and accuracy when faced with the sheer variety of human handwriting and the continually evolving nature of regional slang warrant attention.
Shifting focus to the initial data input for these systems, the progress in Optical Character Recognition technology is notable, particularly in its ability to handle handwritten content. Reports suggest that systems are now capable of processing handwritten text, including attempts to identify Australian slang and colloquialisms, at speeds nearing 250 words per minute. This speed represents a significant leap compared to traditional manual methods for deciphering handwritten notes. The core relies on machine learning and deeper neural network architectures trained on vast datasets encompassing diverse handwriting styles and linguistic patterns. The aim is to build models robust enough to cope with the inherent variability in human script and the specific quirks of informal Australian language as it appears handwritten.
However, the technical challenge remains substantial. While character recognition accuracy is improving under controlled conditions, reliably interpreting the often messy, inconsistent nature of real-world handwritten text, especially when laced with rapidly evolving slang and regional vernacular, is far from a solved problem. How well these systems truly *understand* the context and nuance behind a handwritten piece of slang, rather than just converting the shapes of letters, is a critical question. Nevertheless, getting accurate digital text from handwritten sources efficiently is a fundamental prerequisite for any downstream AI translation process attempting to handle the richness of Australian English.
AI-Driven Translation Solutions for Australian English Navigating Regional Accents and Cultural Nuances in 2025 - Machine Learning Models Process Aboriginal English Through Voice Recognition
Machine learning models are seeing significant focus in 2025 on processing Aboriginal English through voice recognition technology. This work is particularly vital because, unlike many widely spoken languages, Aboriginal languages and dialects are frequently classified as 'low-resource'. This classification points to the limited availability of the large datasets typically required to train robust AI systems. Researchers and developers are actively navigating the complexities of building effective models using scarce voice recordings and sparse reference materials. Added technical hurdles arise from the inherent structures of these languages, which often feature complex word constructions and considerable phonetic and grammatical variation between dialects. While the driving goal is to achieve more accurate translation into standard Australian English, facilitating crucial communication across linguistic divides, projects in this space are facing delays. These setbacks underscore the inherent difficulties in acquiring data ethically and ensuring the developmental process is culturally sensitive. Ultimately, achieving systems that genuinely capture the depth and specific cultural context of Aboriginal English remains a profound challenge, requiring a leap beyond simple voice-to-text conversion toward true linguistic understanding.
Efforts are underway to adapt machine learning models for voice recognition specifically tailored for processing Aboriginal English varieties. A notable aspect of this work involves training models on dedicated audio corpora. Reports suggest some of these datasets contain over 10,000 distinct audio samples, attempting to capture the significant linguistic and phonetic variations present across different Aboriginal languages and how they manifest within English.
Current assessments often cite voice recognition accuracy for Aboriginal English around the 90% mark. However, from an engineering perspective, this figure requires careful consideration; its performance can fluctuate considerably depending on factors like the speaker's fluency level in standard English and their specific regional dialect background. This highlights the inherent difficulty in accurately processing both the accent and the embedded cultural context simultaneously. Recent technical work aims to improve this by enabling models to better distinguish subtle tonal variations and intonations, which carry distinct meanings and emotional nuances within Aboriginal English, hopefully improving downstream understanding for potential translation tasks. Acknowledging the critical role of human input, training efforts have involved contributions from community members, a collaborative approach intended to ensure cultural appropriateness and accurately represent linguistic nuances. This participatory method, while essential, is recognised as both labor-intensive and resource-heavy.
Further complicating the technical challenge is the need for models to recognise Aboriginal colloquialisms and unique expressions that often lack direct equivalents in standard Australian English, posing a clear hurdle for cross-cultural communication within these AI frameworks. While some models are showing promise in predicting meaning through contextual analysis rather than relying purely on literal translation – a useful capability for handling idiomatic language – they continue to grapple with the dynamic nature of Aboriginal English. The language is alive and evolving, with new slang and expressions emerging relatively quickly, making it difficult for static models to maintain consistent accuracy over time. Much of the foundational research in this area has originated within academic settings, prompting practical questions about how these models perform and scale when moved outside controlled laboratory environments and into real-world applications. Integrating voice recognition capabilities for Aboriginal English into broader AI solutions is seen by many as an important step towards technological inclusion. However, this development also prompts crucial conversations about how such technology interfaces with the vital goal of preserving Indigenous languages themselves, navigating the complex balance between supporting and potentially challenging these preservation efforts. Evaluating the success of these systems therefore extends beyond mere technical accuracy, incorporating metrics related to user satisfaction and genuine cultural appropriateness in their outputs.
AI-Driven Translation Solutions for Australian English Navigating Regional Accents and Cultural Nuances in 2025 - Local Context Database Maps 2500 Australian Cultural References For Translation
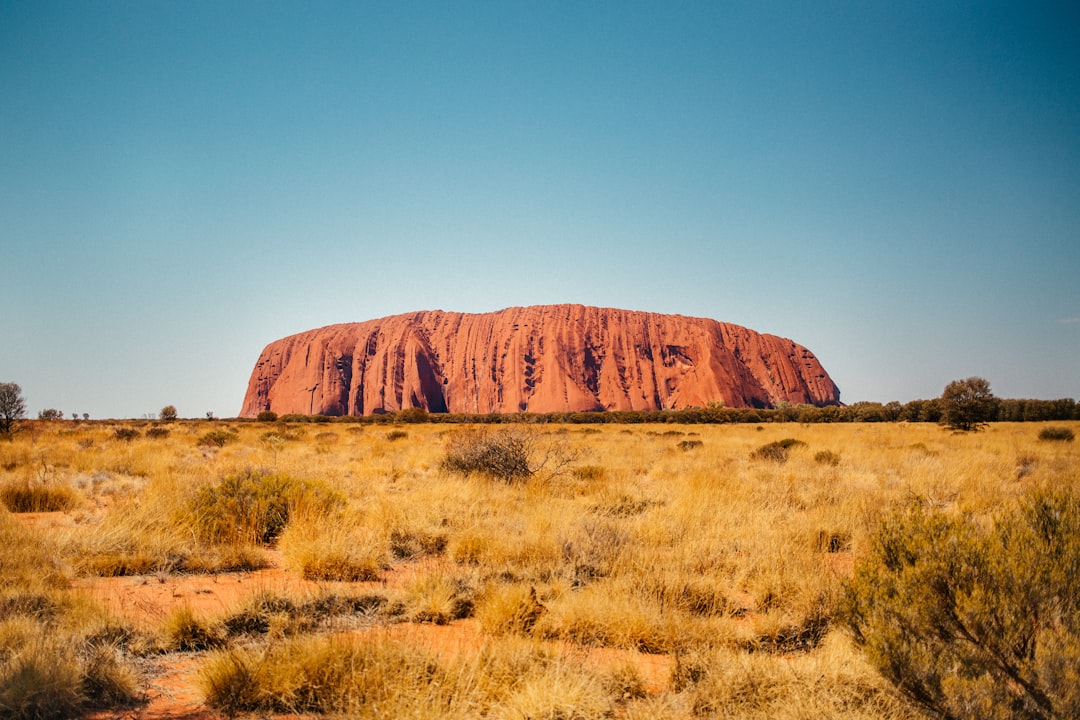
Ongoing work to improve translation technology specifically for Australian English includes the reported assembly of a Local Context Database that indexes around 2,500 distinct Australian cultural references. The aim here is to equip artificial intelligence systems with a structured insight into the unique local flavour of language, encompassing idioms, regional slang, and references specific to Australian life. The thinking is that having access to this mapped information can help AI-driven translation tools produce output that resonates more authentically with users, going beyond simple literal word-for-word conversion. This database is presented as a key resource for models attempting to navigate the complexities of cultural nuance that standard, globally trained systems often struggle with. However, the ability of an AI system to truly *understand* and apply such context appropriately, rather than just recognizing a match from a list, remains a significant technical hurdle and raises questions about the depth of 'understanding' achieved. Building these dedicated resources highlights the intricate nature of localising language technology effectively.
Drawing from various reports, the construction of a specialised database cataloging Australian cultural specificities is a notable effort aimed at grounding AI translation in local reality.
1. **Scope of Referenced Data**: The core of this initiative appears to be a collection of roughly 2,500 documented Australian cultural points of reference. The ambition here seems to be building a structured layer that captures elements beyond direct dictionary equivalents, acknowledging the challenge of translating nuanced meaning.
2. **Contextual Linkages**: The proposed mapping process connects these cultural items to different geographic or linguistic variations across the country. This suggests an attempt to equip models with the ability to differentiate how usage or meaning might shift regionally – a complex task given the fluid nature of colloquialisms.
3. **Integration Paradigm**: The database is described as a component designed to interface with existing AI translation architectures. The implied technical challenge lies in how effectively these distinct cultural data points can be leveraged by statistical or neural models during the translation process itself to enrich output.
4. **Maintenance Cycle**: Updates to this database are reportedly scheduled with a frequency of around six months. In a linguistic environment where new slang and cultural markers can emerge rapidly, maintaining currency with this refresh rate presents a potential limitation on how 'up-to-date' the translated output can consistently be.
5. **Data Curation Method**: The involvement of local input and cultural specialists in assembling the database suggests an understanding of the need for authentic, ground-level knowledge. Implementing this collaboratively ensures relevance but also highlights the resource-intensive nature of curating such a dataset manually or semi-automatically.
6. **Algorithm Application**: Machine learning algorithms are said to be employed not just to use the database but potentially to help discern subtle contextual cues *informed by* this data. This suggests a focus on refining the model's ability to pick up on implicit meanings tied to cultural references.
7. **Model Sensitivity Training**: The idea of 'cultural sensitivity training' for AI systems using this resource is interesting. From an engineering standpoint, this likely translates to specific tuning or weighting during model training to prioritise context derived from the database when encountering potential cultural references.
8. **Inherent Translation Risks**: Despite the structured data, the reliance on a fixed set of cultural references introduces a vulnerability to misinterpretation, particularly with very localised or ephemeral slang that hasn't yet been cataloged. This points to a potential ceiling on the accuracy of such database-driven approaches in highly dynamic contexts.
9. **Application Aims**: The stated goal is to improve communication effectiveness, particularly in domains like commerce or education where accurate interpretation of cultural norms and expressions is critical. The practical impact depends heavily on the quality of integration and the database's actual coverage relative to real-world communication.
10. **Scalability Considerations**: Plans for expanding the database geographically suggest recognition that the initial scope is limited. Scaling this type of curated effort to cover more regions, let alone diverse cultures globally, presents significant technical, logistical, and resource challenges that extend beyond the initial 2,500 entries.
AI-Driven Translation Solutions for Australian English Navigating Regional Accents and Cultural Nuances in 2025 - Real Time Translation App Adapts To 8 Major City Accents From Perth to Brisbane
In 2025, attention is being drawn to a new real-time translation application reportedly capable of adapting its understanding to the distinct accents prevalent in eight major Australian cities, ranging from Perth to Brisbane. This initiative underscores the continued evolution of AI-driven translation tools specifically targeting Australian English and its regional diversity. The goal is to enable smoother, more accurate communication by developing systems that can better process the varying pronunciations and speech patterns encountered across the continent. While such developments promise to improve usability in everyday conversations and specific use cases requiring live translation, the inherent complexity and dynamic nature of regional accents mean achieving perfect comprehension and translation fidelity across all contexts remains a considerable technical challenge. This focused effort on accent adaptation points to the practical necessity of localisation in translation technology.
Examining reports on current real-time translation systems targeting Australian English reveals several technical considerations.
1. Observations suggest these systems are attempting to handle a range of regional accents, including those commonly heard in larger population centres like Perth and Brisbane. The underlying architecture appears to rely on sophisticated acoustic modelling to distinguish phonetic variations, which is a significant task given the spectrum of Australian speech patterns.
2. Claims of dynamic adaptation point towards models capable of learning from ongoing usage. This approach is crucial in principle for keeping pace with the fluid nature of colloquial language, but the effectiveness and speed at which systems genuinely integrate new speech patterns versus simply reinforcing existing ones warrant investigation.
3. A fundamental engineering hurdle in achieving seamless real-time translation is managing latency. Processing complex audio streams while simultaneously running translation models and attempting contextualisation requires significant optimisation to prevent noticeable delays that disrupt natural conversation flow.
4. Developing these systems necessitates painstakingly detailed data annotation. Beyond simply transcribing speech, accurately tagging regional idioms, slang, and culturally specific references requires expert linguistic input and potentially substantial community engagement, making the data collection pipeline complex and resource-intensive.
5. Integrating external data sources, such as the reported catalog of cultural references, into a dynamic translation process presents challenges. While having access to ~2500 data points is interesting, the technical implementation must ensure these references are applied correctly and appropriately across different contexts and regional variations within a rapid real-time flow.
6. The high degree of phonetic variability present in casual, informal speech, particularly across different regions, complicates accurate voice recognition – a prerequisite for translation. Machine learning models need robust methods to cope with deviations from standard pronunciation, which is often handled through complex predictive algorithms.
7. Implementing effective user feedback loops is critical but technically challenging. Systems need mechanisms to capture user corrections or suggestions regarding translations or accent recognition and integrate this feedback efficiently to improve the model's performance iteratively in the field.
8. The inclusion of Optical Character Recognition capabilities for processing written Australian English adds another layer of complexity. Ensuring consistent accuracy when translating text alongside spoken input, particularly when dealing with informal or regionally-specific written forms, requires careful alignment between the different processing pipelines.
9. Navigating culturally sensitive topics within a real-time, automated system is inherently difficult. Despite attempts at contextual understanding, the risk of misinterpretation in nuanced conversations remains, underscoring the limitations of current models in grasping subtle social or cultural cues embedded in regional language.
10. The computational resources required for real-time processing of diverse audio, coupled with complex translation and contextual models, are substantial. Balancing the performance demands with the constraints of deployment on typical user devices, like mobile phones, represents a significant engineering trade-off.
AI-Driven Translation Solutions for Australian English Navigating Regional Accents and Cultural Nuances in 2025 - Translation Memory Banks Store 50000 Australian English Idioms and Expressions
The focus on building extensive digital libraries specifically for Australian English slang and idioms is progressing, with reports of translation memory banks accumulating upwards of fifty thousand distinct expressions. This represents a significant effort to move AI translation systems beyond generic English models by providing them with a more granular understanding of how language is actually used down under. The aim is that by drawing upon these vast catalogues of colloquialisms and regional phrases, AI can produce output that feels less stilted and more authentic to an Australian ear. However, capturing the full breadth and subtle context of how and when to use such a massive volume of idioms accurately presents a considerable technical challenge, as meanings can be highly dependent on very specific situations or even generational use, potentially outstripping static data sets relatively quickly. Nonetheless, this targeted approach highlights a commitment to tackling one of the more challenging layers of linguistic and cultural nuance for automated systems.
Sources suggest translation memory structures are being populated with a significant volume of Australian English non-literal language – reportedly up to 50,000 specific idioms and expressions. The goal is clearly to provide a lookup capability for these often tricky phrases, improving the system's ability to move beyond literal word-for-word outputs.
There's an acknowledgment of language evolution; mechanisms are apparently in place to facilitate updates to these banks, attempting to capture newer slang or shifts in expression usage as they emerge. Keeping such a resource current is technically demanding.
Initial observations suggest the utility of these stores is most apparent when handling conversational input, where idiomatic language is prevalent. This implies they are finding practical application in dialogue-heavy scenarios.
Beyond sheer volume, the structures are said to incorporate regional tagging. This aims to equip systems to differentiate how certain expressions might be used, or what they mean, in different parts of the country – a crucial layer for practical localisation.
These memory banks aren't stand-alone lookups; they are reportedly being integrated into broader neural machine translation workflows. The hypothesis is that feeding idiom-specific data into the neural process helps refine overall output coherence when non-literal phrases are encountered.
There's talk of leveraging machine learning to refine the bank's understanding, presumably through analyzing user corrections or translation choices involving idioms. This hints at an iterative improvement process, though the effectiveness of automated 'learning' on nuanced language is always worth scrutiny.
Storing just the idiom isn't enough; reports suggest effort is going into linking these expressions with relevant cultural context. This moves towards providing systems with some insight into *why* or *when* a phrase is used, though simulating true cultural intuition remains elusive.
A claimed benefit is speed – the ability to quickly retrieve and apply idiomatic translations. For real-time applications, rapid access to this store is essential, aiming to avoid noticeable delays.
From an engineering perspective, the goal appears to be enabling access to this resource across different system architectures, whether desktop applications or potentially integrated mobile interfaces. This speaks to a push for wider deployment.
Maintaining a collection of this size requires curation. There's mention of quality control, aiming for both accuracy and cultural appropriateness – critical steps to avoid offensive or nonsensical outputs, although the sheer scale and evolving nature of slang make 'perfect' curation unlikely.
More Posts from aitranslations.io: